Rheumatic diseases offer distinct challenges to researchers because of heterogeneity in disease phenotypes, low disease incidence, and geographic variation in genetic and environmental factors. Emerging research areas, including epigenetics, metabolomics, and the microbiome, may provide additional links between genetic and environmental risk factors in the pathogenesis of rheumatic disease. This article reviews the methods used to establish genetic and environmental risk factors and studies gene-environment interactions in rheumatic diseases, and provides specific examples of successes and challenges in identifying gene-environment interactions in rheumatoid arthritis, systemic lupus erythematosus, and ankylosing spondylitis. Emerging research strategies and future challenges are discussed.
Key points
- •
Genetic and environmental risk factors have been identified for rheumatic diseases using case-control, cohort, and genome-wide association studies.
- •
The identification of gene-environment interactions (GEIs) may elucidate biological mechanisms for rheumatic diseases by causally linking established genetic and environmental risk factors.
- •
The most well studied example of GEIs in rheumatic disease susceptibility is for cigarette smoking and the HLA-DRB1 for seropositive rheumatoid arthritis (RA); the presence of both risk factors greatly increases the risk for RA development.
- •
Owing to the relative rarity of systemic lupus erythematosus (SLE), comprehensive studies of GEIs have not yet been performed for SLE. However, there is some evidence that genes may interact with smoking and ultraviolet-B radiation exposure in increasing the risk for SLE.
- •
HLA-B27 is the most potent genetic risk factor for ankylosing spondylitis, and there are suggestions that molecular mimicry by gut microbes might stimulate autoimmunity through GEIs with HLA-B27.
- •
Emerging research frontiers such as epigenetics, metabolomics, and the study of the oral, respiratory, and gastrointestinal microbiome may provide new biological mechanisms to link genetic and environmental risk factors in the pathogenesis of rheumatic diseases.
Introduction
The current paradigm for the etiology of autoimmune rheumatic disease is that several preclinical stages precede the onset of clinically apparent disease. When individuals at increased genetic risk are exposed to environmental or lifestyle factors, early alterations in the immune system and the breakdown of self-tolerance ensue, eventually leading to the presentation of overt disease ( Fig. 1 ). Indeed, several genetic and environmental risk factors have been strongly associated with the risk of incident rheumatic diseases, and many more are weakly associated or hypothesized to be related. However, the pathogenesis and biological mechanisms for the development of autoimmune rheumatic diseases remain poorly understood.

Interactions between genetic and environmental factors may elucidate biological mechanisms for rheumatic disease susceptibility and bridge findings in several fields of research. Greater understanding of the etiology of rheumatic disease may provide important insights into prevention, screening, and treatment options. Therefore, researchers in rheumatic disease are motivated to explore the intersection of genetic and environmental risk factors. However, rheumatic diseases present distinct challenges for the identification of gene-environment interactions (GEIs). These challenges include heterogeneous phenotypes, low disease incidence and prevalence, geographic variation in epidemiology, and the difficulty in identifying individuals at elevated risk for disease before clinical diagnosis.
This article serves as an overview to contextualize genetic and environmental risk factors in the development of rheumatic diseases, and highlights future research directions according to study designs and molecular approaches. Specific successes and challenges concerning rheumatoid arthritis (RA), systemic lupus erythematosus (SLE), and ankylosing spondylitis (AS) are addressed.
Introduction
The current paradigm for the etiology of autoimmune rheumatic disease is that several preclinical stages precede the onset of clinically apparent disease. When individuals at increased genetic risk are exposed to environmental or lifestyle factors, early alterations in the immune system and the breakdown of self-tolerance ensue, eventually leading to the presentation of overt disease ( Fig. 1 ). Indeed, several genetic and environmental risk factors have been strongly associated with the risk of incident rheumatic diseases, and many more are weakly associated or hypothesized to be related. However, the pathogenesis and biological mechanisms for the development of autoimmune rheumatic diseases remain poorly understood.

