RCTs
Observational studies
Strengths
Randomization
Large sample sizes and greater statistical power
Blinding
Greater external validity (more representative population)
Limitations
External validity is limited (usually highly selected population)
Case definition/validation
Short duration (limiting assessment of long-term efficacy and safety)
Selection bias, information bias, and confounding
Relatively small samples and low statistical power (limiting ability to detect rare harms)
Informative patient dropout and missing data
Occasionally not ethical (e.g., randomizing to a harmful exposure such as smoking)
In the case of administrative databases, secondary use of data that lack patient-level data (e.g., measures of disease activity, laboratory tests)
Recruitment challenging in rare diseases
Comparison of RCTs and Observational Study Designs
Evidence-based medicine is the application of the most valid scientific evidence to the care of patients. The strength of evidence is graded in large part based on study design, with randomized clinical trials (RCTs) receiving a higher grade than observational studies. Indeed, randomization and blinding are key attributes of RCTs that deal with the major problems due to confounding and other potential sources of bias inherent in observational studies.
However, RCTs are subject to some important limitations. First, RCTs may lack external validity. Indeed, to be clinically useful, the results of a study “must be relevant to a definable group of patients in a particular clinical setting”[5]. Common issues that potentially affect external validity of RCTs include the setting (e.g., recruitment from primary or academic care centers), eligibility criteria (e.g., magnitude of disease activity), exclusion criteria (e.g., patients with various comorbidities), characteristics of the study subjects (e.g., sociodemographic characteristics such as sex, ethnicity, and education), fixed treatment regimens and intense follow-up. Many examples of lack of generalizability in rheumatology are available. Several papers have been published showing that RA patients in routine care would not be eligible for major clinical trials of anti-TNF drugs, based on strict eligibility and exclusion criteria [6–8]. Geographic setting has also been recognized as a potential factor affecting generalizability. In 2011, belimumab was the first therapy in more than 50 years to be approved by the FDA for the treatment of systemic lupus erythematosus (SLE). At least half of the trial research done for belimumab was conducted outside North America and benefits were found to be consistently lower for subjects in the USA and Canada [9]. Factors contributing to such geographic differences were postulated to include variations in the underlying patient characteristics and variation in study execution. The increasing numbers of trials being conducted globally makes this threat to generalizability of growing concern. Finally, the exclusion of patients with comorbidity is particularly of concern in the context of drug safety rather than effectiveness, as these are the very same patients who may be susceptible to adverse events of the study drug.
Second, RCTs are expensive to undertake and for that reason are often of relatively short duration and underpowered to detect rare outcomes. This limits the ability of demonstrating long-term effectiveness or safety. Open-label extension (OLE) studies are often performed following the successful completion of an RCT and are reported commonly in the rheumatology literature [10]. Although the purpose of OLE studies includes collecting valuable long-term efficacy and safety data, OLE are not as robust as RCTs and should be viewed with considerable circumspection. At this point, the RCT has essentially turned into an observational study. Indeed, to begin with, the characteristics of the study participants who continue in an OLE study may differ significantly from the individuals who dropped out of the RCT. In addition, bias may also be introduced in the assessment of outcomes because of unblinding. Hence, OLE studies may underestimate side effects or harms because those who continue on treatment are those less likely to have dropped out or had side effects during the course of the RCT or overestimate treatment effects because assessments are no longer blinded. In addition, the lack of a comparator group limits the ability to draw robust conclusions from OLE studies. Indeed, the effect of treatment knowledge on behavior may be profound and lead to an inability to distinguish temporal trends from treatment-related effects. In a recent OLE study of strontium ranelate for the treatment of postmenopausal osteoporosis, the 10-year population consisted of only 7 % (237/3,352) of the original trial population [11]. Although the baseline characteristics of the OLE study group were representative of the original population, the possibility of confounding bias cannot be ruled out. The authors also acknowledged that the absence of a comparator group was an important limitation. Finally, it should be noted that if an original RCT was underpowered to detect rare harms, the likelihood of observing these rare harms in OLE remains low. Indeed, OLE of bisphosphonates for the treatment of postmenopausal osteoporosis failed to identify the increased risk of atypical femoral fractures associated with these drugs [12].
RCTs are not always feasible. In studies of harm in particular, it would not be ethical to randomize subjects to harmful exposures (e.g., smoking) and most RCTs are underpowered to detect rare harms.
