Fig. 1.1
StrokeBack rehabilitation cycle
The project goal is achieved by investigating the following objectives:
Telemedicine supervision of rehabilitation exercise
Continuous monitoring of impact of exercises also in ‘normal’ life situations
Integration of telemedicine rehabilitation and Personal Health Records for improved long-term evaluation of patient recovery
Feedback to healthcare professionals on impact of rehabilitation exercises
In order to provide remote rehabilitation exercises at gold standard level, i.e. as good as in a face-to-face training with rehabilitation experts, we plan to exploit the advanced features of today’s body area networks (BAN). A BAN attached to the patient enables permanent monitoring of patients activity and vital parameters. We aim to monitor and record the patients’ activity enabling them to regularly, maybe daily, exercise independently from the guidance of the physical therapist. With a correct instrumentation, we expect to be able to detect also unwanted additional movements. In order to achieve a comparable monitoring by cameras, at least two of them need to be deployed at the patient’s home. This is a costly solution which also bears a privacy risk. Both issues can be solved with our BAN-based solution.
As one possible application, the physical therapist has to look after the patient once a week only to exploit the level of rehabilitation, to analyse the results of last exercises based on recorded data and to take corrective action if necessary. Furthermore, a physical therapist may show new exercises and configure a new exercise schedule. By that, we intend to boost the rehabilitation process at home.
The BAN can be worn by the patients throughout the whole day which enables us to compare actual movements in their daily life with the correct movement pattern define in rehabilitation exercises. To simplify the configuration process of the system, we analyse and evaluate self-learning techniques for exercise recognition, i.e. the StrokeBack system may learn the correct behaviour (patient’s movements) when exercises are carried out under instruction of the physical therapist.
We aim to additionally evaluate a feasibility and requirements of using electronic personal health records to store and document recorded data and to remotely track the rehabilitation process, e.g. by the attending doctor. This includes the recordings done during rehabilitation exercises and during daily life. The recorded data is stored and can then be processed by healthcare professionals. The evaluation can be used to deduce detailed information of effects of individual exercises. This feedback can be used to select exercises for other patients, to assess effectiveness of exercises for specific groups of patients, etc. In addition, the vital parameters can be used to assess the healthiness of the patients which might even help to assess the probability of a further stroke. In order for StrokeBack vision to become reality, the following research challenges need to be addressed:
High speed signal processing inside the BAN
Security and privacy inside BAN and PHS, including evaluation function
High confidence decision making on the basis of the pre-processed BAN data
The respective StrokeBack architecture is shown in Fig. 1.2.
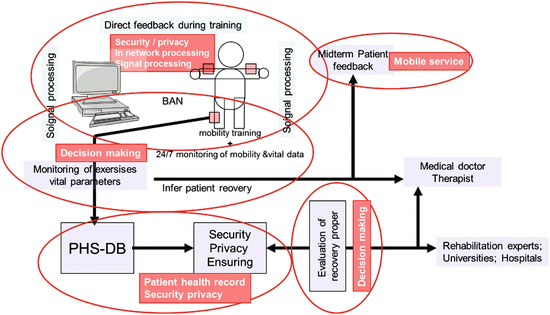
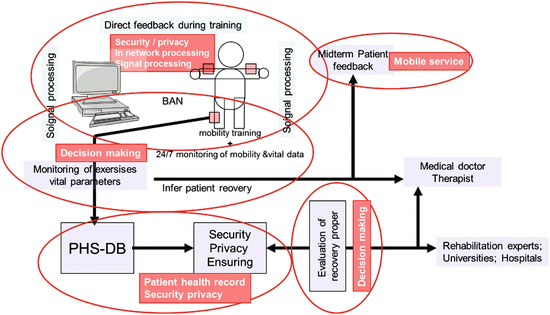
Fig. 1.2
Classification of research issues within the StrokeBack architecture
1.2 Relevance to Research Directions Defined by EU Commission
The European Commission has set research targets in its Research Agenda for European Framework programmes, including the Health domain.
