Rheumatoid arthritis (RA) results from an interaction between genetic susceptibility and environmental factors. Several of these factors are known, such as family history of RA, high birth weight, smoking, silica exposure, alcohol nonuse, obesity, diabetes mellitus, rheumatoid factor, anti-citrullinated protein antibody, and genetic variants such as the shared epitope and protein tyrosine phosphatase nonreceptor type 22. The impact of these factors can be modeled in the 2 main groups at risk of RA: family members of patients with RA and seropositive persons with or without arthralgia. Current models have the potential to select individuals for preventive strategies.
Key points
- •
Risk factors for rheumatoid arthritis (RA) include family history, birth weight, smoking, silica, alcohol nonuse, obesity, diabetes mellitus, autoantibodies, and genetic variants.
- •
Symptoms, antibodies, and inflammatory biomarkers can be useful in late at-risk stages, and genetic scores plus environmental factors more useful in early at-risk stages.
- •
Prediction models of RA can help to select candidates for intervention studies.
- •
The best target populations for screening are relatives of patients with RA and (seropositive) patients with arthralgia. However, only a minority of persons at risk can thus be recognized.
- •
Screening for RA risk is still experimental, because there is no validated screening tool and no proven therapy to prevent disease.
Introduction
Rheumatoid arthritis (RA) on average becomes clinically manifest around the age of 55 years. During the healthy part of life, the risk of future RA is determined by genetic, reproductive and environmental factors ( Fig. 1 , green bar). Over time, people at risk for RA may pass through a phase of autoimmunity, accompanied by subclinical inflammation, followed by a symptomatic phase, which may last a few months to several years. In the symptomatic phase, markers of autoimmunity and inflammation increase before the onset of clinical arthritis. Therefore, prediction can be based on different characteristics in the asymptomatic phase and in the symptomatic phase.

The expectation that intervening in the preclinical phase of RA could be beneficial is based on the success of treatment of RA within 1 to 2 years after onset of clinical disease. The new criteria for RA from 2010 with a focus on early signs such as involvement of even only a few small joints together with serology and acute phase reactants facilitate treatment in the earliest clinical phase, and the further characterization of the preclinical phase offers new opportunities for intervention studies even before clinically apparent arthritis occurs. Because RA is the most prevalent inflammatory rheumatic disease, with a high burden for the patient and society, it seems the ideal candidate rheumatic disease for screening and intervention programs. However, a lot of steps need to be taken before such programs can be offered to persons at risk.
This article summarizes the present knowledge on risk factors for RA, including genetic, reproductive, and hormonal factors; environmental exposures; biomarkers; personal characteristics and symptoms; and how these can be combined in risk models attempting to increase the accuracy of the prediction of RA. Genetic risk and gene-environmental interactions are dealt with elsewhere in this issue and are only mentioned here in relation to their roles in prediction models. Risk scores from such models require further validation, but could be used to select candidates for intervention studies.
Introduction
Rheumatoid arthritis (RA) on average becomes clinically manifest around the age of 55 years. During the healthy part of life, the risk of future RA is determined by genetic, reproductive and environmental factors ( Fig. 1 , green bar). Over time, people at risk for RA may pass through a phase of autoimmunity, accompanied by subclinical inflammation, followed by a symptomatic phase, which may last a few months to several years. In the symptomatic phase, markers of autoimmunity and inflammation increase before the onset of clinical arthritis. Therefore, prediction can be based on different characteristics in the asymptomatic phase and in the symptomatic phase.

The expectation that intervening in the preclinical phase of RA could be beneficial is based on the success of treatment of RA within 1 to 2 years after onset of clinical disease. The new criteria for RA from 2010 with a focus on early signs such as involvement of even only a few small joints together with serology and acute phase reactants facilitate treatment in the earliest clinical phase, and the further characterization of the preclinical phase offers new opportunities for intervention studies even before clinically apparent arthritis occurs. Because RA is the most prevalent inflammatory rheumatic disease, with a high burden for the patient and society, it seems the ideal candidate rheumatic disease for screening and intervention programs. However, a lot of steps need to be taken before such programs can be offered to persons at risk.
This article summarizes the present knowledge on risk factors for RA, including genetic, reproductive, and hormonal factors; environmental exposures; biomarkers; personal characteristics and symptoms; and how these can be combined in risk models attempting to increase the accuracy of the prediction of RA. Genetic risk and gene-environmental interactions are dealt with elsewhere in this issue and are only mentioned here in relation to their roles in prediction models. Risk scores from such models require further validation, but could be used to select candidates for intervention studies.
Methods
We searched the PubMed database on January 29, 2014, for the terms risk, prediction, and development in relation to RA. After excluding articles not directly related to prediction of RA, such as studies on prevalence, diagnosis, treatment, outcome, or comorbidities of RA, more than 200 articles remained on this topic after screening 2000 abstracts. Additional articles were added that were found after the search date until May 1, 2014, by screening rheumatologic journals.
Risk factors: the building blocks of prediction
The current evidence on risk factors for RA is summarized in Table 1 . Besides the factors reported in the table, many others have been investigated for their association with the risk of RA, but these studies have led to negative, inconclusive, or conflicting results. Among these are variables such as silicone implants ; consumption of coffee, tea, or red meat ; geographic area ; and socioeconomic status. In contrast, some of the factors that have statistically significant associations with RA show opposite directions of risk in different studies. Examples of such cases are age at menarche, breastfeeding, and parity. This uncertainty makes the value of such variables questionable, even if they have been included in prediction models, as is the case with parity and breastfeeding in the model by Lahiri and colleagues.
Risk Factor | Comments |
---|---|
Family history | Risk increases with number of affected family members The longer the disease duration and the higher the age of the proband, the higher the risk Some studies did not find an association between relatives with RA and risk of RA |
Genetic factors | Around 60 risk loci for RA are known, explaining 16% of total susceptibility 65% of RA risk is thought to be heritable |
Reproductive and hormonal factors | Risk is 2–4 times higher in women A protective effect of oral contraceptives is suggested High birth weight (more than 4 kg) increases risk Lower risk during pregnancy, compensated by an increased risk in the first postpartum year Complications during pregnancy may be related to a higher risk Inconclusive or conflicting results for breastfeeding, age at menarche, irregular menstrual cycles and age at menopause, postmenopausal hormone use, lower testosterone levels, parity, age at first childbirth, low birth weight, and being small for gestational age |
Environmental factors | Smoking is the most established risk factor Smoking interacts with the strongest genetic risk factor ( HLA-SE ) in a dose-dependent manner to increase the risk of seropositive RA Alcohol consumption (even in small quantities) protects High consumption of olive oil and fish (oil) protects Inconclusive results were found for vitamin D intake and ultraviolet B exposure, antioxidant and trace element intake, and exposure to toxic elements |
Occupations and occupational exposures | Farmers, blue collar workers, and hairdressers are at increased risk Silica exposure gives increased risk Exposures that could not be related to RA: asbestos, mineral oil, organic dust, herbicides, insecticides, carbamates, organophosphates, carbaryl, glyphosate, malathion, and ambient air pollution |
Infections and vaccinations | Frequent infections may predispose One study reported increased risk after influenza vaccination Risks could not be quantified for: Ebstein-Barr virus infection, hepatitis C, HIV, Yersinia enterocolitica , mycoplasma, or Porphyromonas gingivalis infection of the gums, and for immunization (other than influenza) |
Comorbidities | Diabetes types 1 and 2 and inflammatory lung disorders increase risk Schizophrenia is protective Obesity and the related condition obstructive sleep apnea syndrome increase the risk Dyslipidemia is present before RA and predicts RA Other associations, such as for thyroid disease, are inconclusive |
Autoantibodies | Status and levels of (isotypes of) RF and ACPA associate with RA risk Higher levels and the combination of RF and ACPA confer a higher risk Additional predictive ability independent of RF and ACPA was shown for anti–carbamylated protein antibodies and anti–peptidyl arginine deiminase type 4 antibodies |
Other biomarkers in blood | Several acute phase reactants and cytokines are increased in pre-RA or at-risk cohorts TNF (receptor), cartilage oligomeric matrix protein, and a high interferon gene score are quantified risk factors |
Imaging | Ultrasonography abnormalities (mainly power Doppler signal) in seropositive patients with arthralgia were predictive of arthritis at the joint level in 1 study and at the patient level in another study Technetium bone scintigraphy is predictive of RA in patients with arthralgia and can exclude inflammatory joint disease Macrophage-targeted positron emission tomography predicts arthritis in ACPA-positive patients with arthralgia The predictive capacity of MRI in arthralgia is not yet clear |
Symptoms | Predictive symptoms in combination with the presence of autoantibodies: duration <12 mo, intermittent symptoms, arthralgia in upper and lower extremities, morning stiffness ≥1 h, self-reported joint swelling, tenderness of hand or foot joints, and morning stiffness ≥30 min |
In conclusion, there are not many risk factors with strong and confirmed associations with RA. Among these are family history of RA, high birth weight, smoking, silica exposure, alcohol nonuse, obesity, diabetes mellitus, rheumatoid factor (RF), anti–citrullinated protein antibody (ACPA), and genetic variants such as the shared epitope (SE) and protein tyrosine phosphatase nonreceptor type 22 (PTPN22).
