Orthopaedic research encompasses a wide range of clinical, basic science, and epidemiologic investigation on the embryology, growth and development, injury, and regeneration/repair of the musculoskeletal system. The single clinician-scientist working alone in a laboratory is becoming increasingly rare; most research efforts of recent years are interdisciplinary and take the form of teams of clinicians, biologists, bioengineers, epidemiologists, and biostatisticians.
While the execution of biomedical research requires significant effort and time, considerable effort is typically dedicated to developing the research approach, and importantly, preparing research proposals, through which the investigators request permission or funds to conduct a study, as well as to reporting study results to the general scientific community.
The Research Proposal
Research proposals are submitted to intramural, institutional bodies and/or extramural agencies including (but not limited to) not-for-profit organizations, corporations and government agencies, often in response to a request for applications (RFA) announced by the agency. By far, the largest monetary source of orthopaedic research funding in the United States is the National Institutes of Health (NIH) , an agency of the Department of Health and Human Services consisting of 19 separate institutes (e.g., the National Institute of Arthritis and Musculoskeletal and Skin Diseases [NIAMS] ).
There are many avenues for research proposals based on different stages of one’s career. Grants are available for training and initiation of early-career research proposals from government grants such as NIH and the Department of Defense. Also, foundations such as the Orthopaedic Research and Education Foundation, Musculoskeletal Transplant Foundation, and the Arthritis Foundation offer various sized grants for new and established investigators. Orthopaedic Speciality Societies (e.g., American Association for Hand Surgery, American Orthopaedic Foot and Ankle Society) also commonly offer grants for residents, fellows, and junior physician-scientists.
The objective of the research proposal is to demonstrate to peer reviewers that the proposed studies are feasible, rigorously designed, clinically and scientifically significant, innovative, and impactful. The research proposal format and length may differ among funding mechanisms and agencies, but most follow this general outline:
The investigators list specific aims for the proposed research. Aims are the central questions that the research project will address via the generation of results.
The investigators propose a testable hypothesis or hypotheses associated with the specific aims, which the proposed work will address.
The background and significance of the research question is reviewed; what is known in the field, and what the knowledge gaps of relevance, are established and delineated.
Preliminary data (either supporting the proposed hypotheses, or demonstrating the ability to perform the proposed experiments) and a sample size (i.e., power) analysis are presented.
The methods (approach) detail the experimental design and procedures of the proposed project, as well as the data analysis strategy.
Biographical sketches for the principal (lead) investigator and key members of the scientific team (co-investigators) highlight the training, expertise, and productivity of the personnel who will conduct the research.
Finally, a research budget is proposed, with provisions for both direct costs (those required to perform the study, including supplies, equipment, personnel, time, etc.) and indirect costs (usually a percentage of the direct costs used for institutional overhead [keeping the lights on]).
Review processes differ between applications and institutions, but generally this task is given to a panel of anonymous peer reviewers (scientists working in the same or closely related field). Critiques from the reviewers are often forwarded to the investigators in the interest of improving the proposal for subsequent submissions. Typically, the task of evaluating the quality of a proposal is kept institutionally separate from the actual funding decisions. As a result, at times a research proposal may receive highly favorable feedback but not receive approval for funding.
Provisions for adequate protection and ethical and humane use of subjects must be addressed (in the case of human patients, by the institutional review board [IRB] ; in the case of other animals by the institutional animal care and use committee [IACUC]) . Local, institutional committees (e.g., institutional biosafety committee [IBC] ) are also charged with reviewing the proposed use of radioisotopes, toxic material, or recombinant DNA; the concerns of these bodies must be addressed before the study is allowed to begin.
Reporting the Results
Usually the first step in reporting results to the scientific community takes the form of abstracts (poster presentations or short podium presentations) at regional, national, or international meetings, such as those of the American Academy of Orthopaedic Surgeons (AAOS), the Orthopaedic Research Society (ORS), or the American Society for Bone and Mineral Research (ASBMR). Some of these venues provide anonymous peer reviews but with limited feedback to the investigators in the form of comments (in most cases, the investigators are informed that the submitted abstract is either accepted or rejected for presentation, with no further comment). Published abstracts are increasingly becoming available to those members of the community who do not personally attend the meetings, but these reports tend to be short, preliminary, and terse. The advantage of these venues is the opportunity for feedback from peers at the actual meeting as well as a centralized forum to learn of new research findings that have not yet been published in scientific journals.