Regarding rehabilitation of stroke and neurological conditions: the Commission seeks providing patient services at home, with tele–supervision by health professionals as and when required. The StrokeBack project follows this approach and aims at empowering stroke patients in doing physical therapy at home. It goes beyond the tele-assistance by implementing assessment features into the training equipment thus ensuring a correct execution of training sequences. This way it allows the patient to exercise more often than physical therapists are available. In addition, it provides an offline data to medical doctors as well as physical therapists to enable them to monitor their patients’ recovery process and evaluating the effect of selected training sequences.
From technology perspective the Commission looks for solutions may build on robotic and haptic technologies, wearable systems, implants, human–computer interfaces, web services or virtual reality environments to facilitate continuity of personalised cognitive and functional rehabilitation. In this context, the StrokeBack system consists of two major parts. One of them, the BAN tracks patient’s movements while a graphical HCI environment offers a virtual reality representation of rehabilitation exercises and the success level of the patient.
The proposed system further offers an auto–adaptive, self–calibrating and energy–efficient module with multi–sensing, advanced on–board processing, communication and actuation capabilities. The BAN can be realised by using resource constraint wireless sensor nodes deployed at the body of the patient. Those nodes are used to gather tracking data for body movements as well as patients vital parameters. The signal processing required to provide direct feedback to the patients on the execution quality of the exercises to be done at the BAN nodes. Such systems are expected to provide accuracy of measurements as well as remote control and reliable operation of the devices/systems. High level of accuracy is of movement detection is key for proper assessment of the exercises done by patients. This issue is tackled by using high-quality sensors, specific evaluation and calculation models and algorithms. The reliability of the system is enhanced by using an extra redundancy in the BAN. This is achieved by data replication in the BAN.
From system level the needs are to provide context–aware, multi–parametric monitoring of health parameters, activity, lifestyle, ambient environment and operational parameters of the devices. Therefore, BAN is used to gather vital parameters as well as movement patterns (by that also activity levels) throughout the whole day. The idea is to detect if and when movement patterns trained during the therapy is adapted during normal life. In addition, clinicians can evaluate the health state of patients on detail data over long-term period.
From sensing point of view solutions need to implement closed–loop approaches and integrate components into wearable, portable or implantable devices coupled with appropriate platforms and services. Such approaches offer analysis, interpretation and use of the multi–parametric data, in conjunction with established or newly created medical knowledge, for shared patient–doctor decision support systems. Furthermore, clinical workflows, guidelines and patient pathways to support remote applications. They also address interoperability issues related to heterogeneous data sources, devices and links with electronic health records (EHRs); the use of standards and of any suitable open software platform is recommended. In order to comply with such needs, the second part of the StrokeBack system implements a custom PHS, allowing for offline/online evaluation of patient data based on web services and by that allow for closed-loop assessment and monitoring when necessary. The online evaluation may be used to provide personal feedback from the therapist to the patient while exercising. The data collected via the StrokeBack system enables medical and therapeutic experts to offline deduce relations between the training done by patients and the improvement of the patients’ health status. As result of this evaluation, the therapist might adapt the exercises or try to increase the patient’s motivation, i.e. if the requested number of training sessions was not done by the patient. The analysis of collected data offers an additional benefit in revealing interdependencies of specific exercises and rehabilitation success. When designing the StrokeBack system, standardised approaches were used as much as possible in order to ensure its architectural design for interoperability.
The Strokeback system is an advanced system offering education and motivation of users. The project idea was partly influenced by the observation that patients who have been using gaming consoles as the WII were more motivated and exercised more than other patients. Hence, the project embedded the physical therapy training sequences into serious gaming applications. Furthermore, recording the number of executed exercises as well as the level of correctness allowed more accurate tracking of progress that the patients made. This has contributed to improving patients’ motivation, i.e. they can see that their effort has visible impact and this fact teaches patients indirectly since it shows them that proper exercising helps while laziness may lead to degradation of already achieved improvement.
