This article reviews recently proposed clinical tools for predicting risks and outcomes in total hip arthroplasty and total knee arthroplasty patients. Additionally, we share the Massachusetts General Hospital experience with using the Risk Assessment and Prediction Tool to predict the need for an extended care facility after total joint arthroplasty.
Key points
- •
Clinical tools have been developed to help predict outcomes in total joint arthroplasty (TJA) patients to aid efficient care delivery as demand for TJA increases.
- •
The Risk Assessment and Prediction Tool (RAPT) uses preoperative patient factors to predict patient need for an extended care facility after TJA. Our experience shows that length of stay and percentage of patients discharged home can be improved.
- •
The Predicting Location after Arthroplasty Nomogram (PLAN) is an alternative tool for predicting patient discharge needs.
- •
The Morbidity and Mortality Acute Predictor (arthro-MAP) uses patient characteristics and intraoperative factors to predict a patient’s probability of significant postoperative complications.
- •
The Penn Arthroplasty Risk Score predicts a patient’s need for postoperative intensive care unit monitoring.
Introduction
The demand for total knee arthroplasty (TKA) and total hip arthroplasty (THA) in the United States is growing rapidly. Kurtz and colleagues projected the demand for primary THA to grow 174% to 572,000, and primary TKA to grow by 673% to 3.48 million procedures per year by 2030. Demand for THA and TKA revision procedures is likewise expected to experience a large increase in demand of 147% and 601% by 2030, respectively. Hospital costs associated with total joint arthroplasty (TJA) were estimated at $30 billion in 2004, and are expected to increase as demand increases.
TKA and THA are considered safe, long-term, cost-effective treatments for osteoarthritis and it is therefore desirable that the growing demand be met. The predicted shortage of qualified arthroplasty surgeons in relation to the increasing demand represents an additional challenge. The United States health care system’s ability to meet the rapidly growing demand will be predicated on safe and efficient delivery.
The capacity to predict patient outcomes and needs preoperatively allows more efficient delivery of care, which increases the ability of joint replacement surgeons to meet this demand. Numerous clinical tools have been developed to predict a variety of TJA patient outcomes ( Table 1 ). Such tools may facilitate efficient resource utilization by helping select better candidates for TJA, anticipating resource-intensive intensive care unit (ICU) monitoring, and encouraging timely planning for patient transition to an extended care facility. These tools may also allow for early planning of discharge and postoperative care, potentially reducing hospital length of stay (LOS). The financial savings associated with decreasing LOS have been documented extensively. However, to meet the goal of increasing the productivity of joint replacement surgeons, tools must predict accurately meaningful clinical outcomes and be generalizable widely.
Study | Study Design | Patients (n) | Tool Name | Predicts | Variables Measured | Internal C-Statistic | Notes |
---|---|---|---|---|---|---|---|
Oldmeadow et al, 2003 | Prospective | 520 | RAPT | Postoperative rehabilitation need | (6) Age, gender, preoperative walking distance, gait aid, community support, home caregiver | 0.75 | Based on Australian population |
Barsoum et al, 2010 | Retrospective | 517 | PLAN | Postoperative rehabilitation need | (17) Type of surgery, age, gender, BMI, comorbidities, preoperative ambulation status, predicted postoperative ambulation status, home environment variables (no. of steps, bedroom on first or second floor, bathroom on first or second floor), baseline caregiver assistance, home distance relative to the OR | 0.867 | Externally Validated |
Wuerz et al, 2014 | Retrospective | 3511 | arthro-MAP | Postoperative complications | (8) Lowest intraoperative HR, EBL, preoperative BUN, procedure type, race, ASA score, comorbidities, presence of fracture | 0.76 | Awaiting external validation |
Courtney et al, 2014 | Retrospective | 1594 | PARS | Postoperative ICU care need | (5) COPD, CHF, CAD, EBL >1000, intraoperative vasopressor use | 0.822 | 7-point scale, with postoperative ICU care recommended if ≥3 points |
Sabry et al, 2014 | Retrospective | 314 | Unnamed nomogram | Reinfection after 2-stage TKA revision for infection | (12) BMI, time from index surgery, duration of symptoms, number of previous surgeries, preoperative hemoglobin, soft tissue coverage required, prior infection in the same joint, previous 2-stage revision, type of organism, diabetes, immunocompromise, and heart disease | 0.773 | Not externally validated |
This article reviews recently proposed clinical tools for predicting risks and outcomes in THA and TKA patients. Our definition of a clinical tool includes “scoring systems, nomograms, and algorithms using preoperative or operative factors to predict outcomes or events in THA or TKA.” Additionally, we share the Massachusetts General Hospital (MGH) experience with using the RAPT to predict the need for an extended care facility after TJA.
