This article reviews neural interface technology and its relationship with neuroplasticity. Two types of neural interface technology are reviewed, highlighting specific technologies that the authors directly work with: (1) neural interface technology for neural recording, such as the micro-ECoG BCI system for hand prosthesis control, and the comprehensive rehabilitation paradigm combining MEG-BCI, action observation, and motor imagery training; (2) neural interface technology for functional neural stimulation, such as somatosensory neural stimulation for restoring somatosensation, and non-invasive cortical stimulation using rTMS and tDCS for modulating cortical excitability and stroke rehabilitation. The close interaction between neural interface devices and neuroplasticity leads to increased efficacy of neural interface devices and improved functional recovery of the nervous system. This symbiotic relationship between neural interface technology and the nervous system is expected to maximize functional gain for individuals with various sensory, motor, and cognitive impairments, eventually leading to better quality of life.
The primary goal of rehabilitation is to restore physical, psychological, and social functions and improve the quality of life for individuals with various motor, sensory, or cognitive impairments. Although treatment options vary from pharmacologic agents to physical exercise, this review article focuses on neural interface technology, also called neuroprosthetics, which functions by directly interacting with the nervous system either electrically or magnetically. More than 200 years ago, Luigi Galvani documented that leg muscle contraction can be elicited by electrical stimulation of the femoral nerve. However, it is only in the last several decades that neural interface technology started to receive increasing attention from biomedical engineers, neuroscientists, and clinicians, largely due to great advances in electronic medical devices and system neuroscience research. The US Food and Drug Administration (FDA) approved cochlear implants (CIs) for treating hearing loss in 1984 and deep brain stimulators (DBS) for treating Parkinson disease in 2000. The National Institutes of Health currently holds a biannual Neural Interface Conference with the goal of bringing industry partners, clinicians, and researchers together to discuss key issues and future directions related to neural interface technology.
This review classifies neural interface devices and systems into 2 categories, neural recording systems and neural stimulation systems, based on the direction of information flow. Neural recording systems retrieve information from the nervous system through electrophysiological recording methods, such as electroencephalography (EEG) and microelectrode recording of single-neuron activities. Neural stimulation systems feed information into the nervous system by electrically or magnetically activating or inhibiting neural activity. These 2 categories represent a simplified classification scheme, as certain neural interface devices, such as implantable responsive neurostimulators for epilepsy treatment, are capable of neural recording and stimulation. As the term neural interface technology covers a broad range of devices and systems, it is a daunting task to provide a detailed and informative discussion on each device and system in 1 review article. Many books, special journal issues, and review articles are excellent references for neural interface technology. Hence, this article reviews neural interface technology from 3 unique perspectives: (1) neural interface systems that are currently under active research but have not yet reached clinical practice, to illustrate not only what has been accomplished but also what will be accomplished in the near future; (2) neural interface technology with systems that the authors have directly worked with in their rehabilitation research and clinical practice to provide a first-hand view of neural interface technology; (3) neural interface technology in association with neuroplasticity, a foundation for neurologic rehabilitation, demonstrating that those 2 concepts work symbiotically to improve the quality of life for individuals with disabilities ( Fig. 1 ). Neuroplasticity will help those individuals to make better use of their neural interface devices, and neural interface technology can also promote neuroplasticity for functional recovery.
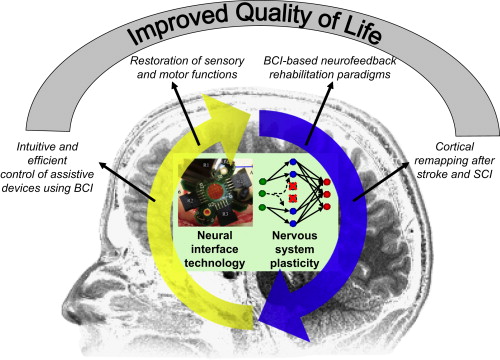
Neural interface technology for neural recording
This section focuses on the application of neural interface technology to support brain-computer interface (BCI) devices. The primary goal of BCI technology is to establish a direct communication pathway between the brain and external devices enabling faster and more intuitive communication and control for individuals with motor disabilities caused by stroke, spinal cord injury (SCI), limb amputation, and degenerative neurologic disorders. In this sense, BCI is a type of assistive technology, and what differentiates it from traditional assistive devices is that user commands are extracted directly from brain activity without the need for users to exert any overt movement. BCI systems, with the capability to provide real-time feedback of neural activity, have also received considerable attention as rehabilitation tools for stroke and SCI patients. The goal is to induce neuroplasticity through the operation of BCI devices for functional recovery instead of using BCI systems permanently as assistive technology. In a typical BCI setup, multiple channels of neural signals are recorded simultaneously and fed into a decoding module. The decoding module then processes and decodes neural signals in real time to extract a BCI signal that can be used to control movement of a computer cursor or other external devices, such as a robotic arm or a communication aid.
