© Springer International Publishing Switzerland 2014
Hasan Yazici, Yusuf Yazici and Emmanuel Lesaffre (eds.)Understanding Evidence-Based Rheumatology10.1007/978-3-319-08374-2_3Disease Classification/Diagnosis Criteria
(1)
Division of Rheumatology, Department of Medicine, Cerrahpasa Medical Faculty, Cerrahpasa Hospital, University of Istanbul, Cerrah PaÅŸa Mh No 53, Istanbul, 34098, Turkey
(2)
Division of Rheumatology, Department of Medicine, Seligman Center for Advanced, Therapeutics and Behcet’s Syndrome Center, NYU Hospital for Joint Diseases, New York University School of Medicine, New York University, 333 East 38th Street, New York, NY 10016, USA
The science and practice of rheumatology rely heavy on criteria. This is true for both clinical practice and research. This chapter will focus on disease classification and diagnostic criteria only. Outcome and remission criteria are handled in Chapter “Outcome measures in rheumatoid arthritis”.
The prevailing view is that we should have separate criteria sets for research and diagnosis, the former requiring classification and the latter diagnostic criteria. We propose this is not only unnecessary but unfounded. The main aim of this chapter is to discuss why we indeed have to put heavy emphasis on disease criteria in rheumatology and how we should we go about it and why it is wrong to have separate classification and diagnostic criteria for any one disease. In doing this we will resort to specific examples from the recent attempts in criteria making for rheumatoid arthritis (RA) and vasculitides, especially Behçet’s syndrome (BS).
A Brief History of Disease Criteria in Rheumatology
Generations of physicians around the globe have been and still are taught the Jones criteria to diagnose rheumatic fever [1]. These criteria were developed by Dr. Jones in 1944 by a strictly ad hoc and eminence based approach and we are afraid this legacy continued in several later updates. The last attempt to better these criteria was a consensus conference in 1992 [2]. In this last conference the issue of potential geographical differences in the utility of these criteria were brought up, as if this was something unique to rheumatic fever. As we will bring up once more below, the utility of any diagnostic criteria is strictly dependent in the setting in which the criteria are applied. It is no surprise then that more recent formal surveys keep on showing that the sensitivity of the Jones criteria for diagnosing rheumatic fever is only around 30 % in endemic areas like India [3].
In 1974 Dr. Desmond O’Duffy proposed his Behcet’s Disease criteria [4]. The more senior author of this chapter (HY) was in the audience as a young fellow when this set of criteria was presented in a rheumatology meeting. At the end of the presentation he got up and had the courage to ask the presenter “ How successfully do your criteria tell Behcet’s from ingrown toe nails, particularly since I saw no attempts to prospectively test these criteria in a real setting nor a control group in your exercise?” There was little discussion and few heated exchanges, but this outburst was probably the initial stimulus for the latter work related to the formulation of the International Study Group Criteria for Behcet’s Disease (ISGC) [5] currently in use.
Perhaps a new era began in criteria making when American College of Rheumatology (ACR) began publishing criteria for many of the vasculitides [6]. These criteria were no longer ad hoc. A survey was conducted seeking formal sensitivity and specificity for many of the common primary vasculitides. Granted they were based on retrospective analyses and lacked prospective testing, they were an important step away from sheer eminence.
Then came the realization that these ACR vasculitis criteria were not useful for diagnostic purposes [7]. In a formal sensitivity and specificity study it was shown that these criteria had limited (17 % to 29 %) positive predictive values when applied to 198 patients with various vasculitides and connective tissue diseases. Two years later, a further study showed that Chapel Hill Consensus Conference (CHC) criteria, another widely recognized vasculitis criteria set mainly based on the size of the vessel involved, correctly identified only 8 of 27 patients with Wegener’s granulomatosis and 4 of 12 patients with microscopic polyangiitis [8]. The response to this issue was that these two sets of criteria were not intended for diagnostic use but were strictly classification criteria for research and educational purposes [9]. This contention sounded very reasonable when first heard and over the years it became the standard to call all disease criteria classification criteria. This was followed by a new desire and the promise to prepare diagnostic criteria in addition to classification criteria for our diseases, an exercise, which we are afraid, might be likened to constructing a perpetual motion machine.
Why do we Need Disease Criteria?
As with other major and hotly debated issues the “why” of an exercise is often a neglected caveat. Apart from preparing for boards and other such evils there are some very good reasons for having disease criteria. These include:
1.