An important aspect of the project was careful attention to user acceptance, patient compliance, patient data security and confidentiality. StrokeBack has investigated security/privacy issues in order to ensure confidentiality and data integrity in all parts of the system and in all stages of processing. This means strong, but energy-efficient crypto-operations implemented for ensuring confidentiality of patients data while transmitting it wirelessly in the BAN. Privacy protection was also investigated for protecting patients’ privacy when their data is analysed by medical experts, who are not directly their leading physicians or physical therapists. In order to ensure user acceptance, patients who had indicated their consent to take part in the project were involved from the very beginning in the design process.
An important aspect of any development project is certainly the evaluation of its results in clinical environment in order to demonstrate the proof of concept, efficiency gains and, if possible, cost-effectiveness of the proposed solution. Validation should include comparison versus currently accepted gold standards and include quantitative indicators of the added value and potential impact of the proposed solutions. The StrokeBack solutions has therefore been extensively evaluated throughout the whole project duration by clinicians and physical therapists with their patients. This way we were able to assess the quality of rehabilitation and patient acceptance early enough and use this feedback to improve the overall solutions.
1.3 Progress Beyond the State-of-the-Art
The StrokeBack project pursued advanced applied development in several key research and technological areas: Personal Health Record (PHR), Tracing Body Movement, Wireless Body Area Networks (W-BAN), Protection of Privacy and Private Data, Knowledge Representation and Reasoning (KRR) and Immersive Gaming Environments for Stroke Rehabilitation. Progress beyond the state-of-the-art in each of those areas is detailed in the following subsections.
1.3.1 Personal Health Record
The main objective of this activity was to develop an European online PHR database system as an alternative to the already existing US-based ones from Google (Google Health) and Microsoft (MS Health Vault) with advanced functionalities and fully compliant with European standards. Such a system complying with the latest HL7 standard would allow seamless integration with hospital and private medical assistance service systems. Having WSDL web services as the main programming interface, it simplifies and enables the development of secure third party systems, services and applications built upon the core PHR database. A significant innovation is the API programming layer and similar scripting device integration interface allowing to avoid pre-installation of the device support for each medical devices within the device layer of the core system before users can use the device to upload measurement data to their PHR account. Furthermore, the PHR therapy definition has been extended to include physical training/exercises prescribed by the physician in the same way that other medicines are prescribed. The added advantage of such an approach is to provide data to physicians for assessing the effectiveness of the exercises against the changing patient’s condition. In order to do this reliably, the correctness of performing such exercises needs to be carefully monitored against predefined templates (temporal and spatial record of body movements and their frequency/retentiveness, treated each as a different therapy type).
State-of-the-Art: EHR platforms have been developed through various European activities, ranging from LinkCare platform promoted by the LinkCare Alliance [9] or the intLIFE PHR platform [10] from Intracom, developed alongside the ICT-PSP-NEXES project [11]. The popularity of EHR/PHR systems has given rise to the development of open-source platform too [12–14]. Their capabilities and features can closely compete with commercial and proprietary implementation, except when it comes to interoperability and flexibility in developing add-on services and applications.
Beyond State-of-the-Art: The base PHR platform has been further enhanced with fully HL7 complaint web service programming interfaces, the PHR database has been supplemented with extra medical information as required by the NHS and/or necessary for supporting the specific project needs. Furthermore, an open-source approach enables an easier integration with existing applications and services contributed by project partners and easier integration with third party systems.
1.3.2 Tracing Body Movement
In order to enable the tracking, the correctness of performed exercises automatically without the constant assistance of the physicians, an automated means of tracking and comparing patient’s body movement against correct ones (templates) has been developed. Although many methods are in existence, most of them employ elaborate sets of wearable sensors and/or costly visual observations. In our project, we adopted initially visual-light scanning methods allowing computationally effective and cheap dynamic 3D object (in our case patient’s body) tracing. The idea was similar to laser scanning employed in the Kinect game interfaces for Xbox (now also available for Windows operating systems on PCs), but using specially designed images projected on the person. The part of the R&D work was to determine if shifting to light wavelengths just beyond visible spectrum (infrared and ultraviolet) would allow achieving necessary accuracy and performance. Considering extremely small sizes of such sensors (less than 5 × 5 mm each) a development of lightweight wireless energy-autonomous (employing energy harvesting technologies) were possible, leading to the target sensor node form factor of a small sticker. Considering a vast amount of products appearing in the recent years, the Strokeback project has also taken advantage of such products, among them the Leap Motion IR sensor [15], Emotiv EPOCH EEG sensor [16], Myo EMG sensor [17], etc.