Finally, RCTs for rare diseases can be particularly challenging logistically because of the difficulty in recruiting sufficient numbers of subjects. In such small RCTs, it may be particularly difficult to distinguish true negative results from false negative results due to low power. Systemic sclerosis is a relatively rare rheumatic disease and this has contributed to the fact that there have been few RCTs in this disease. One of the few RCTs in systemic sclerosis randomized a total of 71 early diffuse subjects to methotrexate or placebo [13]. The study found that methotrexate was associated with a nonsignificant (p > 0.05) improvement in two primary outcomes (modified Rodnan skin score and UCLA skin score) and a statistically significant benefit in physician global assessments of disease activity (p = 0.04), a third primary outcome, compared to placebo. The authors concluded that there was insufficient evidence to reject the null hypothesis of no treatment effect. Unfortunately, the study was labelled a “negative” trial, when in fact the study had been powered to find an optimistically large effect (35 % difference in skin scores over 1 year) and was therefore clearly underpowered to detect smaller but clinically relevant treatment effects. Indeed, a reanalysis of the data using Bayesian models, which make efficient use of all available data and present results that are more clinically relevant than that which is possible with a p-value from an RCT of a rare disease, found that there was 96 % probability that at least two of three primary outcomes were better on methotrexate compared to placebo [14]. The results of the initial RCT notwithstanding, methotrexate has continued to be commonly used in systemic sclerosis in routine clinical practice [15].
The limitations of RCTs highlight the fact that data from methodologically rigorous observational studies can be extremely valuable. Indeed, with improved methodology to minimize confounding and other biases, as well as newer statistical methods, estimates of treatment effects in observational studies are, for the most part, similar to those from those in RCTs [16–18]. In addition, by expanding the setting to more representative populations, observational studies of treatment effects in routine clinical settings, in particular among subjects who would not have been eligible for RCTs, are useful sources of data. For example, anti-TNF drugs have been shown to be effective in routine clinical practice for patients who would not have been eligible for RCTs albeit with more modest results [19, 20]. These important findings suggest that the current use of biologics in routine clinical practice may be suboptimal and alternative, more cost-effective ways of using these potent but expensive and potentially toxic drugs should be explored. Nevertheless, observational studies of the intended effects of a drug to assess effectiveness remain a challenge as they are subject to greater degree of confounding by indication.
Time-Related and Other Biases in Observational Studies
In routine clinical practice, exposure to drug therapy is not randomized, but rather dependent on a multitude of patient and physician characteristics. Thus, analysis of clinical data captured in registries and administrative datasets is generally subject to confounding bias and several other sources of bias. The biases are generally classified as selection, information, or confounding bias with different mechanisms described leading to these (Table 2) [21]. In the field of rheumatology, confounding by indication, channeling bias, immortal time bias, and depletion of susceptibles are among the more common mechanisms of bias that have threatened the validity of observational studies of registry and administrative health data.
Table 2
Summary of biases in observational studies
Definition | Examples | Clues for identification of bias | Possible solutions | |
---|---|---|---|---|
Selection bias | Selection or exclusion criteria for entering the study associated with both the drug exposure and the outcome | Depletion of susceptibles | Is the risk of the outcome a function of time, higher early after the initiation of drug exposure? | Equal cohort entry point for all drug exposure groups |
New-user designs | ||||
Information bias | Also known as measurement or classification bias; this bias results from the inaccurate determination of exposure or outcome | Immortal time bias | Is information about exposure classified in the same way for cases and comparisons? | Survival analysis with time-dependent exposures |
Confounding | Lack of comparability between drug groups under comparison. The observed association between an exposure and an outcome may be accounted by a third factor, when that factor is associated with both the exposure and outcome but is not in the causal pathway between the exposure and the outcome | Confounding by indication or confounding by disease severity | Could the outcome attributed to the drug also be an outcome of more severe disease? | Restriction, matching, stratification |
Multivariate regression techniques | ||||
Propensity score modeling | ||||
Channeling bias | Could the treatment have been preferentially prescribed to patients with special preexisting morbidity or because of ineffective current therapy? | Stratification |
Confounding by Indication
Confounding by indication may occur in observational studies if patients with more severe disease are preferentially prescribed selected, presumably more intensive, treatments (e.g., different drugs, regimes or doses) [22]. In such situations, differences in outcomes of treatment groups may be due to differences in baseline disease severity rather than treatment itself. Such confounding may affect results in different ways, including attenuating the true effect of treatment (i.e., making it harder to show the effect of treatment because those treated have more severe disease) or suggesting increased harms associated with treatment. In rheumatoid arthritis, for example, patients selected to receive anti-TNF drugs likely have more active disease than those who are not given these drugs. Yet, there is evidence to suggest that the risk of lymphoma in rheumatoid arthritis is particularly associated with disease activity [23]. Thus, increased rates of lymphoma associated with anti-TNF therapy could reflect, at least in part, confounding by indication, whereby patients with the highest risk of lymphoma preferentially receive anti-TNF drugs. The problem of confounding by indication is compounded by the fact that treatment decisions may be affected not only by differences in baseline disease severity but by the natural course of the disease and by treatment response, which can both be subject to considerable interindividual variation.
