Key words
Statistics, Sensitivity, Specificity, Reliability, Validity
Introduction
In 1880, John Venn, a priest and lecturer in Moral Science at Caius College, Cambridge University, England, introduced and popularized Venn diagrams ( Fig. 1.1 ). Each circle represents a distinct domain that interacts with and is overlapped by other domains. The areas of overlap are more significant than the circles themselves, for within the overlapping areas “truth” can be found. While these diagrams were originally designed as models for mathematics and logic, they can also be used in the philosophy and practice of modern clinical medicine.

The “truth” in medicine represents the underlying diagnosis giving rise to a patient’s symptoms and signs. In this version of the Venn diagram, the large circle represents a patient’s relevant clinical history. It is the largest of the circles and where the majority of useful information can be found. Partially overlapping the patient’s history is a smaller circle representing the physical examination. The exam substantiates findings from the clinical history, but the nonoverlapping area represents its ability to identify issues not uncovered in the patient’s history. Last, the smallest circle represents additional clinical testing—whether laboratory, imaging, or electrophysiologic—that can further refine and confirm the true diagnosis denoted by the black dot. The true diagnosis lies within the clinical history, is supported by the physical examination, and is corroborated with other clinical studies.
In modern medicine, the bulk of clinical practice is predicated on the research question and the quality of support by which the question is answered. When critically reviewing the literature, it is paramount to understand these concepts. Indeed, an appreciation of the scientific method is necessary to fully understand the merits and pitfalls of the medical literature such that a conclusion can be properly applied to a specific clinical scenario. This chapter discusses the concepts of validity and reliability, how they give rise to sensitivity and specificity for diagnostic tests, and how the reported statistics of the various diagnostic tests should be interpreted in the clinical setting.
In contrast to observational cohort, case-control, and cross-sectional studies, the evaluation of diagnostic tests is different. Most observational studies attempt to show an association between the test result (a predictor variable) and the disease. In contrast, diagnostic studies attempt to discriminate between the diseased and the nondiseased. It is insufficient to merely identify an association between the test result and the disease. The concepts of specificity and sensitivity as well as positive and negative predictive value are discussed here.
Validity
Validity represents the truth, whether it is deduced, inferred, or supported by data. There are two types of validity: internal and external. Internal validity is the degree to which the results and conclusions from a research study correctly represent the data that were measured in the study. That is, the truth in the study can be correctly explained by the results of the study. However, it is important to recognize that this may not correctly answer the clinical question at hand. While a conclusion can be properly reached based on the available study findings, if the question asked or methods used are incorrect, then meaningful interpretation of the results is suspect. Once internal validity issues are satisfied, then the greater issue is that of external validity.
External validity is the degree to which the internal validity conclusions can be generalized to situations outside of the study. This is the sine qua non of meaningful clinical research. That is, can the conclusion of a study that has correctly interpreted its results be used outside of that specific research setting? The variables designed in a study must correctly represent the phenomena of interest. A research study that is so contrived or so artificially oversimplified to a degree that does not exist in the real world clinical setting is of guarded value.
Errors in study design and measurement tools greatly affect validity. How well a measurement represents a phenomenon is influenced by two major sources of error: sampling error and measurement error. In order for a study to be generalizable, the study population needs to parallel the target population. That is, the inclusion criteria for entrance into the study must represent the clinical characteristics and demographics of the population for which the study is intended. The sample size needs to be sufficiently large to avoid bias and increase power (see the following section). It is important to recognize that reporting errors can also occur, though these should be, and often are, identified in the peer review process.
Likewise, measurement errors need to be avoided so that valid conclusions can be drawn from the results. This brings up the concept of the accuracy and precision of a measurement ( Fig. 1.2 ). Accuracy is the degree to which the study measurement reflects the actual measurement. In other words, accuracy represents the validity of the study, whether internal or external. Greater accuracy increases the validity of the study.

Accuracy is influenced by systematic errors or bias. Limiting consistent distortion from the observer, subject, or instrument reduces accuracy. Observer distortion is a systematic error on the part of the observer in data gathering or reporting data. Subject bias refers to the consistent distortion of the facts as recalled or perceived by the subject. Instrument bias results from an error in the measurement device, either by malfunctioning or inappropriate usage for a study purpose for which it was not designed. Comparing the measurement to a reference standard best assesses accuracy.