Three factors are critical for the success of BCI applications. The first is the neural substrate (ie, neural activities), which encode a certain type of information that can be decoded as a BCI control signal. Many BCI applications were developed based on cortical representations of movement. Motor system neurophysiology studies have shown that activity of motor cortical neurons is modulated by movement, and that firing rates of a population of motor cortical neurons can be used to predict hand movement direction, speed, and position. This provides a neural substrate for extracting movement signals from the brain. The second factor for success is cortical plasticity induced by training through biofeedback. When subjects are trained to operate a BCI system, visual or other sensory feedback of the decoded movement is presented to subjects in real time. It has been observed that, over time, modulation of recorded neural signals is enhanced as user performance improves. Neuroplasticity induced through BCI training not only helps BCI operation itself but also has the potential to promote functional recovery after damage to the nervous system from stroke and SCI. The third factor is an appropriate neural recording method that has sufficient spatial and temporal resolution to take full advantage of the aforementioned neural substrates and neuroplasticity. Various neural recording modalities have been used in BCI systems, including microelectrode recording of single-neuron activity, subdural electrocorticography (ECoG), scalp EEG, magnetoencephalography (MEG), and functional magnetic resonance imaging (fMRI). Depending on the targeted application, different BCI systems may have different requirements for the neural recording method. For example, a BCI system that acts as an assistive device needs to be portable, potentially fully implantable, and has the capability to record highly specific neural activity from a small cortical area. In contrast, portability may be less critical for a BCI system that serves as a rehabilitation tool if it can offer whole-head coverage with reasonable spatial and temporal resolution noninvasively, such as an MEG-based BCI system.
BCI as Assistive Technology
Considerable progress has been made using various neural recording methods, such as microelectrode recording of single-unit activities and scalp EEG. Each recording modality has its own advantages and disadvantages. Single-unit recording requires craniotomy and surgical insertion of microelectrode arrays into the cortex, and it has been suggested that microelectrode recording may lack long-term reliability due to foreign body reaction. In addition, single-unit recording typically requires sophisticated hardware and software systems. To capture the occurrence of spikes associated with action potentials, advanced data acquisition hardware with a high sampling rate (typically 25,000–50,000 Hz) is required. However, it offers the highest spatial and temporal resolution and can extract BCI control signals with multiple degrees of freedom with high accuracy. Researchers have demonstrated that nonhuman primates can achieve high-precision control of robotic arms to perform a self-feeding task, and clinical trials in human subjects are currently underway to test the safety and efficacy of BCI systems based on microelectrode recording of neuronal activity. At the other end of the spectrum, scalp EEG can be recorded noninvasively with much simpler recording systems (eg, sampling rate can be less than 1000 Hz), but it has a low spatial resolution (30 mm) and a low signal-to-noise ratio, especially at higher frequencies (>60 Hz) due to signal attenuation caused by the skull. It has been demonstrated that subjects can control the movement of a two-dimensional cursor with EEG by modulating their sensorimotor rhythm (10–30 Hz), but extensive training is often required.