To diagnose diseases to help our patients. This encompasses both managing and explaining to the patient the nature of his/her illness.
2.
To conduct valid clinical or basic research about these conditions.
3.
To explain to the public, health authorities, third party payers, research supporters and financial source allocators the nature of our patients’ illnesses.
The presence of separate reasons, at first sight, might be taken to indicate that we might actually need separate classification criteria for research and diagnostic criteria for our patients but perhaps other sets of criteria for still other purposes and even perhaps one for Brussels and another for Washington, as well. We, however, propose that one set of criteria should be good enough for diagnosis, research and public awareness as long we explain, first to ourselves, then to our patients and rest of the public what we intend to with these criteria openly, frankly admitting we cannot diagnose every ill we see. This explanation should obviously continue with the explanation that we can manage some of these ills rather effectively even when we do not know what the exact diagnosis is.
Rheumatologic Diseases as Constructs
As we emphasized in the previous chapter, many rheumatologic diseases do not have specific clinical, histologic, laboratory or radiologic features. Hence we have to come up with constructs to specify what we mean by a “disease”. For example if we have a shoulder which is swollen in the shape of a shoulder pad and when we biopsy it we find amyloidosis, we do not have to come up with a construct to tell us and the patient that he/she has amyloidosis. The same is true for a painful, hot and swollen knee from which you isolate staphylococci. On the other hand, in a patient with chronic mouth ulcers, attacks of diarrhea and episodes of uveitis you have to build up a construct to identify Behçet’s syndrome and another to identify Crohn’s. Still yet, you have to build up a construct to tell one from the other. Why do you have to resort to constructs? Simply because neither Behçet’s nor Crohn’s can be identified by a specific appearance, histology or a laboratory finding. So you need to build up a concept composed of specific features, in other words a construct. Surely the need for such constructs in rheumatology is not as extensive as in psychiatry with their voluminous standard reference manual, DSM (Diagnostic and Statistical Manual of Mental Disorders) of the American Psychiatric Association which includes definitions of over 400 different mental disorders [10] but we still need them. A set of criteria in turn is nothing more or less than the declaration of the components of a construct with some hierarchy (more commonly called weighing) of these components. It is to be underlined that elements which we decide to exclude from this construct make up the exclusions of our criteria. We propose that the first step in understanding disease criteria is to realize that they are constructs put together for specific purposes to explain and convey departures from the normal. In addition such constructs are needed not only for diseases of unknown origin. Sometimes we resort to constructs in handling diseases we know in depth the etiology and/or the pathogenesis of. For example in a tuberculosis endemic area we can justifiably begin treating a patient with a cough for so many weeks and a chest radiograph according to a well built up construct for diagnosis of tuberculosis or admit a patient with a chest pain for a suspected myocardial infarction if he/she fulfills the Cook County criteria for chest pain [11]. The main message then is that in the science and practice of medicine a diagnosis is needed mainly after we consider what we do with it.
The Basic Elements of Criteria Making
We have emphasized that we need disease criteria especially when our disease is a construct. The 3 basic elements of criteria making all have to do with concepts in probability. They are sensitivity, specificity and the pretest probability.
Sensitivity:
Sensitivity is an easy concept. It is simply the percentage of true positives. If 95 % of patients with systemic lupus erythematosus (SLE) are positive for antinuclear antibodies (ANA) then the sensitivity of ANA for SLE is 95 %. Alternatively if 85 % percent of patients fulfilling a particular set of disease criteria for SLE then it is said that this set of criteria is 85 % sensitive in detecting SLE.
Specificity:
Specificity is a more difficult concept. It is the percentage of true negatives. Following the example we gave in defining sensitivity, if 80 % of all people not having SLE are not positive for ANA, then the specificity of ANA for SLE is 80 %. Similarly if a particular set of criteria is negative in 85 % among a group of individuals without SLE then we say that this set of criteria is 85 % specific in detecting SLE. Why is specificity more difficult [12]? We propose two reasons. First, before defining either the sensitivity or the specificity of any finding or a set of criteria for any disease, we have to first define what we mean by individuals with and without the disease. This is intuitively easier in sensitivity where our job is to only define what we mean by the disease we are interested in. If we are trying to assess the sensitivity of laboratory finding we are only concerned with one disease, SLE. We can surely also specifically want to assess the sensitivity among a subset of SLE patients like early, mild or severe disease. Whichever is the case, when at the end we say that “The sensitivity of the test A is 75 % in SLE we say practically all that needs to be said. With specificity, however the situation is more involved. When we declare that “The test A is 70 % specific for SLE.” the information we convey is incomplete. What we need to define here is not SLE but what is not SLE. On the one hand, we can make our test very specific if we test it among healthy people only or we can make it noticeably less specific for SLE if we test it among patients with a particular disease with a known propensity for having a positive test A. In brief, the definition of specificity of any finding for any disease is incomplete unless we also clearly define the population without having the disease of our concern. What needs to be said is “The test A is 70 % specific for SLE when tested among x number of healthy individuals, y number of patients with disease B and z number of patients with disease C”.
