Fig. 13.1
Overview network analysis. Simplified workflow of the network analysis integrating GWAS and transcriptome data to search for disease-specific sub-networks in DD. In the first step SNP-based p-values are translated into gene-based p-values. Genes with p-values are then imposed on protein-protein interaction (PPI) data, and a search for connections between genes with small p-values is conducted. Once sub-networks (modules) enriched for genes with small p-values are identified, these can be validated and further analyzed for functional annotation in the context of the disease. To further increase the power to detect relevant sub-networks, tissue-specific expression data can also be integrated in the network analysis approach
13.3.1 Network Analysis Workflow
In the first step SNP-based p-values are translated into gene-based p-values. For this, SNPs must be assigned to genes. The simplest method to do this is to define a window around each gene and assign all SNPs within this window to this gene. But this is no trivial task as SNPs not necessarily act on the nearest gene and long-range interactions are possible. We used the software VEGAS2 (Mishra and Macgregor 2014), which also takes into account linkage information (e.g., from the 1000 Genomes Project reference population) and gene sizes. VEGAS2 combines the test statistics of all SNPs within ±50 kb of each gene. Based on SNP association p-values, the software calculates empirical gene-based p-values by a simulation procedure.
The next step is to search for modules enriched in small p-values within a protein-protein interaction (PPI) dataset. The assumption behind is that in complex genetic settings, many different variants affecting several different genes may contribute to the disease, but these genes are assumed to act in a limited number of pathways or cellular functions. Because of the limited number of affected pathways/cellular functions, truly associated genes are expected to be more functionally connected to each other than random genes. The search for modules instead of individual genes increases statistical power since association does not rely on individual genes but a module of functionally connected genes.
To further increase the power to detect true associations in the statistical noise of GWAS, one can combine the GWAS data with tissue-specific whole-genome transcription data by considering only genes that are expressed or co-expressed in the tissue of interest when searching for connections between genes with small p-values.
Network analysis results in lists of genes. The next logical step to do is to look for enrichment of functional annotations in these lists of genes (e.g., canonical pathways or gene ontology (GO) terms). Although the function of all genes in a sub-network may not be known, this constitutes the first insight into which pathways may be affected by genetic alterations in DD. The ultimate aim is to unravel which roles the specific genes in the detected sub-networks play in the pathway in the context of the disease and how genetic alterations change these functions in DD. For these more functional experimental, studies are necessary.
13.4 Targeted Sequencing
13.4.1 Ongoing Studies to Identify Genetic Variants in GWAS Loci by Targeted NGS
The SNPs tested in GWAS are selected as a set of informative single SNPs able to tag common haplotype blocks. To explicitly capture causative variants in GWAS-identified DD susceptibility loci, it will be critical to sequence each candidate locus using targeted next-generation sequencing (NGS). By mapping NGS data to the human genome reference sequence, the variability of the entire locus can be exhaustively identified, including both coding and noncoding regions and comprising all common and rare variants (Udler et al. 2010).
As a first step, we have selected a 500 kb region containing the lead SNP rs16879765 (chromosome 7p14.1) for targeted sequencing (Fig. 13.2). DNA was isolated from peripheral blood of 96 DD patients. The DD-associated locus was enriched in these samples using a custom designed Agilent SureSelect XT2 kit and sequenced on the Illumina HiSeq 2000 platform. Sequencing data are analyzed with the Varbank pipeline (v2.13) (CCG, Cologne) and Ensembl Variant Effect Predictor (http://www.ensembl.org/info/docs/variation/vep/index.html).


Fig. 13.2
UCSC Genome Browser plot of target region containing rs16879798 for enrichment capturing and sequencing. UCSC Genome Browser plot of target region containing rs16879798 for enrichment captures and sequencing. Target region: chr7:37,714,869 – 38,214,857. Predicted Agilent SureSelect XT2 coverage: 98.3 %
Once the potential candidate variants are discovered and validated, the next step will be to prioritize the candidates based on the following criteria: (1) exclude known, assumed harmless variations present in dbSNP databases (http://www.ncbi.nlm.nih.gov/SNP) and published studies; (2) select variants causing changes in protein-coding sequences and likely to compromise protein structure, function, or stability; and (3) select noncoding variants that may affect regulation of gene expression.
13.4.2 Functional Studies of Variants Within Coding Regions
All coding variants identified in DD patients are validated by Sanger sequencing, in particular variants in regions that contain multiple and/or recurrent variants in patients as compared to controls. Then, replication of the results in an independent cohort is needed. The identified variations are analyzed to predict the structure of the gene carrying variations and the function of the resulting protein by using tools such as SIFT (http://sift.jcvi.org/www/SIFT_dbSNP.html), PolyPhen-2 (http://genetics.bwh.harvard.edu/pph2), Mutation Profiling (http://profile.mutdb.org), and ModBase (http://modbase.compbio.ucsf.edu). After identification of a set of DD-predisposing gene variants, in vitro studies to test the functional consequences of these candidates are crucial.