In parallel with the work of other groups using microelectrode recording and scalp EEG, the authors have been developing BCI systems using ECoG and micro-ECoG. ECoG (sometimes also called intracranial EEG) recently received considerable attention as a promising modality for BCI application. ECoG recordings are often performed for patients with intractable epilepsy for presurgical brain mapping and seizure foci localization. Patients’ ECoG signals are recorded continuously for epilepsy monitoring, and those signals can be split off and fed into a second neural recording system for BCI research. Because its electrodes are placed inside the skull, ECoG preserves a wide range of high-frequency components (40–200 Hz) of the electrical field potential generated by neuronal activities. Several groups of researchers, including the authors, found that the power of high-frequency bands increases significantly during movement, and varies systematically with movement direction ( Fig. 2 ). The high-frequency band of ECoG signals encodes desired movement direction, similar to the high-frequency band of local field potential signals recorded in animal studies. Thus, ECoG potentially contains rich information for extracting BCI control signals. Several studies have shown that human subjects can achieve effective control of cursor movement within a short period of time with ECoG ( Fig. 3 ).
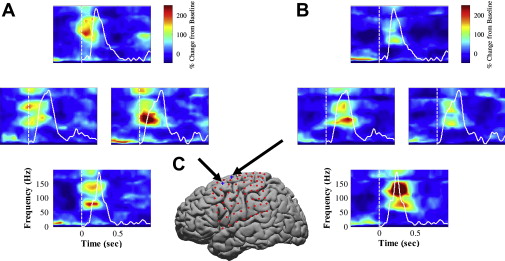
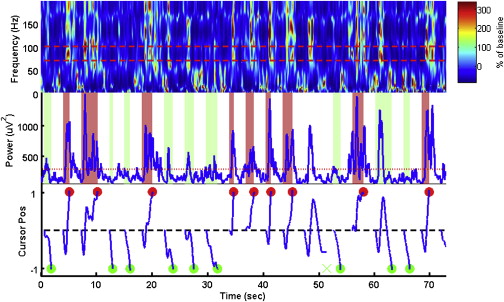
Implantation of these standard subdural ECoG grids typically requires a craniotomy that exposes a large portion of cortical surface. For BCI applications, such an invasive craniotomy is impractical and can be avoided with a new technique called micro-ECoG. A micro-ECoG electrode grid can be described as a miniature version of the regular ECoG grids with smaller electrodes and closer spacing between electrodes. Micro-ECoG grids can record neural activity from a localized cortical volume with high spatial and temporal resolution, which makes it possible to extract even richer and more specific information from micro-ECoG signals for controlling BCI devices. Furthermore, a micro-ECoG grid can easily be implanted through a small burr hole in a minimally invasive surgery, and it can be placed on top of the dura mater. By leaving the dura intact, risks of various complications, such as infection, can be significantly reduced. Previously, the Cleveland Clinic Foundation group reported no serious complications from epilepsy monitoring using epidural electrodes, and there was zero instance of purulent wound infection from 500 epidural electrodes inserted. Hence, micro-ECoG recording may provide high-quality neural recording with low clinical risks, making it a desirable platform for developing clinical BCI devices.
With approval from the Institutional Review Board at the University of Pittsburgh, the authors recently conducted a micro-ECoG study in a human subject undergoing subdural ECoG recording for the purpose of epilepsy monitoring. A micro-ECoG grid with 14 recording electrodes was implanted over the motor cortical area, and the high-frequency band of neural signals recorded from this micro-ECoG grid showed significant modulation by hand movement, including individual finger movement and grasping/pinching movement of the hand. Modulation of micro-ECoG signals by hand movement offers a neural substrate that is critical to the success of a BCI system intended to restore volitional control of finger movement. During the experiment, the subject achieved real-time control of one-dimensional cursor movement within 30 minutes. Offline analysis showed that hand posture (open vs closed) can be predicted accurately using micro-ECoG signals and that individual finger movement can be decoded with an accuracy of 73% (chance level 20%).
The capability to decode hand movement from micro-ECoG recording has great clinical significance. Loss of hand function leads to difficulties in simple daily tasks, such as grooming, feeding, and dressing, and it significantly affects quality of life. In addition to high-level SCI patients, many individuals with hand function impairments caused by other neurologic disorders, such as stroke, may benefit from this technology. A large number of stroke patients have upper limb paresis on admission to hospital (60%–70%) and 55% to 75% of stroke survivors have limited upper-extremity function. Our study suggests that it is possible to develop a minimally invasive implantable BCI device based on micro-ECoG recording to provide control signals for prosthetic hands or functional electrical stimulators to reanimate paralyzed hands.
