Highlights
- •
The adaptation of a brain-computer interface (BCI) application may be optimized by the user-centered design approach.
- •
Effectiveness, efficiency and satisfaction are the 3 main aspects to be evaluated.
- •
The perceived workload of a BCI task affects patient performance.
- •
Mood, motivation and quality of life are also important aspects in BCI performance.
Abstract
Background
People who survive severe brain damage may eventually develop a prolonged consciousness disorder. Others can regain full consciousness but remain unable to speak or move because of the severity of the lesions, as for those with locked-in syndrome (LIS). Brain-computer interface techniques can be useful to disentangle these states by detecting neurophysiological correlates of conscious processing of information to enable communication with these individuals after the diagnosis.
Objective
The goal of our study was to evaluate with a user-centered design approach the usability of a mental imagery task to detect signs of voluntary information processing and enabling communication in a group of severely disabled individuals.
Methods
Five individuals with LIS participated in the study. Participants were instructed to imagine hand, arm or feet movements during electroencephalography (EEG) to detect patterns of event-related synchronization/desynchronization associated with each task. After the user-centered design, usability was evaluated (i.e., efficiency, effectiveness and satisfaction).
Results
Two participants achieved significant levels of accuracy in 2 different tasks. The associated workload and levels of satisfaction perceived by the users were moderate and were mainly related to the time demand of the task.
Conclusion
Results showed lack of effectiveness of the task to detect voluntary brain activity and thus detect consciousness or communicate with non-responsive individuals. The application must be modified to be sufficiently satisfying for the intended end-users and suggestions are made in this regard.
1
Introduction
Detection of consciousness in non-responsive patients (NRPs) due to severe brain injuries remains challenging. Particularly difficult is differentiating individuals with a real disorder of consciousness (DoC), such as coma, vegetative state/unresponsive wakefulness syndrome (VS/UWS) or minimally conscious state (MCS) , from those who preserve full consciousness but cannot express voluntary actions or speak because of lesions in the motor pathways, such as people with locked-in syndrome (LIS). The American Congress of Rehabilitation Medicine defined the LIS as the:
- •
presence of sustained eye opening with persistence of vertical eyelid and eye movements;
- •
preservation of cognitive skills;
- •
severe hoarseness or hypophonia;
- •
quadriplegia or quadriparesis, and;
- •
a primary mode of communication using eye movements or blinking .
Three types of LIS are described on the basis severity of motor deficits:
- •
classical LIS, whereby individuals are unable to move, except for eye movements or blinking, and enable to speak;
- •
incomplete LIS, in which residual voluntary movements in addition to eye movements can be present and;
- •
total LIS, with total immobility, including eye movements .
A variety of LIS cases with multisensory deafferentation have been recently described . Clinical differentiation between these entities may be difficult, for example, between total LIS and coma (eyes closed without any sign of voluntary behavior) and with VS/UWS (eyes open but without evident voluntary responses). Other types such as cognitive-motor dissociation, in which individuals show command-following in mental imagery (MI) tasks evidenced by neuroimaging or neurophysiologic methods without showing behavioral signs of such mental activity, should be also considered . Thus, new diagnostic tools are needed to detect signs of consciousness in the absence of explicit movement or language of the individuals. Besides, once the diagnosis of LIS has been established, we must provide individuals with communication methods independent of muscular control. This can be done through brain-computer interface (BCI) techniques.
A BCI allows for recording brain activity and translating this signal into an output for controlling an application without motor or peripheral nerve pathways . BCI has been found useful for evaluating possible voluntary brain activity in individuals with DoC and instantiated communication with those unable to move or speak due to cerebral or peripheral lesions affecting the motor system. One remarkable example for a BCI used to evaluate the state of consciousness of an NRP was described by Owen and colleagues . By using an MI task registered with functional MRI (fMRI), voluntary brain activity, indistinguishable from that of healthy people, was found in an individual clinically diagnosed with VS. An essay for communication through this paradigm was realized later by Monti et al. . Taking into account some limitations with the use of fMRI (motion sensitivity, contraindication in people with metallic prostheses, high costs), different tasks have been developed to detect voluntary cerebral activity in NRPs by using a low-cost and easy-to-use technique such as EEG .
