Evaluating Trauma Center Performance
Turner Osler
Laurent G. Glance
The mission of trauma centers is to prevent death and optimize the recovery of injured patients. The performance of trauma centers is therefore of critical interest to patients and their families, and increasingly to those who pay for trauma care as well: insurers and the government.1 But the evaluation of trauma center performance requires some measure or measures of performance, and such metrics have proved elusive. In part, this is because there are so many possible (and possibly conflicting) outcomes upon which a trauma center might be evaluated. The underlying heterogeneity of trauma patients further compounds the problem because we must always adjust our expectations for an individual patient predicated on his potential for a good outcome. Importantly, the required adjustment will depend on the outcome under consideration.
Perhaps because of these underlying difficulties the measurement of trauma center performance is still in its infancy. Tellingly, although the mostbasic and unequivocal outcome measure, survival, has been extensively examined over the last 25 years there is not yet agreement on the best model for this outcome: Injury Severity Score (ISS), International Classification of Disease Injury Severity Score (ICISS), Trauma and Injury Severity Score (TRISS), and A Severity Characterization Of Trauma (ASCOT) all have their advocates and still other survival models are likely to emerge. (See appendix for a brief discussion of these measures.) Early work suggests that the choice of outcome prediction model affects conclusions about trauma center performance,2 and therefore the choice of scoring system is likely to be of considerable interest to all concerned, particularly if trauma center certification or reimbursement becomes contingent upon such measures.
If we consider outcomes for individual patients, we might think of survival as of primary interest, but the degree of residual disability that patients experience may be of even greater importance in the case of injuries that are rarely fatal. Many other outcomes are also of interest: the efficiency with which care is rendered (in terms of length of stay or cost), the effectiveness of care (complication rates, readmission rates, accuracy of medical decision making), satisfaction with care (patients, families, referring physicians), all are important. Developing risk-adjusted measures for any of these outcomes is likely to prove at least as challenging as finding a single risk-adjusted measure for survival to discharge has. Moreover, because the risk factors for different outcomes are likely to be different, every outcome of interest will require its own risk adjustment model. Some thoughtful authors have gone so far as to conclude that “outcome is neither a sensitive nor a specific marker for quality of care” and should be used only “…to help organizations detect trends and spot outliers…”.3 It is sobering to observe that to date we do not have a single risk-adjusted trauma outcome measure that is universally accepted.
Even if performance measures could be defined and measured, methodological difficulties remain. For these measures to be useful metrics of performance, they must be used to make comparisons, either within a single institution over time or between institutions. Deciding if two institutions with different patient mixes differ significantly on a given outcome measure is not trivial, however, as is evidenced by the number of statistical approaches that have been advocated. Although the simple “Z, W, and M statistics”4,5 approach to comparing institutional mortality rates of 20 years ago
never achieved mathematical respectability, subsequently many other methods have been explored: cumulative sum charts,6 hierarchal models,7 Bayesian methods,8 propensity scoring,9 and matching algorithms10 have all been proposed, and other methods are likely to emerge.
never achieved mathematical respectability, subsequently many other methods have been explored: cumulative sum charts,6 hierarchal models,7 Bayesian methods,8 propensity scoring,9 and matching algorithms10 have all been proposed, and other methods are likely to emerge.
Faced with the enormity of the task of “measuring performance” in terms of numbers of possible outcomes to be measured, the effort required to develop even a single such measure, and the statistical complexities of comparing institutions based on such measures, organizations such as the American College of Surgeons (ACS)11 have wisely eschewed performance measures in favor of measures of structure and process. This approach, first outlined by Donabedian 25 years ago,12 advocates the evaluation of structures that are believed necessary for excellent care (physical facilities, qualified practitioners, training programs, etc.) and processes that are believed conducive to excellent care (prompt availability of practitioners, expeditious operating room access, patients who underwent postsplenectomy receiving overwhelming postsplenectomy infection [OPSI] vaccines, etc.) Although outcome measures were also included in Donabedian’s schema, he recognized that these would be the most difficult to develop and employ.
Although it might seem that measures of structure and process would be straightforward to posit and use, in practice difficulties immediately arise. The selection of structural characteristics requires that we know which aspects of a trauma center’s design affect performance, and here intuition may mislead us. An instructive example of this was the suggestion of “case volume” as structural criteria for trauma centers. Although case volume has been shown to be associated with outcomes for some high-risk surgical procedures, when this criteria was examined for trauma centers it was unclear how “case volume” should be defined (all trauma admissions?, all “major” trauma cases?, “major” trauma cases per trauma surgeon?, etc.) or what actual volume of cases was adequate. Indeed, it is not even clear that there is an association between volume and outcome in trauma care.13 Process measures are subject to these same difficulties with definition and implementation.
