Rheumatic Autoimmune Inflammatory Diseases such as Sjögren’s and lupus lack modern treatments. Less than 5% of drugs approved by the FDA from 2014 to mid-2016 had a RAID indication. Many RAID standard-of-care drugs were repurposed based on serendipitous observations, similarity-of-disease categorization, and/or off-target effects. Recently, drug repurposing has become more intentional, relying on an evolving awareness of molecular underpinnings, as well as a better understanding of drug-target interactions by computational modeling. Understanding mechanisms of disease pathogenesis can be synergistic in identifying new drug candidates and target pathways using unbiased Big-Data repositioning approaches as genomics, PheWAS (disease mechanism-of-action), GWAS and/or epigenetic-profiling.
Key points
- •
RAID such as systemic sclerosis, myositis, Sjögren’s syndrome and systemic lupus erythematosus (SLE) have had few new treatments developed over the past half century.
- •
Of the 82 drugs approved by the FDA from 2014 to mid-2016, only three had a RAID indication ( http://www.centerwatch.com/drug-information/fda-approved-drugs/year/ ).
- •
There are four major approaches to drug repositioning: computational modeling, disease mechanism-of-action based approaches, genetic profiling and translational bioinformatics.
- •
Artificial intelligence cognitive computer systems (AICCS) or human bioinformaticians narrow down drug repositioning candidates using similar tools but the background and experience of human biologists inform the bioinformatic analysis in a way that a machine cannot replicate.
Introduction
There are many and varied approaches to drug repositioning that have been used in the search for effective treatments for patients with a variety of different diseases ( Box 1 ). Methodologies typically use information from one of the following: structural similarity, adverse events, literature mining, clinical trials, gene expression, genome-wide association studies (GWAS), pathways, and/or the interactome. Drug repositioning is an important approach, as it can decrease the time/cost of drug approval because it takes into account issues of toxicity and specificity from early testing and, thereby, paves the way for future discoveries by categorization of drugs by physiologic proxy. Purposeful drug repositioning is often called retooling, reprofiling, retasking, and even drug rescue.
The field of drug repositioning has emerged over the past decade, driven by collaborations among structural scientists, physicians, medicinal chemists, animal model experts, geneticists, computational modelers, immunologists, artificial intelligence/machine learning experts, and bioinformaticians. There are 4 major approaches to drug repositioning that have been used over the years: computational modeling, disease mechanism of action–based approaches, genetic profiling, and translational bioinformatics. Some approaches, such as computational modeling, are most appropriate for small molecules, whereas other approaches can be used to reposition either biologics or small molecules. The focus here is on techniques that stem from genomic information gathered from patients with rheumatic autoimmune inflammatory disease (RAID) and compared with healthy individuals. Small molecules that bind protein products of differentially expressed genes (DEGs) identified following microarray or RNA-Seq can be predicted by computational modeling. Patterns of DEGs in a particular disease can be compared with those in a variety of databases composed of cells cultured in vitro with small molecules or with small hairpin RNAs (shRNAs) to identify either small molecules or biologics that may be effectively repositioned. Translational approaches use information from GWAS as well as phenome-wide association studies (PheWAS) to narrow down drug repositioning candidates. Machine learning can be a useful tool to assist in all approaches to drug repositioning, as the size and scope of the targets to potential drug repositioning candidates is not an issue.
Introduction
There are many and varied approaches to drug repositioning that have been used in the search for effective treatments for patients with a variety of different diseases ( Box 1 ). Methodologies typically use information from one of the following: structural similarity, adverse events, literature mining, clinical trials, gene expression, genome-wide association studies (GWAS), pathways, and/or the interactome. Drug repositioning is an important approach, as it can decrease the time/cost of drug approval because it takes into account issues of toxicity and specificity from early testing and, thereby, paves the way for future discoveries by categorization of drugs by physiologic proxy. Purposeful drug repositioning is often called retooling, reprofiling, retasking, and even drug rescue.