Interactions between genetic and environmental factors may elucidate biological mechanisms for rheumatic disease susceptibility and bridge findings in several fields of research. Greater understanding of the etiology of rheumatic disease may provide important insights into prevention, screening, and treatment options. Therefore, researchers in rheumatic disease are motivated to explore the intersection of genetic and environmental risk factors. However, rheumatic diseases present distinct challenges for the identification of gene-environment interactions (GEIs). These challenges include heterogeneous phenotypes, low disease incidence and prevalence, geographic variation in epidemiology, and the difficulty in identifying individuals at elevated risk for disease before clinical diagnosis.
This article serves as an overview to contextualize genetic and environmental risk factors in the development of rheumatic diseases, and highlights future research directions according to study designs and molecular approaches. Specific successes and challenges concerning rheumatoid arthritis (RA), systemic lupus erythematosus (SLE), and ankylosing spondylitis (AS) are addressed.
Current research strategies for environmental and genetic risk factors
As in other areas of research, bias, confounding, chance, and generalizability are major threats to validity that must be considered in study designs and analyses investigating genetic and environmental risk factors ( Table 1 ). Investigators studying environmental and lifestyle risk factors for rheumatic disease risk have primarily used traditional epidemiologic techniques: the case-control and prospective cohort study designs.
Case-Control Study | Cohort Study | Genome-Wide Association Study | |
---|---|---|---|
Bias | Selection bias Recall bias Inappropriate matching Reverse causation | Selection bias Misclassification Length-time bias Reverse causation Loss to follow-up | Phenotype misclassification Inappropriate controls Genomic inflation |
Confounding | Unmeasured confounders | Unmeasured confounders | Population stratification |
Chance | Multiple testing Power | Multiple testing Power | Multiple testing False discovery rate Rare variants Power |
Generalizability | Study-specific | Cohort-specific | Race/region-specific Phenotype-specific |
Case-Control Studies
Case-control studies identify incident disease cases and match them to controls. Advantages of case-control studies are relative efficiency, especially when cases are rare and difficult to identify prospectively. Disadvantages are inherent biases based on the retrospective nature of these studies: selection and recall bias. In particular, the selection of inappropriate controls may introduce biases that lead to erroneous conclusions. Preclinical disease changes, such as dietary intake, physical activity, or weight changes, might also truly influence factors, introducing the potential for reverse causation bias. Important environmental exposures may occur early in life and may be difficult to assess. In addition, when genes are associated with established disease, it is unclear whether the effect of these genes is for disease etiology or disease propagation. Given these nuances, multiple case-control studies in diverse populations are usually warranted to establish an association between an environmental factor and disease susceptibility. Population-based case-control studies whereby inclusion is compulsory, as in national registries, may overcome some of these challenges.
Cohort Studies
Cohort studies offer solutions to some of the biases inherent in case-control studies. This study design follows populations prospectively based on a common exposure or demographic factor before individuals might develop incident disease. The major advantage of cohort studies is that data are collected without bias for all individuals before disease onset. Design issues such as selection or recall bias are therefore less problematic, although subtle subclinical manifestations might still introduce the potential for reverse causation bias. Inclusion criteria, however, might affect generalizability. Nested case-control studies on stored samples performed within cohort studies can evaluate biomarkers in the preclinical period. Confirmation of incident cases is often a limiting factor in cohort studies, because most rheumatic diseases have a low incidence and require either extremely large cohorts, long follow-up, or specific populations at increased risk, such as first-degree relatives or those with preclinical symptoms (such as arthralgias). Meta-analyses of multiple large cohorts may overcome some of these limitations; however, heterogeneity in study designs and populations may also be limiting. These different cohort designs (general population, unaffected relative, and symptomatic but nonclassifiable individuals) may correspond to particular phases in the development of autoimmune diseases (see Fig. 1 ).
Early studies seeking to identify genetic risk factors often focused on heritability, usually in the context of familial disease. Heritability was estimated by evaluating rates of disease within homozygote and heterozygote twins. Linkage analyses used entire families, with affected and unaffected family members. These methods were able to establish the familial heritability of a disease, although shared environmental factors might still contribute to this heritability. Linkage analyses were most useful in identifying highly penetrant genes with large effect sizes. In addition, candidate gene studies genotyped a few loci at a time, a slow process focusing on known mechanisms of cellular pathways.
