Fig. 4.1
Overview of a wireless body area network system for remote healthcare monitoring. On-body sensors monitor physiological parameters (ECG, SpO2) and motion and postural data. The collected data is transmitted to a PDA and/or transmitted through GPRS/Bluetooth to a medical server, physician or caregiver for further intervention [28]
The sensor nodes may contain accelerometers, gyroscopes, magnetometers and bio-amplifiers and can be attached to the body and used for recording kinematic data. Additionally, sensors for monitoring vital physiological parameters, such as electrocardiography (ECG) and electromyography (EMG), may also be included. Recent advances in material science have led to the development of e-textile-based systems which integrate sensing capability into garments. The sensors embedded on the garments can be used for collecting ECG and EMG signals, by weaving the electrodes into the fabric, and also used for gathering kinematic data by printing elastometer-based substances on the garments and sensing changes in their electrical characteristics (resistance or capacitance) associated with stretching of the garments when movements are performed by the wearer [24, 28]. Some remote health monitoring systems have also combined wearable sensors and ambient sensors (sensors and motion detectors on doors, objects of daily use such as RFID tags) with an aim of developing smart homes that provide intelligent systems for health assistance in the subject’s living environment, also referred to as ambient assisted living (AAL) [29, 30]. In this context, information collected by body-worn sensors can be augmented by the data from ambient sensors, such as motion sensors, distributed throughout the home environment to determine the pattern of activities performed by the patient and provide feedback concerning exercises and living behaviours for better health management.
4.2 Physiological Monitoring
Research into remote health monitoring over recent years has led to the development of many research prototypes [31–34], commercially available systems [35–41] and smartphone-based systems [42–46]. These developments have enabled long-term physiological monitoring of various clinically important parameters including heart rate, blood pressure, oxygen saturation, respiratory rate, body temperature and galvanic skin response, thereby improving the diagnosis and treatment of life-threatening incidents involved in cardiovascular or neurodegenerative disorders [47].
A medical sensor platform, Code-Blue, was developed at Harvard University which could monitor multiple patients and consisted of custom-designed biosensor boards for pulse oximetry, three-lead ECG, EMG and motion activity [48]. It also caters to the transmission of data from the sensors to multiple receivers which include PDAs with the clinical staff. Live-net is another system developed by the MIT Media Laboratory for detecting Parkinson-like symptoms and epileptic seizures by using sensors to measure three-dimensional acceleration, ECG, EMG and galvanic skin conductance [35].
As part of the research initiatives undertaken by the European Commission over the years, some of the projects worth mentioning in the field of remote health monitoring employing a wearable sensor platform are: MyHeart [49], WEALTHY [50] and MagIC [51]. These projects mainly led to the development of garment-based wearable sensors for general health monitoring of people within the home and community settings. One such project was AMON that led to the development of a wrist-worn device capable of monitoring blood pressure, skin temperature, blood oxygen saturation and ECG [52].
A custom-built u-healthcare system consisting of ECG and blood pressure sensors as well as a cell phone for signal feature extraction, communication and display was developed in [53]. Only abnormal or alarming ECG and blood pressure (BP) patterns are transmitted to the hospital server, rather than transmitting all collected data, to save power. A wrist-worn sensor was used to record blood pressure readings and transmitted to the hospital server if found to be out of range.
A BAN including sensors to measure ECG, PPG and PCG was developed in [54]. Another embedded sensor system named Bi-Fi was developed for wireless bio-signal recording, incorporating ECG, EEG and SpO2 sensors which performed on-board signal processing to cut-off transmission power [55]. A system named BASUMA [56] was developed with custom non-invasive sensors for monitoring parameters such as ECG, air and blood content of a thorax, body temperature, breath rate and cough control, blood pressure, pulse rate and oxygen saturation.
A research project in the Netherlands (Human++) developed a BAN consisting of three sensor nodes for acquiring, amplifying, filtering, processing and wirelessly transmitting multi-channel signals of ECG, EEG and EMG [57]. An autonomous pulse oximeter was developed in Belgium research centre IMEC that transfers the body heat of the wearer into electrical energy storing it in a super capacitor for short-time storage, replacing batteries [58].
There have been some designs which have targeted novel sensor locations like the one mentioned in [59], where a sensor worn as a ring measures blood oxygen saturation (SpO2) and heart rate. The ring sensor has techniques integrated within for motion artefact separation to improve measurement accuracy. This system was used for diagnosing hypertension and congestive heart failure.
A microphone was used for measuring respiratory rate by placing it on the neck to capture acoustic signals associated with breathing. The signals obtained were band-pass filtered to remove noise and artefacts and used for detection of obstructive sleep apnea (OSA) [60]. A low-power flexible, ear-worn PPG sensor was developed for heart rate monitoring which was suited for long-term monitoring owing to its unobtrusive design [61]. However, the system suffered from motion artefacts which adulterated the sensor signals.
Chemical sensors have also been used in wearable systems to detect and monitor the presence of harmful compounds and alert people working in hazardous environments. A non-invasive wearable closed-loop quasi-continuous drug infusion system was developed by researchers for measuring blood glucose levels and infuses insulin automatically [62].
4.2.1 Mobile Phone-Based Physiological Monitoring
The use of mobile phones has also been very popular in recent years, where the inbuilt inertial sensors have been used for collecting data. With the advent of smartphones having Internet connectivity and expandable low-cost memory, they have also been used as a base station with which other sensors communicate and also used as a display unit. We will review a few phone-based wearable health monitoring systems.
A prototype system developed by Microsoft (HealthGear) consists of a non-invasive blood oximeter, a sensor assembly to measure oxygen saturation and heart rate signals, Bluetooth-enabled wireless transmission and a cell phone for user interface. This has been used for detecting mild and severe OSA. The system also had satisfactory feedback from the users in terms of wearability and functionality [47].
A cell phone-based real-time wireless ECG monitoring system (HeartToGo) was developed for the detection of cardiovascular abnormalities [46]. A mobile care system developed in [44] utilizes a Bluetooth-enabled blood pressure monitor and ECG sensor. A mobile phone was used as the processing core with an alert mechanism generated depending on the detection of an emergency situation. Finally, an application for detecting arrhythmia events has been described in [43] which used a handheld device as a PDA. The PDA serves as the smart device for processing and analysing the continuously transmitted ECG signals. It also communicates remotely with a clinician, transmitting alarm signals and minute long raw ECG samples.
A wearable system for stress monitoring depending on the emotional state of an individual (AUBADE) has been developed by researchers [63]. It consists of a 3-lead ECG sensor and a respiration rate sensor strapped to the chest, a 16-lead EMG sensor embedded in textile and a galvanic skin response sensor attached within a glove. It employs an intelligent emotion recognition module with a three-dimensional facial representation mechanism to provide feedback.