Prediction rules: putting the blocks together
In a manner similar to the way clinical characteristics, signs, and symptoms can be combined to diagnose a disease in a patient, the potential risk factors for a given disease can be combined by statistical modeling of variables measured in an at-risk population in order to produce prediction rules. The advantage of such models is that they clarify the relative impact of the individual variables and quantify the overall risk for individuals coming from that population. The validity of these models can then be further confirmed by testing them in other populations.
Recently, several prediction models have been published that attempt to quantify progression to RA ( Table 2 ). Two of these models were based on large population studies, of which 1 was designed for investigating other diseases as well. One of these used clinical characteristics to predict either seropositive or seronegative RA, the other used the combination of clinical characteristics, autoantibodies, and a genetic risk score containing multiple genes (see Table 2 for the variables in the models). Both studies achieve good prediction. However, it is uncertain whether these values can be reproduced in smaller populations.
First Author and Year (Ref.) | Cohort; Variables | Numbers | Results |
---|---|---|---|
Van de Stadt et al, 2013 | Seropositive patients with arthralgia Prediction rule variables: alcohol consumption, family history, symptoms <12 mo, intermittent, in upper and lower extremities, VAS ≥ 50, morning stiffness ≥1 h, swollen joints reported by patient, autoantibody status | Arthralgia: 374 (131 developed arthritis) | Prediction rule: AUC 0.82 (CI 0.75–0.89) Intermediate-risk vs low-risk group: HR 4.52 (CI 2.42–8.77) High-risk vs low-risk group: HR 14.86 (CI 8.40–28) |
de Hair et al, 2013 | Seropositive patients with arthralgia Predictive variables: smoking and BMI | Arthralgia: 55 (15 developed arthritis) | Smoking (ever vs never) and risk of RA: HR 9.6 (CI 1.3–73) Obesity (BMI ≥ 25 vs <25) and risk of RA: HR 5.6 (CI 1.3–25) |
Lahiri et al, 2014 | European Prospective Investigation of Cancer, Norfolk, United Kingdom 40–79 y Prediction rule variables: alcohol consumption, smoking, occupation, BMI, diabetes mellitus, parity | Total participants: 25,455 (184 developed IP, 138 developed RA) | Pack-years smoking in men and risk of IP: HR 1.21 (CI 1.08–1.37) Seropositive in men and risk of IP: HR 1.24 (CI 1.10–1.41) Having DM (I or II) and risk of IP: HR 2.54 (CI 1.26–5.09) Alcohol and risk of IP (per unit/d): HR 0.36 (CI 0.15–0.89) Overweight vs normal-weight and risk of seronegative IP: HR 2.75 (CI 1.39–5.46) Parity ≥2 vs no children and risk of IP: HR 2.81 (CI 1.37–5.76) Breastfeeding for every 52 wk and risk of IP: HR 0.66 (CI 0.46–0.94) |
Sparks et al, 2014 | NHS, United States, women 30–55 y EIRA, Sweden, 18–70 y Prediction rule variables: family history, alcohol consumption, smoking, BMI, parity, autoantibody status, genetic risk score | RA cases: 1625 Controls: 1381 | NHS seropositive RA (model family history, epidemiologic, genetic): AUC 0.74 (CI 0.70–0.78) NHS seropositive RA and positive family history: AUC 0.82 (CI 0.74–0.90) EIRA ACPA-positive RA (model family history, epidemiologic, genetic): AUC 0.77 (CI 0.75–0.80) EIRA ACPA-positive RA and positive family history: AUC 0.83 (CI 0.76–0.91) EIRA ACPA-positive RA and positive family history, high genetic susceptibility, smoking, and increased BMI: OR 21.73 (CI 10–44) |
Rakieh et al, 2014 | Yorkshire, United Kingdom ACPA-positive patients with arthralgia Prediction rule variables: joint tenderness, morning stiffness ≥30 min, high positive autoantibodies, positive ultrasonographic power Doppler signal | Arthralgia: 100 (50 developed RA) | Power Doppler model: Harrell C 0.67 (CI 0.59–0.74) Progression to IA: Low risk (0 points) 0% Moderate risk (1–2 points) 31% High risk (≥3 points) 62% |
Three other studies investigated the development of RA in ACPA-positive and/or RF-positive patients with arthralgia. The patients were partly recruited in primary care, and partly in the rheumatology clinic. The models were based on clinical characteristics, symptoms, and antibody characteristics, in 1 study supplemented by ultrasonographic power Doppler signal (see Table 2 ). All 3 models provide good discrimination between persons who do or do not develop RA. However, they require ongoing validation as other studies select and follow such cohorts of people at risk for RA. Similar studies from North America designed to predict RA in first-degree relatives of patients with RA are underway but have not yet gathered enough arthritis cases to enable the construction of prediction models. These studies are hampered by the low frequency of autoantibodies or of increased biomarkers in relatives of patients with RA.
Measuring the risk of RA is also a matter of timing. During the early at-risk stage, before the onset of autoimmunity, clinicians can only measure genetic susceptibility and environmental factors (see the left part of Fig. 1 ). The predictive capability of models in this situation is becoming good, with areas under the curve of 72% to 77% for the prediction of ACPA-positive RA. However, the measured risk is a lifetime risk, which makes it an abstract figure for the individual person at risk. Prediction including a time frame becomes possible nearer to the onset of clinical RA, when the aspects of symptoms, autoimmunity, and inflammation can be taken into account. In the Amsterdam risk model, points can be gathered for clinical characteristics, symptoms, and serology, with more points for high levels of ACPA or positivity for both ACPA and RF. The more points, the higher the risk and the sooner the onset of arthritis can be expected ( Fig. 2 ). This prediction reflects studies in pre-RA blood donors, in which autoantibody levels increase during the 1 to 3 years before the onset of clinical arthritis. In an US cohort of 81 patients with clinical RA from whom stored serum was available from 1 to 12 years before disease onset, a biomarker profile including autoantibodies and cytokines was identified that predicts the imminent onset of clinical arthritis within 2 years. Autoantibody epitope spreading by itself in the preclinical phase also predicts progression to classifiable RA.