State-of-the-Art: The need to monitor movement and activity both within and outside the laboratory has been the focus of considerable research over the last decade. Body-worn sensors such as goniometers [18], pressure tubes [19], gyroscopes [20] and accelerometers [21–24] have been used in a range of activities and clinical conditions [22, 24–29] and for control of Functional Electrical Stimulation (FES) in walking and upper limb movement [29–31] and in standing with spinal cord injured patients [32–34]. However, body-worn sensors are often bulky and require associated instrumentation and accurate placement of the sensor elements. Nevertheless, such studies have already demonstrated the feasibility and potential benefits of long-term monitoring of movement [35, 36] and what is needed now is a shift in approach to make this feasible and usable in clinical practice and particularly in the home environment for rehabilitation. Such an approach has already been embraced by the textile industry. The so-called smart textiles have been developed to measure vital signs, such as the Electrocardiogram (ECG), and have even been commercialised in, for example, the ProArmBand from SenseWear™ [37] or various systems from Vionetics [38]. Predictions are that wearable systems become a part of routine clinical investigations [39]. Movement and muscle activity are, however, more challenging than vital signs and, in addition, processing, storing, transmitting and using the data poses an even greater challenge. The SMART project (funded by the UK Engineering and Physical Sciences Research Council through the EQUAL initiative) has explored how technology can be used in the home to support rehabilitation following stroke. Devices have been developed that are attached to the wrist and upper arm using clothing resembling sportswear to give a measure of movements made by the patient in activities prescribed by the therapist. Data are recorded and displayed on a computer or TV screen. Joint angles have also been measured using conductive fibres integrated into fabric which change their resistance due to bending as the joint angle varies [32] and by covering the fabric with an electrically conductive elastomer with piezo-resistive properties so that when deformed acts as a strain sensor able to detect movement [40].
Muscle activity poses problems for measurement since it has been well known for many years [41] that the EMG reflects effort rather than output and so becomes an unreliable indicator of muscle force as the muscle becomes fatigued. Consequently measurement of force, in addition to the EMG activity, would be a considerable step forward in assessing the effectiveness of rehabilitation strategies and could not only indicate that fatigue is occurring, but also whether the mechanism is central or peripheral in origin [42]. Similarly, conventional surface EMG measurement requires accurate placement of the sensor over the target muscle, which would be inappropriate for a sensor system integrated within a garment for home use. Electrode arrays are, however, now being developed for EMG measurement and signal processing is used to optimise the signal obtained.
Some of the building blocks for a body sensor network of the form proposed are therefore already in place. Existing commercial systems provide basic information about activity such as speed and direction of movement and postures. Providing precise information about performance, for example, relating movement to muscle activity in a given task and detecting deviations from normal, expected patterns or subtle changes associated with recovery, requires a much higher level of sophistication of data acquisition and processing and interpretation. The challenge is therefore to design and develop an integrated multimodal system along with high-level signal processing techniques and optimisation of the data extracted. The Kinect sensor has potential for use in haptic interfacing [43] and software support has already been offered, while by Open Kinect Community [44] and the official Kinect SDK programming support offered by Microsoft [45].
Beyond State-of-the-Art: The existing techniques for taking measurements on the human body are generally considered to be adequate for the purpose but are often bulky in nature and cumbersome to mount, e.g. electro-goniometers, and they can also be expensive to implement, e.g. Vicon camera system [46]. Their ability to be used in a home environment is therefore very limited. This proposal addresses these deficiencies by extending the state-of-the-art in the following areas:
Extending the application of existing sensor technology into new areas. For example, we aim to use commercially available MEMS accelerometers with integrated wireless modules to measure joint angles on the upper and lower limbs to allow wire-free, low-cost sensor nodes that are optimised in terms of their information content and spatial location.