4.2.2 Commercially Available Systems
A range of wearable health monitoring systems are already commercially available, such as the wrist-worn device called Vivago WristCare for monitoring skin temperature, skin conductivity and movement [42]. A similar device known as SenseWear Armband monitors ambient temperature and heat flow [41]. Both communicate collected data to a base station and report any alarming situations for further evaluation by responsible clinicians. A washable lightweight vest including respiratory rate sensors, one-lead ECG for heart rate measurements and an accelerometer for activity monitoring was developed by VivaMetrics and known as LifeShirt [38]. Another garment-based physiological monitoring tool for monitoring heart rate, respiration rate, posture, activity, skin temperature and GPS location was developed in [37], known as Watchdog. Sensatex also came up with a smart T-shirt-based wearable system for measuring ECG, respiration rate and blood pressure working on conductive fibre sensors [64]. A mobile cardiac outpatient telemetry (MCOT) system was developed by CardioNet for measuring ambulatory ECG aimed at treating patients with arrhythmia [36].
Manufacturers like Philips [65], Nellcor [66], Agilent [67] and Nonin [68] are providing low-cost, lightweight fingertip pulse oximeters providing real-time display of heart rate and blood oxygen saturation. Chest-worn belts and wristwatch for displaying heart rate measurements have been developed by Polar [69] and Omron [70].
4.2.3 Safety Monitoring Systems
A number of devices designed for safety monitoring are already commercially available. Simple systems such as Life Alert Classic [71] and AlertOnce [72] provide the basic facility of manual alarm activation through the use of a push button contained in a wristwatch. When activated by the user, an alarm message is transmitted to the nearest remote care facility, and the appropriate emergency response is initiated. By comparison, the Wellcore system is more sophisticated and uses accelerometers and advanced microprocessors to monitor the body position and is able to detect falls and generate an alarm message in times of emergency that is relayed to the nearest response centre [73]. Another such device in the form of a chest strap is MyHalo [74], which can be used for detecting falls, monitoring heart rate, skin temperature, sleep/wake patterns and activity levels. The BrickHouse system also employs an automatic fall detector and an alarm generation facility [75].
Reliable fall detection using wearable sensors has also been a well-researched topic, and there exists various research prototypes. An automatic fall detection and alarm generation device was developed by researchers at CSEM [76]. They achieved high recognition precision with simulated falling situations with a wrist-worn sensor, which was easy to put on. There have been other alternative approaches where researchers have embedded a tri-axial accelerometer in a custom-designed vest to detect falls. A barometric pressure sensor has also been used to measure altitude and discriminate fall situations from normal bending down or sitting down activities [77]. An accelerometer embedded in an assistive device such as a cane, which is used by many elderly people for maintaining balance, was used as a fall detecting mechanism in the Smart-Fall system [78].
Smartphones have also played a major part in modern fall detection systems, where it is augmented by the GPS facility within the phone to detect the location of the subject who has fallen [79]. The accelerometer within the smartphone was used effectively for robust detection of falls along with the Google map facility for locating the event, and an associated alarm was generated to the respective caregiver or to the family members through a messaging facility (sms) [80].
More recently, there has been a paradigm shift towards prevention of fall-related injuries by pre-empting a falling incident and using airbag technology to minimize impact [81]. However, further development in the miniaturization of airbags is necessary to produce unobtrusive and comfortable systems that would be acceptable to the user. A system designed to detect freezing of gait (FOG) events that are commonly associated with neurological disorders such as Parkinson’s disease was developed in [82]. It provides subjects with a rhythmic auditory signal for stimulating them to resume walking when an FOG episode is detected. An android application, iWander, using GPS and communication facilities available on the smartphone was developed to provide assistance with tracking location for subjects suffering from dementia [83].
4.3 Activity Monitoring
Activity monitoring is a well-researched and broad topic. Human activity monitoring has gained prominence with the use of wearable sensors and video-based sensing technologies and is a major area of remote health monitoring systems. For post-stroke rehabilitation, tracking the number of times a patient performs specific movements (e.g. exercises) with their impaired body parts (e.g. paretic arm) during training and also throughout the day can provide useful information on the progress of the patient. The frequency of specific movements and the quality of the movements performed (e.g. fluidity/smoothness) are likely to increase as the motor functionality of the patient improves. It can also provide information on the patient’s compliance to the specific guidelines set by the respective clinicians during rehabilitation training.
Rehabilitation is primarily carried out by repeated exercises of the impaired limb to maximize the chances of recovery [84]. However, it is well perceived in the medical community that exercises alone do not suffice for achieving a speedy recovery due to various factors. This is due to the lack of motivation among patients to exercise for sustainable period of time and the fact that exercises comprise only a minor proportion of time and energy spent by a subject as compared to the wide range of activities performed throughout the day. Moreover, patients tend to compensate their paretic arm with their non-impaired arm, making rehabilitation progress slower [85–87].
Home-based rehabilitation through monitoring of specific activities has gained prominence over the recent years through the development of various exercise platforms [88–90], virtual reality (VR)-based systems [91, 92], gaming consoles [93, 94] and the widely popular Kinect camera-based system [95]. These approaches mainly aim to monitor the rehabilitation progress of the patients during the exercise or the training phase in a controlled environment within a designated zone (exercise/gaming platforms and vicinity of camera systems). The main difficulty with this approach is that it offers no possibility to monitor the movement quality of the patients and their compliance with the prescribed exercises in their natural environment (i.e. while performing daily activities) which are more objective reflections of the actual rehabilitation state and of the effectiveness of the prescribed therapy.
Hence, there has been a growing demand to monitor subjects as they perform their daily activities within their home and community settings. Quantifying the daily activities performed by patients would help to ascertain their degree of participation and thereby formulate a qualitative index of their lifestyle [96]. A taxonomy of activities known as Activities of Daily Living (ADLs) developed by [97] gained prominence in the research community owing to its relevance to real-world applications. Typical examples of ADLs include brushing teeth, combing, washing, cooking, bathing or walking [98–102]. Accordingly, there have been extensive research efforts to assess the accuracy of wearable sensors in classifying ADLs [103–107] which has supported medical diagnosis during rehabilitation and augmented traditional medical methods in recovery of chronic impairments [108].
Human activity recognition (HAR) is a challenging and highly researched topic in many diverse fields which include pervasive and mobile computing [109, 110], context-aware computing [111, 112] and remote health monitoring systems which also include AAL [113–117]. Advances in wireless sensor technology have caused a paradigm shift from low-level data collection and transmission to high-level information integration, processing and activity recognition [118]. The different approaches are variants of the underlying sensor technology, the machine learning models and the environment in which the activities are performed. Before embarking on activity modelling and recognition methodologies, it is imperative to understand the different levels of granularity inherent in human behaviour.
4.3.1 Movement Categories
Depending on the complexity of activities performed, they can be categorized into mainly four different levels: gestures, actions, interactions and group activities. Gestures are movements performed by the subject’s body parts which are atomic components comprising any holistic movement. Some common examples of gestures are raising the hand or stretching the leg. Actions are activities performed by individuals that are composed of multiple gestures aligned together to form a meaningful movement. For example, walking or reaching and picking a cup can be described as completed actions. Interactions are used to describe human–object or human–human interaction like making tea. Group activities, as the name suggests, are performed by multiple persons, for example, a group marching together [118, 119].
