Fig. 8.1
A comprehensive model for injury causation (Bahr R, Krosshaug T (2005) Understanding the injury mechanisms – a key component to prevent injuries in sport. Br J Sports Med 39:324–329)
8.2 Anterior Cruciate Ligament Injuries
Studies from handball football and other sports has shown that non-contact ACL injuries can be prevented through training programs focusing on knee control, balance, technique and strength (Renstrom et al. 2008). There exist some solid knowledge on the risk factors, e.g. that females are at higher risk compared to men, that match situations involves higher risk than training and that higher surface friction will increase the likelihood of sustaining an injury (Renstrom et al. 2008). However, there is still a lack of knowledge on all the numerous potential risk factors that have been suggested in the literature. The same is true for the injury mechanisms where different injury scenarios are heavily debated in the scientific community over the later years (Yu and Garrett 2007; Quatman and Hewett 2009). What seems to be clear is that most of the ACL injuries in team sports like handball, basketball and football are non-contact injuries occurring in cutting and landing movements. However, there exist several hypotheses for what joint loading that are causing the injury. The “Quadriceps drawer” theory suggests that the quadriceps muscles, through the patellar ligament, will pull the tibia forward relative to the femur. This effect is highest at low knee flexion angles. Valgus bending, internal rotation and vertical compression are other suggested mechanisms that have been suggested to load the ACL, but it is still unknown how important the different loading mechanisms are. This lack of understanding limits our ability to design optimal preventive measures (Krosshaug et al. 2005).
8.3 Research Approaches to Investigate Injury Mechanisms
So how can we then investigate injury mechanisms? From a systematic literature review eight different research approaches were identified. These methods were athlete interview, video analysis, motion analysis in the lab, in-vivo measurements of force/strain in the tissue, cadaver studies, mathematical modeling and simulation, clinical studies (MRI, CT) and in rare cases – accidents that may occur during biomechanical experiments (Krosshaug et al. 2005).
8.4 The Importance of Video Analysis
With exception of the last category (accidents during research experiments), video analysis is the only method where we can objectively describe the motion patterns that are involved in a real injury situation. Such kinematical estimates from real injury situations are essential for several reasons: In addition to the information that can be directly interpreted, these data are also necessary to validate studies that attempt to simulate the injury situation (e.g. cadaver studies, mathematical modeling, motion analysis in the lab and in-vivo studies). Such simulations must produce similar motions to those which are observed in real injury situations in order to be valid. If not, we may risk simulating situations that rarely or never occurs in real life. Additionally, video analysis may be used to describe other important aspects of the injury situation, not only biomechanical information, but information related to the game situation or player/opponent behavior as well. Such information can be used to design preventive measures. Video analysis has therefore been utilized in different sports to study various injury types. Andersen and co-workers (Andersen et al. 2003, 2004a, b) customized methods, previously used in game analysis, to study ankle sprains and head injuries in football. McIntosh and co-workers used video analysis to quantify head velocity during impacts in Australian Rules Football (McIntosh et al. 2000). These studies did, however, not attempted to quantify joint motion.
8.5 Video Analysis of ACL Injuries in Ball/Team Sports
Several video analysis studies have been conducted to investigate ACL injury mechanisms in ball/team sports (Olsen et al. 2004; Boden et al. 2000; Cochrane et al. 2007). These studies concluded that the knee is relatively straight at the moment of injury. Furthermore, is has been reported that the knee was either internally or externally rotated and in many cases in combination with valgus or valgus collapse. Some of these studies have also attempted to quantify joint motion (Olsen et al. 2004; Cochrane et al. 2007).
Unfortunately, there are several obvious limitations in these studies. They are based on relatively few cases and the video quality was in many cases poor, making it difficult to assess joint motion and the time point of injury (Olsen et al. 2004). However, the main problem with previous studies was the fact that only simple visual inspection, e.g. merely “guesstimating”, was used to assess the joint motion.
