, with is the α/2 quantile of a t-distribution with 2n − 2 degrees of freedom.
These steps illustrate a few important things for computing the sample size:
Clinicians must have a good idea of the effect they aim to show, i.e., what value for ΔS to choose, but they do not need to guess what the true effect might be.
Extra information to perform the computations is usually required. Here, it is the common standard deviation. For the comparison of two proportions, it is the proportion of the control arm.
The computation of the sample size is in general quite technical, varies from test to test, and usually requires a dedicated computer program.
Note that for a non-inferiority test, ΔS needs to be replaced by ΔNI and the statistical test needs to be adapted accordingly. For group sequential designs, dedicated programs have been written not only to compute the sample size but also to compute the intermediate significance levels. For more complicated statistical tests, such as for mixed models (see chapter “A review of statistical approaches for the analysis of data in rheumatology”) and adaptive designs, often only a simulation computer program may throw light on the required study size. A comprehensive, but technical, reference for sample size calculation is given in [21].
Intention-to-Treat Versus Per-Protocol Analysis
The eligibility criteria specify which of the screened patients will be included in the statistical analysis. However, during the conduct of the study, a lot of deviations from the initial plan may take place. For instance, it may happen that due to an administrative error, a patient who should have been randomized to treatment A in fact received treatment B, or that a patient violates the protocol (takes forbidden concomitant medication), or even drops out from the study, etc. What to do with such patients? One approach is to take in the analysis only the “pure patient population,” i.e., only patients who strictly adhere to the instructions. This set of patients is called the per-protocol (PP) set and is preferred by many clinicians because it is believed to express best what the effect of the treatment is on the patients. That is true for the patients still included at the end of the study, but not necessarily for all patients randomized. It is rather the intention-to-treat (ITT) set that is the standard in RCTs. The ITT principle states that all patients who have been randomized in the study should be included in the analysis according to the planned treatment irrespective of what happened during the conduct of the trial. This principle may appear logical at first but may have some unexpected implications. For instance, patients wrongly allocated to B will be analyzed as if they received treatment A; protocol violators are in the ITT analysis set, also patients dropping out the study will be part of the ITT population, etc. FDA and EMEA prefer the ITT analysis in a superiority trial, because it delivers a conservative result in case of the abovementioned problems during the conduct of the study. While the ITT principle is clear, in practice, it may not always be easy to implement and consequently several versions of an ITT analysis exist. For example, it is not immediately clear how to include patients in an ITT analysis with missing values on the primary endpoint. In that case, the ITT analysis cannot include all randomized subjects. But if some values of the primary response are available, then techniques for imputing missing values allow for including such dropouts. Statistical methods that can deal appropriately with missing data are quite important to guarantee the internal validity of the RCT, i.e., that the RCT estimates the true treatment effect in an unbiased manner. An imputation technique that was quite popular for many years but now recognized as problematic is the last-observation-carried-forward (LOCF) approach. This imputation technique imputes the last observed value for the missing primary outcome. For example, suppose the total treatment period is 2 years and every 6 months the primary outcome is measured. Then, when a patient drops out at year 1, the imputed value with the LOCF method for the primary outcome at years 1.5 and 2 is equal to the value observed at year 1. The problem with the LOCF approach is that it imputes an unrealistic value for the outcome (not taking into account the natural pattern of the disease and/or of the curing process) and it underestimates the natural variability of the outcome. In [22], more appropriate imputation techniques are discussed.
In an equivalence or non-inferiority study, the ITT analysis is not the primary analysis anymore since the ITT analysis will bias the results and the conclusions towards the desired hypothesis (equivalence or non-inferiority). Because also the PP analysis does not guarantee to provide an unbiased estimate, regulatory agencies require that an ITT and a PP analysis are performed in an equivalence/non-inferiority RCT and that they show consistent results.
RCT and Some Practical Aspects
The protocol is the reference manual for the RCT containing the background of the intervention, the reason and motivation for conducting the trial, a review of the phase I and phase II results, the justification of the sample size, the eligibility criteria, and the primary and secondary endpoints. In addition, it contains details of the randomization procedure, the informed consent document, the administration of the interventions, etc.
Furthermore, NIH developed a document, called the Manual of Procedures (MOP) (http://www.ninds.nih.gov/research/clinical_research/policies/mop.htm), that transforms a protocol into an operational research project that ensures compliance with federal law and regulations. The MOP typically describes in detail all key ingredients of the conduct of the study, for instance, how data capture will be done, how the patients will be followed up in order to maximize data collection, etc. For example, a list of all eligible patients is never available at the start of an RCT, so the process by which potential trial participants are identified needs to be explicitly stated at the start. In practical terms, this implies that it needs to be specified which countries and centers will be involved in the RCT and what characteristics the involved centers should have.
The protocol also specifies which statistical tests will be chosen for analysis. This can be tricky since many statistical tests depend on distributional assumptions. For instance, the unpaired t-test assumes that there is normality in each of the two treatment arms and that the variances are equal. But, one can only test these assumptions when the results roll in. This rigid requirement does not leave much room for creativity, but is needed to preserve the Type I error rate. As an example, suppose that the protocol dictates to choose the unpaired t-test but that this test does not yield a significantly better result for the experimental arm while a nonparametric Wilcoxon rank-sum test (see chapter “A review of statistical approaches for the analysis of data in rheumatology”) does. Hand switching from one statistical test to another only on the basis of the obtained P-value is an example of a data dredging exercise, which is known to produce many spurious results. In a RCT, all statistical activities should be described in even more detail than discussed in the statistical analysis plan (SAP). The SAP is typically finalized prior to locking the database to avoid speculative choices of statistical procedures.