Various statistical techniques can be used to minimize the effect of confounding by indication in observational studies, including multivariate regression analyses, traditional propensity scores, high-dimensional propensity scores, and instrumental variables to adjust for both baseline and time-dependent confounders. In recent years, studies have also been reported using inverse-probability-weighted (also called inverse-propensity-weighted) marginal structural models [24–27]. Simply put, this type of analysis attempts to balance potential confounders among treated and untreated subjects by reweighing observations according to the inverse of the probability of receiving their observed drug exposure. The approach is similar to analysis via propensity matching estimators, applied over time to account for changing values of the confounders [28]. By reweighing, rather than matching subjects, one is able to make use of all of the subject level data available for the analysis and account for their changes over time. Another advantage of weighting is that fewer assumptions need to be made about the underlying probability models [24]. Finally, missing data due to subject dropout can be accounted for in a straightforward manner by incorporating the estimated probability of study completion in the weight for each subject [25]. Thus, marginal structural models attempt to correct for bias due to confounding (both due to the observational nature of the data and time-varying confounding) and bias due to subject dropout and to estimate the causal effect of treatment.
An example of confounding by indication and statistical adjustment for this comes from a study using the Norfolk Arthritis Register to investigate the benefit of disease-modifying antirheumatic drugs (DMARD) treatment on the long-term functional outcomes of patients with inflammatory polyarthritis [29]. The investigators acknowledged that, in the setting of an observational cohort study, the effect of treatment on outcomes could be confounded by differences both in baseline and time-dependent disease characteristics. They therefore used marginal structural models to adjust for time-dependent confounding. They reported on 642 subjects who had completed a Health Assessment Questionnaire (HAQ) both at baseline and at the 10-year assessment. Of these, 54 % had been treated with DMARDs by 10 years. As expected, patients who did not require DMARDs during 10 years of follow-up had better baseline HAQ scores (median 0.50, IQR 0.13; 0.88) and smaller mean change in HAQ over the follow-up period (0.13; 95 % CI 0.05, 0.21) than those who were treated (baseline median HAQ 1.00; IQR 0.50, 1.50 and mean change over 10 years of 0.24; 95 % CI 0.14, 0.33). When adjusted only for baseline differences in HAQ scores, those ever treated with DMARDs had a significantly greater deterioration in function over 10 years than those never treated (adjusted mean difference in change in HAQ 0.30; 95 % CI 0.18, 0.42). However, after adjustment for the time-dependent confounders using a weighted structural marginal model, there was no significant difference in the change in HAQ between those ever treated and those not treated (−0.01; 95 % CI −0.20, 0.19). In other words, after allowing for the fact that treatment was more likely to be given to those with severe inflammatory polyarthritis, treatment appeared to move patients onto a trajectory that they would have followed if they had had milder disease not requiring treatment. Although the possibility of residual confounding cannot be excluded even using this type of sophisticated statistical approach, the magnitude of confounding by indication appears to be greatly mitigated by it.
Channeling Bias
Channeling bias is a form of confounding by indication that involves not only disease status but also individual patient profiles and medication use tailored to this. In other words, patients at high risk for a given complication may be preferentially prescribed or switched to a certain treatment because an alternative treatment is known to be associated with that particular complication. Thus, one subtle difference between confounding by indication and channeling bias is that in the former case the confounding results from a patient’s indication for a certain treatment, whereas in the latter case, it results from a contraindication to that treatment. Another is that channeling bias generally also involves switching of treatment from an older to a newer agent.