Full reports of original research are published in journals (such as Bone, Journal of Biomechanics, Journal of Orthopaedic Research, and Journal of Bone and Mineral Research ). These are submitted to the editors of the journals, who then forward the manuscripts (often with authors and institutions redacted) to anonymous peer reviewers. Detailed critiques are then provided to the investigators regarding major and minor deficiencies of the study as submitted; often, several iterations of reviews are required before a paper is accepted for publication.
Review papers, often invited papers in journals by recognized leaders in a particular field, are designed to summarize the current state of the art and historical perspectives on a topic but generally do not include new data.
General Research Terminology
The following terms are likely to be found in many areas of research. They have been divided into the topic areas of statistics, biomechanics, techniques in measurements, and orthopaedic cell and tissue biology (general, cartilage, and bone).
Statistics and Epidemiology
accuracy: the property of a measurement denoting its proximity to some gold-standard true value. A measurement may be accurate without being precise (e.g., giving a wide range of test results, centered about the true value).
alpha value: the benchmark value that, a priori, is established to determine statistical significance. The alpha value is compared to the p value given by the data. If p is ≤ 0.05 α, then statistical significance has been established. In most cases, α = 0.05.
analysis of variance (ANOVA): a method for evaluating differences in a continuous variable between multiple groups. The ANOVA may be further classified as to the number of grouping types being considered:
one-way ANOVA: considers only one categorical variable, which may itself have many possible values (e.g., gender, ethnicity, disease status, drug dosage). When the number of groups is two (e.g., male/female, young/old, diseased/not diseased), the one-way ANOVA reduces to a t test.
two-way ANOVA: (and more complex versions, involving three, four, or more ways) considers simultaneously the effect of two or more categorical variables (e.g., the effect of gender and ethnicity on patient height would be properly addressed using a two-way ANOVA), along with the interaction between the variables.
Bayesian statistics: (after Rev. Thomas Bayes, 1702–1761, British cleric) a family of statistical techniques in which the interpretation of the results of an experiment includes an evaluation of the results of similar, previous experiments to establish statistical significance. Used in some applications of evidence-based medicine.
categorical variable: a parameter characterized by having a limited number of possible values (e.g., male/female, old/middle-aged/young, right/left) (cf. continuous variable ).
chi-square analysis (χ 2 ): a test for determining whether the proportions of data described by two or more categorical variables is random (e.g., 51% of the people with a particular disease are men. Is the disease gender-related?).
confidence interval (CI): an estimate of the range in which a particular percentage of values (usually 95%) may be found.
continuous variable: a parameter characterized by having an infinite number of possible values (e.g., height, weight, blood pressure, age) (cf. categorical variable ).
correlation: the strength of the relationship between two continuous variables, with no assumption as to which of the variables may be causative (cf. regression analysis ). This analysis yields a p value and an r value. The range of r values is between –1.0 (perfect negative relationship) and 1.0 (perfect positive relationship), with a value of 0.0 denoting no statistical relationship.
Fisher exact test: (after Sir R.A. Fisher, 1890–1962, British statistician and agronomist) a test for determining whether the proportions of data described by two or more categorical variables is random. It is similar in concept to the χ 2 analysis but is more appropriate in cases in which the number of observations is small.
input variable: the parameters that are varied experimentally (cf. output variable); also called predictor variable, independent variable, and the X-axis.
logistic regression: a special form of regression, in which the dependent variable is a categorical (usually binary) parameter. It is often used in outcomes research and epidemiology, in which the output variables are parameters such as diseased/not diseased, healed/not healed, and so forth.
nonparametric test: a distribution-free method (usually rank-ordered) of analyzing data that does not exhibit a normal (Gaussian) distribution (e.g., a Spearman rank-order test in place of a Pearson product-moment correlation; a Wilcoxon signed-rank test in place of a paired t test; a Kruskal-Wallis statistic in place of a one-way ANOVA).
normal distribution: a continuous parameter characterized by a frequency distribution that is Gaussian, that is, bell-shaped, symmetrical, with a maximum number of values at the average, 68% of the values found within one standard deviation of the mean, and 95% of the values found within 1.96 standard deviations of the mean. A normal distribution of the data is a basic assumption of a parametric test; if the data are decidedly nonnormal, then nonparametric tests are advisable.