The second reason we propose for what makes specificity more difficult to grasp than sensitivity is the way we verbalize either concept. When we say “Among 100 patients with SLE 95 patients were ANA positive. Therefore the sensitivity of having a positive ANA test is 95 % sensitive for SLE.”, three positive bits of information follow each other. On the other hand, when we declare “Among 100 patients without SLE, 70 were negative for ANA. Therefore the specificity of having a positive ANA is 70 % specific for SLE.” we again verbalize three consecutive bits of fact however, now, the first two of these are negative while the 3rd is a positive bit of information. We propose that this mental incongruity is the second reason why specificity is a relatively more difficult concept to remember.
Confidence Intervals Around Sensitivity and Specificity
As we will repeatedly see in this book some of the evidence behind evidence-based medicine is surprisingly new. Recall that when we defined sensitivity above, we only gave a percentage. It does not require a great insight to realize that the quality of information coming from 700/1000 = 70 % and 7/10 = 70 % differ substantially.
It is also sobering to note that confidence intervals are still not popular with criteria makers of our day. On the other hand this should not be surprising in that it was as late as 1995 that the science of medicine was introduced to confidence intervals around sensitivity and specificity [13].
The Inverse Relation Between the Sensitivity and Specificity – The ROC
A further important point to be discussed about sensitivity and specificity is their inverse relationship. The graphic description of this relationship is the so-called ROC (receiver operating characteristics) curve. The term comes from signal detection used by engineers for military purposes during World War II [14]. A graph is constructed by plotting the sensitivity (the so called true-positives) against 1- specificity (the so – called false negatives) for a series of hypothetical criteria to diagnose a disease. The criteria set A with a 90 % sensitivity and 85 % specificity will correctly pick up 90 % of the patients with the disease while it will also falsely designate 15 % of the individuals without the disease as having the disease. On the other hand the criteria set B with 95 % sensitivity but this time with 75 % specificity will identify 95 % of all the patients with the disease, however this time a considerably more portion, 25 %, of the individuals without the disease will be incorrectly labeled.
It can be said that a substantial portion of medical decision making is, or more realistically should be, based on constantly working with mostly conceptual ROCs. For example, when confronted with a patient with chest pain you want have criteria as sensitive as possible to put him in a coronary care unit for observation. You can afford to be not very specific for diagnosing him/her as having a myocardial infarction. A short time later when you are debating whether to put a coronary stent in you have to be more specific with a trade off in sensitivity. The decision for a coronary bypass is again another point on the curve, etc. In brief, the relation between what you want to do and where you are on the ROC is all important and without the appreciation of its importance all exercise related to criteria making is in vain.
Importance of Pretest Probabilities and Likelihood Ratios in Making Criteria
It is intuitive that more frequent a disease is, more likely it will be diagnosed and vice versa. Bayes’ theorem (BT) expresses this numerically. The importance of disease frequency (pretest probability in Bayesian terms) in making a diagnosis is not well appreciated in that the usefulness of any disease criteria ultimately depends on this theorem. BT states that given a set of disease criteria is positive in an individual, the probability of that individual having the sought disease is the product of the positive likelihood ratio (LR+) multiplied by the pretest probability (PrP) of that disease in the setting where the patient is seen [15]. Briefly A (the probability of disease being present if the criteria are positive) = B (the PrP) X C (the LR+ as defined by the disease criteria at hand). The formula is usually given in odds but it works with probabilities as well. Since physicians are more used to probabilities we suggest they use these, remembering that a probability is the likelihood of an event happening against the sum of the probabilities of its happening and not happening and thus always expressed as a fraction of unity. The odds, on the other hand, is the ratio of the number of times an event can happen versus the number of times it cannot happen. For example if an event has a 80 % probability of happening then the odds of that event happening versus not happening would be 4:1.