In a population-based safety study examining the association between leflunomide and interstitial lung disease (ILD) using a large claims database, we found that in the overall analysis, leflunomide (rate ratio 1.9), but not methotrexate (rate ratio 1.4), was associated with the risk of ILD [30]. We found that patients with a history of ILD were almost twice as likely to have received leflunomide compared to methotrexate (adjusted odds ratio 1.9; 95 % CI 1.5, 2.3) as a first DMARD. In a stratified analysis, we showed that in patients without prior exposure to methotrexate and without a history of ILD, methotrexate (rate ratio 3.1) but not leflunomide (rate ratio 1.2) was associated with ILD, whereas in the subgroup of patients with either a prior exposure to methotrexate or a history of ILD, methotrexate was highly protective against ILD (rate ratio 0.4) and leflunomide was associated with a significant increase (rate ratio 2.6) in the risk of ILD. We concluded that this stratified analysis provided strong evidence that patients with a history of ILD may have been preferentially prescribed leflunomide rather than methotrexate on the assumption that, in contrast to methotrexate, no lung toxicity was known to be associated with leflunomide. Thus, channeling bias must be considered in studies of harm and proper stratified analysis of the data is necessary to determine whether this bias may have influenced the results.
Immortal Time Bias
A study investigated whether the use of antimalarials in patients with systemic lupus erythematosus (SLE) could be associated with cancer incidence [31]. The authors used a cohort of 235 SLE patients followed for up to 31 years, of which 13 patients developed cancer during follow-up. The comparison of time to cancer incidence was based on comparing the 156 patients who had “ever” received antimalarials during follow-up with the 79 who did not. The Cox proportional hazards model was used to estimate the adjusted hazard ratio of 0.15 (95 % CI 0.02, 0.99). This result implied that the incidence of cancer of all types could be significantly reduced by 85 % in SLE patients treated with antimalarials.
However, this analysis was subject to a bias created by looking at “ever” exposure to antimalarials during follow-up. Immortal time refers to a time period during cohort follow-up when, by design, subjects cannot die or have the outcome event under study [32, 33]. Thus, exposed patients are necessarily “immortal” (in this case cancer-free) during the time span between cohort entry and the first prescription for an antimalarial. On the other hand, the comparison patients who did not receive antimalarials had no such cancer-free period as they could have developed cancer anytime during follow-up. Thus, the comparison of the time to cancer incidence between these two groups provided an advantage to exposed patients because they were guaranteed, by design, a cancer-free period. To the extent that this immortal time period is in fact unexposed but misclassified as exposed, the immortal time bias is a form of information bias. This type of bias will result in lowering the rate ratio (i.e., closer to the null if the effect is harmful (>1) or away from the null if the effect is nil or protective (<1)).
A time-dependent Cox proportional hazard model or similar approach to data analysis that classifies the person-time from cohort entry until the first prescription as unexposed and the subsequent person-time as exposed is a simple approach to avoid an immortal time bias. We replicated the abovementioned study in a population-based cohort of 23,810 rheumatoid arthritis patients, identified from provincial healthcare databases between 1980 and 2003 [34]. We identified all cancer cases occurring during follow-up and obtained information on the timing of antimalarial agents, as well as all relevant concomitant medications. The analysis was based on an approach that considered the time-dependent nature of the antimalarial prescriptions and classified the time prior to the first one correctly as unexposed. As a result, the adjusted rate ratio of cancer incidence with antimalarial use was 1.1 (95 % CI 0.9, 1.3). This is quite different from the protective effect reported using the approach subject to immortal time bias described above.
Other examples of immortal time bias are found in the rheumatology literature. In a study from the LUMINA cohort, the use of hydroxychloroquine was reported to reduce the incidence of renal damage by 88 % (hazard ratio 0.12; 95 % CI 0.02, 0.97) [35]. However, exposure to hydroxychloroquine was measured as “any use during the follow-up period” (i.e., ever/never used). In so doing, unexposed person-time from cohort entry to the start of actual exposure was misclassified as exposed. This “immortal” time period during which the outcome under study could not have occurred conferred an undue advantage to the exposed group. As a result, the protective effect of hydroxychloroquine was overestimated [36]. Another similar example involving hydroxychloroquine comes from the ARAMIS cohort of rheumatoid arthritis patients. In that study, 4 years or more of exposure was associated with a very significant 77 % reduction in the incidence of diabetes (hazard ratio 0.23; 95 % CI 0.11, 0.50) [37]. Immortal time bias was introduced by the inherent requirement of 4 years with no diabetes to determine the exposure, whereas the nonexposed reference patients were permitted, by the analysis, to develop diabetes as of day 1 of cohort entry.
Depletion of Susceptibles
The time-varying hazard functions of several medications commonly used in rheumatology have been described, for example, the higher risk of infection present with early exposure to TNF antagonists [38–40] and the higher risk of myocardial infarction or acute renal failure associated with early exposure to rofecoxib [41, 42] and other NSAIDs [42]. If a study sample is underrepresented by those individuals most susceptible to an event possibly because of early attrition due to the development of a complication and overrepresented by low-risk individuals who tolerate the drug, a depletion of susceptible bias can result and will tend to underestimate the magnitude of harm associated with a treatment.