odds ratio (OR): the effect of a risk factor in increasing or decreasing the probability of an outcome, often determined by a logistic regression. By convention, if OR > 1, the outcome is more probable with addition of the risk factor (e.g., body mass index as a risk factor and osteoarthritis as the outcome); if OR < 1, the outcome is less probable with addition of the risk factor (e.g., regular exercise as the risk factor, and heart disease as the outcome).
output variable: the variables (cf. input variable) that are measured to represent the response to changes in the input variable(s); also called dependent variable and the Y-axis.
p value: the probability that a finding of a difference between groups, or of a correlation between parameters, is due to chance alone.
parametric test: statistical methods, such as linear regressions, ANOVAs, and t tests, which are based on estimates of the mean and variance of a population and that inherently assume a normal distribution of these values ( Table 13-1 ).
TABLE 13-1 Basic Parametric Statistical Techniques
Output variable
Input Variable
Continuous
Categorical
Continuous
Regression, Correlation
ANOVA t test
Categorical
Logistic, Regression
Chi-square, Fisher exact test
ANOVA, Analysis of variance.
post-hoc tests: when an ANOVA provides evidence of a statistically significant effect of a particular parameter with three or more possible values (e.g., ethnicity, old/middle-aged/young), these tests are used in place of multiple t tests to determine specific pairwise differences among groups. For instance, blood pressure may be significantly higher in a group of older patients than in the corresponding group of young patients, but neither may be significantly different from the values found in the middle-aged groups. Several methods are in common use, including the Tukey, Student-Newman-Keuls, Fisher’s least significant difference (LSD), Duncan, and Bonferroni tests when conducting all pair-wise comparisons; Dunnett’s test when comparing test groups with a single control group, or McNemar’s test as a nonparametric, pair-wise test. Also called multiple comparison test.
power: the probability that a finding of no difference between groups, or of no correlation between variables, is in fact true; alternatively, the probability of being able to statistically discern a significant difference between groups, or a correlation between variables, should one exist, given the existing sample size and variance. Generally, the minimum power for a definitive finding of no difference or no correlation is 0.80.
precision: the property of a measurement denoting its repeatability. A measurement may be precise without being accurate (e.g., tightly clustered about a value that is not correct).
pseudoreplication: statistical situation that occurs when more than one measurement is taken from a single individual. Such measurements cannot be considered as independent of one another. Special statistical tests are used in these cases, for example, the paired t test, the repeated-measures ANOVA, and the mixed-model ANOVA.
regression: a method of comparing two or more continuous variables, in which the relationship between the variables (usually in a linear form) is specifically described in terms of one or more independent, or causative, variables and a single dependent, or output, variable. The method gives the geometric equation of the relationship (in the linear form, dependent variable = a constant + [a slope × independent variable]), a p value for both the constant (determining whether the constant is significantly different from zero) and for the slope (determining whether the slope is significantly different from zero), and an r 2 value, which is often interpreted as denoting the percentage of the variability in the data that is explained by the regression equation. The square root of the r 2 value is the r value that would be obtained if a simple correlation between the variables were calculated.
sample size: in general, the sample size (e.g., number of patients or number of animals per experimental group) for a study, is best determined before the study is initiated. Sample size depends on:
- •
the variability of the measurements:
- •
measurement accuracy and precision (which may be estimated by the standard error). The more accurate and precise the measurement, the smaller the sample size required.
- •
variability within the population (which may be estimated by the standard deviation). The smaller the population variability, the smaller the sample size required.
- •
- •
the nature of the question to be addressed:
- •
for comparisons of data between categories (e.g., male vs. female, diseased vs. nondiseased), the difference between means of categories (quantified as the effect size, the difference between means, divided by the pooled standard deviation). The larger the effect size, the smaller the sample size required for a specified power.
- •
for comparisons of continuous variables (e.g., age vs. blood pressure), the slope of the relationship between predictor and outcome variables, in a regression. The further the slope is from 0, the smaller the sample size required.
- •
the chance of being wrong that the investigator is willing to accept (the alpha value, and the power). The higher the chance of being wrong the investigator is willing to accept, the smaller the sample size required.