Genome-Wide Association Studies
The advent of high-throughput genotyping brought about the era of genome-wide association studies (GWAS), which evaluate initially thousands (and more recently, hundreds of thousands) of different genetic loci at once for association with disease. This hypothesis-free method is capable of evaluating the entire genome for potential disease-susceptibility genes. However, GWAS depend on the performance and composition of specific platforms of single-nucleotide polymorphisms (SNPs) across the genome, which might vary widely according to race, ethnicity, and geography. Potentially associated loci may not necessarily be included on each platform, and therefore would not be tested. The ability of GWAS to detect true associations depends on a homogeneous disease phenotype with similar disease pathogenesis. Appropriate control selection, with similar race and genetic structure, is therefore important. For example, most early GWAS in RA were performed for seropositive RA cases among whites with European heritage. Findings from these studies may not be generalizable to seronegative RA or nonwhite European populations. This concept presents major challenges for diseases such as SLE with heterogeneous subtypes and variation by race, ethnicity, and geography. The genetic components of rheumatic diseases might also be more pronounced in early-onset than in older-onset disease, in which environmental or age-related factors may be more important.
GWAS usually offer only statistical associations with probabilities of disease development. Disease susceptibility loci detected through this method are not necessarily causal for rheumatic diseases, but may be proxies for causal loci because of linkage disequilibrium. GWAS are most successful at discovering common variants, as opposed to rare variants. The latter, however, often have higher effect sizes, and therefore may provide important pathogenic information in comparison with common variants with lower effect sizes. For example, single mutations of genes involved in the complement cascade (C1q, C2, and C4) occur rarely but are very strongly associated with an increased risk for SLE. Methodological issues such as genomic inflation, population stratification, false discovery rates, linkage disequilibria, and multiple comparisons are major design and computational obstacles for GWAS, requiring advanced statistical expertise. Despite these caveats, GWAS have rapidly and efficiently detected numerous loci with rheumatic disease susceptibility. To date, however, findings from GWAS for rheumatic diseases have had little clinical impact, as significant SNPs may not be causal and have low effect size estimates, and thus offer little ability to classify individuals according to risk for disease development.
Genetic Risk Scores
Genetic risk scores (GRS) have been developed to combine many validated genetic loci into a single summary variable, improving statistical power to detect potential associations. GRS can be calculated simply by counts of risk alleles. However, if effect sizes of individual genetic components vary appreciably, weighted GRS (wGRS) should be used. Typically, wGRS are weighted using the natural logarithm of odds ratios found in large GWAS or meta-analyses. Because human leukocyte antigen (HLA) loci are often more strongly associated with the risk of rheumatic disease in comparison with non- HLA SNPs, wGRS have been used in rheumatic disease research.
Gene-environment interactions
The rapid emergence of genetic susceptibility loci and the identification and replication of specific environmental risk factors provide the opportunity to evaluate specific GEIs that may offer new biological mechanisms in disease pathogenesis, and potentially provide personalized medicine approaches whereby an individual’s risk for disease can be calculated using a combination of genetic and environmental factors. However, study design, statistical power, and expertise are major challenges in the identification of potential GEIs. Because GWAS require very large samples sizes to detect associations of genetic factors with disease, often with modest effect sizes, studies with statistical power to detect GEIs may not be feasible using current techniques. Although GEIs may suggest biological mechanisms for disease development, it is not clear that they will be able to provide robust predictive abilities for rheumatic diseases, at least in the foreseeable future.
GEIs can be identified statistically through additive or multiplicative interactions ( Table 2 ). An additive interaction is an effect beyond the sum of the risks associated with individual factors, and can be measured by the relative excess risk due to interaction (RERI), attributable proportion due to interaction (AP), or synergy index (S). A multiplicative interaction is an effect greater than the multiplied effects of the individual factors, and is measured by the ratio of odds ratios (ROR). The interpretation and suitability of interaction terms depend on the scale of the statistical model used. Logistic regression models are on a multiplicative scale, whereas linear regression models are on an additive scale. Therefore, in a logistic regression model (eg, with an outcome of development of disease or not), a statistically significant interaction term implies a multiplicative interaction between the 2 factors. Additive interactions have been considered to represent biological interaction of 2 factors within the same pathway. However, a statistical interaction does not necessarily imply a biological interaction. In studies of disease pathogenesis, a statistically significant additive GEI should be ideally replicated in independent studies and animal models to validate biological plausibility. GEIs usually have statistically significant main effects for both genetic and environmental factors in predicting disease onset, although this may not be a requirement if factors work exclusively in synergy.