Screening strategies
Many medical, ethical, and economic issues need to be addressed before screening for risk of future RA can be offered to certain categories of unaffected persons. Basic requirements for screening groups of people to predict a disease are (1) a defined population to test; (2) the existence of an asymptomatic (or nonspecific symptomatic) phase; (3) the availability of a test with good accuracy, low rates of side effects, and low cost; and (4) the availability of a cost-effective intervention in the at-risk phase. Only the second requirement of an asymptomatic phase is clearly fulfilled at present. Regarding items 3 and 4, no single test can identify those at risk for RA and no intervention exists with proven efficacy in the at-risk situation. All efforts to predict RA and treat persons with an increased risk for RA are therefore currently regarded as investigational. The test for RA will eventually be a validated, cost-effective, and accurate prediction rule that is easy to apply. For comparison, consider the screening programs for colonic cancer, which have recently been established in several countries. All persons more than a certain age are offered screening, which leads to huge numbers of colonoscopies. The high cost of this procedure and the possibility of serious side effects need to be weighed against the benefit of removing polyps that would cause a high morbidity and mortality if left unnoticed.
Regarding item 1, careful consideration is needed to decide which population(s) should be screened or tested. The choices from general to specific are general population, relatives of patients with RA, persons with musculoskeletal symptoms, or persons with RA-specific autoimmunity. Because RA is not highly prevalent in most populations, with the possible exception of North American native peoples, at this time it is not practical to test the general population for RA. Two recognizable target groups then remain: relatives of patients with RA and persons with musculoskeletal symptoms. The latter are found both in general practice and in rheumatology clinics. After history taking and physical examination, it must be decided which patients should proceed to further testing for RA risk, and which test to use. At present most clinicians use the RF and/or ACPA test, which are widely available and easy to perform. Except for patients with only RF positivity just above the reference range, the results give useful information. The question of who to test in general practice cannot accurately be answered at this time. This question requires structured longitudinal follow-up of patients in general practice, or the following of cohorts with clinically suspect arthralgia in rheumatology clinics.
Summary
There is a trend toward increasingly sophisticated prediction models for RA in different stages of risk. However, further work is needed to combine patient-level information with the published promising biomarkers into more robust models. For example, models for relatives of patients with RA, reflecting the early at-risk stage, depend largely on personal characteristics and genetic risk, whereas models for patients with arthralgia that reflect the late at-risk stage need to include patient-related and symptom characteristics in combination with biomarkers of autoimmunity and inflammation. In view of the vague and unspecific first symptoms of many patients who later develop RA, it will be necessary to better characterize and measure these symptoms in future models.
However, because much is known about the risks for developing RA, it is already possible to use this information to design preventive interventions in persons at high risk for RA. At least in the late preclinical stage, several such interventions are currently being tested or planned.
Disclosures: None.
1 S.A. Turk and M.H. van Beers-Tas contributed equally to this work.
References
- 1. Nielen M.M., van S.D., Reesink H.W., et al: Simultaneous development of acute phase response and autoantibodies in preclinical rheumatoid arthritis. Ann Rheum Dis 2006; 65: pp. 535-537
- 2. van de Stadt L.A., de Koning M.H., van de Stadt R.J., et al: Development of the anti-citrullinated protein antibody repertoire prior to the onset of rheumatoid arthritis. Arthritis Rheum 2011; 63: pp. 3226-3233
- 3. Boers M., Verhoeven A.C., Markusse H.M., et al: Randomised comparison of combined step-down prednisolone, methotrexate and sulphasalazine with sulphasalazine alone in early rheumatoid arthritis. Lancet 1997; 350: pp. 309-318
- 4. Goekoop-Ruiterman Y.P., de Vries-Bouwstra J.K., Allaart C.F., et al: Clinical and radiographic outcomes of four different treatment strategies in patients with early rheumatoid arthritis (the BeSt study): a randomized, controlled trial. Arthritis Rheum 2008; 58: pp. S126-S135
- 5. Aletaha D., Neogi T., Silman A.J., et al: 2010 Rheumatoid arthritis classification criteria: an American College of Rheumatology/European League Against Rheumatism collaborative initiative. Ann Rheum Dis 2010; 69: pp. 1580-1588
- 6. Britsemmer K., Ursum J., Gerritsen M., et al: Validation of the 2010 ACR/EULAR classification criteria for rheumatoid arthritis: slight improvement over the 1987 ACR criteria. Ann Rheum Dis 2011; 70: pp. 1468-1470
- 7. Janowsky E.C., Kupper L.L., and Hulka B.S.: Meta-analyses of the relation between silicone breast implants and the risk of connective-tissue diseases. N Engl J Med 2000; 342: pp. 781-790
- 8. Perkins L.L., Clark B.D., Klein P.J., et al: A meta-analysis of breast implants and connective tissue disease. Ann Plast Surg 1995; 35: pp. 561-570
- 9. Wong O.: A critical assessment of the relationship between silicone breast implants and connective tissue diseases. Regul Toxicol Pharmacol 1996; 23: pp. 74-85
- 10. Heliovaara M., Aho K., Knekt P., et al: Coffee consumption, rheumatoid factor, and the risk of rheumatoid arthritis. Ann Rheum Dis 2000; 59: pp. 631-635
- 11. Karlson E.W., Mandl L.A., Aweh G.N., et al: Coffee consumption and risk of rheumatoid arthritis. Arthritis Rheum 2003; 48: pp. 3055-3060
- 12. Mikuls T.R., Cerhan J.R., Criswell L.A., et al: Coffee, tea, and caffeine consumption and risk of rheumatoid arthritis: results from the Iowa Women’s Health Study. Arthritis Rheum 2002; 46: pp. 83-91
- 13. Pedersen M., Jacobsen S., Klarlund M., et al: Environmental risk factors differ between rheumatoid arthritis with and without auto-antibodies against cyclic citrullinated peptides. Arthritis Res Ther 2006; 8: pp. R133