Trial participants must be fully aware of the risks and benefits of participation and therefore must fill in an informed consent form. This document is also part of the trial protocol.
Finally, each protocol of a RCT needs to be approved by the Medical Ethical Committee of the centers where the study is conducted; they are also called Institutional Review Boards in the United States. In addition, in order to avoid difficulties when applying for registration, protocols are nowadays often discussed with the regulatory bodies to obtain approval (not the drug!) prior to the start of the RCT.
Reporting the Results of a RCT
The statistical analysis plan specifies in detail which statistical tests need to be chosen. No doubt this is accordingly reported in the registration file for the experimental drug, but this is not necessarily the case for the scientific paper written after the study is finalized. Indeed, most referees of medical journals do not check the consistency of the technical report with the submitted paper. Hence, in principle, the reader cannot be sure that the analysis described in the scientific paper is an exact reflection of what has been specified in the protocol. For example, a recently published phase III trial compared pazopanib with sunitinib with respect to progression-free survival in renal-cell carcinoma patients [23]. In that paper, the authors state that “the results of the progression-free survival analysis in the per-protocol population were consistent with the results of the primary analysis” without providing further details. However, from the technical report, one can infer that the predefined margin of non-inferiority (<1.25) was only met for the ITT population and not for the PP population. This is in conflict with the requirement that in both analysis sets, non-inferiority must be claimed (see also [24]).
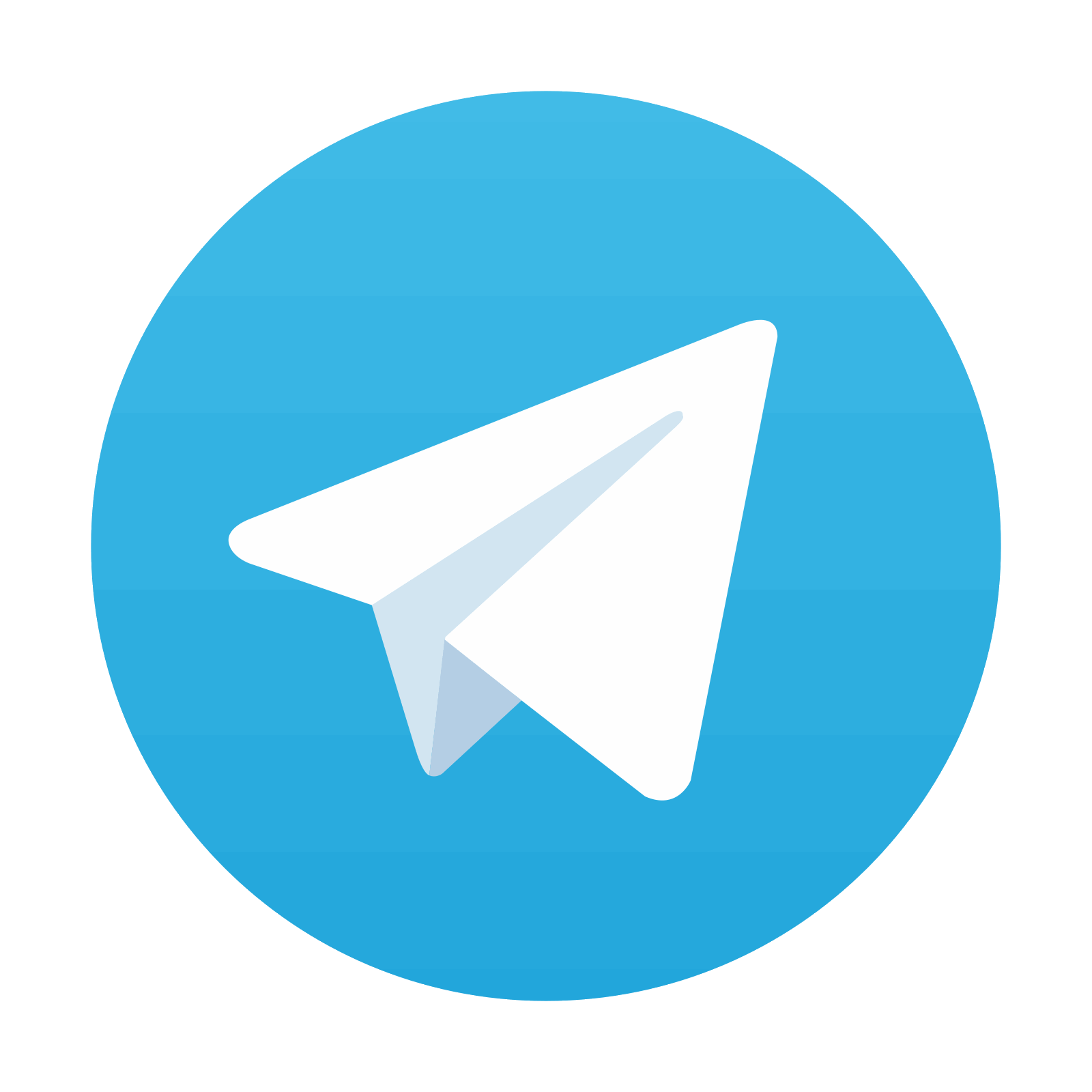
Stay updated, free articles. Join our Telegram channel

Full access? Get Clinical Tree
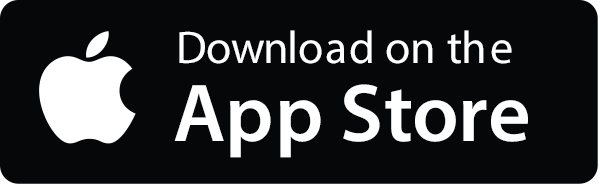