A different type of LR, LR– also helps us in decision making. While a LR+ is expressed is sensitivity/1-specificity or more simply the ratio of the %’s of true positives to false positives while a LR– is expressed as 1-sensitivity/specificity or more simply the ratio of false negatives to the true negatives.
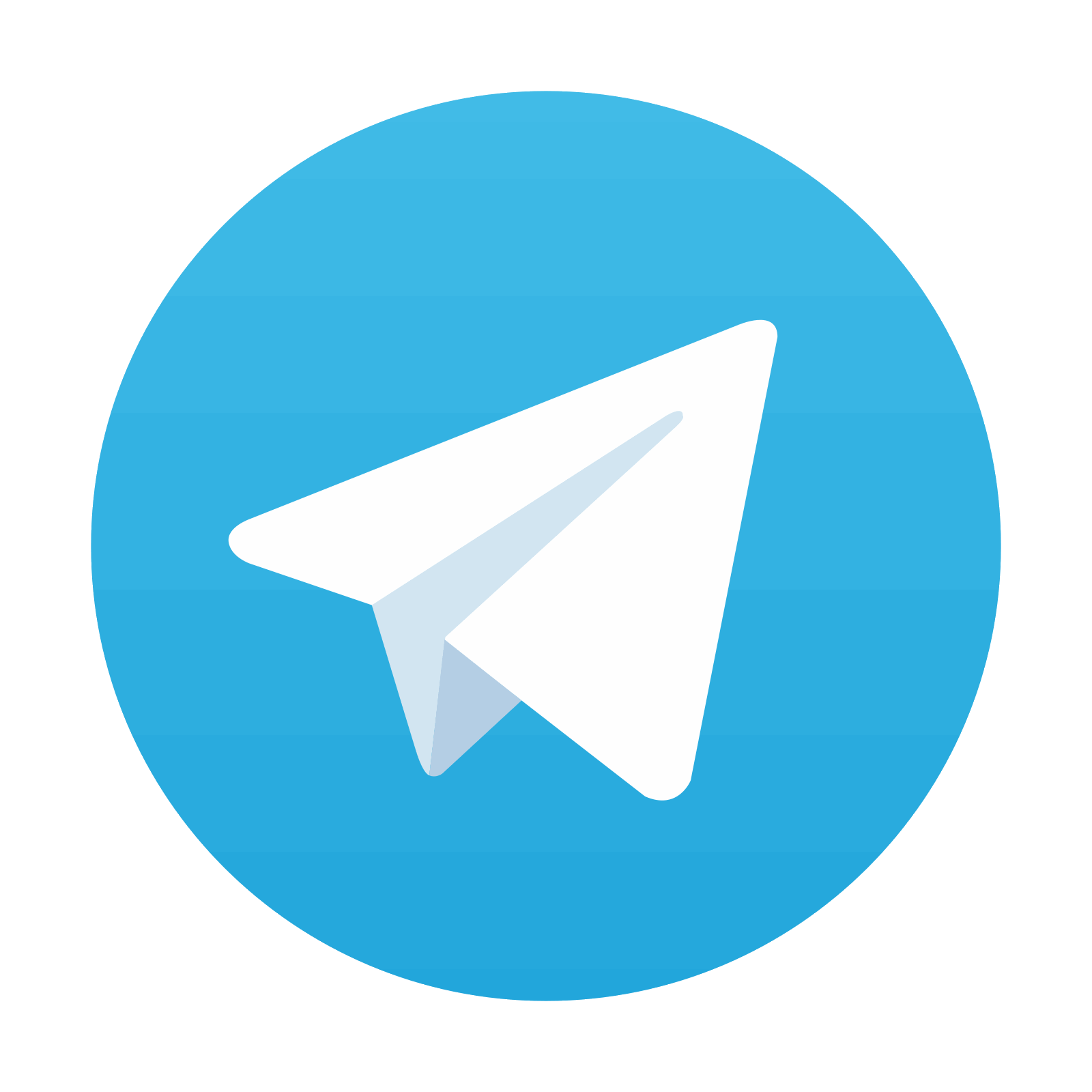
Stay updated, free articles. Join our Telegram channel

Full access? Get Clinical Tree
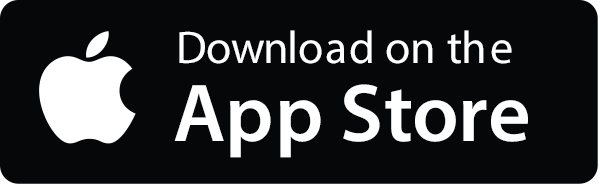