- 14. Benito-Garcia E., Feskanich D., Hu F.B., et al: Protein, iron, and meat consumption and risk for rheumatoid arthritis: a prospective cohort study. Arthritis Res Ther 2007; 9: pp. R16
- 15. Grant W.B.: The role of meat in the expression of rheumatoid arthritis. Br J Nutr 2000; 84: pp. 589-595
- 16. Pattison D.J., Symmons D.P., Lunt M., et al: Dietary risk factors for the development of inflammatory polyarthritis: evidence for a role of high level of red meat consumption. Arthritis Rheum 2004; 50: pp. 3804-3812
- 17. Alamanos Y., Voulgari P.V., and Drosos A.A.: Incidence and prevalence of rheumatoid arthritis, based on the 1987 American College of Rheumatology criteria: a systematic review. Semin Arthritis Rheum 2006; 36: pp. 182-188
- 18. Costenbader K.H., Chang S.C., Laden F., et al: Geographic variation in rheumatoid arthritis incidence among women in the United States. Arch Intern Med 2008; 168: pp. 1664-1670
- 19. Kallberg H., Vieira V., Holmqvist M., et al: Regional differences regarding risk of developing rheumatoid arthritis in Stockholm County, Sweden: results from the Swedish Epidemiological Investigation of Rheumatoid Arthritis (EIRA) study. Scand J Rheumatol 2013; 42: pp. 337-343
- 20. Li X., Sundquist J., and Sundquist K.: Risks of rheumatic diseases in first- and second-generation immigrants in Sweden: a nationwide followup study. Arthritis Rheum 2009; 60: pp. 1588-1596
- 21. Silman A., Bankhead C., Rowlingson B., et al: Do new cases of rheumatoid arthritis cluster in time or in space? Int J Epidemiol 1997; 26: pp. 628-634
- 22. Silman A., Harrison B., Barrett E., et al: The existence of geographical clusters of cases of inflammatory polyarthritis in a primary care based register. Ann Rheum Dis 2000; 59: pp. 152-154
- 23. Bankhead C., Silman A., Barrett B., et al: Incidence of rheumatoid arthritis is not related to indicators of socioeconomic deprivation. J Rheumatol 1996; 23: pp. 2039-2042
- 24. Bengtsson C., Nordmark B., Klareskog L., et al: Socioeconomic status and the risk of developing rheumatoid arthritis: results from the Swedish EIRA study. Ann Rheum Dis 2005; 64: pp. 1588-1594
- 25. Jacobsson L.T., Jacobsson M.E., Askling J., et al: Perinatal characteristics and risk of rheumatoid arthritis. BMJ 2003; 326: pp. 1068-1069
- 26. Olsson A.R., Skogh T., and Wingren G.: Aetiological factors of importance for the development of rheumatoid arthritis. Scand J Rheumatol 2004; 33: pp. 300-306
- 27. Pedersen M., Jacobsen S., Klarlund M., et al: Socioeconomic status and risk of rheumatoid arthritis: a Danish case-control study. J Rheumatol 2006; 33: pp. 1069-1074
- 28. Uhlig T., Hagen K.B., and Kvien T.K.: Current tobacco smoking, formal education, and the risk of rheumatoid arthritis. J Rheumatol 1999; 26: pp. 47-54
- 29. Lahiri M., Luben R.N., Morgan C., et al: Using lifestyle factors to identify individuals at higher risk of inflammatory polyarthritis (results from the European Prospective Investigation of Cancer-Norfolk and the Norfolk Arthritis Register–the EPIC-2-NOAR Study). Ann Rheum Dis 2014; 73: pp. 219-226
- 30. Koumantaki Y., Giziaki E., Linos A., et al: Family history as a risk factor for rheumatoid arthritis: a case-control study. J Rheumatol 1997; 24: pp. 1522-1526
- 31. Svendsen A.J., Holm N.V., Kyvik K., et al: Relative importance of genetic effects in rheumatoid arthritis: historical cohort study of Danish nationwide twin population. BMJ 2002; 324: pp. 264-266
- 32. Deighton C.M., Roberts D.F., and Walker D.J.: Effect of disease severity on rheumatoid arthritis concordance in same sexed siblings. Ann Rheum Dis 1992; 51: pp. 943-945
- 33. Hemminki K., Li X., Sundquist J., et al: Familial associations of rheumatoid arthritis with autoimmune diseases and related conditions. Arthritis Rheum 2009; 60: pp. 661-668
- 34. Larkin J.G.: Family history of rheumatoid arthritis–a non-predictor of inflammatory disease? Rheumatology (Oxford) 2010; 49: pp. 608-609
- 35. Jones M.A., Silman A.J., Whiting S., et al: Occurrence of rheumatoid arthritis is not increased in the first degree relatives of a population based inception cohort of inflammatory polyarthritis. Ann Rheum Dis 1996; 55: pp. 89-93
- 36. Viatte S., Plant D., and Raychaudhuri S.: Genetics and epigenetics of rheumatoid arthritis. Nat Rev Rheumatol 2013; 9: pp. 141-153
- 37. Karlson E.W., Chibnik L.B., McGrath M., et al: A prospective study of androgen levels, hormone-related genes and risk of rheumatoid arthritis. Arthritis Res Ther 2009; 11: pp. R97
- 38. Colebatch A.N., and Edwards C.J.: The influence of early life factors on the risk of developing rheumatoid arthritis. Clin Exp Immunol 2011; 163: pp. 11-16
- 39. Mandl L.A., Costenbader K.H., Simard J.F., et al: Is birthweight associated with risk of rheumatoid arthritis? Data from a large cohort study. Ann Rheum Dis 2009; 68: pp. 514-518
- 40. Peschken C.A., Robinson D.B., Hitchon C.A., et al: Pregnancy and the risk of rheumatoid arthritis in a highly predisposed North American Native population. J Rheumatol 2012; 39: pp. 2253-2260
- 41. Spector T.D., Roman E., and Silman A.J.: The pill, parity, and rheumatoid arthritis. Arthritis Rheum 1990; 33: pp. 782-789
- 42. Jorgensen K.T., Pedersen B.V., Jacobsen S., et al: National cohort study of reproductive risk factors for rheumatoid arthritis in Denmark: a role for hyperemesis, gestational hypertension and pre-eclampsia? Ann Rheum Dis 2010; 69: pp. 358-363
- 43. Karlson E.W., Mandl L.A., Hankinson S.E., et al: Do breast-feeding and other reproductive factors influence future risk of rheumatoid arthritis? Results from the Nurses’ Health Study. Arthritis Rheum 2004; 50: pp. 3458-3467
- 44. Pikwer M., Bergstrom U., Nilsson J.A., et al: Early menopause is an independent predictor of rheumatoid arthritis. Ann Rheum Dis 2012; 71: pp. 378-381
- 45. Berglin E., Kokkonen H., Einarsdottir E., et al: Influence of female hormonal factors, in relation to autoantibodies and genetic markers, on the development of rheumatoid arthritis in northern Sweden: a case-control study. Scand J Rheumatol 2010; 39: pp. 454-460
- 46. Pikwer M., Bergstrom U., Nilsson J.A., et al: Breast feeding, but not use of oral contraceptives, is associated with a reduced risk of rheumatoid arthritis. Ann Rheum Dis 2009; 68: pp. 526-530
- 47. Brennan P., and Silman A.: Breast-feeding and the onset of rheumatoid arthritis. Arthritis Rheum 1994; 37: pp. 808-813