An entirely separate question is: “If accurate measures of trauma center performance were developed, how might such measures be employed?” One possible use would be to carefully examine centers with the best performance to determine which characteristics and practices lead to superior outcomes. This approach has been fruitfully employed by the Northern New England (NNE) Cardiovascular Disease Study, where a 24% reduction in hospital mortality rates was observed,14 and by the National Surgical Quality Improvement Program15 where a similar decrease in mortality in Veterans Administration Hospitals was described. Other approaches are possible, however. Insurers might simply require trauma centers to attain some benchmark before agreeing to pay for services. Government regulators might follow the “No Child Left Behind” model, and require that all trauma centers meet defined standards or face funding sanctions or perhaps state control. Although the experimental rigor of the NNE approach has been questioned and the unintended consequences of the latter two approaches are difficult to envision, it seems certain that, if predicated on inaccurate or unfair outcome measures, the result of performance evaluation is likely to be worse, not better, care of the injured. It is therefore imperative that any outcome measures be reliable before they are employed.
In this essay we will expand briefly on the philosophic and technical difficulties involved in measuring performance. We will find that measures of structure and process are much better developed at this time, but are not without serious problems in their application. We will then briefly describe how trauma centers are currently evaluated by the American College of Surgeons Trauma Center Verification process. In the end, we will conclude that the perceived need for measures of performance currently outstrips our ability to provide them. Indeed, it is uncertain if such measures can ever be developed.
PHILOSOPHIC CONSIDERATIONS
Any summary measure loses some of the information present in the data during the process of summarization, and this is true for measures of quality. Therefore, we must recognize that any single measure of quality is likely to hide as much as it reveals. For example, a hypothetical trauma center might be judged to have “satisfactory survival results,” but the actual data may consist of poor results for patients with closed head injuries and excellent results for patients with penetrating trauma. To simply accept the judgment of “satisfactory” would be to miss an important opportunity to improve the care of patients with head injuries at that trauma center. Because care can vary over so many types of injuries, we are likely to need many measurements to gain a clear picture of quality of care. The problem is compounded because individual patients may have several types of injuries. Additionally, quality can vary in many dimensions. While it may be relatively easy to discern a difference in outcome between patients with blunt and penetrating trauma, care that varies from day to day, or care that is worse on weekends may be much more difficult to detect and will likely escape notice unless it is specifically sought.
Many different outcomes may be of interest, and some of these will inevitably be in conflict. Therefore, short length of stay may be regarded as a marker for good care, but high readmission rates would likely be regarded as a marker for poor care. It is a matter for considered judgment to decide what the appropriate balance for these two conflicting outcomes might be. Indeed different arbitrators (a patient’s insurance company vs. the same patient’s lawyer, for example) might have different perspectives of where the balance ought to be. As another example, although it
is paramount for physicians to respect patients’ wishes concerning medical treatment, such deference may lead to “unnecessary” deaths which might be held on superficial analysis to reflect “bad” care. It may be difficult to ferret out all such confounding circumstances.
is paramount for physicians to respect patients’ wishes concerning medical treatment, such deference may lead to “unnecessary” deaths which might be held on superficial analysis to reflect “bad” care. It may be difficult to ferret out all such confounding circumstances.
Even after we decide on a dimension of care that we wish to evaluate, many different competing measures may be available. Problematically, different risk adjustment models may lead to different conclusions about the overall success of care,16,17 and it is usually unclear which measure is the most valid. In the absence of a gold standard, it is likely that disagreements over the most appropriate measure will arise.
STATISTICAL CONSIDERATIONS
At its heart, statistical analysis is simply the mathematical procedure used to distinguish real differences from random variations. Although statisticians have developed powerful techniques to attack this fundamental problem over the last century, in practice comparisons between different trauma centers’ performances present several challenges.
Because of the low incidence and binary nature of many measures that might be used in performance assessment, very little information is available per measurement. Therefore, large numbers of patients are usually required to reliably detect real differences between trauma centers. Consider the simple example of mortality rates. Suppose two trauma centers had identical patient populations and further, that trauma center A had a mortality rate of 3% whereas trauma center B had a mortality rate twice as great (6%). A simple calculation reveals that 1,000 patients from each trauma center would be required to have a 90% chance of declaring this difference significant. More subtle differences, say between mortality rates of 5% and 6%, would require more than 10,000 patients per trauma center, a number unlikely to be available in any reasonable time frame. Fortunately, binary outcomes with higher incidences (say complications) require fewer observations to establish significant differences, but to establish a difference between a 50% rate and a 60% rate still requires 500 patients per trauma center.
