Fig. 5.1
Fast patient-specific modeling using statistical shape modeling techniques. A Reference skull is morphed to match the patient’s anatomy, as imaged via CT or CBCT, following population-level statistics and anatomical landmarks. A displacement vector field (DVF) is then obtained allowing propagation of other type of information. As exemplified in the lower part of the figure, facial muscle information can then be effectively estimated
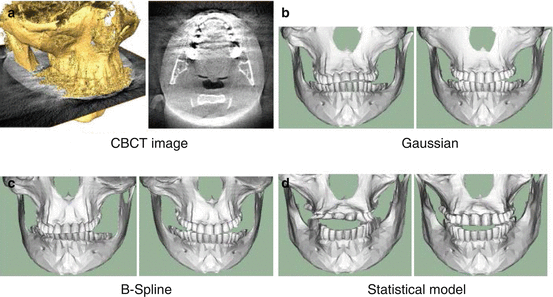
Fig. 5.2
Registration of skulls from CBCT data: (a) shows a slice through the image and a reconstruction of the surface obtained using threshold segmentation. (b–d) show registration results obtained using different deformation models. The left images show a normal registration, while in the right images a spatially-varying registration has been used, showing the ability of the method to deal with metal artefacts
Seamless Surgical Planning: The Direct and Inverse Surgical Planning
Despite of the complexity of the surgical scenario, the available technologies must ultimately serve as a vehicle for the surgeon to plan the surgical plan in a seamless manner. It is thus crucial to develop technologies that leverage the work of the surgeon, allowing him to test different options and interact with the bone and soft tissue components of the surgical plan.
Recently, a shift paradigm was presented whereby the necessary planning is computed from the desired post-operative outcome [75]. This paradigm shift, coined “Inverse Planning”, enables the surgeon to look at the surgical plan from a different perspective, allowing him to directly define the desired outcome, without the need of the commonly used trial-and-error scheme available in current solutions.
Inverse Soft Tissue Modeling
The proposed approach employs a fast biomechanical model to derive from the desired facial outlook the necessary surgical plan. Based on the desired facial outlook the deformation of internal soft tissues is calculated, followed by constrained surface registration between bone segments and internal soft tissues. The proposed registration component considers collision and occlusion constraints, and its formulation allows us to derive in a straightforward manner different levels of interplay between quality of occlusion and compliance to the desired outlook (i.e. constraints relaxation). Furthermore, and in regards to a biomechanical simulation that would model the entire ensemble of bone and soft tissues, the proposed approach avoids known issues of layer detachment and convergence related to the high elasticity transition present at the interface of bone and soft tissue materials. We remark that this approach differs from the classical inverse modeling proposed in computational mechanics and used in implant shape design in [28], as our method deals with the ill-posedness of the problem by considering occlusion and geometrical constraints through a registration component that effectively penalizes the set of numerical solutions.
By combining the direct (i.e. soft tissue simulation from bone displacements) and the inverse soft tissue modeling (i.e. specification of bone displacement to yield a desired outcome) it is possible to yield an effective system that, in a transparent way, enables the surgeon to work on the surgical plan.
Due to airways and tongue volume constraints in complex CMF cases, large rotational and translational planning are rarely operated in one single step and surgeons typically divide it into a series of surgeries, which in turns translates into small deformations in engineering mechanics. Nonetheless, to cover these rare cases for large deformation problems, we will consider modifying the classical FEM inverse approach [77] in which the inverse modelling is transferred to a direct problem by super-imposing boundary conditions and transferring the unknown set of displacements to the other side of the continuity equation [78].
Preliminary results, shown on Fig. 5.3, on a set of clinical cases showed in five out of six CT cases a high level of agreement to the actual surgical plan. In one case the proposed approach was confirmed to improve the actual executed plan. As an additional evaluation, simulated soft-tissue outcomes were compared using the predicted and real clinical plan, resulting in a close agreement between the facial simulation results using the predicted and actual planed approach (Fig. 5.4.).