- 48. Carette S., Marcoux S., and Gingras S.: Postmenopausal hormones and the incidence of rheumatoid arthritis. J Rheumatol 1989; 16: pp. 911-913
- 49. Deighton C.M., Sykes H., and Walker D.J.: Rheumatoid arthritis, HLA identity, and age at menarche. Ann Rheum Dis 1993; 52: pp. 322-326
- 50. Hernandez A.M., Liang M.H., Willett W.C., et al: Reproductive factors, smoking, and the risk for rheumatoid arthritis. Epidemiology 1990; 1: pp. 285-291
- 51. Heikkila R., Aho K., Heliovaara M., et al: Serum androgen-anabolic hormones and the risk of rheumatoid arthritis. Ann Rheum Dis 1998; 57: pp. 281-285
- 52. Pikwer M., Giwercman A., Bergstrom U., et al: Association between testosterone levels and risk of future rheumatoid arthritis in men: a population-based case-control study. Ann Rheum Dis 2014; 73: pp. 573-579
- 53. Masi A.T., Aldag J.C., and Chatterton R.T.: Sex hormones and risks of rheumatoid arthritis and developmental or environmental influences. Ann N Y Acad Sci 2006; 1069: pp. 223-235
- 54. Carlens C., Jacobsson L., Brandt L., et al: Perinatal characteristics, early life infections and later risk of rheumatoid arthritis and juvenile idiopathic arthritis. Ann Rheum Dis 2009; 68: pp. 1159-1164
- 55. Rogers M.A., Levine D.A., Blumberg N., et al: Antigenic challenge in the etiology of autoimmune disease in women. J Autoimmun 2012; 38: pp. J97-J102
- 56. Ma K.K., Nelson J.L., Guthrie K.A., et al: Adverse pregnancy outcomes and risk of subsequent rheumatoid arthritis. Arthritis Rheumatol 2014; 66: pp. 508-512
- 57. Simard J.F., Costenbader K.H., Hernan M.A., et al: Early life factors and adult-onset rheumatoid arthritis. J Rheumatol 2010; 37: pp. 32-37
- 58. Carlens C., Hergens M.P., Grunewald J., et al: Smoking, use of moist snuff, and risk of chronic inflammatory diseases. Am J Respir Crit Care Med 2010; 181: pp. 1217-1222
- 59. Di G.D., Discacciati A., Orsini N., et al: Cigarette smoking and risk of rheumatoid arthritis: a dose-response meta-analysis. Arthritis Res Ther 2014; 16: pp. R61
- 60. Kallberg H., Ding B., Padyukov L., et al: Smoking is a major preventable risk factor for rheumatoid arthritis: estimations of risks after various exposures to cigarette smoke. Ann Rheum Dis 2011; 70: pp. 508-511
- 61. Sugiyama D., Nishimura K., Tamaki K., et al: Impact of smoking as a risk factor for developing rheumatoid arthritis: a meta-analysis of observational studies. Ann Rheum Dis 2010; 69: pp. 70-81
- 62. Klareskog L., Stolt P., Lundberg K., et al: A new model for an etiology of rheumatoid arthritis: smoking may trigger HLA-DR (shared epitope)-restricted immune reactions to autoantigens modified by citrullination. Arthritis Rheum 2006; 54: pp. 38-46
- 63. Jin Z., Xiang C., Cai Q., et al: Alcohol consumption as a preventive factor for developing rheumatoid arthritis: a dose-response meta-analysis of prospective studies. Ann Rheum Dis 2013; undefined:
- 64. Scott I.C., Tan R., Stahl D., et al: The protective effect of alcohol on developing rheumatoid arthritis: a systematic review and meta-analysis. Rheumatology (Oxford) 2013; 52: pp. 856-867
- 65. van de Stadt L.A., and van S.D.: Alcohol consumption protects against arthritis development in seropositive arthralgia patients. Ann Rheum Dis 2012; 71: pp. 1431-1432
- 66. Di G.D., Wallin A., Bottai M., et al: Long-term intake of dietary long-chain n-3 polyunsaturated fatty acids and risk of rheumatoid arthritis: a prospective cohort study of women. Ann Rheum Dis 2013; undefined:
- 67. Linos A., Kaklamanis E., Kontomerkos A., et al: The effect of olive oil and fish consumption on rheumatoid arthritis–a case control study. Scand J Rheumatol 1991; 20: pp. 419-426
- 68. Linos A., Kaklamani V.G., Kaklamani E., et al: Dietary factors in relation to rheumatoid arthritis: a role for olive oil and cooked vegetables? Am J Clin Nutr 1999; 70: pp. 1077-1082
- 69. Oliver J.E., and Silman A.J.: Risk factors for the development of rheumatoid arthritis. Scand J Rheumatol 2006; 35: pp. 169-174
- 70. Pattison D.J., Harrison R.A., and Symmons D.P.: The role of diet in susceptibility to rheumatoid arthritis: a systematic review. J Rheumatol 2004; 31: pp. 1310-1319
- 71. Pedersen M., Stripp C., Klarlund M., et al: Diet and risk of rheumatoid arthritis in a prospective cohort. J Rheumatol 2005; 32: pp. 1249-1252
- 72. Rosell M., Wesley A.M., Rydin K., et al: Dietary fish and fish oil and the risk of rheumatoid arthritis. Epidemiology 2009; 20: pp. 896-901
- 73. Shapiro J.A., Koepsell T.D., Voigt L.F., et al: Diet and rheumatoid arthritis in women: a possible protective effect of fish consumption. Epidemiology 1996; 7: pp. 256-263
- 74. Song G.G., Bae S.C., and Lee Y.H.: Association between vitamin D intake and the risk of rheumatoid arthritis: a meta-analysis. Clin Rheumatol 2012; 31: pp. 1733-1739
- 75. Nielen M.M., van S.D., Lems W.F., et al: Vitamin D deficiency does not increase the risk of rheumatoid arthritis: comment on the article by Merlino, et al. Arthritis Rheum 2006; 54: pp. 3719-3720
- 76. Feser M., Derber L.A., Deane K.D., et al: Plasma 25,OH vitamin D concentrations are not associated with rheumatoid arthritis (RA)-related autoantibodies in individuals at elevated risk for RA. J Rheumatol 2009; 36: pp. 943-946
- 77. Hiraki L.T., Munger K.L., Costenbader K.H., et al: Dietary intake of vitamin D during adolescence and risk of adult-onset systemic lupus erythematosus and rheumatoid arthritis. Arthritis Care Res (Hoboken) 2012; 64: pp. 1829-1836
- 78. Arkema E.V., Hart J.E., Bertrand K.A., et al: Exposure to ultraviolet-B and risk of developing rheumatoid arthritis among women in the Nurses’ Health Study. Ann Rheum Dis 2013; 72: pp. 506-511
- 79. Cerhan J.R., Saag K.G., Merlino L.A., et al: Antioxidant micronutrients and risk of rheumatoid arthritis in a cohort of older women. Am J Epidemiol 2003; 157: pp. 345-354
- 80. Comstock G.W., Burke A.E., Hoffman S.C., et al: Serum concentrations of alpha tocopherol, beta carotene, and retinol preceding the diagnosis of rheumatoid arthritis and systemic lupus erythematosus. Ann Rheum Dis 1997; 56: pp. 323-325
- 81. Heliovaara M., Knekt P., Aho K., et al: Serum antioxidants and risk of rheumatoid arthritis. Ann Rheum Dis 1994; 53: pp. 51-53
- 82. Knekt P., Heliovaara M., Aho K., et al: Serum selenium, serum alpha-tocopherol, and the risk of rheumatoid arthritis. Epidemiology 2000; 11: pp. 402-405
- 83. Pattison D.J., Silman A.J., Goodson N.J., et al: Vitamin C and the risk of developing inflammatory polyarthritis: prospective nested case-control study. Ann Rheum Dis 2004; 63: pp. 843-847