The task established by Owen et al., which requires imagining playing tennis (motor task) and navigation through one’s own apartment (spatial task), led to 100% accuracy in the recognition of the associated brain activation. Hence, we aimed to transfer this paradigm to EEG. Furthermore, we extended the task by adding a third motor imagery task: the movement (dorsiflexion) of feet intended to evoke the beta-rebound phenomenon. This activity has a high signal-to-noise ratio for identifying feet movement and has been tested in healthy subjects as a possible BCI-based brain switch .
To assess the results of this experiment, we followed the user-centered design (UCD) for BCI applications . This approach establishes 3 aspects to be evaluated for the optimal adaptation of a BCI system to end-users: effectiveness, efficiency and satisfaction with the device. Effectiveness is a measure of how accurate and complete an end-user can accomplish the task. Efficiency relates the invested costs (effort and time) for effectiveness. Satisfaction informs the comfort and acceptability while using the product, here the BCI to estimate the level of consciousness and to communicate. In our clinical context, results provided by BCI-controlled applications must be executable by individuals with severe brain damage (effectiveness) and reliable, because otherwise the costs invested for communication are too high (efficiency) and no meaningful communication is possible. High satisfaction with the device ensures its repeated use by the primary end-users (i.e., patients) for communication and by secondary end-users (i.e., medical and paramedical staff) to evaluate the level of consciousness.
In this paper, we report on the evaluation via the UCD of an EEG-adapted MI task to be potentially used for detecting consciousness and communication with NRPs. We included individuals with LIS because they can still communicate and thus provide us with feedback, and the presence of consciousness is ascertained. Good acceptance of the protocol by participants and easy implementation by the professional staff/caregivers was also assessed with the UCD. Factors that might influence BCI performance such as mood, motivation and quality of life (QoL) were also addressed.
2
Methods
2.1
Participants
Five individuals with LIS recruited with the cooperation of the French Association of Locked-In Syndrome participated. Signed consent was obtained from all participants or their legal representatives. The study was approved by the Ethics Committee of the University of Liège.
To evaluate the system from the user-researcher perspective, 3 BCI scientists at the Cyclotron Research Centre (University of Liège, Belgium) involved in testing individuals (but not in BCI development) were asked to answer 2 questionnaires to evaluate the devices.
2.2
MI tasks
Participants were instructed to perform different MI tasks known to induce distinctive event-related desynchronization (ERD)/event-related synchronization (ERS) patterns. The specific MI task was announced by verbal cues (“sport”, “navigation”, “feet”) via ear-in headphones. For the “sport” task, participants were instructed to imagine performing their favourite sport. For the “navigation” task, they had to imagine navigating through their house. For the “feet” task, participants were instructed to repeatedly attempt feet dorsiflexion. A single task was performed 15 times during a run, for a total of 3 runs (45 trials/task). Random pauses of 4 to 6 sec were included between successive trials ( Fig. 1 ).

2.3
Passive feet condition
To exploit the similarities in brain responses during passive and active movements, which can help to establish an initial classifier , a passive condition was added: manual dorsiflexion of the participant’s feet executed by the examiner in an identical protocol to the MI task. These sequences were followed by the 3 MI tasks.
2.4
EEG recording
In total, 32 active electrodes were used, mounted on a standard EEG cap (EasyCap GmbH, Herrsching, Germany) following the 10–20 international system at positions AFz, F1, Fz, F2, FC3, FC1, FCz, FC2, FC4, C5, C3, C1, Cz, C2, C4, C6, CP3, CP1, CPz, CP2, CP4, P5, P3, P1, Pz, P4, P6, PO3, POz, PO4, Oz; connected to g.USBamp amplifiers (g.tec, Graz Austria); with sampling rate 512 Hz, band pass filters 0.5 to 100 Hz and notch filter 50 Hz.
2.5
Data analysis
Analysis was carried out offline. Data were high-pass – filtered with a cutoff frequency of 1 Hz. Binary Infomax independent component analysis (ICA) was used to separate EEG and EOG signals into independent components (ICs). ICs representing eye movements, eye blinks and muscle activity were identified by visual inspection using methods described in and removed. The remaining components were multiplied by the mixing matrix produced by the ICA algorithm to reconstruct cleaned EEG data.