- •
standard deviation (SD): a measure of the variability of a parameter in a population, equal to the positive square root of the variance. By definition, in a normally distributed population, 95% of the values of the parameter are within 1.96 standard deviations of the mean. Unlike standard error, the standard deviation is relatively insensitive to the sample size. It is always larger than the standard error.
standard error (SE): a measure of the reliability of the mean of a parameter. By definition, if samples are taken from a normally distributed population, 95% of the time, the true mean will fall within 1.96 standard errors of the sample mean. The standard error is quite sensitive to the sample size; as the sample size approaches a census of the target population, the standard error by its very definition approaches zero. The standard error may be estimated as (the standard deviation) / (the square root of the sample size). Also called standard estimate of the mean (SEM).
statistical errors: these are generally divided into two types:
type I error: in which a correlation between continuous variables or a difference between groups is asserted when such correlations or differences do not in fact exist. The probability of making a type I error is α.
type II error: in which a finding of no correlation between continuous variables, or no differences between groups is asserted, when such correlations or differences do in fact exist. The probability of making a type II error is 1-power, or β.
t test : a method for evaluating differences in a continuous variable between two groups. As such, it comprises a special case of the one-way ANOVA. Also called Student’s t test. paired t test: used to determine the difference between two variables for the same subject.
unpaired t test: used to determine the difference between two independent groups such as men and women.
variance (s 2 ): a measure of the spread of the data. The formula for this measure is:
. ,

where s 2 is the sample variance, χ i is the value of one observation, χ is the mean of all observations, and η is the number of observations. Evaluating Clinical Tests
Assuming that there is some readily available gold standard of disease status, clinical tests may be evaluated using the terms found in Table 13-2 .
Test Result | True Disease Status | ||
---|---|---|---|
Positive | Negative | Negative | |
Positive | A | b | a + b |
Negative | C | d | c + d |
Totals | a + c | b + d | a + b + c + d |
Population Indices on the Columns
sensitivity: a/a + c (the part of the first column that is true).
specificity/selectivity: d/b + d (the part of the second column that is true).
Individual Indices on the Rows
positive predictive value: a/a + b (the part of the first row that is true).
negative predictive value: d/c + d (the part of the second row that is true).
Therefore, for example, if {Low Test Result} = Diseased, and {High Test Result} = Not diseased, then
- •
make a test more sensitive (move the cutoff to the right in the previous example; Fig. 13-1 ), and one will correctly classify more of the diseased population (more true positives). One will also falsely classify more of the nondiseased population as diseased (more false positives).
- •
make a test more specific or selective (move the cutoff to the left in the previous example), and one will correctly classify more of the nondiseased population (more true negatives). One will also falsely clear more of the diseased population (more false negatives).
Study Design
Studies may be classified as a series of binary descriptors:
experimental/interventional: the investigator actively does something to change a risk factor (e.g., drug/placebo tests) compared with observational/effectiveness, in which the investigator takes no active role in the status of a risk factor.
prospective: the risk factor may or may not have occurred at the time of the study, but the outcome has not; compare with retrospective, in which both the risk factor and the outcome have occurred at the time of the study.
descriptive: in which the outcomes are qualitatively reported; compare with analytic, in which the outcomes are subjected to quantification and numerical analysis.
Study Groups
control group: group on individuals who do not receive a treatment in a research study.
disease incidence: new cases per population at risk per time. This may be estimated with a cohort study, in which a group of subjects are followed, with multiple observations, over a defined period.
disease prevalence: cases per population at risk at one point in time (generally given as a percentage). This may be estimated with a cross-sectional study, in which all observations are made, once per subject, at a defined time (e.g., next Thursday, or at first presentation).
experimental group: in an experimental study, group that receives treatment. There may be more than one treatment used in the same study.
sample population: the specific part of the target population that the investigator has the ability to measure.
target population: the group to whom the results of the study will be inferred (e.g., all mammals, all people, all young people).