- 84. Costenbader K.H., Kang J.H., and Karlson E.W.: Antioxidant intake and risks of rheumatoid arthritis and systemic lupus erythematosus in women. Am J Epidemiol 2010; 172: pp. 205-216
- 85. Pattison D.J., Symmons D.P., Lunt M., et al: Dietary beta-cryptoxanthin and inflammatory polyarthritis: results from a population-based prospective study. Am J Clin Nutr 2005; 82: pp. 451-455
- 86. Afridi H.I., Kazi T.G., Brabazon D., et al: Association between essential trace and toxic elements in scalp hair samples of smokers rheumatoid arthritis subjects. Sci Total Environ 2011; 412–413: pp. 93-100
- 87. Afridi H.I., Kazi T.G., Brabazon D., et al: Interaction between zinc, cadmium, and lead in scalp hair samples of Pakistani and Irish smokers rheumatoid arthritis subjects in relation to controls. Biol Trace Elem Res 2012; 148: pp. 139-147
- 88. Bergstrom U., Jacobsson L.T., Nilsson J.A., et al: Pulmonary dysfunction, smoking, socioeconomic status and the risk of developing rheumatoid arthritis. Rheumatology (Oxford) 2011; 50: pp. 2005-2013
- 89. Cooper G.S., Miller F.W., and Germolec D.R.: Occupational exposures and autoimmune diseases. Int Immunopharmacol 2002; 2: pp. 303-313
- 90. Khuder S.A., Peshimam A.Z., and Agraharam S.: Environmental risk factors for rheumatoid arthritis. Rev Environ Health 2002; 17: pp. 307-315
- 91. Olsson A.R., Skogh T., and Wingren G.: Occupational determinants for rheumatoid arthritis. Scand J Work Environ Health 2000; 26: pp. 243-249
- 92. Turner S., and Cherry N.: Rheumatoid arthritis in workers exposed to silica in the pottery industry. Occup Environ Med 2000; 57: pp. 443-447
- 93. Stolt P., Yahya A., Bengtsson C., et al: Silica exposure among male current smokers is associated with a high risk of developing ACPA-positive rheumatoid arthritis. Ann Rheum Dis 2010; 69: pp. 1072-1076
- 94. De Roos A.J., Cooper G.S., Alavanja M.C., et al: Rheumatoid arthritis among women in the Agricultural Health Study: risk associated with farming activities and exposures. Ann Epidemiol 2005; 15: pp. 762-770
- 95. Olsson A.R., Skogh T., Axelson O., et al: Occupations and exposures in the work environment as determinants for rheumatoid arthritis. Occup Environ Med 2004; 61: pp. 233-238
- 96. Sverdrup B., Kallberg H., Bengtsson C., et al: Association between occupational exposure to mineral oil and rheumatoid arthritis: results from the Swedish EIRA case-control study. Arthritis Res Ther 2005; 7: pp. R1296-R1303
- 97. Stolt P., Kallberg H., Lundberg I., et al: Silica exposure is associated with increased risk of developing rheumatoid arthritis: results from the Swedish EIRA study. Ann Rheum Dis 2005; 64: pp. 582-586
- 98. Gan R.W., Deane K.D., Zerbe G.O., et al: Relationship between air pollution and positivity of RA-related autoantibodies in individuals without established RA: a report on SERA. Ann Rheum Dis 2013; 72: pp. 2002-2005
- 99. Hart J.E., Kallberg H., Laden F., et al: Ambient air pollution exposures and risk of rheumatoid arthritis. Arthritis Care Res (Hoboken) 2013; 65: pp. 1190-1196
- 100. Hart J.E., Kallberg H., Laden F., et al: Ambient air pollution exposures and risk of rheumatoid arthritis: results from the Swedish EIRA case-control study. Ann Rheum Dis 2013; 72: pp. 888-894
- 101. Ray P., Black S., Shinefield H., et al: Risk of rheumatoid arthritis following vaccination with tetanus, influenza and hepatitis B vaccines among persons 15-59 years of age. Vaccine 2011; 29: pp. 6592-6597
- 102. Blaschke S., Schwarz G., Moneke D., et al: Epstein-Barr virus infection in peripheral blood mononuclear cells, synovial fluid cells, and synovial membranes of patients with rheumatoid arthritis. J Rheumatol 2000; 27: pp. 866-873
- 103. Buskila D., Shnaider A., Neumann L., et al: Musculoskeletal manifestations and autoantibody profile in 90 hepatitis C virus infected Israeli patients. Semin Arthritis Rheum 1998; 28: pp. 107-113
- 104. Sawada T., Hirohata S., Inoue T., et al: Development of rheumatoid arthritis after hepatitis C virus infection. Arthritis Rheum 1991; 34: pp. 1620-1621
- 105. Medina-Rodriguez F., Guzman C., Jara L.J., et al: Rheumatic manifestations in human immunodeficiency virus positive and negative individuals: a study of 2 populations with similar risk factors. J Rheumatol 1993; 20: pp. 1880-1884
- 106. Saebo A., and Lassen J.: [object Object]. Int J Tissue React 1994; 16: pp. 51-57
- 107. Schaeverbeke T., Vernhes J.P., Lequen L., et al: Mycoplasmas and arthritides. Rev Rhum Engl Ed 1997; 64: pp. 120-128
- 108. Hitchon C.A., Chandad F., Ferucci E.D., et al: Antibodies to . J Rheumatol 2010; 37: pp. 1105-1112
- 109. Mikuls T.R., Thiele G.M., Deane K.D., et al: [object Object]. Arthritis Rheum 2012; 64: pp. 3522-3530
- 110. Bengtsson C., Kapetanovic M.C., Kallberg H., et al: Common vaccinations among adults do not increase the risk of developing rheumatoid arthritis: results from the Swedish EIRA study. Ann Rheum Dis 2010; 69: pp. 1831-1833
- 111. Cohen A.D., and Shoenfeld Y.: Vaccine-induced autoimmunity. J Autoimmun 1996; 9: pp. 699-703
- 112. Schattner A.: Consequence or coincidence? The occurrence, pathogenesis and significance of autoimmune manifestations after viral vaccines. Vaccine 2005; 23: pp. 3876-3886
- 113. Shoenfeld Y., and Aron-Maor A.: Vaccination and autoimmunity-‘vaccinosis’: a dangerous liaison? J Autoimmun 2000; 14: pp. 1-10
- 114. Symmons D.P., and Chakravarty K.: Can immunisation trigger rheumatoid arthritis? Ann Rheum Dis 1993; 52: pp. 843-844
- 115. Boyer J.F., Gourraud P.A., Cantagrel A., et al: Traditional cardiovascular risk factors in rheumatoid arthritis: a meta-analysis. Joint Bone Spine 2011; 78: pp. 179-183
- 116. Reynisdottir G., Karimi R., Joshua V., et al: Structural changes and antibody enrichment in the lungs are early features of anti-citrullinated protein antibody-positive rheumatoid arthritis. Arthritis Rheumatol 2014; 66: pp. 31-39
- 117. Verstappen S.M., Lunt M., Luben R.N., et al: Demographic and disease-related predictors of abnormal lung function in patients with established inflammatory polyarthritis and a comparison with the general population. Ann Rheum Dis 2013; 72: pp. 1517-1523
- 118. Demoruelle M.K., Weisman M.H., Simonian P.L., et al: Brief report: airways abnormalities and rheumatoid arthritis-related autoantibodies in subjects without arthritis: early injury or initiating site of autoimmunity? Arthritis Rheum 2012; 64: pp. 1756-1761