Activity recognition is a complex process and can be categorized into four main steps: (1) choice and deployment of appropriate sensors and the environment in which the activity will be performed; (2) collection, storage and processing of information through data analysis techniques and knowledge representation formalisms at appropriate levels of abstraction; (3) creation of computational models which allows reasoning and manipulation and (4) to develop reasoning algorithms from sensor data that helps to recognize activities performed.
4.3.2 Modalities of Activity Recognition
Home-based activity recognition can be classified as: vision-based and sensor-based recognition. Vision-based activity recognition uses visual sensing facilities, such as video cameras and still cameras, to monitor a subject’s movement in a designated area. The generated sensor data are video sequences or digitized visual data. Recognition of activities further takes place by the use of computer vision techniques which include feature extraction, structural modelling, movement segmentation, action extraction and movement tracking to analyse visual observations. The use of gaming consoles with camera systems and also the Microsoft Kinect has been quite popular in the field of rehabilitation. However, they suffer from occlusion problems since they are designated to mostly indoor activities with their surveillance restricted within a specific zone. Moreover, inferring the movements performed often involves the use of complex image processing algorithms [120].
Sensor-based activity recognition was further explored owing to a paradigm shift towards monitoring of activities in unconstrained daily life settings. The sensors used in activity recognition mainly generate time series data of various parameters or state changes. The data is processed through statistical analysis methods, probabilistic models, data fusion or formal knowledge technologies for recognizing the underlying activity. Sensors for activity recognition are generally attached to the body of the subject as wearable sensors or are inherent in portable instruments such as smartphones. Sensors can also be embedded within the living environment of the subject and thereby create ambient intelligent applications such as smart environments. For example, sensors attached to objects of daily use can record human–object interaction. Recognition methodologies utilizing multimodal miniaturized sensors present in the environment is referred to as dense sensing approach. In this approach, activities are characterized by the objects that are manipulated during the performed movements in real-world settings. They are widely used in AAL through the smart home paradigm [121–123]. Sensors in smart homes are used to initiate a time-bound context-aware ADL assistance. For example, a pressure mat or force plate can indicate position and movement of a subject within a defined environment, and a pressure sensor in a bed can suggest sleeping activity of the subject [31]. In general, wearable sensor-based monitoring is used in pervasive and mobile computing, while the dense sensing-based approach is more suitable for intelligent environment-enabled applications. Both approaches are not mutually exclusive, however, and in some applications they can work well together such as in RFID-based activity monitoring, where objects in the environment are instrumented with tags, and users wear an RFID reader fixed to a glove or a bracelet [117, 124].
4.3.3 Inertial Sensor-Based Activity Recognition
Activity recognition using wearable inertial sensors primarily involves the capturing of kinematic signals which are used to measure acceleration, velocity, distance, rotation, rate of rotation, angle and time. These measurements help to determine position of a limb segment or the angle of flexion of a limb joint. These classes of signals are widely used as health indicators encompassing gait, posture, spasticity, tremor and balance, some of which are subjective parameters in clinical assessment. Most of these parameters can be measured with the help of kinematic sensors like accelerometers, gyroscopes and magnetometers [125, 126], thereby removing the subjective quotient from symptomatic data [127].
Kinematic sensors are based on the transformation of physical parameters to an electrical signal which is further processed. Microelectromechanical systems (MEMS) play a key role in remote health monitoring applications, where size and power consumption are of vital importance. In MEMS-based transducers, changes in the electromechanical characteristics of the silicon are used to generate resistive or capacitive variations when the microstructure within the transducer is excited by external forces such as compression, pressure, temperature and acceleration. [128]. Some of the popular kinematic sensors used in the field of HAR are accelerometers, gyroscopes and magnetometers [125, 127].
An MEMS accelerometer is probably the most frequently used wearable sensor used for activity recognition. Gyroscopes are used primarily for computing rates of rotation (°/s) and, when combined with accelerometers, form an inertial measurement unit (IMU) [129], in which the gyroscopes are additionally used to compensate for errors in the accelerometers due to changes in orientation. Hence, IMUs can be used to measure acceleration, velocity, distance, rotation and orientation [128]. A magnetometer is another device that is sometimes used in remote monitoring applications that responds to the strength and direction of the Earth’s magnetic field. Considering that the magnetic field vector has a constant direction and magnitude within a predefined area on the Earth’s surface (given by the latitude and longitude), a magnetometer can be used to track orientation with respect to this localized constant field vector [128]. Magnetometers are not extensively used in health monitoring applications due to the fact that the Earth’s magnetic field can be distorted by the presence of ferromagnetic materials [130]. Patients requiring wheelchair support (with steel frame) or the presence of other ferromagnetic substances within the home environment (e.g. in the kitchen) are likely to distort the reference magnetic field.
4.3.4 Data-Driven Versus Knowledge-Driven Approach
Processing of sensor data for recognizing activities can be categorized into two approaches: data-driven and knowledge-driven. Development of activity models is important for interpreting the sensor data to infer activities. In the data-driven approach, sensor data collected as a result of the movements performed by the subjects are used to build activity models with the help of data mining techniques and relevant machine learning algorithms. Since this involves probabilistic or statistical methods of classification driven by the data, the process is generally referred to as data-driven or bottom-up approach. Although this approach has its advantages in being robust to uncertainties and temporal variation in information, it requires the availability of a large dataset for training the activity model. Further it suffers from reusability and scalability as often it has been seen that activity models developed and evaluated on a particular subject’s data does not work on the movement data of another subject owing to the large degree of variability inherent in human movement [118].
The knowledge-driven approach, on the other hand, is used to exploit the rich prior domain knowledge to build upon an activity model. This involves knowledge acquisition, formal modelling and representation and is hence referred to as knowledge-driven or top-down approach. It is based upon the observation that most activities are performed in a relatively specific location, time and space. A suitable example would be an act of brushing teeth, which takes place in the morning, evening or at night and involves the use of a toothbrush. Similarly, cooking in the kitchen involves the use of the microwave or cutlery. This implicit relationship between activities, temporal and spatial context and the entities involved provides a rich domain knowledge and heuristics for activity modelling and pattern recognition [118]. This approach is semantically clear, logically simple but weak in cases of uncertainty and temporal information.
Having discussed the different modalities and approaches of HAR, we will take an in-depth look into the process of activity modelling, classification and recognition using the data-driven approach. Prior to this, we shall first examine the various challenges concerning activity recognition.
4.3.5 Challenges in Activity Recognition
Activity recognition presents more degrees of freedom with respect to system design and implementation when compared to language processing or speech recognition [131]. However, owing to the diversity inherent in the data collected by different individuals performing the same action or by the same individual performing an action in different environments, it requires careful consideration of the type and placement of sensors and data analysis techniques used, depending on the application scenario and activities to be monitored [132].