A study of novel-sensing methodologies to reduce the number of sensor nodes on the human body, while maintaining good information quality. For example, many homes now have at least one games console (e.g. Xbox, Nintendo Wii) as part of a typical family home entertainment system. With the advent of the Xbox Kinect system, the position and movement of a human can be monitored using a low-cost camera mounted below the television.
Easy installation and calibration of the system by non-experts for use in a non-clinical environment offering solution suitable for first-time home users.
Fully transparent verification whether the patient has done his/her exercises and if he/she has done them correctly. Based on data recorded by the BAN, correlation of prescribed therapies with medical condition allows determining their effectiveness on patient’s condition, either positive or negative.
1.3.3 Wireless Body Area Networks
The central aspect of the BAN is the data processing and handling. This is realised by the processing logic that is physically represented by the distributed system of nodes interconnected by (wireless) network means. All the features of this underlying system, like data handling middleware, compression, data aggregation or fusion, networking protocols and hardware architecture, help reaching the goals set by the primary aspect on different layers. Due to the small size of the network there is not really a need for sophisticated routing mechanisms. Thus, the MAC protocol becomes the central part of the networking.
State-of-the-Art: The wireless medium can be multiplexed in code (CDMA), space (SDMA), time (TDMA) and frequency (FDMA). Time multiplexing is best suited to be used for coordination of the communication between nodes in a single BAN. The main MAC protocols for BANs use TDMA and the access is random [47], contention-based [48–51] and or scheduled [52–67]. The schedule of the access can also be created as a result of a contention phase. The main advantages of schedule-based protocols is that they provide more predictable communication latency important for Quality of Service (QoS) and allow duty-cycling, i.e. saving energy by switching the radio off while there is no communication planned for a particular node.
Beyond State-of-the-Art: We intend to investigate a cross layer approach to ensure real-time behaviour of the protocol stack. Here, design and implementation is focused on adaptable TDMA-based data flow control to provide QoS for real-time evaluation of the movements tracked by the sensors attached to BAN nodes.
1.3.3.1 Proactive Data Storage Middleware
State-of-the-Art: There are several approaches that offer the data storage and management functionality in the field of sensor networks [68–74]. More specific approaches, i.e. [75–78] have been developed to ease the data handling in the application layer. They provide a middleware layer that abstracts the direct data and message exchange and simplifies the development of the processing logic module. TeenyLime [75] provides a possibility to share the data using a common set of tuples, available to the nodes in the network, i.e. every node can insert and remove a tuple to and from the shared set. The nodes can also be notified if a specific tuple is inserted into the set. In TinyDB [77], the network topology is a tree rooted at the central node that can order data from the sensor nodes on an on-demand or periodic basis, and the data is delivered down the tree. MacroLab [76] provides mechanisms to programme the network of nodes as a vector of data items. Such a global programme is then optimised for a chosen deployment platform and broken into individual programmes for the nodes. The tinyDSM [78] middleware provides a shared memory abstraction realised as a shared set of variables that can be defined as array with one item per node or as singletons. The former is similar to the vector in MacroLab, and the latter is similar to the tuple concept provided by TeenyLime. The tinyDSM is configurable to support the needs of the application and be optimal at the same time. It provides an event mechanism that notifies the application running on top of it in case a specified condition described using a logical equation with the shared variables as terms is fulfilled. It can be also extended to support security and privacy.
