32 Clinical Trial Design and Analysis
Trial Design
Randomized Clinical Trials
To better understand differences in trial design, it is often helpful to distinguish explanatory RCTs and pragmatic RCTs.1–3 Trials of new drugs, such as those designed for drug registration, aimed at showing efficacy and short-term safety, belong to the group of explanatory RCTs. In general, all elements of trial design, such as selection of patients, sample size, choice of the comparative intervention, and duration of the trial, are chosen in such a manner that the trial can optimally demonstrate a treatment effect, that is, a difference in efficacy between the new drug and the control intervention. The methodologic robustness of a trial, which is dependent on these elements of trial design, is referred to as internal validity. Explanatory trials do not always resemble clinical practice. As an example, they often include for methodologic reasons patients with a high level of disease activity who form only a minority in clinical practice. The extent to which clinical trial results can be extrapolated to the common clinical practice is referred to as external validity. As a rule of thumb, explanatory trials have a high level of internal validity, which may, however, jeopardize external validity to some extent. Pragmatic trials more closely resemble the clinical situation. Such trials aim to optimize treatment by further exploring existing drugs or treatment strategies. Pragmatic trials incorporate fundamental principles of RCTs, such as randomization, but include a more realistic representation of patients, may have a longer duration, and may allow co-interventions. In general, pragmatic trials have a lower level of internal validity as compared with explanatory trials, but a higher level of external validity. Often, explanatory trials are initiated and sponsored by pharmaceutical industry, but most pragmatic trials are (academic) investigator-driven initiatives.
Design Considerations
In a superiority design, the question is whether the new treatment is more efficacious than the control intervention (e.g., placebo). Formally, such a study tests whether the null hypothesis of no difference between both treatment groups can be rejected. To do so, investigators agree on a minimally clinically important difference (MCID) between the intervention of interest and the control intervention such that a study should be able to demonstrate, and they design the study in such a way that this difference can be demonstrated with high likelihood (statistical power) when it really exists (see later). In a noninferiority design, the reasoning is opposite. The null hypothesis is that the new treatment is less efficacious than the control intervention.4,5 Even if the new intervention and the control intervention are truly similarly effective, a trial will almost never yield a result with a treatment effect of exactly zero (no difference). There will be variation around zero, and it is the task of investigators to decide in the design phase of the study which deviation from a treatment effect of zero they will accept to conclude that the interventions are equivalent, the noninferiority margin. Determination of the MCID in a superiority design and the noninferiority margin in a noninferiority design is a subjective decision with important consequences for the sample size. When it is important in a superiority design to be able to demonstrate very small treatment effects with a high likelihood, large sample sizes are needed; the same is true with a very narrow noninferiority margin in a noninferiority design. Especially with a noninferiority design, considerations other than efficacy alone may give guidance to the level of the noninferiority margin. If a new drug is less toxic or less costly than existing drug(s) on the market, and as such may provide additional benefits, one could be more lenient with regard to determining the noninferiority margin. In general, noninferiority designs require (far) more patients than are required by superiority designs.
Subject Selection
Subjects who are entered into clinical studies should meet accepted criteria for the disease or disorder under study. Most rheumatologic conditions lack single and unequivocal diagnostic tests, and classification criteria have been developed to identify patients with similar characteristics.6 These classification criteria serve as eligibility criteria in an RCT. To homogenize patient populations for scientific purposes, classification criteria are designed to be highly specific. As a consequence, sensitivity may fall short, and classification criteria are often of limited use in diagnosis. The high specificity of classification criteria has implications for the makeup of the trial population. In general, patients with classic, often severe disease are overrepresented, and those with early, less typical disease are underrepresented.
Informed Consent
Ethical considerations determine whether eligible subjects participate in a clinical trial. Governmental agencies of most countries require that institutions involved in human research have a local institutional review board (IRB). The IRB reviews all protocols before implementation and monitors ongoing studies at its institution. A crucial element in the review of a trial is the informed consent process.7 The consent form should explain to the study participant the purpose of the study, all potential benefits and risks (including risks to pregnant mother and fetus), alternatives to participation, and who is responsible for conducting the study. Patient confidentiality should be ensured. The consent form should clearly state that participation is completely voluntary, and that refusal to participate or withdrawal from the study will not affect future care. If compensation is provided, this must be documented in the consent form. Participants should be given contact information for questions or in case of injury and a statement about whether any medical treatment will be given if injury occurs. Investigators are responsible for ensuring that the risk to subjects is minimized and appropriate for the anticipated benefits.
Choice of Outcome Variables
The Outcome and Measurement in Rheumatology Clinical Trials (OMERACT) initiative was created to bring unanimity to the multitude of outcome measures in rheumatology on the basis of expert consensus.8 Its activities were initiated in RA and were expanded to include most other rheumatologic diseases. The OMERACT framework is the so-called OMERACT filter, which describes the methodologic prerequisites that an appropriate outcome measure should fulfill to be considered valid for clinical trials. The OMERACT filter prescribes three validation requirements: An outcome measure should be truthful, discriminatory, and feasible.