4.3.5.1 Class Variability
A recognition system has to be robust enough to handle intra-class variability. In this context, class refers to the activities that are to be detected by any recognition methodology. This variability is primarily due to the fact that a same activity is performed differently by different individuals. Further this might also happen with an individual who repeats the same activity over time due to factors as fatigue or environmental changes. Therefore, there can be two approaches towards training an activity recognition system.
A system trained with movement data of more than one subject, or a person-independent training system would be susceptible to considerable inter-person variability [132]. To address this issue, the number of data points for each subject can be increased or an alternative approach can be person-dependent training, i.e. training the system on the movement data of single person. This might as well be robust enough towards capturing considerable intra-person variability. This system, however, requires the collection of a large set of data collected from one individual to train the system, thereby capturing as much variability as possible. The choice of the training sample is application dependent and hence a trade-off is required between the selection of a highly specific and discriminative dataset or a generic dataset which is potentially less discriminative but robust across multiple subjects [131]. In general, for remote health applications, formulating a person-centric training data would be beneficial when applied to monitoring of individual patients who demonstrate differences in levels of impairment depending on their stage of rehabilitation [133]. Another interesting challenge in recognizing activities is the similarity in characteristics prevalent across activities [134]. For example, if we would like to distinguish between drinking water from a glass and drinking coffee from a cup, it would be very difficult to differentiate from the kinematic data pertaining to both the movements. Therefore, in such cases sensors deployed in the environment like RFID tags attached to objects can prove to be helpful. Therefore, this is dictated by the requirements of the application [124].
An intriguing problem occurs during activity recognition on continuous streaming data or real-time monitoring applications where the data needs to be segmented depending on the activities we want to monitor and those that are irrelevant to the application. This is referred to as the NULL class in the relevant literature [135] and is difficult to model since it represents a plethora of activities in infinite space. It can however be identified if the signal characteristics gathered from the sensor data are completely different to the ones that are being monitored and hence involves a threshold-based mechanism to filter out the unwanted data. Class imbalance is a major problem, especially during long-term monitoring where all activities being tracked do not have the similar number of occurrences. A common example would be the number of instances of a drinking action and a walking action [136]. There are however a couple of techniques which can be adapted to get around this problem of class imbalance. Firstly, generating artificial training data for a class that is underrepresented to balance out inequality; secondly, oversampling or interpolating smaller class sizes to match a bigger class size [137].
4.3.5.2 Ground Truth Annotation
Annotating the ground truth of activities being monitored in real-life scenarios is another interesting challenge, especially with data from wearable inertial sensors as opposed to data obtained from video recordings. With activities performed in the laboratory or controlled environment, annotations of the training data can be performed post hoc based on video footages. However, in nomadic settings, ground truth annotation of activities is a very difficult problem to solve. Researchers generally depend on self-recalling methods [138], experience sampling [139] and reinforcement learning, all of which involves testimonies from the subject themselves. Therefore, many researchers have based their work on a list of activities performed under a semi-naturalistic condition, where the subjects perform the movements as they would do in normal daily life, and another person annotates their activities by means of visual inspection in real time [100]. This therefore helps in gaining the ground truth information required for evaluating the recognition methodology.
4.3.5.3 Sensor Requirements
The experimental design gives rise to another challenge that of data collection, sensor selection, placement and the number of sensors to be used. As opposed to other computer vision problems like heart monitoring, brain activity modelling or speech recognition, HAR does not have a standard allocated dataset to start with the data analysis as it is completely dependent on the requirement, and experiments are designed in pursuit of recognizing only the selected movements. Sensor characteristics also present a significant amount of challenge for long-term monitoring of activities as hardware failures, sensor drifts and errors in the software aimed at capturing the data can lead to erroneous situations. External factors such as temperature, pressure and change in positioning due to loose straps can cause the need for frequent recalibration, thereby affecting the sensor data being recorded [140, 141].
One of the last challenges is power requirement of the battery-operated wireless sensors which are increasingly being used in the field of remote health monitoring. The remote monitoring system in place at present transmits the captured signals collected by the sensor nodes placed on the patient’s body to the remote server at the back-office service platform wirelessly, where the signals are analysed [142]. This system requires continuous transmission of data from the sensors to the server using wireless protocols taking into account the nomadic environment. The fundamental problem with continuous data transmission is the energy requirement. A result from respective investigations into continuous data transmission at 1 kHz suggests that it can be supported for 24-h monitoring using a 1200-mAh battery [143].
The analysis presented in [143] regarding the power consumption and longevity of batteries pertains to transmission energy only, added to it the energy involved in pre-processing the physiological data at the sensor nodes including analogue-to-digital conversion, quantization, filtering and the microcontroller operation would bring down the effective time of monitoring to 8–10 h, thereby making the entire system power hungry and affecting the life of the batteries. An increased battery capacity like the prismatic zinc–air battery—1800 mAh operating at 1.4 V used recently in the medical community would increase the respective sizes of the sensor nodes. Furthermore, the use of Bluetooth transceivers consuming 40–55 mA with operating voltage in the range of 3–3.6 V would necessitate the use of three such zinc–air batteries, making it non-ideal in terms of volume for body-worn applications. The supply voltage is quadratically proportional to the power dissipation and therefore an optimal power supply to sustain the continuous Bluetooth transmission would have an adverse impact on the operational lifetime of the battery-powered sensor nodes. Considering Bluetooth as the primary means of communication, the energy dissipation is directly dependant on the packet format of the data being transmitted which can be optimized using standard duty cycling and might eventually lead to delays and packet loss of data which would be highly undesirable for applications involving remote health monitoring [142].
Therefore, from the long-term system operation perspective, when implementing a wireless body area network (WBAN) that comprises heterogeneous sensors, it is imperative to select data analysis algorithms having low computational complexity. This is because energy consumption is directly proportional to the computational complexity of the processing algorithms used. Therefore, for applications such as real-time movement detection requiring online operation, it is imperative to perform the data processing (feature extraction, classification) in a low-power way on a sensor platform [132, 142] itself while for applications supporting long-term behavioural or trend analysis, offline data processing may be sufficient [144].
4.3.6 Activity Recognition: Process Flow
In this section, we discuss the sequence of signal processing and pattern recognition techniques that help to implement a specific activity recognition behaviour using supervised learning methodologies. The process flow is shown in Fig. 4.2.
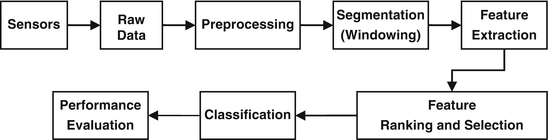
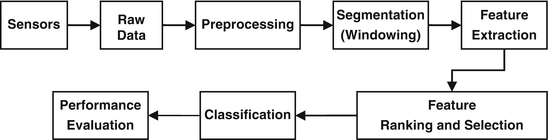
Fig. 4.2
Activity recognition process flow
4.3.6.1 Data Acquisition and Pre-processing
Raw data is collected from multiple sensors attached to the body or sensors placed on objects or in the environment or from both, depending on the application requirement as discussed in Sect. 4.3.2. Data coming from each sensor sampled at regular intervals results in a multivariate time series. However, the sampling rates of different types of sensors might differ. Therefore, there is a need to synchronize multimodal sensor data. Inertial sensor data are generally sampled at low frequencies, 20–30 Hz, depending on the movements to be monitored. Certain sensors like accelerometers, gyroscopes and magnetometers can produce data that have multiple dimensions (X-, Y– and Z-axis). Therefore, a multidimensional and a multimodal sensor output can be represented by (1), where S represents the sensor output, m represents the number of sensors and d 1 …d n represents the sensor-specific data sampled at regular intervals.