Type of Interaction | Statistical Measure | Interpretation | Null Hypothesis | |
---|---|---|---|---|
Additive | RERI | Relative excess risk due to interaction | Additional risk compared with the expected risk from adding the risks for each exposure | RERI = 0 |
AP | Attributable proportion due to interaction | Proportion due to interaction of overall risk among those with both exposures | AP = 0 | |
S | Synergy index | Excess risk from combined exposures relative to the risks from each exposure | S = 1 | |
Multiplicative | ROR | Ratio of odds ratios | Additional risk compared with the expected risk from multiplying risks of each exposure | ROR = 1 |
Recent advancements in fine mapping of genetic regions of interest and high-throughput next-generation sequencing will result in yet more genetic loci associated with the risk of rheumatic diseases, so these issues are timely. Specific examples of genetic factors, environment factors, and GEIs in RA, SLE, and AS susceptibility are provided here.
Rheumatoid Arthritis
The study of genetic and environmental risk factors for RA is the most developed among the rheumatic diseases, owing to a relatively homogeneous disease phenotype and high disease prevalence. Despite these advantages, differences in the risk-factor assessment for RA are appreciated based on serologic status. Early studies of RA risk factors were stratified by the presence of absence of rheumatoid factor (RF). However, RF positivity may be seen in other diseases or as a consequence of aging. Recent studies have used anticitrullinated peptide antibody (ACPA) to classify RA, which is more specific for RA and thus has less potential for misclassification. Despite validated classification criteria for RA, atypical presentations of other systemic rheumatic diseases have the potential to be misclassified as seronegative RA.
Genetic risk factors for rheumatoid arthritis: the shared epitope and beyond
Genetic variants in the major histocompatibility complex (MHC) region on chromosome 6 were identified to be potently associated with RA susceptibility and were deemed the “shared epitope.” Polymorphisms in 3 HLA genes ( HLA-DRB1 , HLA-DPB1 , HLA-B ) in the MHC region are highly associated with RA. Polymorphisms in HLA-DRB1 , in particular, the classic shared epitope genotypes of *04:01 and *04:04 are strongly associated with RA (odds ratios of approximately 4 for risk variants). Specific amino acid positions (11, 71, and 74) that correspond to the HLA-DRB1 shared epitope classic genotypes are located in the peptide-binding groove of the protein HLA-DRβ1, offering a potential biological mechanism for this potent RA genetic risk factor.
Large genetic consortia have identified many non- HLA SNPs associated with RA using GWAS. Most recently, a large transethnic GWAS associated 101 genetic loci with RA across European and Asian populations. However, most of the non- HLA SNPs are only modestly associated with RA. An exception is an SNP near the gene PTPN22 (encoding a tyrosine-phosphatase protein expressed in lymphoid tissue), which is strongly associated with RA (odds ratio 1.78). SNPs near the genes TNFAIP3 and TYK2 also have relatively large effect sizes for RA risk.
Most early genetic studies focused on seropositive RA among Caucasian or Japanese populations. In a recent large genetic consortium study, Han and colleagues made special efforts to correctly classify ACPA-positive and APCA-negative RA cases by using a highly specific ACPA assay. This study identified new genetic risk factors associated with ACPA-negative RA: serine or leucine at amino acid position 11 in HLA-DRB1 and aspartate in position 9 in HLA-B . These findings provide further evidence that ACPA-positive and ACPA-negative RA are genetically distinct, and thus may have separate pathogeneses.
Despite the significance of the HLA shared epitope to RA risk and the growing number of non- HLA SNPs associated with RA, most genetic heritability remains unexplained. The HLA shared epitope explains only 12% of the genetic variance for RA while only about 4% is explained by all other non- HLA SNPs. Gene-gene interactions for RA may also be present, in particular an association between the HLA shared epitope and PTPN22, specifically based on the presence of the R620W allele, for the development of seropositive RA. Studies among familial RA and twins suggest that environmental factors have an important role in the development of RA and RA-related antibodies, so GEIs may be important in linking these discoveries.