- 119. Oken R.J., and Schulzer M.: At issue: schizophrenia and rheumatoid arthritis: the negative association revisited. Schizophr Bull 1999; 25: pp. 625-638
- 120. Crowson C.S., Matteson E.L., Davis J.M., et al: Contribution of obesity to the rise in incidence of rheumatoid arthritis. Arthritis Care Res (Hoboken) 2013; 65: pp. 71-77
- 121. de Hair M.J., Landewe R.B., van de Sande M.G., et al: Smoking and overweight determine the likelihood of developing rheumatoid arthritis. Ann Rheum Dis 2013; 72: pp. 1654-1658
- 122. Symmons D.P., Bankhead C.R., Harrison B.J., et al: Blood transfusion, smoking, and obesity as risk factors for the development of rheumatoid arthritis: results from a primary care-based incident case-control study in Norfolk, England. Arthritis Rheum 1997; 40: pp. 1955-1961
- 123. Wesley A., Bengtsson C., Elkan A.C., et al: Association between body mass index and anti-citrullinated protein antibody-positive and anti-citrullinated protein antibody-negative rheumatoid arthritis: results from a population-based case-control study. Arthritis Care Res (Hoboken) 2013; 65: pp. 107-112
- 124. Kang J.H., and Lin H.C.: Obstructive sleep apnea and the risk of autoimmune diseases: a longitudinal population-based study. Sleep Med 2012; 13: pp. 583-588
- 125. Maki-Petaja K.M., Booth A.D., Hall F.C., et al: Ezetimibe and simvastatin reduce inflammation, disease activity, and aortic stiffness and improve endothelial function in rheumatoid arthritis. J Am Coll Cardiol 2007; 50: pp. 852-858
- 126. Steiner G., and Urowitz M.B.: Lipid profiles in patients with rheumatoid arthritis: mechanisms and the impact of treatment. Semin Arthritis Rheum 2009; 38: pp. 372-381
- 127. van de Stadt L.A., van Sijl A.M., van S.D., et al: Dyslipidaemia in patients with seropositive arthralgia predicts the development of arthritis. Ann Rheum Dis 2012; 71: pp. 1915-1916
- 128. van Halm V.P., Nielen M.M., Nurmohamed M.T., et al: Lipids and inflammation: serial measurements of the lipid profile of blood donors who later developed rheumatoid arthritis. Ann Rheum Dis 2007; 66: pp. 184-188
- 129. Myasoedova E., Crowson C.S., Kremers H.M., et al: Total cholesterol and LDL levels decrease before rheumatoid arthritis. Ann Rheum Dis 2010; 69: pp. 1310-1314
- 130. Dobson R., and Giovannoni G.: Autoimmune disease in people with multiple sclerosis and their relatives: a systematic review and meta-analysis. J Neurol 2013; 260: pp. 1272-1285
- 131. Avouac J., Gossec L., and Dougados M.: Diagnostic and predictive value of anti-cyclic citrullinated protein antibodies in rheumatoid arthritis: a systematic literature review. Ann Rheum Dis 2006; 65: pp. 845-851
- 132. Berglin E., Padyukov L., Sundin U., et al: A combination of autoantibodies to cyclic citrullinated peptide (CCP) and HLA-DRB1 locus antigens is strongly associated with future onset of rheumatoid arthritis. Arthritis Res Ther 2004; 6: pp. R303-R308
- 133. Brink M., Hansson M., Mathsson L., et al: Multiplex analyses of antibodies against citrullinated peptides in individuals prior to development of rheumatoid arthritis. Arthritis Rheum 2013; 65: pp. 899-910
- 134. Dorner T., and Hansen A.: Autoantibodies in normals–the value of predicting rheumatoid arthritis. Arthritis Res Ther 2004; 6: pp. 282-284
- 135. Kokkonen H., Mullazehi M., Berglin E., et al: Antibodies of IgG, IgA and IgM isotypes against cyclic citrullinated peptide precede the development of rheumatoid arthritis. Arthritis Res Ther 2011; 13: pp. R13
- 136. Majka D.S., and Holers V.M.: Can we accurately predict the development of rheumatoid arthritis in the preclinical phase? Arthritis Rheum 2003; 48: pp. 2701-2705
- 137. Nielen M.M., van S.D., Reesink H.W., et al: Specific autoantibodies precede the symptoms of rheumatoid arthritis: a study of serial measurements in blood donors. Arthritis Rheum 2004; 50: pp. 380-386
- 138. Rantapaa-Dahlqvist S., de Jong B.A., Berglin E., et al: Antibodies against cyclic citrullinated peptide and IgA rheumatoid factor predict the development of rheumatoid arthritis. Arthritis Rheum 2003; 48: pp. 2741-2749
- 139. Saraux A., Berthelot J.M., Chales G., et al: Value of laboratory tests in early prediction of rheumatoid arthritis. Arthritis Rheum 2002; 47: pp. 155-165
- 140. Scofield R.H.: Autoantibodies as predictors of disease. Lancet 2004; 363: pp. 1544-1546
- 141. Koivula M.K., Heliovaara M., Rissanen H., et al: Antibodies binding to citrullinated telopeptides of type I and type II collagens and to mutated citrullinated vimentin synergistically predict the development of seropositive rheumatoid arthritis. Ann Rheum Dis 2012; 71: pp. 1666-1670
- 142. Nielsen S.F., Bojesen S.E., Schnohr P., et al: Elevated rheumatoid factor and long term risk of rheumatoid arthritis: a prospective cohort study. BMJ 2012; 345: pp. e5244
- 143. van de Stadt L.A., van der Horst A.R., de Koning M.H., et al: The extent of the anti-citrullinated protein antibody repertoire is associated with arthritis development in patients with seropositive arthralgia. Ann Rheum Dis 2011; 70: pp. 128-133
- 144. Bos W.H., Wolbink G.J., Boers M., et al: Arthritis development in patients with arthralgia is strongly associated with anti-citrullinated protein antibody status: a prospective cohort study. Ann Rheum Dis 2010; 69: pp. 490-494
- 145. van de Stadt L.A., Witte B.I., Bos W.H., et al: A prediction rule for the development of arthritis in seropositive arthralgia patients. Ann Rheum Dis 2013; 72: pp. 1920-1926
- 146. Shi J., van de Stadt L.A., Levarht E.W., et al: Anti-carbamylated protein antibodies are present in arthralgia patients and predict the development of rheumatoid arthritis. Arthritis Rheum 2013; 65: pp. 911-915
- 147. Kolfenbach J.R., Deane K.D., Derber L.A., et al: Autoimmunity to peptidyl arginine deiminase type 4 precedes clinical onset of rheumatoid arthritis. Arthritis Rheum 2010; 62: pp. 2633-2639
- 148. Aho K., Palosuo T., Knekt P., et al: Serum C-reactive protein does not predict rheumatoid arthritis. J Rheumatol 2000; 27: pp. 1136-1138
- 149. Barra L., Summers K., Bell D., et al: Serum cytokine profile of unaffected first-degree relatives of patients with rheumatoid arthritis. J Rheumatol 2014; 41: pp. 280-285
- 150. Deane K.D., O’Donnell C.I., Hueber W., et al: The number of elevated cytokines and chemokines in preclinical seropositive rheumatoid arthritis predicts time to diagnosis in an age-dependent manner. Arthritis Rheum 2010; 62: pp. 3161-3172
- 151. Edwards C.J., and Cooper C.: Early environmental factors and rheumatoid arthritis. Clin Exp Immunol 2006; 143: pp. 1-5