![$$ {S}_i=\left[{d}_1,{d}_2\dots {d}_n\right],\kern0.46em i=1,\dots, m $$](/wp-content/uploads/2016/09/A327370_1_En_4_Chapter_Equ1.gif)
![$$ {S}_i=\left[{d}_1,{d}_2\dots {d}_n\right],\kern0.46em i=1,\dots, m $$](/wp-content/uploads/2016/09/A327370_1_En_4_Chapter_Equ1.gif)
(4.1)
The raw sensor data contains noise and is often corrupted by artefacts caused due to various factors. Artefacts are generally induced into the data due to sensor malfunctioning (e.g. drift) or due to unwanted movements of the body [131]. The pre-processing stage aims to remove the low-frequency artefacts and high-frequency noise components by using high-pass and low-pass filters [132]. The pre-processing algorithms also synchronize the data coming from various sensors and prepare it for the next stage of feature extraction. It preserves the signal characteristics which carries the relevant information about the activities of interest. Data from inertial sensors are in general calibrated; their units converted (as most sensor outputs are arbitrary units), normalized, resampled, synchronized, then filtered or fused in the pre-processing stage. Sensor fusion is generally performed where signals from multiple sensor axes are selected a priori, based on the activities being tracked. For example, specific accelerometer and gyroscope axes can be fused (both sensors placed on the wrist) for detecting a reach and retrieve action [132].
4.3.6.2 Data Segmentation
The pre-processed signal is segmented to identify only those segments that contain information about the activities that are being monitored. This process is also commonly referred to as event detection or activity spotting since it detects the signal frame representative of the activity of interest. The boundary of each segregated time series data is represented by the start and stop time. Thus, each segmented time series represents the potential activities to be monitored. Segmenting a continuous stream of data is a difficult task, especially for monitoring ADL. For example, consider a drinking activity. This may be considered as starting when reaching for a cup or glass. Alternatively, it may be considered as starting when the cup is raised to the lips. But what about when the cup is simply resting in the hand between individual sips? Should this still be classified as a drinking activity? In such circumstances, it is difficult to determine the boundaries of the activity from the signal. There are various segmentation algorithms that are used in relevant research, popular among them being the sliding window technique, energy-based segmentation, rest-position segmentation and using data from one sensor to segment another sensor reading [131].
The sliding window technique is one of the most popular segmenting schemes followed in diverse applications. As is suggested by the name, a fixed-size window representing definite time duration is used to extract segments of a signal [108]. If a very small interval is chosen, there is a possibility of missing out on a relevant activity whereas a longer window size would pertain to multiple activities, thereby affecting the classification decision. Hence, a dynamic window selection technique based on a data-driven or a probabilistic approach for segmenting each individual activity would be an optimal solution although this increases the computational load [118].
Another popular approach adopted for segmenting different activities is based on the energy content of the signal reflecting the change in intensity levels. The differences in energy levels in the signal are representative of the intensity variations of the activities that produce these kinematic signals. The energy content of a signal s(t) is given by (4.2).
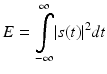
Therefore, a threshold-based mechanism based on the value of E can help to identify segments of activities which are identical [145]. Researchers have explored energy-based segmentation with the assumption of a rest period between each activity which is particularly useful for gesture recognition involving discrete activities and momentary pauses [146].
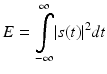
(4.2)
4.3.6.3 Feature Extraction
The choice of features is a fundamental step for classification and a highly problem-dependant task. Although each of the sensors exhibits signal patterns that are distinctive for each of the movements and may be recognizable to the human eye as shown in Fig. 4.3, in order for a machine to recognize these patterns a set of characterizing features must be extracted from the data. Features represent the transformation of the raw data into another space known as the feature space. It is a measure to define the raw data in a quantitative as well as a qualitative manner such that it characterizes the raw data. The total number of features extracted from a feature space, where ideally identical activities should be clustered together whereas features corresponding to different activities should lie far apart. The selection of features is dependent on the activities that are to be classified.
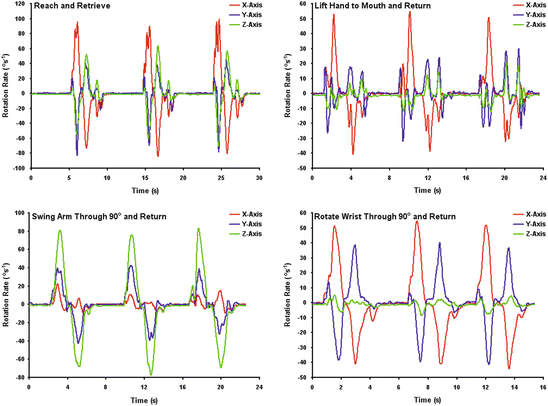
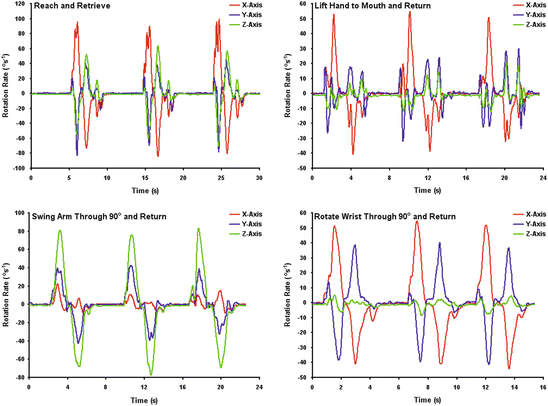
Fig. 4.3
The signal patterns generated by a tri-axial gyroscope placed near the elbow for three repetitions of four different actions—reach and retrieve, lift hand, swing arm and rotate wrist
The patterns shown in Fig. 4.4 clearly stress the importance of deciding on the right feature. Typical feature sets for HAR include statistical functions, time and/or frequency domain features, as well as heuristic features [147]. Some of the commonly used time-domain features extracted from sensor data and reported in the literature are: arithmetic mean, variance, median, skew, kurtosis, inter-quartile range, root mean square, standard deviation and correlation between axes [148, 149]. Correlation between accelerometer axes can improve recognition of activities involving movements of multiple body parts. For example, the activities of walking and climbing stairs might exhibit the same degree of periodicity and magnitude of a kinematic signal, but walking involves translation in one dimension whereas climbing stairs involves translation in multiple dimensions [150]. The often used mathematical features of variance, inter-quartile range, root mean square and standard deviation are useful measures of the variation in the data representing an action. The statistical functions of kurtosis and skew may appear out of place when considering human activity since these are more usually associated as descriptors of the shape of a probability distribution. Nevertheless, these functions can still return values that uniquely identify different classes of activity, and therefore are rightly considered as appropriate classification features.