For all the BCI systems mentioned above, regardless of the neural signal (single-unit activity, EEG, ECoG, and so forth), when a subject operates a BCI system, neuroplasticity plays a critical role. Through closed-loop training with real-time feedback of cursor or robotic arm movement, BCI systems induce neuroplasticity that greatly facilitates BCI control. Meanwhile, neuroplasticity induced by BCI not only benefits BCI operation itself, but may also potentially facilitate functional recovery. In the next 2 sections, the goal of using BCI devices changes from simply operating the device to serving as a tool for stroke and SCI rehabilitation.
BCI as a Rehabilitation Tool
In addition to controlling assistive devices that can replace or augment impaired functions, BCI technology can also facilitate the restoration of function through neuroplasticity. Traditional therapies for restoring motor function focus on forced use of the impaired limb. Most notably, constraint-induced movement therapy and electromyogram (EMG)-based biofeedback have proven to be fairly successful strategies for improving muscle strength and performance of activities of daily living. However, the success of these therapies depends on the patient having a moderate degree of residual function to start with. In the case of SCI or severe hemiplegia, alternative therapies for restoration of function need to be explored. In particular, this article focuses on research that seeks to engage neuroplasticity using BCI as a neurofeedback tool to elicit changes along the corticospinal pathway.
Neurofeedback is a technique in which an individual learns to voluntarily modulate, or change, his or her brain activity. Current clinical applications of neurofeedback include the treatment of epilepsy, anxiety, and, more recently, attention deficit hyperactivity disorder (ADHD). Users are provided with real-time feedback of some feature of their neural activity, often the amplitude of oscillatory signals originating within the cortex. Based on this feedback, the individual learns to control the neural signal of interest. Voluntary modulation of sensorimotor rhythm amplitude is often learned through neurofeedback to control a BCI device. It is well established that neuroplasticity can be induced, and that control of voluntary modulation can be improved, with BCI training. In addition to improving brain control of external devices, such as computer cursors and robotic arms, neuroplasticity induced by BCI training may also directly facilitate motor function recovery. Many previous studies have shown that functional recovery is often associated with cortical activity returning to a state close to that of an unimpaired individual. BCI training through neurofeedback can directly influence cortical activity and potentially restore a normal cortical activation pattern, which could have a positive effect on downstream neuromuscular systems.
A recent study investigated the feasibility of restoring movement using BCI training in chronic stroke patients. In that study, subjects learned to modulate their brain activity to control an orthosis that opens and closes the hand. Eight participants with chronic hand weakness secondary to stroke underwent training focused on modulating sensorimotor rhythm amplitude as measured by MEG. MEG sensors over the sensorimotor region that were activated during imagery of movement of the paralyzed hand were selected as control channels for the MEG-based BCI. Motor imagery and observation, for example, of an individual performing the intended task, represent powerful inductors of cortical plasticity. Sensorimotor rhythm amplitude controlled the vertical position of a computer cursor and the subjects tried to hit a top or bottom target. In addition, the same neural signals were coupled to control of the hand orthosis. The orthosis guided the hand into an open or closed position depending on the sensorimotor rhythm amplitude relative to a predetermined threshold. After 3 to 8 weeks of training, these 8 patients were able to achieve an average success rate of 72% for the 2-target task. Although this success rate may seem modest, it should be noted that most of these patients were able to achieve control with MEG sensors above the sensorimotor areas ipsilateral to their subcortical stroke lesions. No significant changes in gross hand motor function were measured for any of the patients. Hand function improvement was not expected in those patients, as they started the study with a 0/5 score on the Medical Research Council scale with completely paralyzed hands. To further improve the efficacy of this neurofeedback paradigm, particularly in patients who retain some level of hand motor function, BCI can be combined with functional neuromuscular stimulation to generate brain-controlled muscle contraction. The famous Hebb rule for neuroplasticity is often summarized as “the neurons that fire together, wire together”. Temporally coupled motor cortex activation, muscle contraction, and somatosensory feedback may promote neuroplasticity for motor functional recovery based on similar principles.