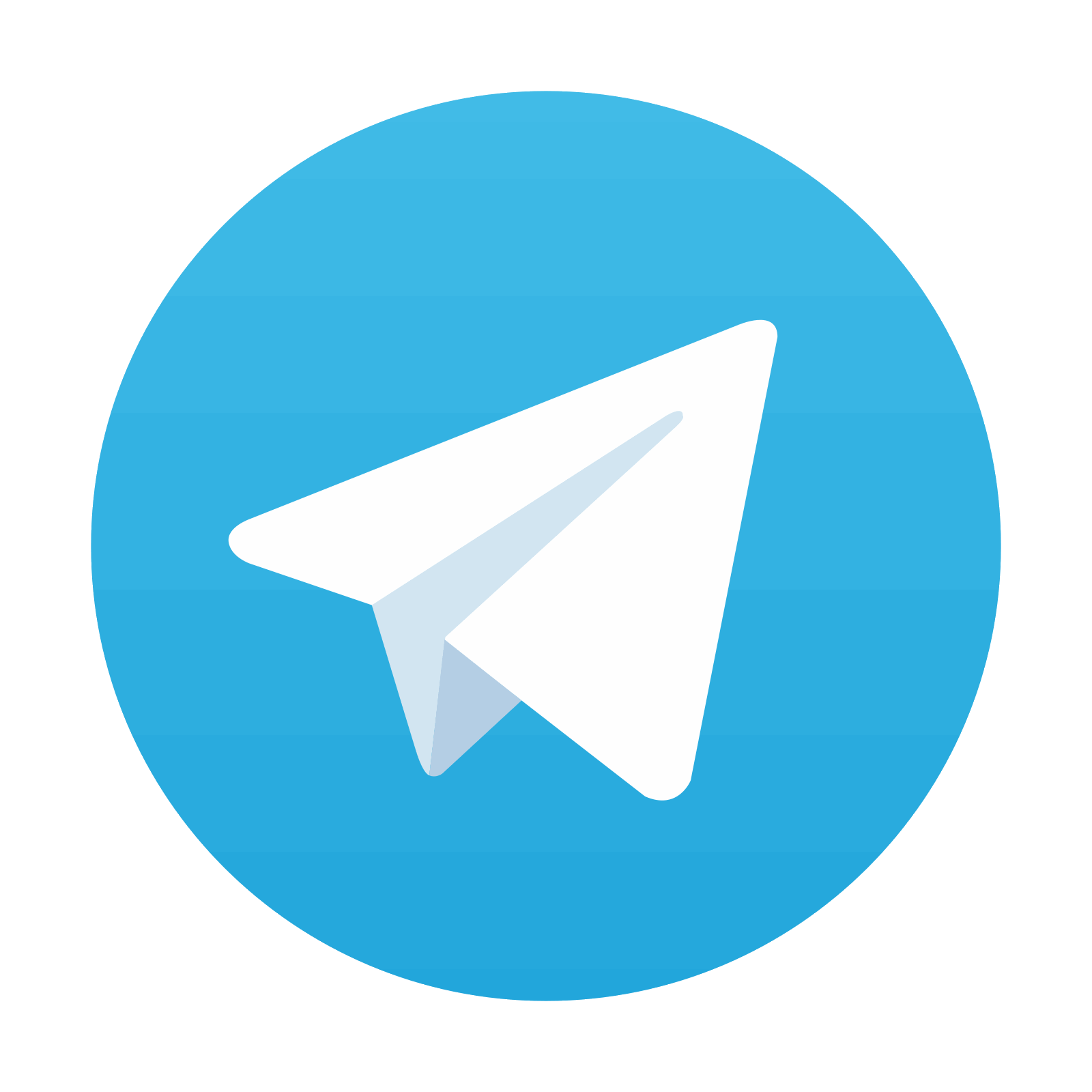
Stay updated, free articles. Join our Telegram channel

Full access? Get Clinical Tree
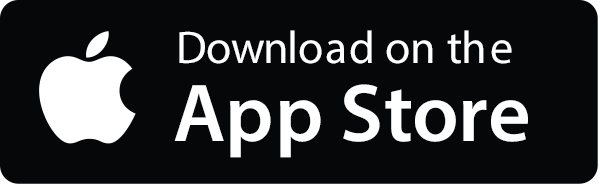