Fisher’s linear discriminant analysis was used as a classifier based on logarithmic band power features, calculated from a single orthogonal Laplacian derivation and for multiple frequency bands (α: 8–13 Hz; βL: 13–19 Hz; βM: 19–25 Hz; βU: 25–30 Hz). A logarithmic band power feature was calculated by band-pass filtering, squaring and averaging over 1 sec in a sample-by-sample manner. A nested block-wise cross-validation (10 × 10 inner fold; leave-one-out-block outer fold) was applied to calculate the accuracy for each 0.5 sec from t = 0 sec to the end of a trial.
To analyse the percentage of power decrease (ERD) or power increase (ERS) relative to a reference interval (seconds 1 to 2 in the paradigm), a time-frequency map for frequency bands between 6 and 40 Hz was calculated. Logarithmic band power features, calculated by band-pass filtering, squaring and subsequently averaging over the trials were used to assess changes in the frequency domain. To determine the statistical significance of the ERD/ERS values, a t -percentile bootstrap algorithm with significance level of α = 0.01 was applied . The chance level was estimated as described by Müller-Putz et al. taking into account the effect of the number of trials on the classification accuracy.
At the end of the session, participants were asked to answer the questionnaires to evaluate the workload, QoL, mood and motivation. In a second meeting, they were informed about their performance and asked to give their free opinion on the tests. Researchers were asked to answer their questionnaires at the end of the tests with the whole group of participants.
2.6
Workload (efficiency)
The NASA-Task Load Index (NASA-TLX) evaluates the subjective workload experienced by the subject during the performance of a task in 6 domains: mental demand, physical demand, temporal demand, performance, effort and frustration . With a weighted average obtained by a pairwise comparison procedure between the 6 subscales, the workload associated with each aspect of the task can be determined. A simpler procedure can be used to estimate the workload by calculating the raw task load index (RTLX), which is the average of the scores of each domain and has been shown to be equivalent to the weighted scores of the NASA-TLX . We applied both procedures comparing the results and the coefficient of correlation to test the similarity between the obtained scores of each method.
2.7
Satisfaction
The Quebec User Evaluation of Satisfaction with Assistive Technology 2.0 (QUEST 2.0) evaluates the degree of satisfaction with assistive technology (AT) (devices and services), with 12 items scored from 1 (“not satisfied at all”) to 5 (“very satisfied”). We used the extended QUEST to adapt the evaluation to the 2 different end-users (participants and researchers).
2.8
QoL
The Anamnestic Comparative Self-Assessment (ACSA) scale for evaluating QoL takes as endpoints the worst and the best time of the individual’s life, assigning a score of -5 and +5, respectively, to these situations. The individual is asked to compare their current life situation with those situations and to assign a score representing his or her currently experienced QoL.
2.9
Mood and motivation
These were evaluated by a visual analog scale (VAS). We chose these coarse measures over questionnaires for time economic reasons to not overstrain the participants. The VAS for mood and motivation was presented as a continuous line between 2 extreme mood states (extremely bad/good mood) and 2 extreme degrees of motivation (not at all/extremely motivated).
2.10
Statistical analysis
Questionnaire data were analysed with use of Statistica v10 ( www.statsoft.fr ).
3
Results
3.1
Effectiveness
Among our 5 participants with LIS (characteristics in Table 1 ), participant 1 achieved significant levels of accuracy in the passive (71%) and attempted (64%) feet movements at FC2 and C3 Laplacian channels, respectively, in the theta frequency band. Participant 2 showed 64% accuracy at C4 also in the theta frequency during the sport task. Fig. 2 shows the ERD/ERS maps for participants 1 and 2 in the above-mentioned tasks. None of the other participants achieved significant accuracy in any of the tasks, and no beta-rebound was identified in the passive or attempted feet movement.
Participant | Age | Sex | Time with LIS (Years) | Etiology | Residence | Means of communication |
---|---|---|---|---|---|---|
1 | 46 | M | 16 | Brainstem stroke | Institution | Eye blinking/PC |
2 | 33 | M | 12 | Brainstem stroke | Institution | Eye blinking/PC |
3 | 46 | F | 18 | Brainstem stroke | Home | Eye and head movements/PC |
4 | 21 | F | 4 | Brainstem stroke | Institution | Eye movements |
5 | 43 | F | 4 | Brainstem stroke | Institution | Eye movements |

3.2
Efficiency: NASA-TLX
Table 2 lists the raw and weighted scores for each subscale of the NASA-TLX and the simple averages for each participant. We found no significant difference between both scores (Wilcoxon signed-rank test, P = 0.37), and the Spearman coefficient showed a very high correlation ( r = 0.9) between raw and weighted scores, which indicates that both calculations lead to the same results. Temporal demand was considered to contribute most significantly to workload, followed by effort and performance.