8.6 The Validity of Simple, Visual Inspection
To investigate the accuracy of visual inspection, a systematic study was carried out where three test subjects performed sport specific movements (Krosshaug et al. 2007a). The movements were filmed with three ordinary consumer cameras; front, side and rear. The test subjects had 33 reflective markers attached to anatomical landmarks. Eight infrared, high-speed cameras (ProReflex, Qualisys Inc., Gothenburg, Sweden.) recorded the marker paths, enabling accurate measurement of joint angles in three dimensions.
The study revealed that experienced researchers were unable to produce reliable estimates of joint angles. Knee flexion angles were underestimated by 19° on average (Fig. 8.2). This implies that we must be cautious when interpreting results from studies using this approach. In other words, we can not trust that the injuries occur with a straight leg. As can be seen from Fig. 8.2, the experienced researchers estimated the knee flexion angle to be 30° when it in fact was 60°!
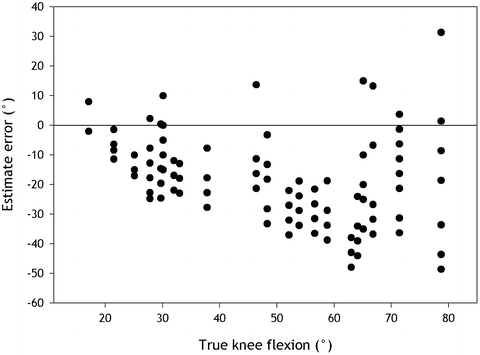
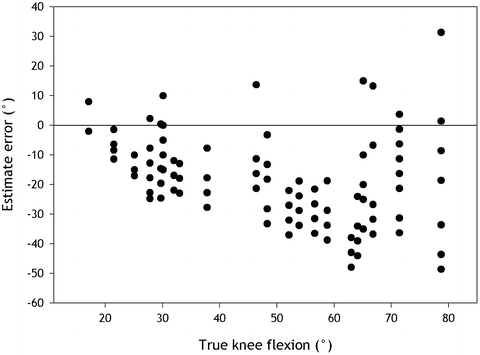
Fig. 8.2
Error estimates of knee joint angles as a function of true joint angle, as measured with a marker-based motion capture system (Krosshaug T, Nakamae A, Boden B, Engebretsen L, Smith G, Slauterbeck J, Hewett TE, Bahr R (2007) Estimating 3D joint kinematics from video sequences of running and cutting maneuvers – assessing the accuracy of simple visual inspection. Gait Posture 26:378–385)
8.7 Development of a Model-Based Image-Matching (MBIM) Method for 3D Motion Reconstruction from Uncalibrated Camera Sequences
As a result of the poor results from visual inspection, there was a need for a new, more accurate and less subjective method. To make possible a more detailed biomechanical analysis, it was desirable to find a method that could produce continuous estimates of joint kinematics. A literature review showed that such methods had already been developed (Halvorsen 2002; Trewartha et al. 2001). However these methods were designed with automatic motion reconstruction in mind rather than accuracy. Additionally these methods could not be used for analyzing injury videos since they required e.g. fixed cameras, clothes with color codes, one-colored background or other simplifications in order to work. For this reason it was necessary to develop a new method for 3D reconstruction of injury situations. A solution to the problem was to manually configure a computerized skeleton model to imitate the injured person by superimposing it onto the video. This method is based on commercially available software Poser® 4 Pro Pack (Curious Labs, Inc., Santa Cruz, California, USA). Poser has the possibility to import video from up to four video cameras.
In order to reconstruction the injury situation in the computer, it is necessary to build a model of the surroundings (Fig. 8.3), as well as a customized skeleton model of the person of interest (Fig. 8.4). The model of the surroundings can for instance utilize the standard measurements of e.g. a handball court, including exact positioning of floor markings, commercial boards, goal posts etc.