Introduction
The demand for total knee arthroplasty (TKA) and total hip arthroplasty (THA) in the United States is growing rapidly. Kurtz and colleagues projected the demand for primary THA to grow 174% to 572,000, and primary TKA to grow by 673% to 3.48 million procedures per year by 2030. Demand for THA and TKA revision procedures is likewise expected to experience a large increase in demand of 147% and 601% by 2030, respectively. Hospital costs associated with total joint arthroplasty (TJA) were estimated at $30 billion in 2004, and are expected to increase as demand increases.
TKA and THA are considered safe, long-term, cost-effective treatments for osteoarthritis and it is therefore desirable that the growing demand be met. The predicted shortage of qualified arthroplasty surgeons in relation to the increasing demand represents an additional challenge. The United States health care system’s ability to meet the rapidly growing demand will be predicated on safe and efficient delivery.
The capacity to predict patient outcomes and needs preoperatively allows more efficient delivery of care, which increases the ability of joint replacement surgeons to meet this demand. Numerous clinical tools have been developed to predict a variety of TJA patient outcomes ( Table 1 ). Such tools may facilitate efficient resource utilization by helping select better candidates for TJA, anticipating resource-intensive intensive care unit (ICU) monitoring, and encouraging timely planning for patient transition to an extended care facility. These tools may also allow for early planning of discharge and postoperative care, potentially reducing hospital length of stay (LOS). The financial savings associated with decreasing LOS have been documented extensively. However, to meet the goal of increasing the productivity of joint replacement surgeons, tools must predict accurately meaningful clinical outcomes and be generalizable widely.
Study | Study Design | Patients (n) | Tool Name | Predicts | Variables Measured | Internal C-Statistic | Notes |
---|---|---|---|---|---|---|---|
Oldmeadow et al, 2003 | Prospective | 520 | RAPT | Postoperative rehabilitation need | (6) Age, gender, preoperative walking distance, gait aid, community support, home caregiver | 0.75 | Based on Australian population |
Barsoum et al, 2010 | Retrospective | 517 | PLAN | Postoperative rehabilitation need | (17) Type of surgery, age, gender, BMI, comorbidities, preoperative ambulation status, predicted postoperative ambulation status, home environment variables (no. of steps, bedroom on first or second floor, bathroom on first or second floor), baseline caregiver assistance, home distance relative to the OR | 0.867 | Externally Validated |
Wuerz et al, 2014 | Retrospective | 3511 | arthro-MAP | Postoperative complications | (8) Lowest intraoperative HR, EBL, preoperative BUN, procedure type, race, ASA score, comorbidities, presence of fracture | 0.76 | Awaiting external validation |
Courtney et al, 2014 | Retrospective | 1594 | PARS | Postoperative ICU care need | (5) COPD, CHF, CAD, EBL >1000, intraoperative vasopressor use | 0.822 | 7-point scale, with postoperative ICU care recommended if ≥3 points |
Sabry et al, 2014 | Retrospective | 314 | Unnamed nomogram | Reinfection after 2-stage TKA revision for infection | (12) BMI, time from index surgery, duration of symptoms, number of previous surgeries, preoperative hemoglobin, soft tissue coverage required, prior infection in the same joint, previous 2-stage revision, type of organism, diabetes, immunocompromise, and heart disease | 0.773 | Not externally validated |
This article reviews recently proposed clinical tools for predicting risks and outcomes in THA and TKA patients. Our definition of a clinical tool includes “scoring systems, nomograms, and algorithms using preoperative or operative factors to predict outcomes or events in THA or TKA.” Additionally, we share the Massachusetts General Hospital (MGH) experience with using the RAPT to predict the need for an extended care facility after TJA.