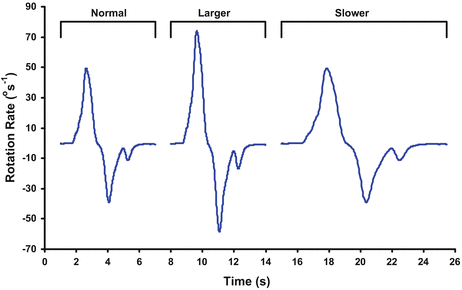
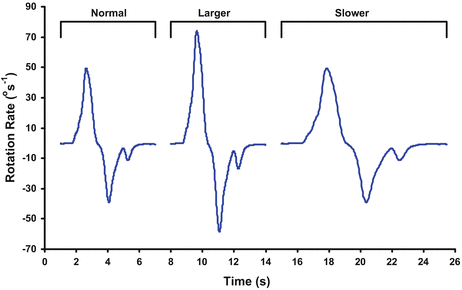
Fig. 4.4
Examples of movement patterns that exhibit obvious differences but are essentially the same movement
Commonly used frequency domain features are extracted from the coefficients of various time–frequency transforms such as the Short Time Fourier transform (STFT), Fast Fourier transform (FFT) and the Continuous or Discrete Wavelet transform (WT). The high-frequency component of an accelerometer signal also known as the AC component is primarily related to the dynamic motion of the subject like walking, running and handshaking while the low-frequency DC component of the signal is related to the gravitational acceleration and hence is informative about the orientation of the body in space and can be used to classify static postural positions [151].
The signal energy and its distribution at different frequency bands are popular choices for discriminating activities of differing intensities. More specifically, some of the commonly used features are spectral centroid, spectral spread, estimation of frequency peak and estimation of the power of the frequency peak and signal power in different frequency bands [148, 151]. Frequency domain entropy, calculated from the normalized information entropy of the discrete FFT component magnitudes of the signal, helps to discriminate activities with similar energy content. For example, cycling and running might result in similar values of energy if captured with an accelerometer placed near the hip. Cycling involves a uniform circular motion, and discrete FFT of the acceleration data in the vertical direction may show a single dominant frequency component at 1 Hz and low magnitude for all other frequencies. By comparison, the action of running may produce major FFT components in the low-frequency range 0.5–2 Hz [100].
4.3.6.4 Feature Selection
With a higher dimensional feature space, learning the parameters becomes a difficult task for the classifier because in such cases a large number of data samples (i.e. more training data) are required for the parameter extraction of the model and hence the computational complexity of the classification increases. The performance of the classification algorithm depends on the dimension of the feature space and hence methods to reduce the dimensionality are considered in the field of activity recognition. Particularly for real-time activity recognition, it is imperative to use the minimum number of features with an eye on computational complexity and memory utilization. If features with minimum discriminatory abilities are selected, the subsequent classification would lead to poor recognition whereas if information-rich features having a large between-class distance and small within-class distance in the feature space are selected we can expect to have a better classification. This would imply that features would take distant values in different classes and closely located values in the same class. However, before proceeding with feature selection, the feature vectors need to be pre-processed to remove the outlier points and feature normalization [152].
An outlier is a point that appears as a result of noisy measurement and lies far away from the mean of the corresponding feature vector causing large errors during the training of the classifier. For normally distributed data, a threshold of up to three standard deviations from the mean is used to filter out the outliers. For non-normal distributions, more complex measures like cost functions are considered [152].
Feature normalization is another key step adopted for feature values lying in different numeric ranges, such that features with large values do not dominate the cost function in the design of the classifier. A common technique is linear normalization as shown in (4.3), where the features are normalized by removing the mean from each sample and dividing the samples by their standard deviation. This ensures that each feature has zero mean and unit variance and can be represented as:
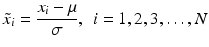
where x i represents the respective feature values, μ is the mean value, σ is the standard deviation and
represents the normalized feature values. Alternatively, other linear techniques can be used to normalize the feature values by restricting them between a minimum and a maximum value as expressed in (4.4). Selecting the range depends on the nature of the data.

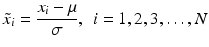
(4.3)


(4.4)
Non-linear methods of normalization are also applied for data which are not evenly distributed about their mean. In such circumstances, non-linear functions like logarithmic or sigmoid can be used to transform the feature values within specific intervals [152]. Another option is scaling the feature vectors by the Euclidean length of the vector.
The normalization step is followed by the feature ranking step. Fisher’s Discriminant Ratio (FDR) and Bhattacharyya distance are such techniques used to quantify the discriminatory ability of each individual feature between two equi-probable classes. Another class separability technique, based on scatter matrices, can be used for a multiple-class scenario [152]. The rank of each individual feature is determined, where a high rank represents a small within-class variance and a large between-class distance among the data points in the respective feature space [153]. The class separability based on scatter matrices is further explained.
The rank of each individual feature for a multiple-class scenario is determined by the J value [152] as calculated below:

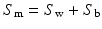
S w and S b are the within-class and between-class scatter matrices, respectively, and S m is the mixture scatter matrix. The respective expressions are presented below:
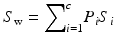
where P i denotes the priori probability of a given class i = 1, 2,…c and S i is the respective covariance matrix of class i.

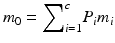
m 0 is the global mean vector. A high value of J represents a small within-class variance and a large between-class distance among the data points in the respective feature space [152].

(4.5)
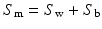
(4.6)
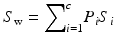
(4.7)

(4.8)
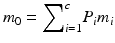
(4.9)
The ranked features are sorted in a descending order to determine the one having the highest rank. As opposed to other popular multi-class feature ranking algorithms used in activity recognition like the ReliefF algorithm [3] and Clamping technique [108], the use of scatter matrices is computationally less complex and at the same time it quantifies the scatter of feature vectors in the respective feature space [152].
We come to the core problem area wherein we have to now select a subset of l features from the best ranked m features (where l ≤ m). The two major approaches are—scalar feature selection and feature vector selection. In the scalar feature selection technique, each feature is treated individually and their class separability measure is ascertained using any of the above-mentioned criterion c(k) (FDR, Scatter Matrices) for each feature. Features are then ranked in a descending order according to criterion c(k), and l best features are considered for classification. Considering features individually involves low computational complexity but is not effective for complex classification problems and for cases where features are mutually correlated. Feature vector selection can be approached in two ways:
1.
Filter approach: The features are selected independent of any classification technique. For each combination of features chosen, we apply the class separability criterion as mentioned above and select the best feature combination. Considering m = 10 and l = 5, we can have 252 feature vector combinations which are very large, and the number l is also not known a priori.