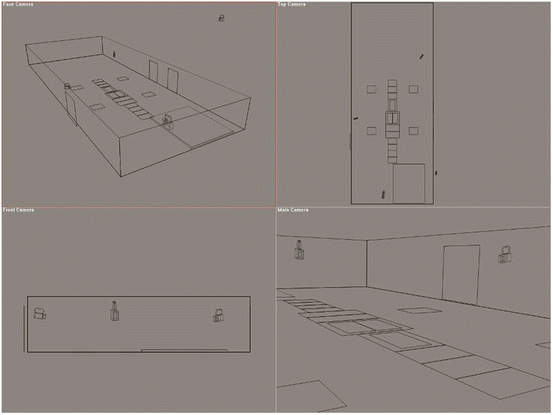
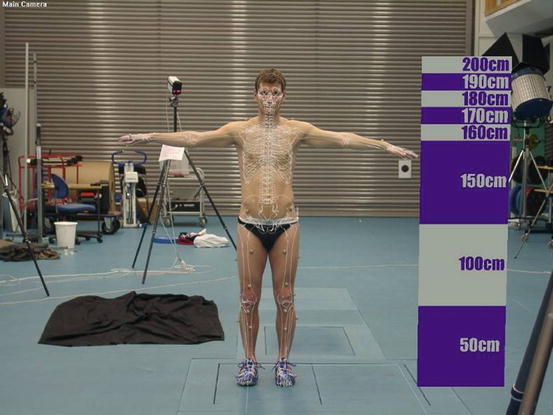
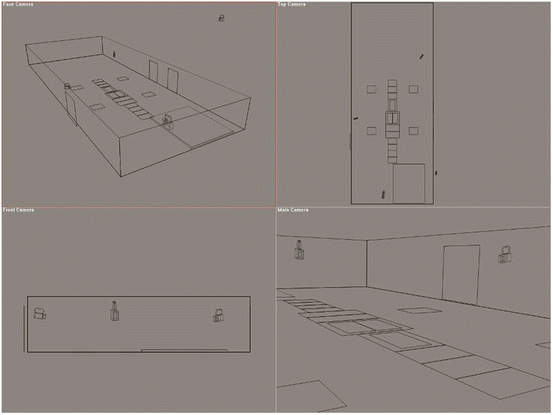
Fig. 8.3
Customizing the model of the surroundings. A computer model of the surroundings is built based on exact field measurements
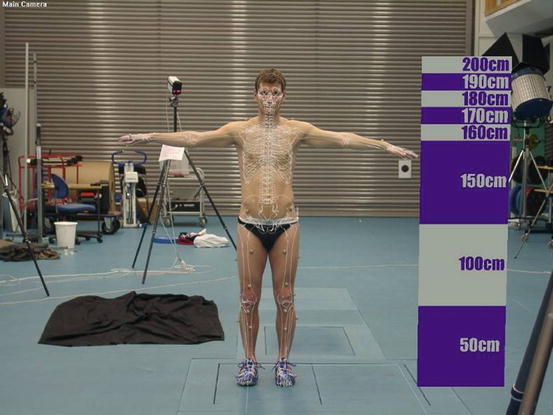
Fig. 8.4
Customizing the skeleton model. The model dimensions are adjusted to the exact measurements of the subject. If these measurements are not available, scaling of the segments will be part of the matching process
The skeleton model consists of 21 segments (forefoot, rear foot, tibia, femur, pelvis, lower spine, upper spine/chest, neck, head, shoulder girdle, humerus, ulna, hand). All joints have three degrees of freedom with the exception of the sternoclavicular, elbow and hand joint which are assigned with two degrees of freedom. In addition, the pelvis has three rotational and three translational degrees of freedom. In total, this gives 57 degrees of freedom for the skeleton model. All segments are individually customized to the body dimensions of the person by scaling along the three axes of the segment. In the cases where this information is not available, scaling of the segments will be part of the manual matching process.