Beyond State-of-the-Art: The data collected by the sensors shall be stored and evaluated directly in the BAN. Such a pre-evaluation as an additional step in the data path helps to detect abnormal situations as fast as possible. In the StrokeBack project, we want to evolve the data storage middleware tinyDSM to support the distributed architecture of the StrokeBack BAN. The middleware shall provide a consistent view of the data stored together with mechanisms to evaluate the data to detect abnormal events of a predefined character. This might be deviations from expected movements but also deviations of the vital parameters. The efforts are mainly put in the data consistency research and in the migration of the pure software approach to a combined SW/HW one. The physical realisation includes microcontroller enhancements providing the DSM (Distributed Shared Memory) functionality to the Wireless Sensor Networks (WSN).
1.3.3.2 Signal Processing in BAN
State-of-the-Art: Medical data captured at the sensors require sophisticated signal processing for signal de-noising, artefact removal, signal classification and feature extraction. Wavelet Transform (WT), Kalman filtering and Short Time Fourier Transform (STFT) are widely used for these purposes [71, 79–82]. Approaches like Blind Source Separation (BSS), in particular Independent Component Analysis (ICA), has been used for accurately separating out the target signal from its artefacts and in its feature extraction [83, 84]. However, all the methods (traditional and advanced) mentioned above, although accurate to clinical standards, are extremely demanding in terms of computation. Thus, in a traditional patient monitoring system owing to the lack of energy (in terms of battery power) at the sensor nodes, these processes are carried out on a more powerful computation platform like a central server or using an on-board microprocessor. Therefore under the continuous monitoring scenario, the sensors are used as data collectors only and the unconditioned data are continuously transmitted to the central station for further processing. This puts a huge burden on the wireless network owing to the plethora of data to be transmitted continuously. In addition, the continuous data transmission over long distance puts high energy demand on the analogue front-end of the embedded radio structure leading to fast draining of the battery.
In addition to the regular signal processing tasks, in order to reduce the amount of data to be transmitted and; in the PREACTION system; to increase the on-sensor storage time-efficient data compression algorithm needs to be developed. Some data compression techniques provide an alternative to merely reducing the sampling frequency. These techniques can be characterised as either ‘lossy’ or ‘loss-less’. When data is compressed using a ‘loss-less’ method, it is possible to reconstruct the original waveform without losing information. Such non-distorting compression modes are exemplified by the Huffman coding method and the Lempel-Ziv method [85, 86]. Loss-less compression modes are computationally expensive, resulting in a more complex circuit design, increased power consumption, and a longer data processing time. Moreover, the compression rate that is achieved depends on the content of the physiological signals. The content may vary depending on the physiological condition being monitored. As a result, the amount of memory needed to store the compressed data cannot be determined in advance. As an alternative to the use of a loss-less compression process, a lossy technique may be employed. Lossy techniques (SAPA or ‘fan’ method [87], Wavelets [88, 89], Lifting Scheme WT or its combination with distributed source coding [90]) may require less processing power to implement. The resulting system may therefore be both smaller and more energy efficient. However, when data is compressed using a lossy method, some information is lost when the compressed data is later used to reconstruct the original waveform. However, owing to the required reliability in medical application it may not be always recommendable to use lossy compression.
Beyond State-of-the-Art: In contrast to the classical architecture of remote monitoring in our architecture, sophisticated signal processing is carried out within the BAN with an emphasis on medically trustworthy parameter extraction in order to reduce the burden on the network and transmission energy. Separate optimisation of signal processing algorithms and architecture cannot be applied here since this approach does not guarantee overall energy optimisation. Thus, a cooperative and holistic approach needs to be taken for increasing energy efficiency. Following this route in this part of the present proposal, we have developed novel energy optimal algorithms and architectures leading to an ultra-low-energy ASIC realisation for on-board signal de-noising, artefact removal and accurate feature extraction and classification. Especially, the latter allows measuring in real time how accurately the patient carries out the prescribed exercise from the data acquired by sensor nodes using low-complexity computer arithmetic.