Development of clinical tools
Many recently proposed clinical tools have been developed using similar methodology, although the specific statistical methods may vary. First, an outcome of interest is identified. The outcome is often binary or categorical, such as postoperative discharge to an extended care facility. Next, based on a literature review and often expert opinion, a number of variables are identified that may be associated with the outcome of interest. These variables may include demographic data, comorbidities, preoperative function scores using validated instruments, or intraoperative factors such as estimated blood loss (EBL). These data, as well as the outcome of interest, are collected from a cohort of TJA patients, either retrospectively or prospectively. Univariate logistic regression is often first used to explore the association of each variable independently with the outcome of interest. Then, multiple logistic regression is used frequently to explore how well multiple variables explain the outcome of interest when used simultaneously. Variables are often considered for inclusion one at a time to assess whether they improve substantially how well the logistic regression model fits the observed data.
The predictive power of a model is typically evaluated using a C-statistic, which determines if the model predicts the outcome better than chance alone. This is also known as the area under the receiver operating characteristic curve. Models are typically considered reasonable when the C-statistic is greater than 0.7 and strong when it exceeds 0.8. The final multiple logistic regression model coefficients are subsequently converted into a more easily utilized clinical risk prediction tool or nomogram.
Validation of the tool is essential before using it in clinical practice. Most authors use “internal validation.” Internal validation is based on the study’s internal dataset, often using the C-statistic as a summary measurement. Internal validation may overestimate the performance of the tool. External validation aims to address the accuracy of a model in patients from a different population with the same underlying disease. This step is particularly important for relatively small datasets.
Prediction tools for discharge to extended care facilities
Tools allowing the surgeon to predict the need for discharge to an extended care facility could potentially increase efficiency by reducing LOS in the acute setting. Such tools include the Risk Assessment and Prediction Tool (RAPT) and the Predicting Location after Arthroplasty Nomogram (PLAN).
The RAPT was developed in Australia in 2003 by Oldmeadow and colleagues to predict whether TJA patients would require discharge to an extended care facility ( Fig. 1 ). The system was developed using a cohort of patients that included primary THA and TKA patients, as well as revision and hemiarthroplasty patients. The tool uses 6 variables, including age, gender, preoperative ambulatory distance, use of a gait aid, community support, and presence of a home caregiver. The tool is scored on a 12-point scale, with fewer than 6 points predicting need for an extended care facility, and more than 9 points predicting discharge directly home. The tool correctly predicted discharge destination in 75% of the study sample.
In a follow-up study, the authors used the RAPT on 50 consecutive patients and found an increase in home discharges from 34% to 64%, an average 1.1-day decrease in LOS, and no increase in readmission rates. Other authors have used the RAPT, demonstrating good predictive accuracy and a low relative risk of complications in patients with a RAPT score of greater than 9. In contrast with many of the other scores, the RAPT has been validated in multiple countries and continents with relatively similar results between the different institutions.
The PLAN was developed by the Cleveland Clinic to predict post-acute care needs as an alternative to RAPT. The authors were concerned that the RAPT may not be generalizable to US patients, particularly given that the average LOS in Australia at the time of the RAPT’s development was 8.6 days. In contrast with the RAPT’s 6 variables, the PLAN includes 17 preoperative variables ( Fig. 2 ), and had a C-statistic of 0.867 with the study’s internal sample of 517 patients. This compared favorably with only 0.565 for the RAPT. The authors also externally validated the tool using 150 patients from 3 different hospitals, with a C-statistic of 0.861. In the author’s home institution, case managers prepare discharge to an extended care facility if the PLAN predicts less than a 50% chance of a direct discharge home. They reported a decrease in LOS of 0.9 days when using the PLAN.
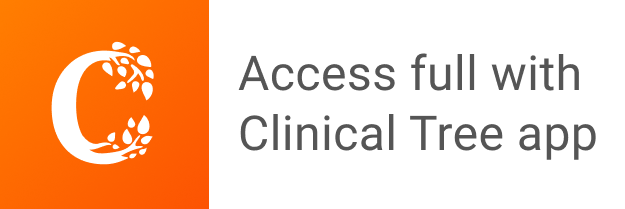