Reliability
Reliability, and the related concept of precision, represents the reproducibility of a test. A test is considered reliable if repeated measurements consistently produce similar results. These results do not need to be compared with a reference standard. Precision refers to the uniformity and consistency of the repeated measurement. It is affected by random error whereby the greater the error, the lower the precision. Standard deviations are typically used to describe precision.
The three primary sources of precision error are observer, subject, and instrument variability. Observer variability is dependent on the observer in gathering data points, whereas subject variability refers to innate differences within the subject population that can contribute to errors. Instrument variability is affected by environmental factors.
Research studies on diagnostic tests are inherently susceptible to random errors. Patients with positive findings may not have the disease by chance alone and vice versa. Because random errors are difficult to control, confidence intervals for sensitivity and specificity should be reported. Confidence intervals allow for the possibility of random errors given the study’s sample size. The ranges of these confidence intervals are perhaps even more important than the actual sensitivity and specificity score.
The degree of concordance between paired measurements is usually expressed as a correlation coefficient (R) or as a kappa statistic (κ). The correlation coefficient is a number between −1 and +1. The absolute value indicates the strength of correlation, where 0 is poor and 1 is high, that is, very precise. Various tests can be used, including the Pearson coefficient, where values are evaluated directly, and the Spearman rank test, where values are placed in rank order and then analyzed.
Reliability measurements need to be observed for test–retest, internal, and interobserver and intraobserver consistency. The test–retest reliability refers to the concordance among repeated measurements on a sample of subjects. Caution must be exercised especially with physical exam maneuvers because the test itself can create errors by factors such as the training effect and learning curve. Internal consistency indicates that separate measures of the same variable will have internal concordance. Intraobserver consistency indicates that repeated measurements by a single observer are reproducible whereas interobserver measurements are reproducible by separate observers of the same event.
Interobserver agreement is often reported as a kappa statistic, which provides a quantitative measure of the magnitude of agreement between observers. For example, the modified scapular assistance test (SAT), as described by Rabin and colleagues, reveals moderate interrater reliability with a kappa coefficient and percent agreement of 0.53 and 77%, respectively, when performed in the scapular plane and 0.62 and 91%, respectively, when performed in the sagittal plane. Based on a higher degree of interobserver agreement, the authors concluded that the modified SAT is more reliable when performed in the sagittal plane.
Precision strongly influences the power of a study. A more precise measurement lends greater statistical power. Power is the probability of rejecting the null hypothesis when it is in fact false. The null hypothesis suggests there is no association between the two variables in question. The power depends on the total number of end-points experienced by a population. By increasing the sample size, the power will increase. This will also decrease the probability that the null hypothesis will be incorrectly accepted.
Validity and reliability are not necessarily linked nor are they mutually exclusive. Although high accuracy and precision are ideal within a given test, unfortunately, this is not often the case. It is possible to have high accuracy yet low precision, and vice versa ( Fig. 1.2 ).
Specificity and Sensitivity
As mentioned previously, the outcome variable of a diagnostic test is the presence or absence of disease or injury when compared with the ideal reference standard known as the “gold standard.” By convention, the gold standard is always positive in patients with the disease and negative in those without the disease. However, in the clinical setting, even the gold standard has its limitations and is not impervious to error. Generally, the quality and efficacy of a diagnostic test is obtained by calculating its sensitivity and specificity.
The outcome variable of a diagnostic test falls into one of four situations ( Table 1.1 ):
- 1.
A true-positive result, where the test is positive for the patient who has the disease
- 2.
A false-positive result, where the test is positive but the patient does not have the disease
- 3.
A false-negative result, where the test is negative but the patient has the disease
- 4.
A true-negative result, where the test is negative for the patient who does not have the disease.
Disease State | |||
---|---|---|---|
Present | Absent | ||
Test result | Positive | (a) True positive | (b) False positive |
Negative | (c) False negative | (d) True negative | |
(a) + (c) = 100% | (b) + (d) = 100% |
Ideally, the best diagnostics tests have no false positives or false negatives. Sensitivities and specificities are unlinked and should not affect one other. It is possible to have any combination of sensitivities and specificities—high sensitivity with high or low specificity, and vice versa. The utility of a test with both low sensitivity and specificity has dubious value.
The sensitivity of a test represents how good it is at identifying disease. Andersson and Deyo used the mnemonic SnNout. If S e n sitivity is high, a N egative test result rules out the target diagnosis. It is calculated by the proportion of patients with the disease who have a positive test:
Sensitivity = True positive / [ True positive + False negative ] = ( a ) / [ ( a ) + ( c ) ] .