2.
Wrapper approach: The selection of the feature is based in association with the classifier to be employed. For each chosen feature vector combination, the classification error probability of the classifier is estimated, and the feature combination with the minimum error is chosen. This approach can be further computationally complex depending on the choice of the classifier.
However, to reduce the complexity there are some effective searching techniques, which have been proposed to select the best feature vector combination: sequential backward selection, sequential forward selection and floating search method [152]. We will discuss the sequential forward selection technique (sfs) with a working example of a feature vector comprising four different features [X 1, X 2, X 3, X 4]. First, we compute the best ranked feature, say X 2 , and evaluate the classification performance with X 2. Secondly, we compute all two-dimensional feature vector combinations with X 2: [X 1, X 2], [X 2, X 3], [X 2, X 4] and evaluate the classification performance for each of the combinations. Thirdly, we compute all three-dimensional feature vector combination with X 2: [X 1, X 2, X 3], [X 1, X 2, X 4] and evaluate the classifier performance with both the combinations. Finally, we select the best feature vector combination as the desired features [152]. Similarly for the sequential backward selection technique, we start with a combination of three features, eliminating one feature from each of the combinations and then evaluate its performance with respect to the classification algorithm employed. From each respective feature combination, we again eliminate one feature and evaluate the two-dimensional feature combinations.
Both these methods suffer from the fact that once a feature is selected or discarded in the forward or backward selection technique, respectively, there is no possibility for it to be discarded or reconsidered again. This problem is referred to as the nesting effect. Therefore, a flexible technique to reconsider previously discarded features and vice versa is known as the floating search method [152].
A popular analytical technique often reported in the literature is Principal Component Analysis (PCA), or the Karhunen–Loeve transform, which transforms feature vectors into a smaller number of uncorrelated variables referred to as the principle components [150, 151]. Another popular approach is Independent Component Analysis (ICA), often applied in problems of blind source separation, which attempts to decompose a multivariate signal into statistically independent non-Gaussian signals [154]. The choice of relevant features and choice of ranking or selection technique are completely dependent on the activities, type of sensors and the application scenario.
4.3.6.5 Classification
A wide range of classifiers have been used for activity recognition in recent years [155]. The determining factors for the selection of the classifier are accuracy, ease of development and speed of real-time execution [131]. Two distinct approaches can be used in classifying human activities—supervised and unsupervised learning. In supervised learning, the association of the training dataset comprises selected feature vectors with each class label is known beforehand [151]. In unsupervised learning, only the number of classes is known and the system assigns a class label to each instance in the training dataset. Clustering-based unsupervised learning has been used in the field of activity recognition [153, 156].
In HAR, the classification schemes used can be broadly categorized into three themes: probabilistic models, discriminative approach and template-based similarity metrics, as described below.
1.
Probabilistic models: Probabilistic models are quite commonly used for behaviour modelling since they are an efficient means of representing random variables, dependence and temporal variation. In this approach, the activity samples are modelled using Gaussian mixture, yielding promising results for offline learning when a large amount of data is available for training. Generative probabilistic models such as HMMs have been used to model activity sequences and have been extended to hierarchical models like Conditional Random Fields (CRFs) and Dynamic Bayesian Networks (DBNs) [156]. Hidden Markov Models (HMMs) have been very popular in speech recognition and have also been used in applications for hand gesture recognition [157, 158]. In general, the HMM is trained on pre-defined class labels using the Baum–Welch algorithm and is tested on new instances. The Baum–Welch algorithm is a generalized Expectation Maximization (EM) algorithm that computes the maximum likelihood estimates of the parameters of an HMM given the observations as training data [159, 160]. The problem with HMMs is the first-order Markov assumption where the current state depends only on the previous one. Further, the probability of a change in the hidden state does not depend upon the time that has elapsed since entering into the current state. Therefore, a time dependence has been added to HMMs and they have been augmented to semi-HMMs where the hidden process is semi-Markovian rather than Markovian. Coupled HMMs have also gained prominence which is considered as a collection of HMMs, where the state at time t for each HMM is conditioned by the states at time t-1 of all HMMs in the collection. They are used to model the dynamic relationships between several signals [161].
2.
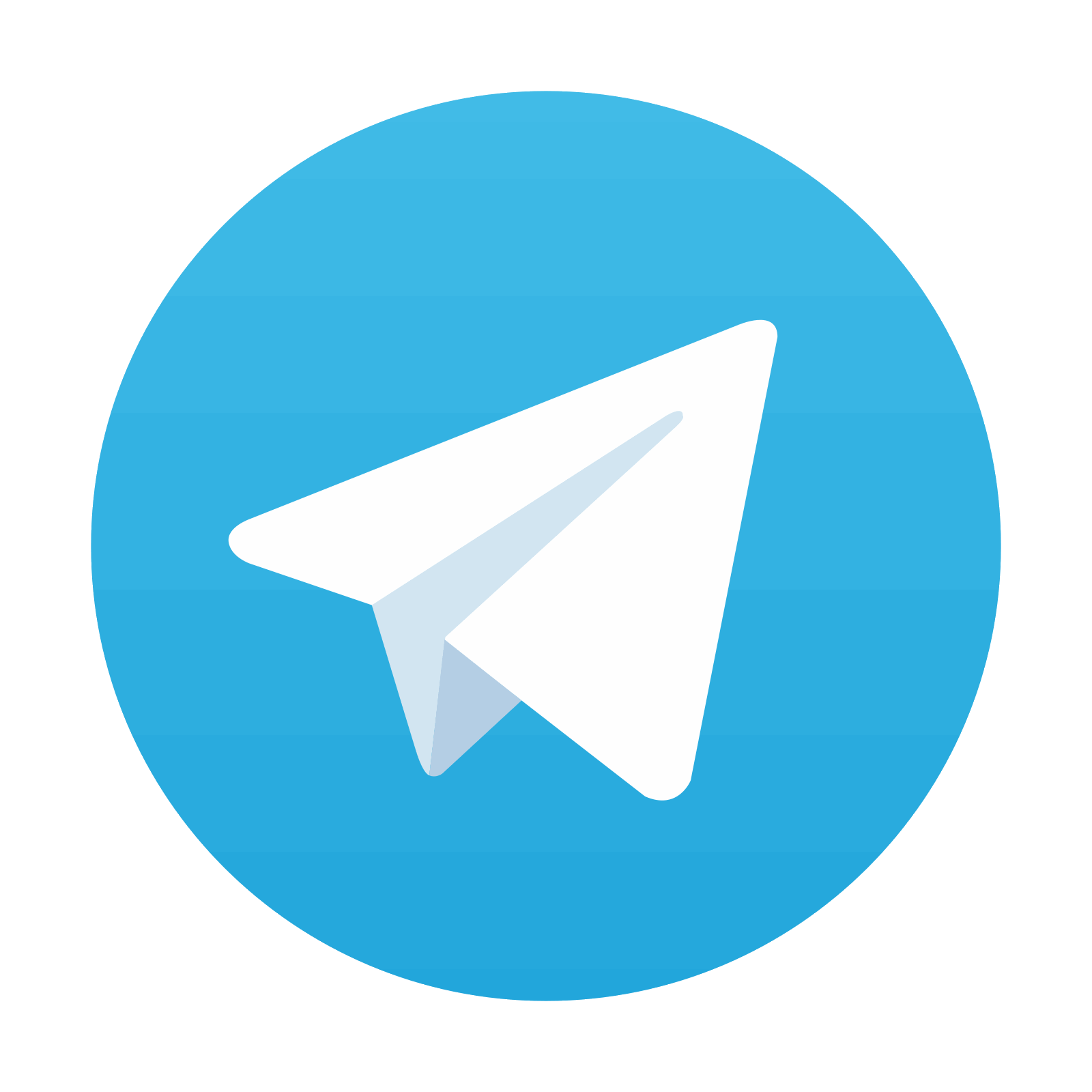
Discriminative approach: The classification is based on the construction of the decision boundaries in the feature space, specifying regions for each class. The decision boundaries are constructed on the feature vectors of the training set, through an iterative or a geometric consideration. The Artificial Neural Network (ANN) commonly used for detecting ADL consists of inputs and outputs with a processing or a hidden layer in between. The inputs are the independent variable, and the outputs represent the dependent variable. The internal (hidden) layers can be adjusted through optimization algorithms such as the resilient back-propagation or scale-conjugate algorithms [106].