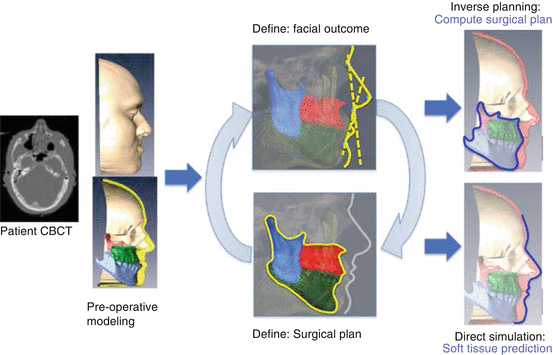
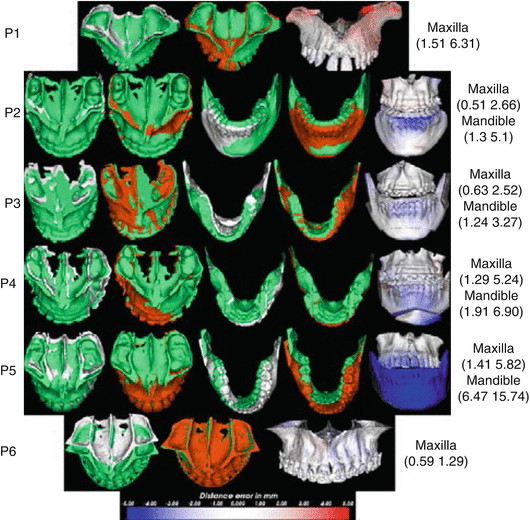
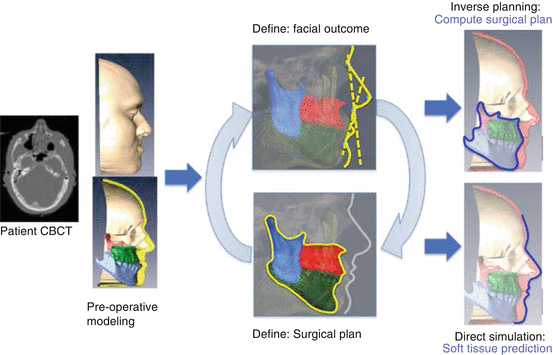
Fig. 5.3
Direct-inverse planning approach. From the pre-operative CBCT scan, a detailed patient-specific model will be created. The surgeon then has the option to interact with the bone segments and perform a direct simulation for soft tissue prediction (lower part of the figure), or define the desired facial outcome and obtain the required surgical plan, subject to functional considerations (upper part of figure), and assisted by cephalometric guides (illustrated with dashed lines). A fast simulation enables the surgeon to seamlessly interact in one mode or the other
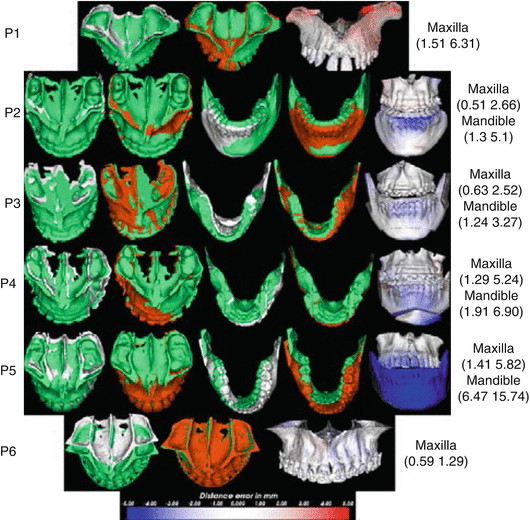
Fig. 5.4
Predicted (orange color), actual post-operative (green color), and pre-operative segments (white color) for the respective patients. The distance errors from the post-operative segments are shown as color-coded images on the predicted model on the rightmost column with the (median maximum) error values in mm indicated for the respective segments. The blue color means the proposed approach falls posteriorly than the real post-operative segment (Adapted from Luthi et al. [74])
Discussion and Conclusions
The human face is a fundamental part of our identity. It centralizes the senses of vision, hearing, taste and smelling, and provides us with channels to participate and integrate in society. The complexity of the clinical scenario is complex, as it requires high understanding of the balance amongst aesthetic, functional, psychological and sociological implications of the surgical outcome. Furthermore, the degree of unpredictability on the surgical outcome makes the decision-making process, on a patient-basis, highly complex. This has called for the development of computational means to assist the surgeon on the task of planning the surgical approach. We believe, however, that more research efforts are essential and needed in order to bring these tools to a level where they can effectively and jointly consider aesthetic and functional aspects for the planning of CMF surgeries.
Based on the observations from the state of the art it can be concluded that functional aspects are of importance and need to be considered in CMF planning. However, there is need to foster the interdisciplinary research with the development of novel approaches that concurrently make use of functional and aesthetic information, and are developed in light of the clinical requirements and workflow. In this regard, it is necessary to enhance these enabling-technologies by developing advanced segmentation algorithms for CBCT imaging as well as algorithms allowing the surgeon to seamlessly interact with the surgical plan or the desired soft tissue outcome, all while jointly considering the functional and aesthetics aspects mentioned above.
References
1.
2.
3.
4.
5.
6.
Gateno J, Xia JJ, Teichgraeber JF. New 3-dimensional cephalometric analysis for orthognathic surgery. J Oral Maxillofac Surg. 2011;69(3):606–22.PubMedCentralCrossRefPubMed
7.
8.
9.
10.
11.
Majeed T, Fundana K, Lüthi M, Beinemann J, Cattin P. A shape prior-based MRF model for 3D masseter muscle segmentation. In: SPIE image processing. San Diego: SPIE Digital Library; 2012. p. 83140O–83140O-7.
12.
Majeed T, Fundana K, Luthi M, Kiriyanthan S, Beinemann J, Cattin PC. Using a flexibility constrained 3D statistical shape model for robust MRF-based segmentation. In: Mathematical methods in biomedical image analysis (MMBIA), 2012 IEEE workshop on, Breckenridge. 2012. p. 57–64.
13.