Participant | Mental demand | Physical demand | Temporal demand | Performance | Effort | Frustration | Average | |||||||
---|---|---|---|---|---|---|---|---|---|---|---|---|---|---|
RS | WS | RS | WS | RS | WS | RS | WS | RS | WS | RS | WS | RTLX | WS | |
1 | 40 | 10.7 | 50 | 10 | 60 | 8 | 50 | 10 | 50 | 10 | 50 | 0 | 50 | 48.7 |
2 | 40 | 2.6 | 20 | 0 | 40 | 10.7 | 40 | 5.3 | 40 | 13.3 | 20 | 4 | 33.3 | 35.9 |
3 | 1 | 0 | 10 | 0.6 | 50 | 13.3 | 50 | 13.3 | 10 | 1.3 | 50 | 13.3 | 28.3 | 41.9 |
4 | 20 | 6.6 | 30 | 2 | 50 | 10 | 1 | 0.06 | 20 | 2.6 | 20 | 4 | 23.5 | 25.2 |
5 | 5 | 10 | 50 | 13.3 | 20 | 2.6 | 30 | 8 | 80 | 10.6 | 10 | 0 | 40 | 44.5 |
Average | 30 | 5.9 | 32 | 5.2 | 44 | 8.9 | 34.2 | 7.3 | 40 | 7.5 | 30 | 4.2 | 35.02 | 39.3 |
3.3
Satisfaction: extended QUEST 2.0
The average satisfaction level of participants was “quite satisfied” for most of the items except for the dimensions and the adjustments, for which participants were “more or less satisfied” ( Table 3 ). Among the researchers, the highest satisfaction scores were for professional services (“very satisfied”) and safety and effectiveness (“quite satisfied”). When asked to identify the most important items for satisfaction with the device, items rated high by participants were weight and adjustments and by researchers, ease of use and effectiveness.