Environmental risk factors for rheumatoid arthritis: cigarette smoking and more
Strong epidemiologic evidence supports cigarette smoking as an environmental risk factor for RA development. Multiple studies have shown an increased RA risk from smoking among men and women in various populations. Furthermore, there is a dose-dependent effect between smoking pack-years and RA development. After 20 years of smoking cessation, RA risk of former smokers returns to the RA risk of the general population. Murine models evaluating exposure to cigarette smoke have induced inflammatory arthritis, providing further biological evidence. This association is particularly strong for the development of seropositive RA. Smoking may contribute up to 25% of RA risk, and up to 35% of risk for ACPA-positive RA. However, studies of smoking and seronegative RA have revealed less powerful associations.
Other environmental factors have been associated with the development of RA. It is beyond the scope of this article to review these factors extensively, but they include occupational exposures such as silica, reproductive factors in women, and excess body mass. Dietary factors that might be protective for RA include intake of alcohol and fish.
Given the knowledge of a period of preclinical RA autoimmunity during which autoantibodies are present in the absence of clinically apparent joint inflammation, there is growing interest that the induction of RA may occur at extra-articular sites. The induction of autoimmunity in RA may specifically occur at mucosal surfaces in the respiratory tract, mouth, or gut. Mucosal involvement in the lung has been posited as a possible site for RA initiation because of the increased risk seen in both smoking and particulate silica exposure. Citrullination of peptides occurs in the lung because of inflammation, and this is most pronounced in smokers. Interstitial lung disease is a well-known nonarticular manifestation of RA, and may occur even without articular involvement. Periodontitis has also been associated with both prevalent and incident RA, although smoking and secondary Sjögren syndrome may confound these associations. Porphyromonas gingivalis , a causative bacterium for periodontitis, expresses the enzyme peptidylarginine deiminase, which can citrullinate both enolase and fibrinogen, self-antigens thought to be involved in the joint specificity of RA. A study composed of RA first-degree relatives reported higher concentrations of P gingivalis antibodies in RA relatives who had developed RA-related antibodies, arguing that preclinical infection, or its immune response, may be important in the development of altered immunity in RA.
Rheumatoid arthritis gene-environment interaction: HLA-DRB1 –cigarette smoking interaction
The strong association of smoking with RA risk and evidence for induction of autoimmunity and citrullination in mucosa led to a hypothesized biological mechanism for the role of smoking in the development of RA. Studies initially performed by Klareskog and colleagues demonstrated a strong GEI between cigarette smoking and HLA-DRB1 in determining RA risk. This association was strongest for seropositive RA and has been replicated in several populations, including United States women, and Swedish and Malaysian populations. The presence of heavy smoking and 2 HLA-DRB1 genes increased the odds for RA by 23-fold compared with those with neither risk factor.
Transgenic mouse models have demonstrated a strong immune response between HLA-DRβ1 and citrullinated self-antigens implicated in smoking, offering more evidence of biological plausibility for this GEI. Smoking in particular, but also other inflammatory exposures, are now thought to cause citrullination of a large array of peptides. The specific responses to these citrullinated peptides are likely genetically determined, generating a diversity of ACPAs to different citrullinated antigens, which arise before onset of clinical disease and have varying pathogenicity. The HLA -smoking interaction may contribute to citrullination processes and autoimmunity and might explain the seroconversion noted in preclinical RA ( Fig. 2 ). This GEI seems to apply only to seropositive RA, and possibly in particular to those with antibodies to citrullinated α-enolase and vimentin. Other genes ( GSTT1 and HMOX1 ) in which polymorphisms might interfere with the metabolism of cigarette smoke are hypothesized to also enhance RA susceptibility.

Although HLA -smoking GEIs have led to robust findings about the pathogenesis of ACPA-positive RA among those with both risk factors, they confer little ability to discriminate between RA cases and controls in risk modeling, emphasizing the need for continued research of other biological pathways involved in RA pathogenesis.