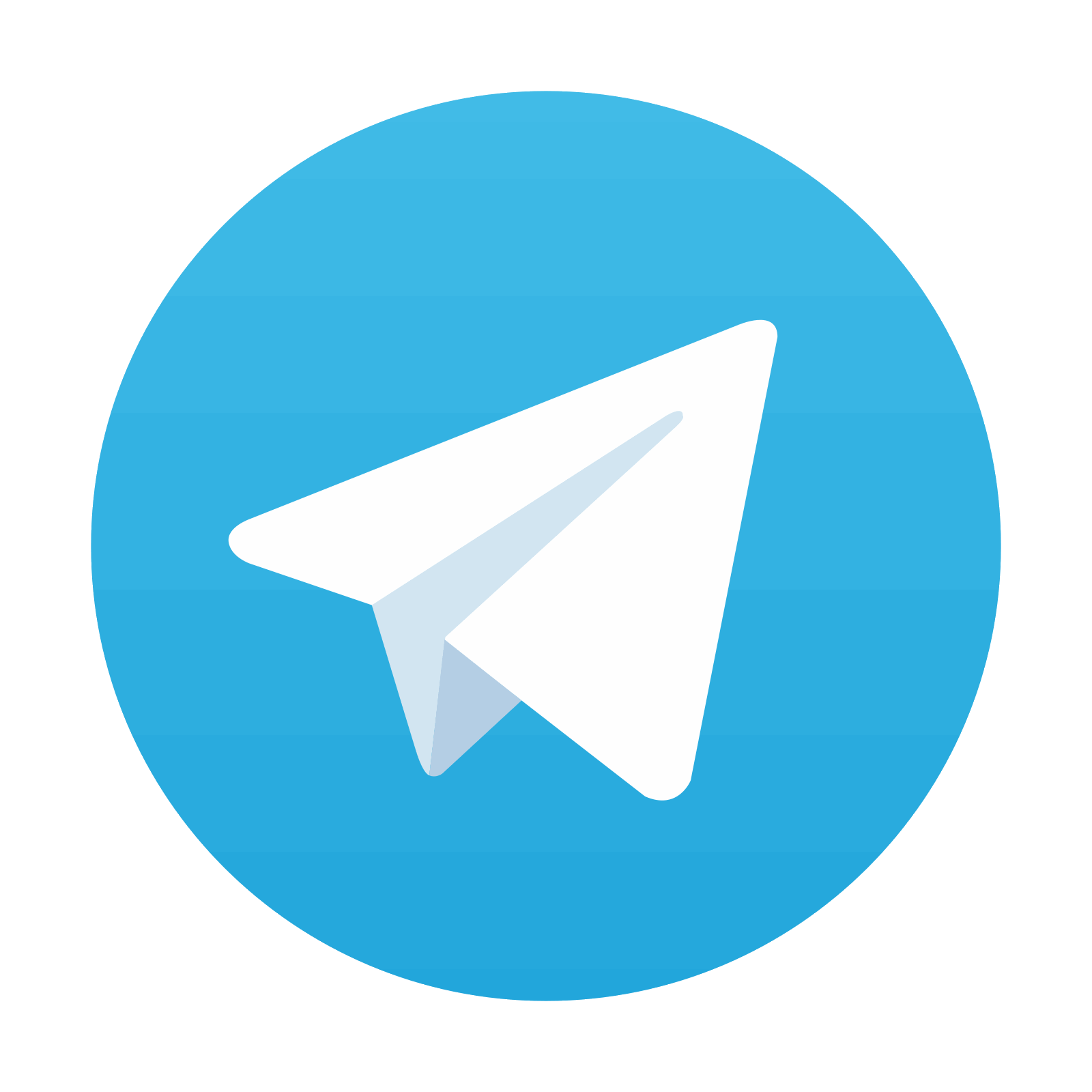
Stay updated, free articles. Join our Telegram channel

Full access? Get Clinical Tree
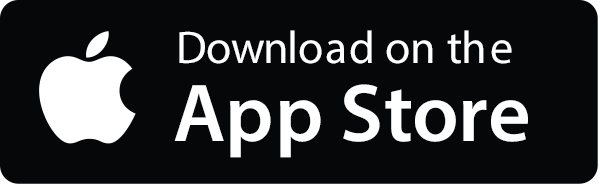