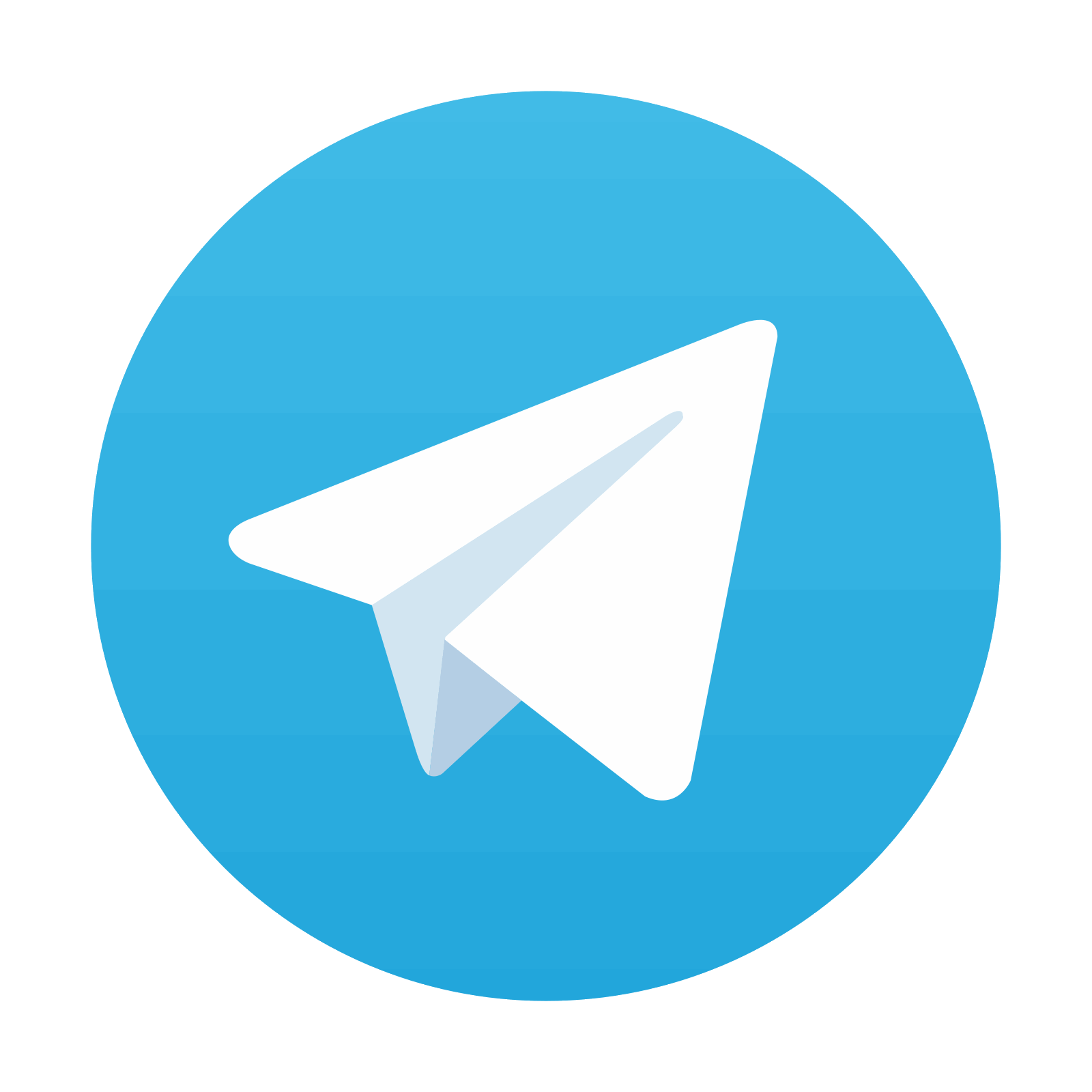
Stay updated, free articles. Join our Telegram channel

Full access? Get Clinical Tree
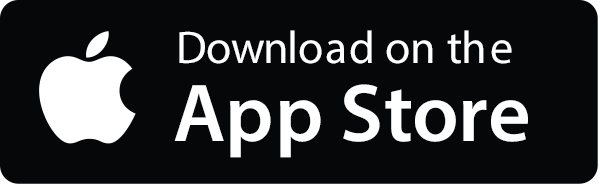