The field of drug repositioning has emerged over the past decade, driven by collaborations among structural scientists, physicians, medicinal chemists, animal model experts, geneticists, computational modelers, immunologists, artificial intelligence/machine learning experts, and bioinformaticians. There are 4 major approaches to drug repositioning that have been used over the years: computational modeling, disease mechanism of action–based approaches, genetic profiling, and translational bioinformatics. Some approaches, such as computational modeling, are most appropriate for small molecules, whereas other approaches can be used to reposition either biologics or small molecules. The focus here is on techniques that stem from genomic information gathered from patients with rheumatic autoimmune inflammatory disease (RAID) and compared with healthy individuals. Small molecules that bind protein products of differentially expressed genes (DEGs) identified following microarray or RNA-Seq can be predicted by computational modeling. Patterns of DEGs in a particular disease can be compared with those in a variety of databases composed of cells cultured in vitro with small molecules or with small hairpin RNAs (shRNAs) to identify either small molecules or biologics that may be effectively repositioned. Translational approaches use information from GWAS as well as phenome-wide association studies (PheWAS) to narrow down drug repositioning candidates. Machine learning can be a useful tool to assist in all approaches to drug repositioning, as the size and scope of the targets to potential drug repositioning candidates is not an issue.
Predicting drugs that bind protein products of genes abnormally expressed in disease
Historically, there are a variety of approaches to predict drugs that bind and potentially interfere with the action of a target protein. Molecular docking approaches to drug repositioning use structural information related to drug:target interactions to predict additional novel targets for known drugs and can be useful when the specific target of interest is known. An example is PharmMAPPER ( http://59.78.96.61/pharmmapper/help.php ), which uses a database of solved crystal structures consisting of 7302 compounds that bind 2241 human proteins (generated from TargetBank, DrugBank, BindingDB, PDTD). This platform supports in silico virtual screening of potential drug repositioning targets for a given protein thought to be involved in disease pathogenesis using a reverse pharmacophore matching method, a type of machine learning. Computational modeling approaches to drug repositioning are data driven and can explore multisystem targets based on activation site analysis and target validation (reviewed in Ref. ). An example is the BioGPS/FLAPdock system using a QSAR (Quantitative Structure-Activity Relationship) modeling and structural algorithm that clusters drugs into similar groups and then looks for target matches based on disease mechanism . An obstacle in using computational modeling for drug repositioning in RAIDs is the lack of a singular agreed on mechanism or pathway for disease pathogenesis; computational modeling has been most successful for diseases such as cancer because of an abnormality in known oncogene products usually within a single cell type. These approaches do not incorporate genomic information into their predictions unlike the 2 algorithms highlighted in the next paragraph: CANDO (Computational Analysis of Novel Drug Opportunities) and SDTNBI (Substructure-Drug-Target Network-Based Inference).
CANDO goes a step further than these approaches by identifying all probable drugs that may interact with protein products of DEGs identified by microarray or RNA-Seq analyses. The initial step is to reveal protein-protein interactions and potential drug-protein interactions using the interactome ( http://interactome.dfci.harvard.edu/H_sapiens/ ) and STITCH (Search Tool for Interactions of Chemicals; ( http://stitch.embl.de/ ). CANDO incorporates these results to predict and then rank the more than 180 million drug-target interactions possible between the 3733 drugs approved by the Food and Drug Administration (FDA) and 48,278 proteins in its database ( http://ram.org/compbio/protinfo/cando/ ). Specifically, CANDO uses a fragment-based (48,278 structures of protein fragments) docking simulation of atomic interactions to determine binding affinities and thus potential inhibitory capacity of drug candidates for a particular protein (or set of proteins). Matrices (ie, heatmaps) are created to visualize putative interactions of proteins and compounds with pseudocolored confidence scores and clustering of drug-protein interaction predictions such as “on-target” (high-affinity agonist), “off-target” (low-affinity agonist) or “antitarget” (antagonist). Using retrospective identification of known drug-target interactions as the gold standard, machine learning accuracy of CANDO is approximately 50% for prediction of the top 10 protein candidates for a given drug.