Participant | Researcher | |||||||||
---|---|---|---|---|---|---|---|---|---|---|
1 | 2 | 3 | 4 | 5 | Average | 1 | 2 | 3 | Average | |
QUEST 2.0 | ||||||||||
Dimensions | 3 | 2 | 3 | 3 | 4 | 3.0 | 1 | 3 | 4 | 2.6 |
Weight | 4 | 4 | 4 | 5 | 5 | 4.4 | 2 | 3 | 4 | 3 |
Adjustment | 2 | 1 | 3 | 5 | 5 | 3.2 | 1 | 4 | 4 | 3 |
Safety | 3 | 5 | 5 | 5 | 5 | 4.6 | 5 | 5 | 4 | 4.6 |
Comfort | 3 | 5 | 5 | 4 | 5 | 4.4 | – | – | – | |
Ease to use | 3 | 4 | 5 | 5 | 5 | 4.4 | 2 | 4 | 5 | 3.6 |
Effectiveness | 3 | 5 | 5 | 5 | 4 | 4.4 | 3 | 4 | 5 | 4 |
Professional services | 3 | – | 5 | 5 | 5 | 4.5 | 5 | 5 | 5 | 5 |
QUEST total score | 3 | 3.7 | 4.3 | 4.6 | 4.8 | 2.7 | 4 | 3.9 | ||
Reliability | – | – | – | – | – | 2 | 5 | 4 | 3.6 | |
Learnability | – | – | – | – | – | 4 | 2 | 5 | 3.6 | |
Speed | – | – | – | – | – | 3 | 3 | 4 | 3.3 | |
Added items total score | – | – | – | – | – | 3 | 3.3 | 4.3 |
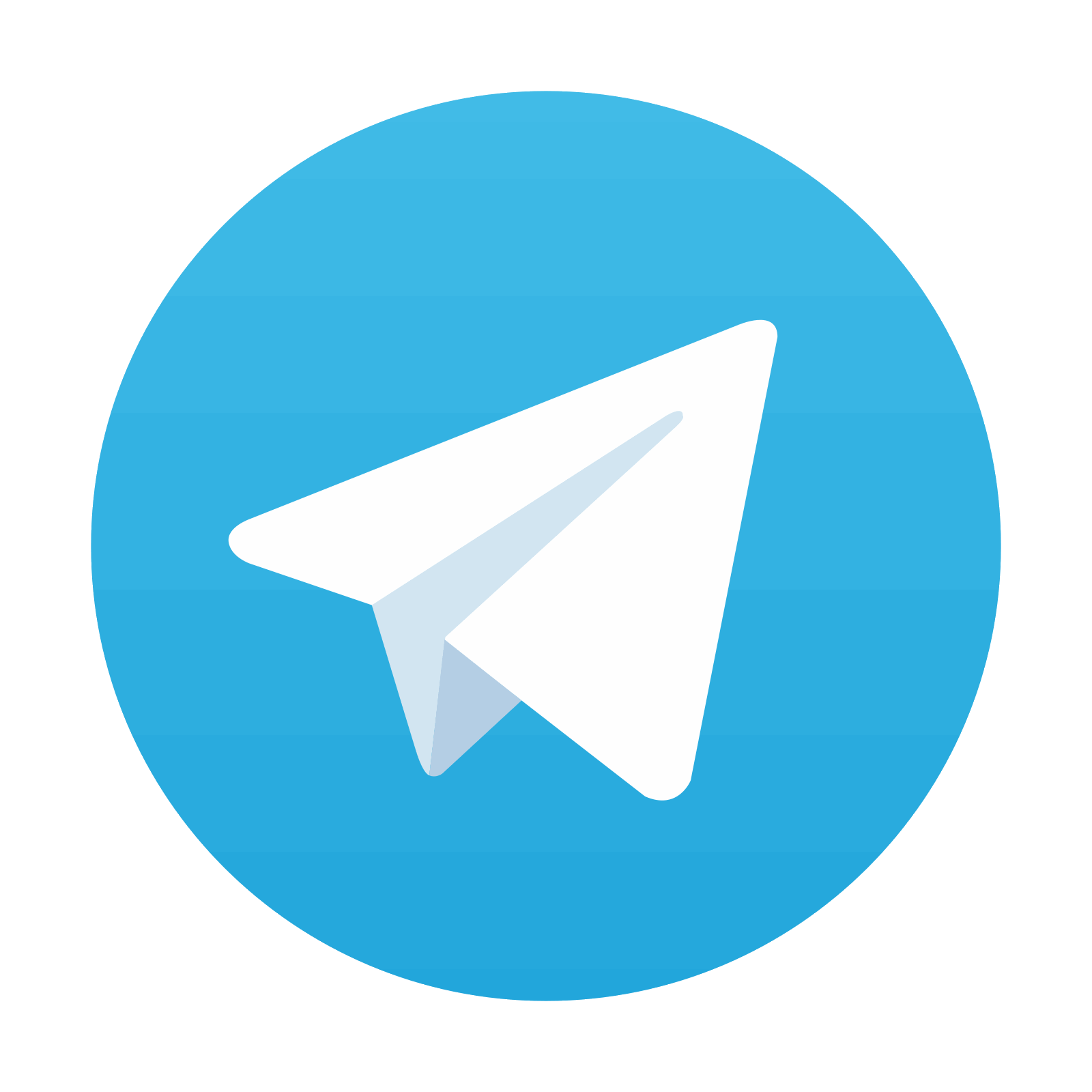
Stay updated, free articles. Join our Telegram channel

Full access? Get Clinical Tree
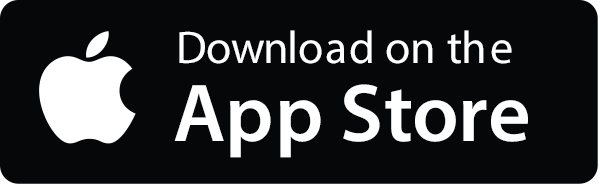

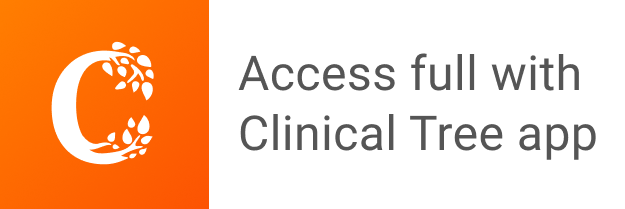