Systemic Lupus Erythematosus
Because of its elevated morbidity and mortality, there is interest in identifying risk factors for the development of SLE. SLE has a heterogeneous clinical phenotype, ranging from mild mucocutaneous or musculoskeletal involvement to severe, life-threatening neurologic or renal manifestations. The American College of Rheumatology (ACR) classification criteria for SLE considers these varied manifestations as a single disease entity. This singularity presents methodological challenges, as different SLE subtypes may have separate pathogeneses and, therefore, different genetic and environmental risk factors. This aspect further partitions an already rare disease, decreasing the statistical power of even large studies with long follow-up periods. Despite these challenges, specific autoantibody and biomarker profiles offer insight into defining distinct SLE subtypes.
Genetic risk factors for systemic lupus erythematosus
As in RA and other autoimmune diseases, HLA genes are thought to have a central role in SLE susceptibility. The haplotypes HLA-DRB1*03:01 and *15:01 are strong genetic risk factors for SLE in European populations. GWAS have associated more than 40 genetic loci with SLE susceptibility. Several genes, such as PTPN22 , are implicated in SLE and other autoimmune diseases, RA in particular. However, many other genes are specifically associated with SLE susceptibility.
SLE-associated genetic loci have been categorized according to putative functionality. These categories include DNA degradation and cellular debris, immune complex clearance, toll-like receptors, interferon regulation, nuclear factor κB, and regulation of B cells, T cells, monocytes, and neutrophils. The role of DNA repair genes, in particular ATG5 , TREX1 , and DNASE1 , may be of particular interest given the nearly ubiquitous presence of antinuclear antibodies in SLE. A wGRS for SLE, composed of 22 SNPs including HLA-DRB1 , has been developed and used to calculate GRS of particular SLE subtypes based on validated SNPs for SLE and ACR criteria (such as renal involvement and presence of anti-dsDNA antibodies). This wGRS is associated with earlier-onset SLE, consistent with the notion that increased genetic burden may correspond to earlier disease onset. Gene-gene interactions may also be present in SLE, specifically between CTLA4 , IRF5 , and ITGAM with HLA-DRB1 as well as between PDCD1 and IL21 , among others.
Environmental risk factors for systemic lupus erythematosus
Smoking and exposure to ultraviolet radiation have been implicated as possible environmental factors for SLE susceptibility. Ultraviolet-B radiation is well known to cause SLE exacerbations, and its potential pathogenic mechanism in aberrant apoptosis and the removal of cellular debris make this a key candidate in the etiology of SLE, particularly for cutaneous manifestations. Epstein-Barr virus (EBV) has been suggested to be involved in the pathogenesis of SLE, based on high EBV viral loads in pediatric SLE patients compared with controls, with posited molecular mimicry of EBV antigens and autoantigens targeted by lupus autoantibodies. However, the association between EBV and risk of SLE remains controversial. The female predominance in SLE argues strongly for hormonal and reproductive influences. Oral contraceptives and postmenopausal hormones have both been related to an increased risk for SLE, in particular among women taking higher doses of ethinyl estradiol.
Systemic lupus erythematosus gene-environment interactions
Unlike RA, there is not yet strong evidence associating particular genetic and environmental factors to SLE susceptibility, which makes GEIs for SLE challenging to identify. However, GEIs for both smoking and ultraviolet-B exposure have been reported. In the Carolina Lupus Study, women with null homozygous genotypes for GSTM1 and more than 2 years of occupational sun exposure had 3-fold increased odds for SLE, with a trend toward statistical significance. Other genes involved in DNA repair (such as ATG5 , TREX1 , and DNASE1 ) have not been specifically studied for a GEI with ultraviolet-B light for SLE development. A Japanese case-control study found that women who were smokers and had the slow acetylator N -acetyltransferase-2 ( NAT2) genotype had 6-fold increased odds of SLE, compared with never smokers with the rapid acetylator form of NAT2 . This interaction bore a significant additive interaction, an AP of 50%, suggesting that metabolism of oxidants from cigarette smoke may play a role in SLE pathogenesis. In addition, genes for toll-like receptors (such as IRF5 and TLR7 ) may engage microbes, inappropriately resulting in SLE autoimmunity. Infections with several potential organisms may trigger immune responses that go awry in genetically predisposed individuals and leading to SLE autoimmunity, although particular pathogens and interactions have not yet been identified.