Biomechanics
The field of biomechanics is concerned with the application of physical and engineering principles to issues of biologic interest.
acceleration: change in velocity per unit of time.
angular acceleration (degrees, or radians/time 2 ): time rate of change in rotational velocity.
linear acceleration (distance/time 2 ): time rate of change in velocity associated with movement in a straight line (no rotation).
adaptation: biologically mediated changes in mechanical properties of tissues associated with the mechanical milieu of the tissues.
anisotropy: exhibiting mechanical properties (e.g., strength, stiffness) that are dependent on the anatomic direction of interest (e.g., tensile stiffness of tendon is considerably higher when loaded along, rather than across, its predominant fiber direction).
bending: deformation of a structure in response to a load applied to an unsupported portion of the structure. Commonly, a bending load can be applied perpendicular to the central axis of a structure such that the latter changes shape.
bending moment: the product of force applied and the distance from the point of application to the point of interest; also called torque and commonly measured in newton meters (N-m).
brittle: characterized by a materials’ tolerance of a relatively small deformation (specifically, post-yield deformation) before failure (cf. ductile ).
center of mass: the point about which the mass of the object can be balanced; also called center of gravity.
centroid: the geometric center of a body. In an object of constant thickness, made of a homogeneous material, the centroid and the center of mass are at the same point.
coefficient of friction: ratio of tangential (frictional) force to the interbody compressive (normal or perpendicular) force required to initiate motion between bodies. Ice on steel has a low coefficient of friction, while rubber on pavement has a high coefficient of friction.
compliance: deformation per unit load (inverse of stiffness).
compression: a mode of load application in which an object experiences inwardly directed forces that result in compaction. By convention, compressive forces or motions are reported as negative in magnitude.
couple: a pair of equal and opposite parallel forces acting on a body and separated by some distance.
creep: deformation with time under a constant load (cf. stress relaxation ).
degrees of freedom: the number of independent variables in a coordinate system required to completely define the configuration of an object in space.
displacement: a measurement of change in position in response to force; expressed in units of millimeters, centimeters, etc.
ductile: characterized by a materials’ tolerance of a relatively large deformation (specifically, post-yield deformation) before failure
dynamic load: time-varying (e.g., sinusoidal) load applied to an object (cf. static load ).
elastic: tending to return to original dimensions after the deforming force or deformation has been removed (cf. plastic )
elongation: deformation induced by a tensile load.
energy: a measurement of work added to a system by a force or a deformation; commonly reported in newton meters (1 N-m = 1 joule).
equilibrium: the state of not accelerating, in which the sum of all forces acting on a body is zero.
factor of safety: ratio of structural or material strength (e.g., failure stress) of a body to its load (structural) or stress (material) encountered in normal service (e.g., peak physiological stress).
fatigue: cyclic application of loads at a magnitude below the monotonic failure load. Typically, fatigue loading results in material damage and/or failure.
fatigue limit: magnitude of cyclic load or stress a material or structure can endure indefinitely without failing; also called endurance limit.
finite element analysis (FE, FEA): a quantitative (numerical) method of analysis performed using computer code. Generally, the geometry of the structure of interest is divided into regularly shaped elements, each with multiple nodes. The material of each element is assigned a set of properties (typically, based on experimental data). Material behavior is assumed to follow a prescribed constitutive relationship (e.g., non-linear viscoelastic behavior). Loading conditions (nodes of application and direction of loads) and constraints (limits on node motion) are defined. Changes in node-to-node distance are then calculated; from these, the software calculates local stresses and/or strains and displays results graphically. Also called finite element modeling (FEM).
free-body diagram (FBD): analytical technique in which a body and all the forces acting on it, as well as their orientation and points of application, are delineated. In its simplest form, an assumption is made of no net motion (equilibrium), although dynamic FBDs are also used.
helical axis of motion: a unique axis in space that completely defines a three-dimensional motion of a rigid body (a rotation about, and a translation along, this axis). It is analogous to an instantaneous axis of rotation for planar motion.
hysteresis: The area of a stress-strain curve between the loading and unloading curves ( Fig. 13-2 ). Hysteresis represents energy dissipation due to material viscosity.
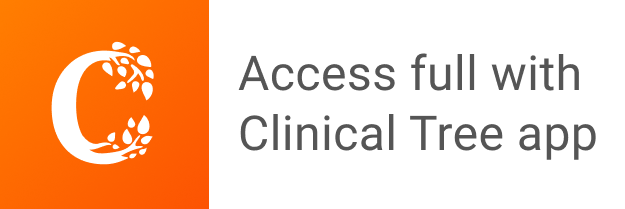