Cross-referencing CANDO’s results to 1439 disease indications with more than 2 FDA-approved compounds revealed some drug-target interactions relevant to RAID, including everolimus/Afinitor, riboflavin, digoxin, and imatinib. Similar to sirolimus, everolimus (a derivative of sirolimus), was predicted to be a polypharmaceutical with more than 150 protein targets. When CANDO cross-referenced everolimus to clinical indications, kidney diseases were identified confirming lupus nephritis as a possible off-label use of this drug. Imatinib also was defined as a polypharmaceutical by CANDO with 46 protein targets. Glomerulonephritis and proteinuria were cross-referenced by CANDO with imatinib. This result confirms earlier reports suggesting that both sirolimus and imatinib are viable candidates for repositioning into lupus because they received drug repositioning Combined Lupus Treatment Scoring (CoLT) scores similar to or greater than lupus standard-of-care (SOC) drugs.
By contrast, riboflavin/vitamin B2 and digoxin were predicted to have very few protein targets and to be effective in skin diseases and autoimmunity, respectively. An ongoing clinical trial is examining the effect of riboflavin/vitamin B2 on moderate to severe plaque psoriasis ( NCT02622386 ; https://clinicaltrials.gov/ct2/show/NCT02622386 ). Interestingly, peptides from riboflavin presented in the context of nonclassical major histocompatibility complex class I–related molecules have been shown to interact with the T-cell receptor of interleukin (IL)-17A–secreting innate T cells (CD8 + CD161 + RORγt + ) called mucosal-associated invariant T cells, whose peripheral presence has been shown to correlate with disease activity in psoriasis.
Another drug CANDO predicted to have very few protein targets is digoxin. Cross-referencing to clinical indications revealed digoxin’s applicability for autoimmune disease. Digoxin binds the orphan nuclear receptor RORγt and inhibits transcription of IL-17A, the hallmark cytokine of Th17 T cells that play a role in autoimmune diseases, such as systemic lupus erythematosus (SLE) and psoriasis. Traditionally, digoxin has been used for congestive heart failure. CANDO’s highlighting digoxin brings up an interesting question: is the standard use of digoxin that was discovered by serendipity an off-target effect and is the on-target effect inhibition of RORγt and IL-17A production? These questions will become more common as bioinformatics approaches elicit novel drug-protein interactions pairs.
SDTNBI ( http://lmmd.ecust.edu.cn/methods/bsdtnbi/ ) has the ability to predict novel drug-target interactions based on known substructures of drug-protein families and connect them to known networks of associations between drugs-gene products, drugs-diseases, and diseases-genes. SDTNBI looks for novel drug-target interactions with potential binding based on chemical substructure. For SDTNBI, substructures must be in SMILE format (Simplified Molecular-Input Line-Entry) and candidate compounds must have the following attributes of optimal drugs: molecular weight (≤500 Da), log p (≤5) for optimal solubility in water and fat, number hydrogen bond donors (HBD≤5), number HB acceptors (HBA≤10), and a K i /K D /IC 50 /EC 50 ≤10 μM.
Using SDTNBI, 6568 compounds with resolved structures from DrugBank were compared to test proteins to detect known interactions and predict novel interactions. DGIdb (Drug-Gene Interactions database; http://dgidb.genome.wustl.edu/ ) and DRAR-CPI (Drug Repositioning and Adverse Reaction–Chemical Protein Interactions; https://cpi.bio-x.cn/drar/ ) were used to predict drug-gene product interactions; the Comparative Toxicogenomics databases ( http://ctdbase.org/ ) were used to predict drug-disease associations; and the DisGeNet was used to predict disease-gene ( http://www.disgenet.org/web/DisGeNET/menu ) associations. As a test relevant to RAID, proteins that bound celecoxib/Celebrex were queried by SDTNBI and new protein interactions were predicted. SDTNBI correctly predicted association of celecoxib, a coxib used as an anti-inflammatory/analgesic in many conditions with PTGS2 (cyclooxygenase 2) but also with the products of 5 other genes (MB/myoglobin, carbonic anhydrase family members-3/13/7 as well as the G-protein–coupled ADRA2B/adrenoreceptor α2b). Interaction between celecoxib and the ADRA2B gene product may contribute to the adverse events of water retention and salt sensitivity.