In reality it would be rare for two trauma centers to actually have identical patient populations, and therefore risk adjustment models are required. The science of risk adjustment is an evolving field, however, and as alluded to in the preceding text, many different statistical techniques for risk adjustment have been suggested.18 It is still unsettled which approach is most reliable, but certain principles are clear. For example, risk adjustment models can be successful only if the patient populations of the centers being compared overlap significantly on predictors that affect the outcome of interest. Therefore, it would not be sensible to compare pediatric trauma centers to adult trauma centers unless the adult trauma center happened to also care for children, and further that such an analysis was limited only to outcomes for children. Although this example seems trivial, the same problem would complicate the comparison of a trauma center caring largely for patients with penetrating trauma to a center caring for patients with primarily blunt trauma.
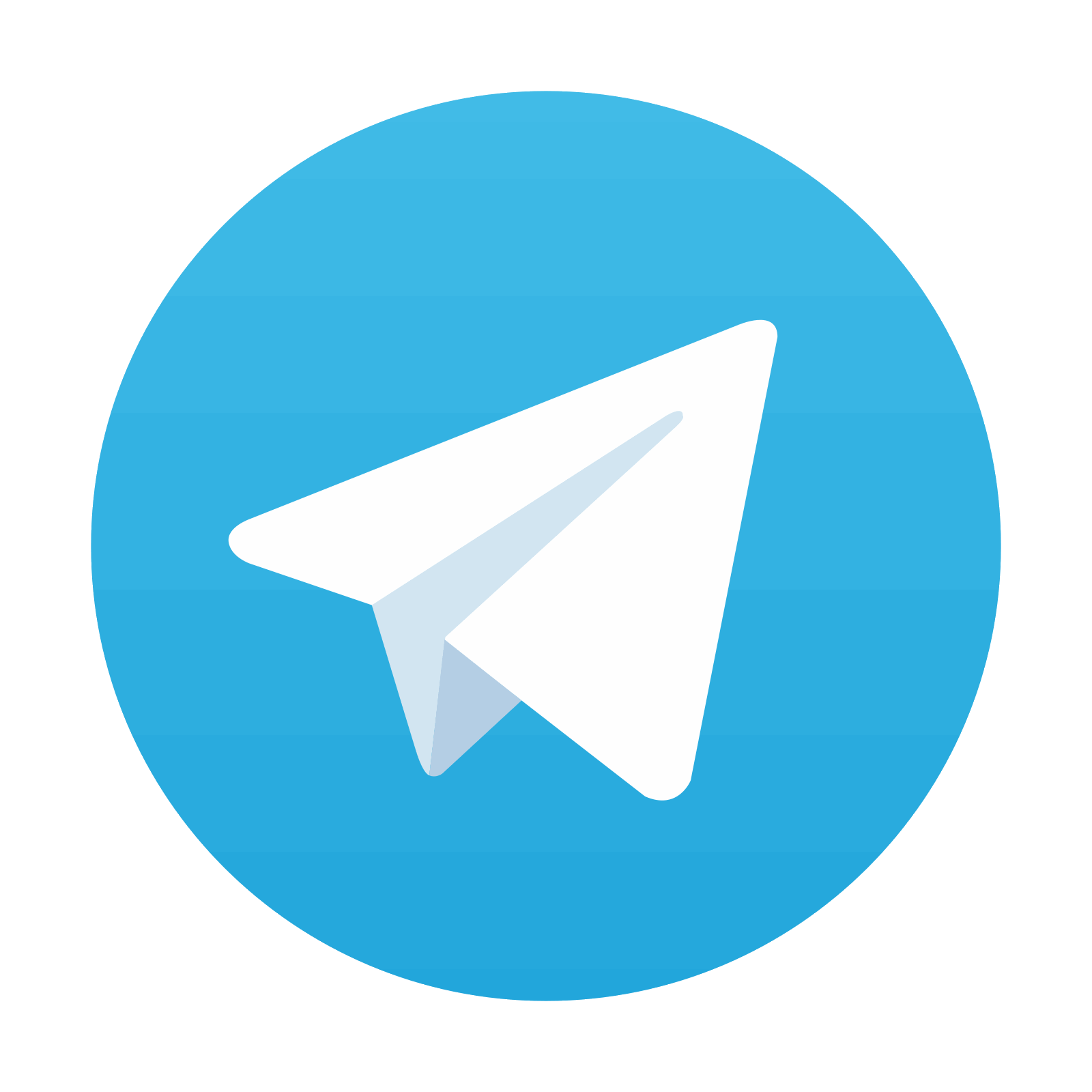
Stay updated, free articles. Join our Telegram channel

Full access? Get Clinical Tree
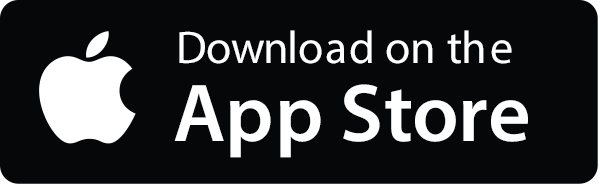