A depletion of susceptible bias can result from study design or analysis. In a study using a large, population-based administrative database designed to examine the risk of myocardial infarction associated with COX-2 inhibitors, only those individuals who were given at least two successive prescriptions of the drugs of interest were included (with the purported intent of excluding “sporadic” users of NSAIDs) [43]. The authors found no increase in the risk of myocardial infarction associated with the use of rofecoxib compared to controls. It is possible that excluding subjects who received only one rofecoxib prescription may have excluded patients among the most susceptible to the increased risk in myocardial infarction that has been since confirmed with rofecoxib and that this may have contributed to an underestimation of the true risk.
Misspecification of the “at-risk” period may also result in a depletion of susceptible bias. Dixon et al. provided an excellent example using the British Biologic Register [38]. This group had previously reported no increase in the risk of serious infection associated with TNF antagonists in rheumatoid arthritis [44]. However, they subsequently reanalyzed their data and found that the magnitude of the risk of infection varied depending on different definitions of the “at-risk” period. For example, when the at-risk period was defined as “receiving treatment,” there was no significant risk of infection associated with anti-TNF therapy (adjusted incidence rate ratio 1.22; 95 % CI 0.88, 1.69). However, there was a strong trend towards increased risk when the at-risk period was defined as “ever receiving treatment” (adjusted incidence rate ratio 1.35; 95 % CI 0.99, 1.85). They concluded that these result were consistent with a “depletion of susceptible” effect, whereby in an analysis with follow-up limited to the period of exposure, those at greater risk of infections are excluded from the analysis early and those who continue to receive treatment are really a healthier group at an overall lower risk. Care in defining the risk window and the use of sensitivity analyses to investigate alternative definitions of exposure can help to address this potential bias.
Finally, the depletion of susceptible phenomenon can have a major impact in prevalent cohort studies, in other words studies that include prevalent users of a drug, because of the exclusion of subjects in the early high-risk period and the over-representation of lower-risk subjects who survived this early high-risk period. The “new-user” design has been proposed as solution to overcome this problem [45].
The Quagmire of Biologics and Malignancies
The relative strengths and limitations of RCTs and observational studies, and the challenges in practicing evidence-based medicine are highlighted by the controversy surrounding the association between biologics and malignancies in RA. A thorough review by Chakravarty et al. found that RA was not associated with a significantly increased overall risk of malignancies compared to the general population [46]. On the other hand, a subsequent meta-analysis of 21 observational studies by Smitten et al. found a small but significant increase in overall risk (standardized incidence ratio (SIR) 1.05, 95 % CI 1.01, 1.09) [47]. More importantly, though, this meta-analysis, demonstrated that such overall findings obscure the more informative fact that RA may be associated with an increase in some and a decrease in other site-specific malignancies. Indeed, in this meta-analysis, there was an increase in the risk of lymphoma (SIR 2.08, 95 % CI 1.80–2.39) and lung cancer (SIR 1.63, 95 % CI 1.43–1.987), but a decrease in the risk of colorectal (SIR 0.77; 95 % CI 0.65–0.90) and breast cancer (SIR 0.84; 95 % CI 0.79, 0.90) [47].
This study underscores the fact that the relationship between RA and malignancies is complex. There are multiple theoretical pathways by which RA and malignancies may be associated [48]. Two such pathways are the disease per se and the drugs used to treat the disease. As far as the disease is concerned, autoimmune dysfunction and chronic inflammation have been proposed as mechanisms whereby the risk of certain malignancies, in particular lymphoproliferative cancers, may be increased in RA. In particular, higher disease severity in RA has been associated with a greater risk of lymphoma [23]. On the other hand, drugs used to treat RA modulate the immune system and may, also in part, be responsible for the increased risk of malignancy [49]. In particular, cytokine pathways, including tumor necrosis factor (TNF), play an important role in tumor surveillance. Thus, blocking this pathway with anti-TNF drugs could theoretically contribute to an increased risk of malignancy. Moreover, lacking a randomized trial, it may be difficult to tease apart the effects of the disease and the drugs to the extent that stronger immunosuppression is used in more severe disease, so that any observational study would be subject to intractable confounding by disease severity. Finally, to add to the complexity, the question of how the possible risks of malignancy resulting from the disease or the drugs relate, whether additively, synergistically, or perhaps negatively with, for example, the reduction of the chronic inflammation by the drugs mitigating the risk associated with the disease itself, remains unresolved [50].