Ankylosing Spondylitis
The incidence and prevalence of AS varies markedly by geography, perhaps owing to the prevalence of HLA-B27 in these populations. The identification of individuals at risk therefore may be comparably easier for AS than for other rheumatic diseases based on geography, HLA-B27 -positivity, or AS relatives. However, AS classification criteria are still evolving, which makes consistent phenotyping challenging. There is considerable clinical overlap between AS and other HLA-B27 –associated diseases, such as reactive arthritis, psoriatic arthritis, and inflammatory bowel disease. Inconsistent phenotyping could hinder the identification of genetic and environmental associations in AS. Because AS patients often present in adolescence or go undiagnosed for many years, identifying exposure windows before disease onset is challenging. Cohort studies used in rheumatic diseases comparing exposed with unexposed subjects are typically not large enough to detect AS and most do not follow children or adolescents, who might go on to develop AS in early adulthood.
Genetic risk factors for ankylosing spondylitis: HLA-B27 dominates
Unlike other rheumatic diseases, AS has long been associated with a gene with very large effect size. HLA-B27 positivity confers an odds ratio of approximately 90 for developing AS compared with HLA-B27 –negative individuals. HLA-B27 is present in about 90% of patients with AS, but only about 5% of HLA-B27 –positive individuals develop AS or another form of spondyloarthritis. This finding illustrates the difficulty in the clinical implementation of genetic testing for rheumatic disease susceptibility. A recent large GWAS associated 31 genetic loci with AS. However, these non- HLA loci are overwhelmed by the influence of HLA-B27 . The overall contribution of HLA-B27 to AS heritability is estimated to be 20%, with about 4% being due to other loci. Other genes implicated in AS risk include IL23R and IL1R2 in addition to the intergenic region at 2p15. These associations offer insight into the role of cytokines interleukin (IL)-17/IL-23 and IL-1 in the pathogenesis of AS.
Several possible explanations might explain the striking association of HLA-B27 with AS development. The arthritogenic peptide hypothesis states that similarity between microbial peptides and HLA-B27–specific CD8-positive lymphocytes could induce autoimmunity. The heavy-chain homodimer hypothesis states that HLA-B27 dimers are resistant to normal degradation and engage natural killer receptors inappropriately, resulting in autoimmunity. The protein misfolding hypothesis, which states that unfolded HLA-B27 accumulates in the endoplasmic reticulum and stimulates the release of proinflammatory cytokines, is the most widely accepted.
Environmental risk factors for ankylosing spondylitis: microbial and gut influences?
Microbes have been postulated to trigger altered immunity in AS through molecular mimicry. Some suggest that this might specifically occur in the gut through the microbiome. The etiologic role of Chlamydia species and enterobacteria in reactive arthritis has been posited to also apply to AS pathogenesis. Transgenic HLA-B27 murine models have also demonstrated that the introduction of bacteria is necessary for the development of spondyloarthropathy. Inflammation in the gut has been consistently observed in spondyloarthritis. Cigarette smoking has also been implicated in AS susceptibility, underscoring its role in multiple inflammatory and autoimmune diseases. Unlike in most other rheumatic diseases, men are more likely than women to develop AS, so male-specific factors such as testosterone may also be involved in the pathogenesis of AS.
Ankylosing spondylitis gene-environment interactions: engaging HLA-B27
Given the large influence of HLA-B27 on AS susceptibility, any GEI will need to have biological plausibility with HLA-B27 functionality. In this sense, finding significant GEIs may be more straightforward in AS than in RA and SLE ( Table 3 ). A proteomic analysis of Chlamydia trachomatis identified peptides that interact with HLA-B27 in mouse models and also stimulate T cells from patients with spondyloarthritis. The role of environmental factors in AS pathogenesis, and the reason why many HLA-B27 –positive individuals never develop AS or other forms of spondyloarthritis, are as yet unsolved.
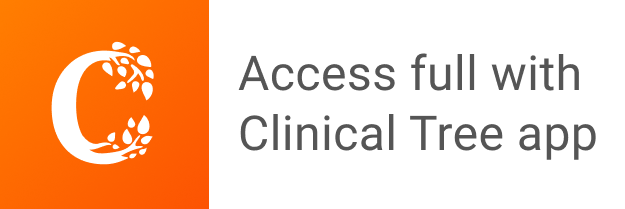