Majeed T, Fundana K, Kiriyanthan S, Beinemann J, Cattin P. Graph cut segmentation using a constrained statistical model with non-linear and sparse shape optimization. In: Medical computer vision. Recognition techniques and applications in medical imaging. Berlin/Heidelberg: Springer; 2013. p. 48–58.
14.
Boykov YY. Jolly M-P. Interactive graph cuts for optimal boundary & region segmentation of objects in ND images. In: Computer vision, 2001. ICCV 2001. Proceedings. Eighth IEEE international conference on. 2001, vol. 1. p. 105–12.
15.
Rezaeitabar Y, Ulusoy I. Automatic 3D segmentation of individual facial muscles using unlabeled prior information. Int J Comput Assist Radiol Surg. 2012;7(1):35–41.CrossRefPubMed
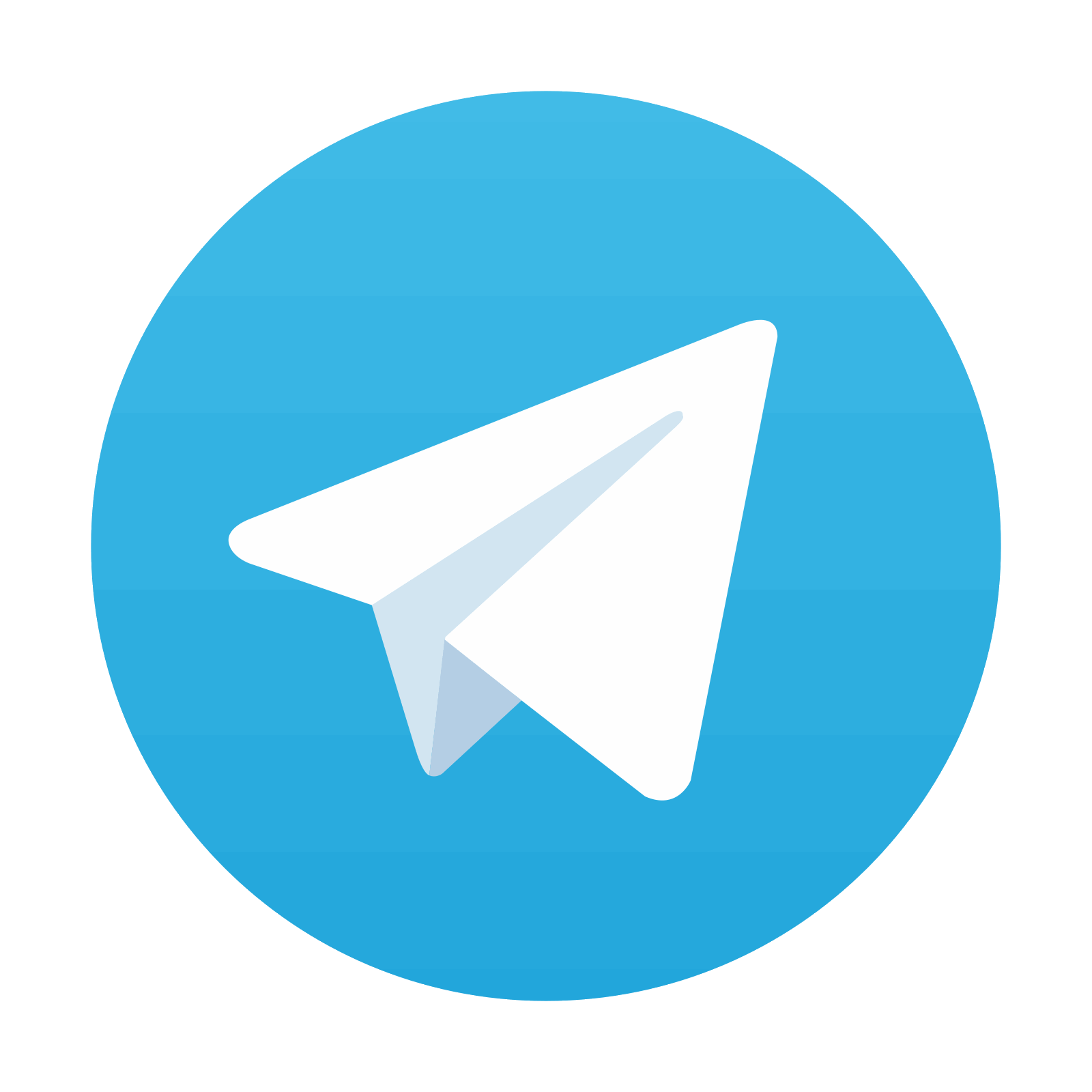
Stay updated, free articles. Join our Telegram channel

Full access? Get Clinical Tree
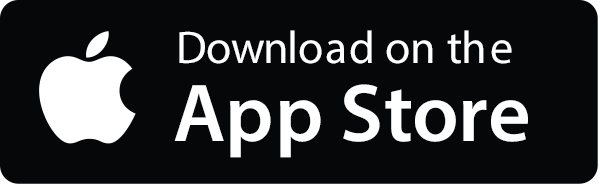