Increasingly, indices are replacing single-outcome variables in rheumatology. An index is a weighted or unweighted combination of single variables that together reflect a particular domain of outcome.9 A general rule is that indices perform better than single-item variables only if they consist of variables that correlate moderately with each other. If variables correlate at a too high level, there is redundancy of information. If variables do not correlate, they will reflect different domains; this complicates interpretability, and it is better to separately describe them. Important examples of useful indices in rheumatology are the already mentioned disease activity score (DAS),10 the ankylosing spondylitis disease activity score (ASDAS),11 and the American College of Rheumatology (ACR) response criteria in RA.12
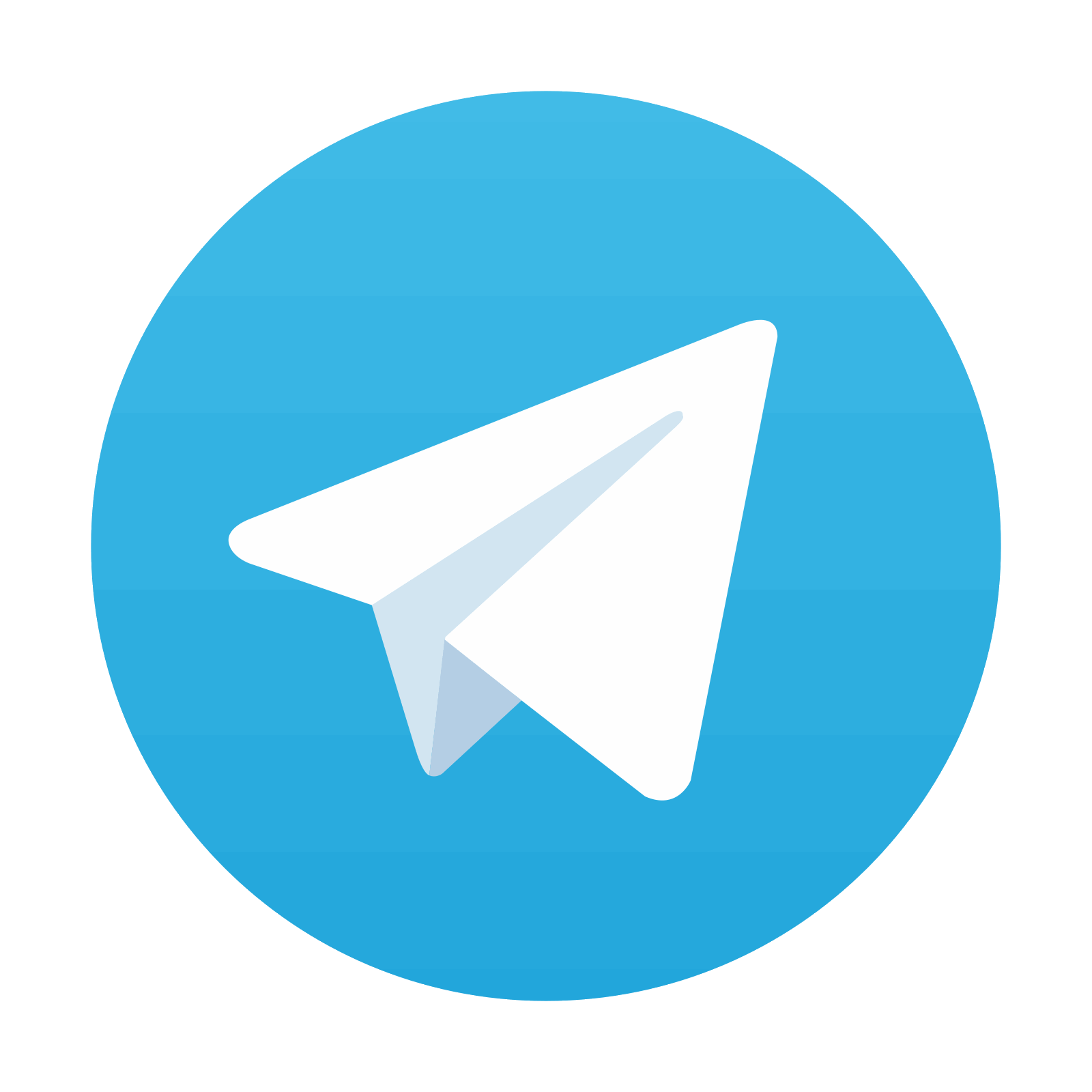
Stay updated, free articles. Join our Telegram channel

Full access? Get Clinical Tree
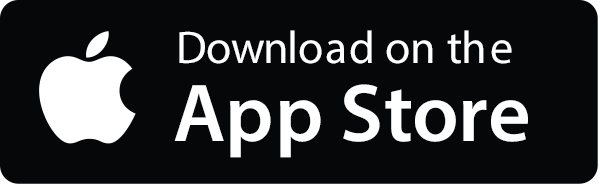