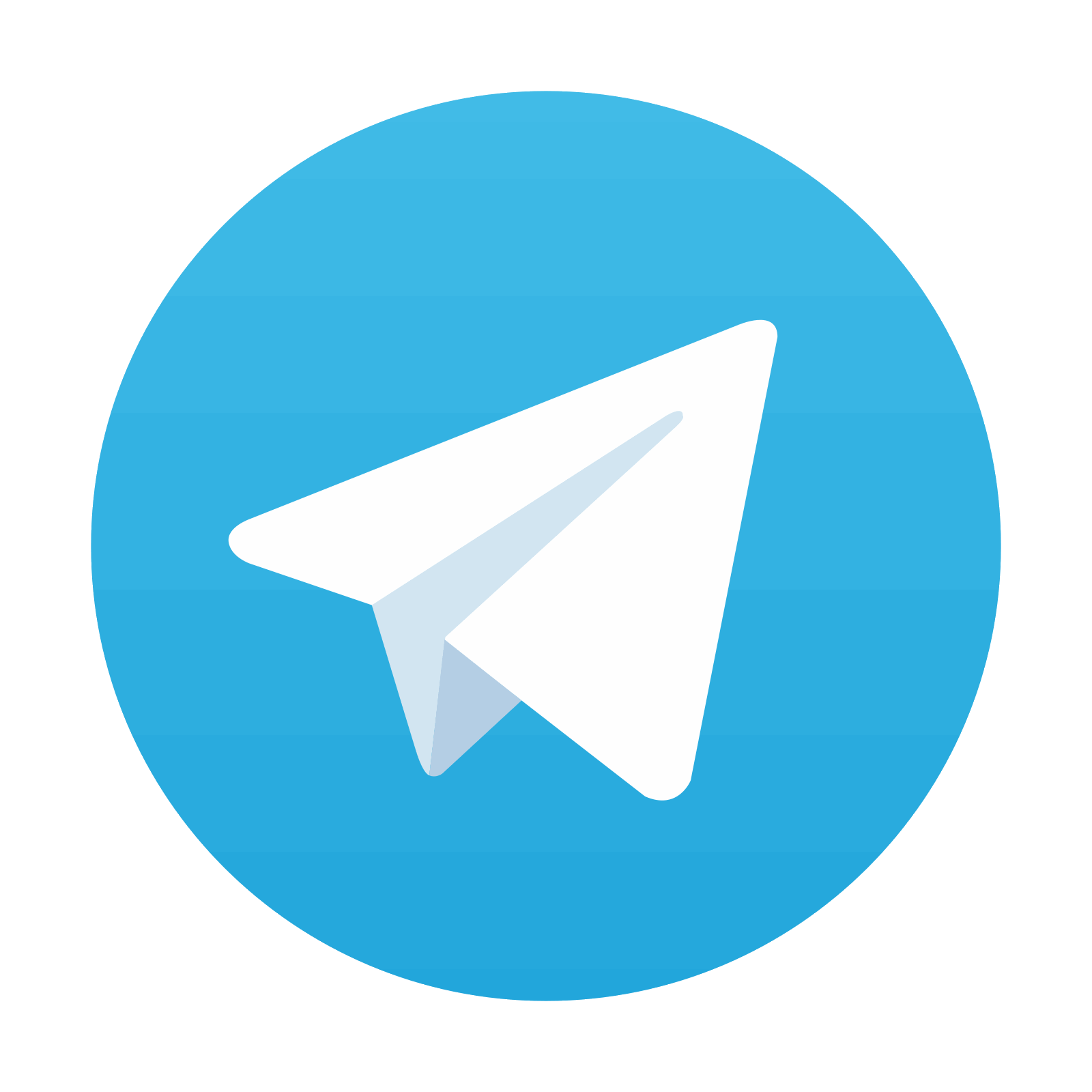
Stay updated, free articles. Join our Telegram channel

Full access? Get Clinical Tree
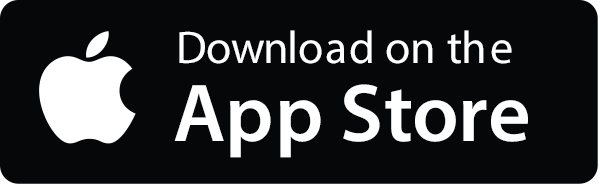