- 152. El-Gabalawy H.S., Robinson D.B., Smolik I., et al: Familial clustering of the serum cytokine profile in the relatives of rheumatoid arthritis patients. Arthritis Rheum 2012; 64: pp. 1720-1729
- 153. Hughes-Austin J.M., Deane K.D., Derber L.A., et al: Multiple cytokines and chemokines are associated with rheumatoid arthritis-related autoimmunity in first-degree relatives without rheumatoid arthritis: studies of the Aetiology of Rheumatoid Arthritis (SERA). Ann Rheum Dis 2013; 72: pp. 901-907
- 154. Jorgensen K.T., Wiik A., Pedersen M., et al: Cytokines, autoantibodies and viral antibodies in premorbid and postdiagnostic sera from patients with rheumatoid arthritis: case-control study nested in a cohort of Norwegian blood donors. Ann Rheum Dis 2008; 67: pp. 860-866
- 155. Karlson E.W., Chibnik L.B., Tworoger S.S., et al: Biomarkers of inflammation and development of rheumatoid arthritis in women from two prospective cohort studies. Arthritis Rheum 2009; 60: pp. 641-652
- 156. Limper M., van de Stadt L., Bos W., et al: The acute-phase response is not predictive for the development of arthritis in seropositive arthralgia – a prospective cohort study. J Rheumatol 2012; 39: pp. 1914-1917
- 157. Masi A.T., Aldag J.C., and Sipes J.: Do elevated levels of serum C-reactive protein predict rheumatoid arthritis in men: correlations with pre-RA status and baseline positive rheumatoid factors. J Rheumatol 2001; 28: pp. 2359-2361
- 158. Nielen M.M., van S.D., Reesink H.W., et al: Increased levels of C-reactive protein in serum from blood donors before the onset of rheumatoid arthritis. Arthritis Rheum 2004; 50: pp. 2423-2427
- 159. Rantapaa-Dahlqvist S., Boman K., Tarkowski A., et al: Up regulation of monocyte chemoattractant protein-1 expression in anti-citrulline antibody and immunoglobulin M rheumatoid factor positive subjects precedes onset of inflammatory response and development of overt rheumatoid arthritis. Ann Rheum Dis 2007; 66: pp. 121-123
- 160. Sokolove J., Bromberg R., Deane K.D., et al: Autoantibody epitope spreading in the pre-clinical phase predicts progression to rheumatoid arthritis. PLoS One 2012; 7: pp. e35296
- 161. Turesson C., Bergstrom U., Jacobsson L.T., et al: Increased cartilage turnover and circulating autoantibodies in different subsets before the clinical onset of rheumatoid arthritis. Ann Rheum Dis 2011; 70: pp. 520-522
- 162. Woolf A.D., Hall N.D., Goulding N.J., et al: Predictors of the long-term outcome of early synovitis: a 5-year follow-up study. Br J Rheumatol 1991; 30: pp. 251-254
- 163. van Baarsen L.G., Wijbrandts C.A., Timmer T.C., et al: Synovial tissue heterogeneity in rheumatoid arthritis in relation to disease activity and biomarkers in peripheral blood. Arthritis Rheum 2010; 62: pp. 1602-1607
- 164. Lubbers J., Brink M., van de Stadt L.A., et al: The type I IFN signature as a biomarker of preclinical rheumatoid arthritis. Ann Rheum Dis 2013; 72: pp. 776-780
- 165. van de Stadt L.A., Bos W.H., Meursinge R.M., et al: The value of ultrasonography in predicting arthritis in auto-antibody positive arthralgia patients: a prospective cohort study. Arthritis Res Ther 2010; 12: pp. R98
- 166. Rakieh C., Nam J.L., Hunt L., et al: Predicting the development of clinical arthritis in anti-CCP positive individuals with non-specific musculoskeletal symptoms: a prospective observational cohort study. Ann Rheum Dis 2014; undefined:
- 167. de Bois M.H., Arndt J.W., Speyer I., et al: Technetium-99m labelled human immunoglobulin scintigraphy predicts rheumatoid arthritis in patients with arthralgia. Scand J Rheumatol 1996; 25: pp. 155-158
- 168. Shearman J., Esdaile J., Hawkins D., et al: Predictive value of radionuclide joint scintigrams. Arthritis Rheum 1982; 25: pp. 83-86
- 169. Gent Y.Y., Voskuyl A.E., Kloet R.W., et al: Macrophage positron emission tomography imaging as a biomarker for preclinical rheumatoid arthritis: findings of a prospective pilot study. Arthritis Rheum 2012; 64: pp. 62-66
- 170. van Steenbergen H.W., van Nies J.A., Huizinga T.W., et al: Characterising arthralgia in the preclinical phase of rheumatoid arthritis using MRI. Ann Rheum Dis 2014; undefined:
- 171. van Steenbergen H.W., van Nies J.A., Huizinga T.W., et al: Subclinical inflammation on MRI of hand and foot of anti-citrullinated peptide antibody-negative arthralgia patients at risk for rheumatoid arthritis. Arthritis Res Ther 2014; 16: pp. R92
- 172. Sparks J.A., Chen C.Y., Jiang X., et al: Improved performance of epidemiologic and genetic risk models for rheumatoid arthritis serologic phenotypes using family history. Ann Rheum Dis 2014; undefined:
- 173. Young K.A., Deane K.D., Derber L.A., et al: Relatives without rheumatoid arthritis show reactivity to anti-citrullinated protein/peptide antibodies that are associated with arthritis-related traits: studies of the etiology of rheumatoid arthritis. Arthritis Rheum 2013; 65: pp. 1995-2004
- 174. Karlson E.W., Ding B., Keenan B.T., et al: Association of environmental and genetic factors and gene-environment interactions with risk of developing rheumatoid arthritis. Arthritis Care Res (Hoboken) 2013; 65: pp. 1147-1156
- 175. Bos W.H., Dijkmans B.A., Boers M., et al: Effect of dexamethasone on autoantibody levels and arthritis development in patients with arthralgia: a randomised trial. Ann Rheum Dis 2010; 69: pp. 571-574
- 176. Karlson E.W., Shadick N.A., Cook N.R., et al: Vitamin E in the primary prevention of rheumatoid arthritis: the Women’s Health Study. Arthritis Rheum 2008; 59: pp. 1589-1595
- 177. del P.A., Knowler W.C., Pettitt D.J., et al: The incidence of rheumatoid arthritis is predicted by rheumatoid factor titer in a longitudinal population study. Arthritis Rheum 1988; 31: pp. 1239-1244
- 178. Peschken C.A., Hitchon C.A., Robinson D.B., et al: Rheumatoid arthritis in a North American native population: longitudinal followup and comparison with a white population. J Rheumatol 2010; 37: pp. 1589-1595
- 179. Stack R.J., Sahni M., Mallen C.D., et al: Symptom complexes at the earliest phases of rheumatoid arthritis: a synthesis of the qualitative literature. Arthritis Care Res (Hoboken) 2013; 65: pp. 1916-1926
- 180. Available at: http://www.trialregister.nl/trialreg/admin/rctsearch.asp?Term=prairi. Accessed on June 20, 2014.
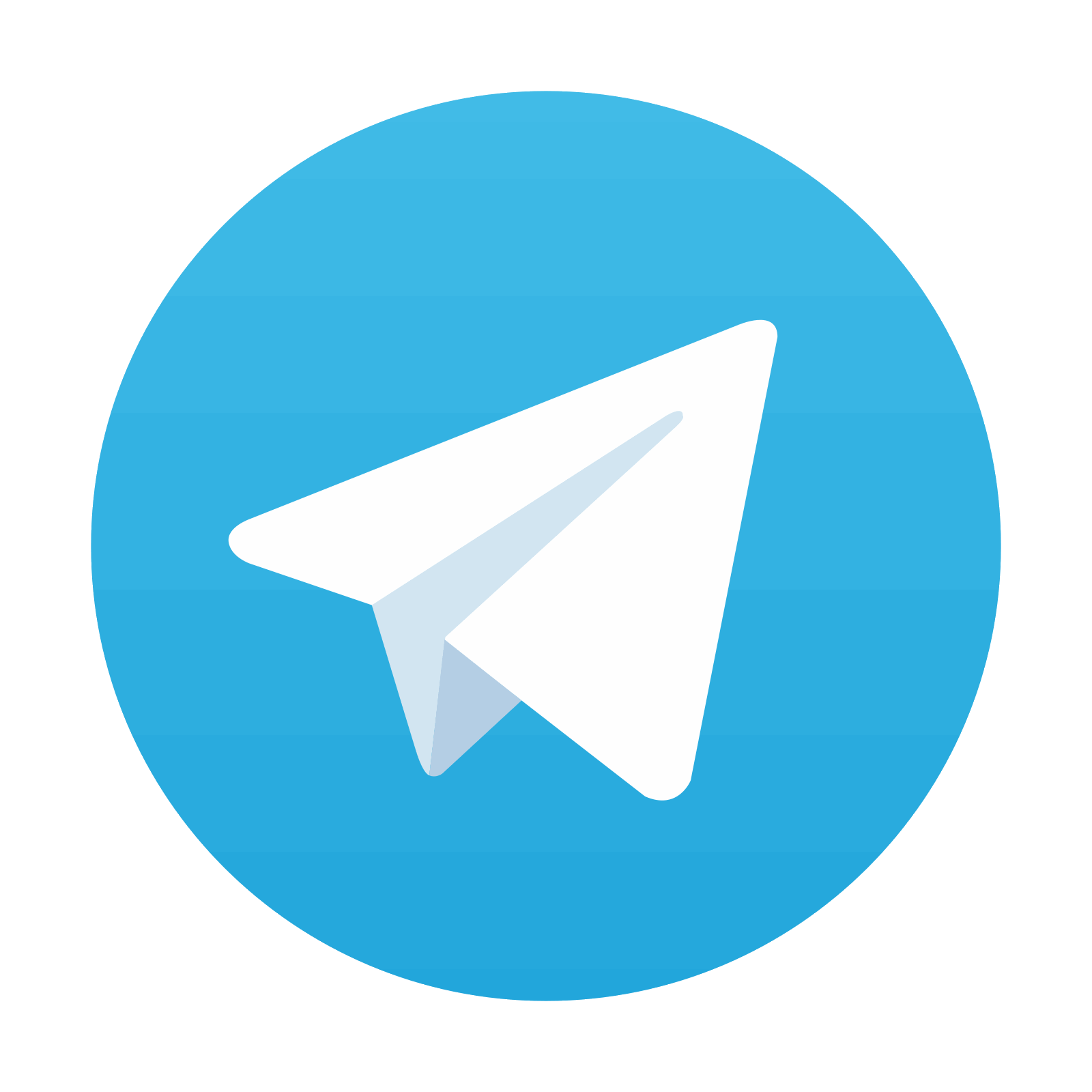
Stay updated, free articles. Join our Telegram channel

Full access? Get Clinical Tree
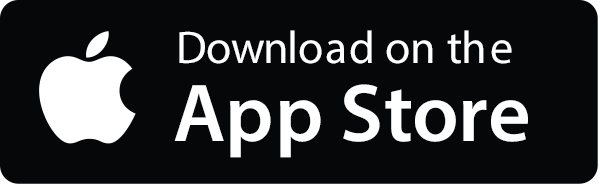