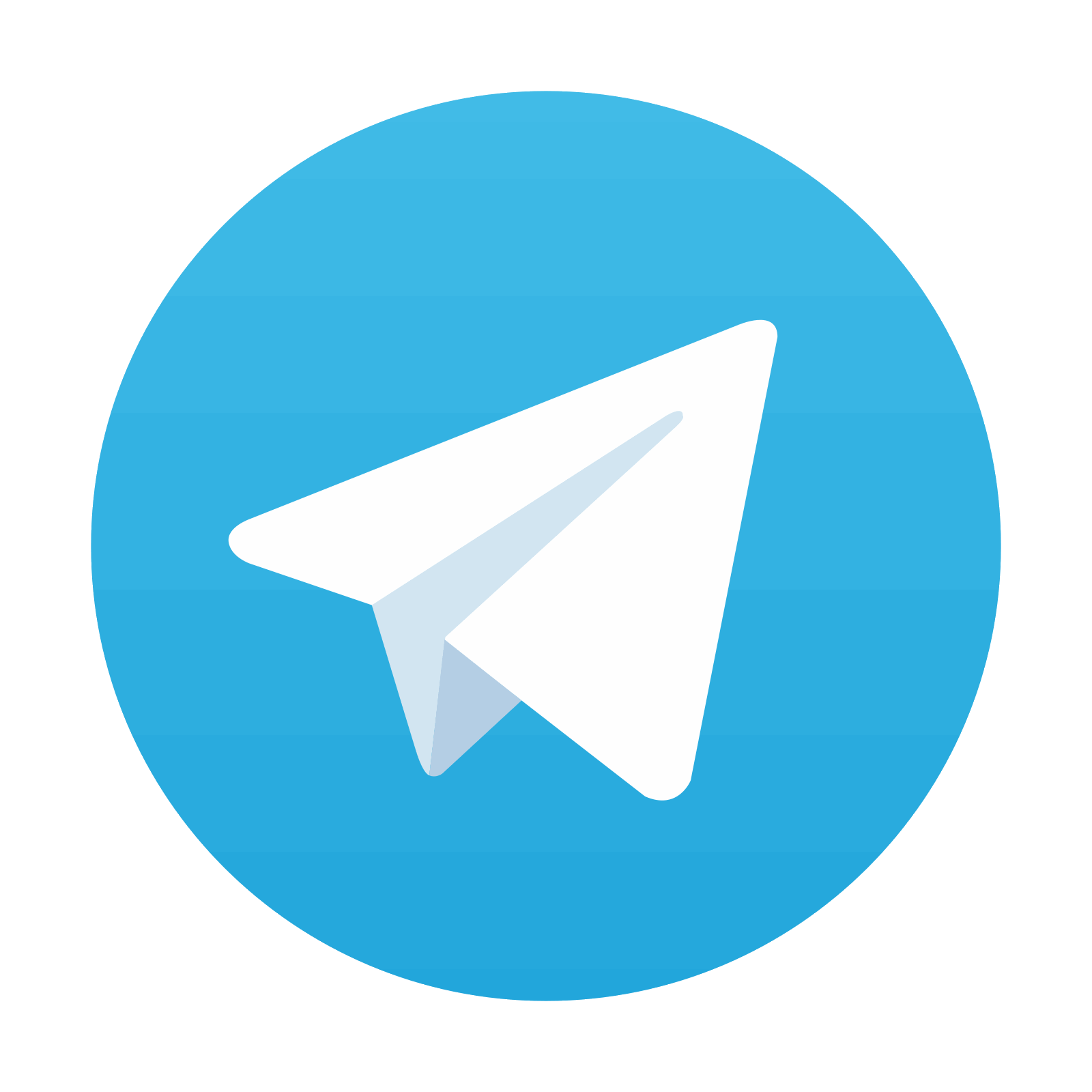
Stay updated, free articles. Join our Telegram channel

Full access? Get Clinical Tree
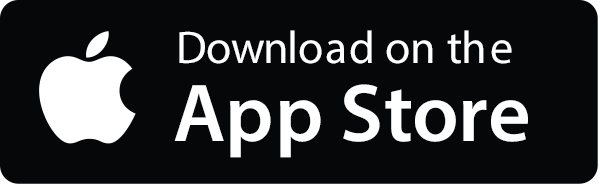