A Comprehensive Rehabilitation Approach Combining Action Observation, Motor Imagery, and BCI Neurofeedback
The authors believe that the feasibility and effectiveness of a BCI-based neurofeedback paradigm can be further enhanced by combining BCI-based neurofeedback with 2 additional training paradigms: action observation and motor imagery. Action observation training is based on the concept of a human mirror neuron system (MNS). Neurons in the human MNS fire when individuals act and when they observe the same action performed by another person. These neurons “mirror” the behavior, as if the observers are themselves acting. The human MNS plays a critical role for motor learning, action imitation, action understanding, speech, and social interaction. In addition to the classic human MNS, including the inferior parietal lobule, ventral premotor cortex, and the caudal part of the inferior frontal gyrus, multiple human and animal studies have also shown that the motor cortex demonstrates congruent activities during action observation and action execution. Just like action observation, motor imagery, or the imagined movement of a body part, can also activate multiple sensory and motor cortical areas. Motor imagery is believed to be a covert stage of action execution that draws on cortical areas that are typically involved in motor planning and execution, such as the supplemental motor area, premotor cortex, and the primary motor cortex (M1).
Action observation and motor imagery can elicit a certain degree of motor cortical activity in the absence of overt movement. They offer clinicians opportunities to directly activate cortical areas that cannot be activated otherwise, such as cortical areas representing a paralyzed limb. They may be able to strengthen neural pathways that remain intact, and also facilitate activation of motor cortical areas. Multiple studies have demonstrated that action observation and motor imagery can elicit sensorimotor cortical activity and, at least temporarily improve motor functions of paralyzed limbs after stroke and SCI. As discussed in the last section, a BCI-based neurofeedback training paradigm has the potential to promote neuroplasticity and functional recovery. A comprehensive rehabilitation approach that merges BCI neurofeedback, action observation, and motor imagery will bring even greater benefits to patients than using any of those paradigms alone. First, action observation and motor imagery will facilitate BCI training. They allow a BCI system to optimize its decoding algorithm to extract motor-related information from cortical activity without overt movement from patients. They also offer an intuitive way for patients to learn to voluntarily modulate their cortical activity to operate a BCI system. Enhanced performance in BCI operation will make the neurofeedback paradigm more effective, potentially improving motor function recovery. Second, BCI systems can at least partially provide real-time feedback of actions that a patient is imagining, making motor imagery training more engaging and effective than simple motor imagery without any feedback.
The authors are currently developing such a rehabilitation paradigm to enhance motor cortical modulation in individuals with tetraplegia. In the case of incomplete SCI, it is our goal to maximize the amount of information transferred through remaining corticospinal pathways to enhance residual function. For others, maximizing voluntary control of cortical modulation may increase the effectiveness of BCI technology and future spinal cord regeneration therapies. Preliminary studies investigated motor cortical activity in able-bodied volunteers and individuals with tetraplegia during action observation and action execution, with a primary focus on hand motor function. The study was approved by the Institutional Review Board at the University of Pittsburgh. Results from 2 participants are reported here. One participant was a 29-year-old man with no history of neuromuscular disease, and the other was a 34-year-old man with a C7-level complete SCI that occurred 15 years before his participation in this study. A whole-head 306-channel MEG system (Elekta Neuromag) was used to record cortical activity noninvasively while subjects performed simple hand movements under 4 different conditions:
- 1)
Observed: participants watched a video of the movements while they remained resting.
- 2)
Imitated: participants performed the movements along with the video presentation.
- 3)
Imagined: participants imagined performing the movements in response to a visual cue.
- 4)
Overt: participants overtly performed the specified movement in response to a visual cue.
The time sequence and visual feedback provided during the experimental paradigm are shown in Fig. 4 . Two different hand movements were tested: (1) grasping an object; (2) tapping an object with the finger tips using wrist flexion and extension. A total of 40 repetitions were collected using a randomized block design for each “condition × movement” combination.
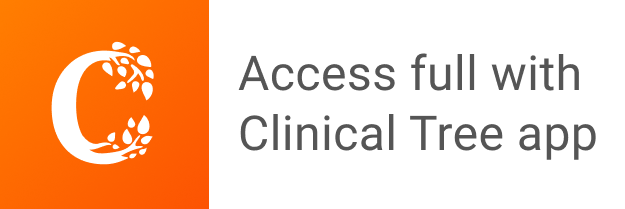