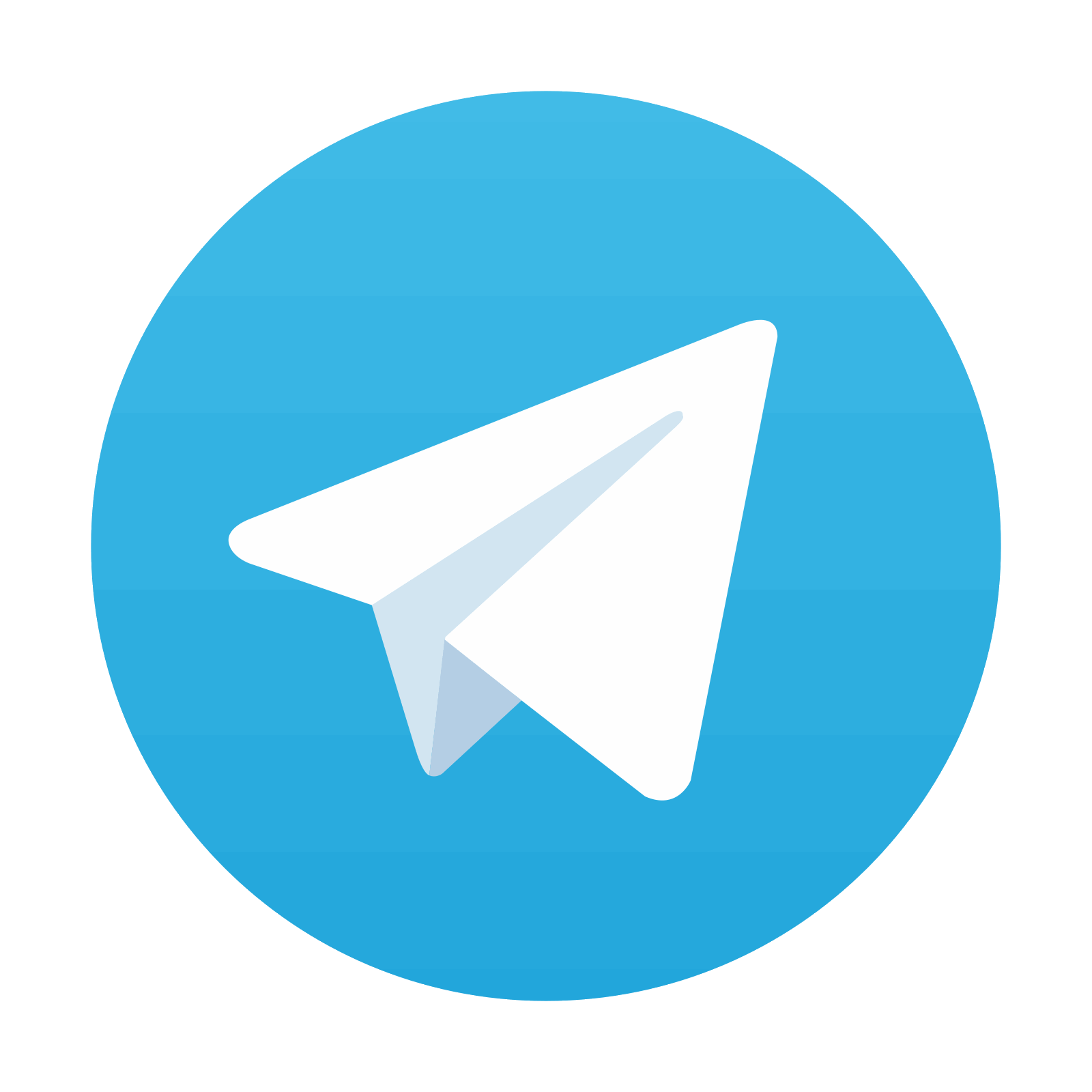
Stay updated, free articles. Join our Telegram channel

Full access? Get Clinical Tree
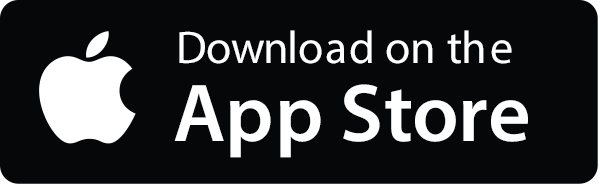