The k-Nearest Neighbour (k-NN) [162] and the Nearest Mean (NM) classifiers work directly on the geometrical distances between feature vectors from different classes [163]. Support Vector Machines (SVMs) work by constructing boundaries that maximize the margins between the nearest features relative to two distinct classes. SVM is a very popular technique in machine learning community and generally produces high accuracy rates with moderate computational complexity (depending on the number of support vectors used) [108, 164]. In principle, it is a binary classifier but has been extended to handle multiple classes using the “one-versus-all” or the “one-versus-one” scheme [165]. However, both of these methods can be computationally intensive depending on the number of target classes. The Naive Bayes classifier has also been successfully used over the years [116, 149]. It assumes conditional independence among all feature vectors given a class label and learns about the conditional probability of each feature. They require large amounts of data and do not explicitly model any temporal information which is very important in activity recognition [118]. Finally, binary tree classifiers have been widely popular in the field of HAR, where the classification process is articulated in several different steps. At each step, a binary decision is made based on different strategies like the threshold-based or template-matching. With each stage, the classification is progressively refined as the tree descends along the branches [166]. The C4.5 Decision Tree (DT) algorithm is by far the most popular algorithm and has been used to achieve successful recognition of daily living activities [100, 148].
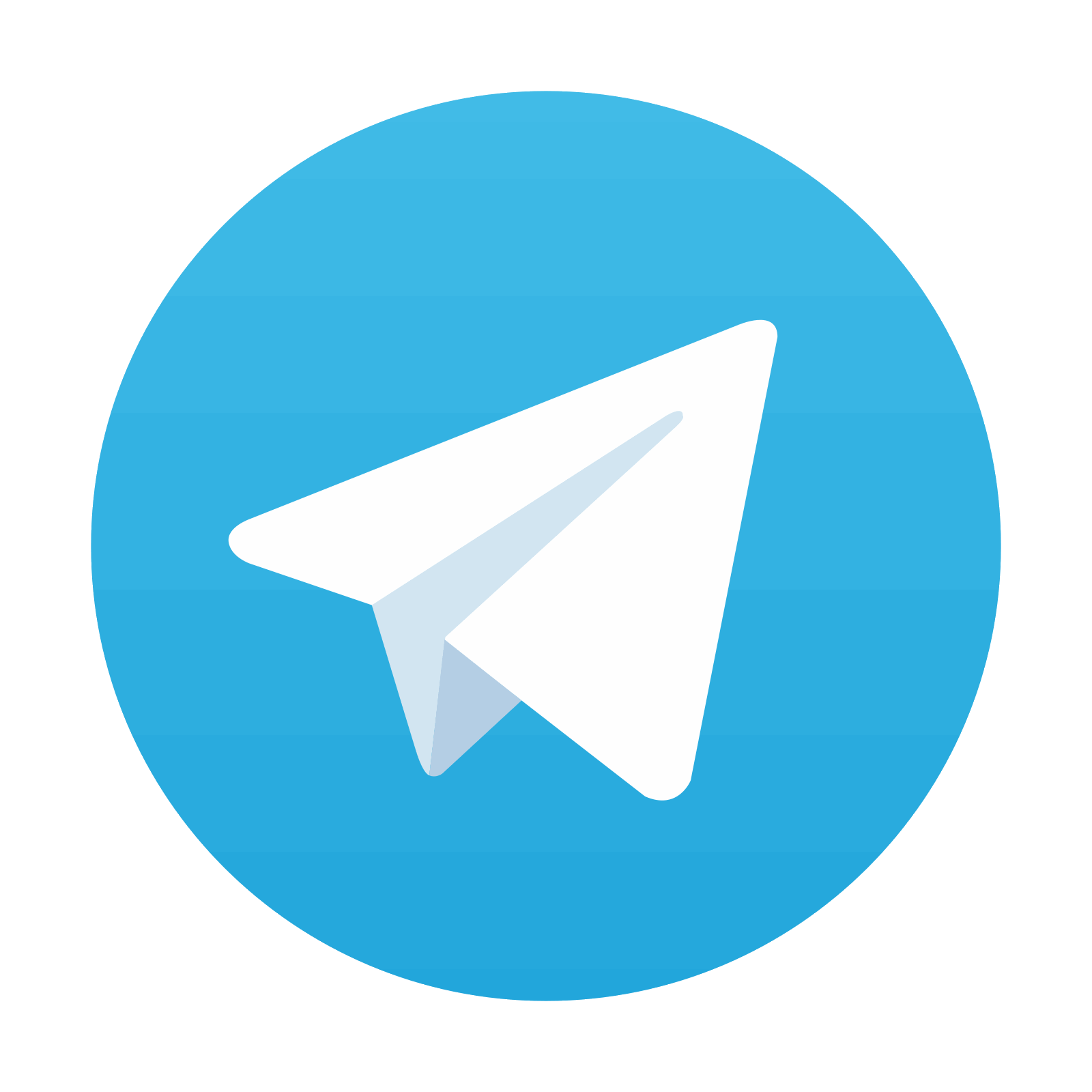
Stay updated, free articles. Join our Telegram channel

Full access? Get Clinical Tree
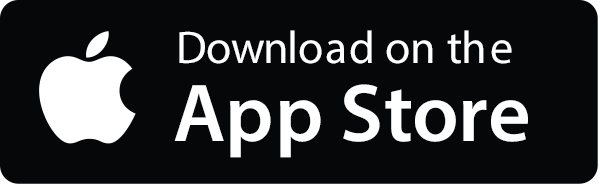

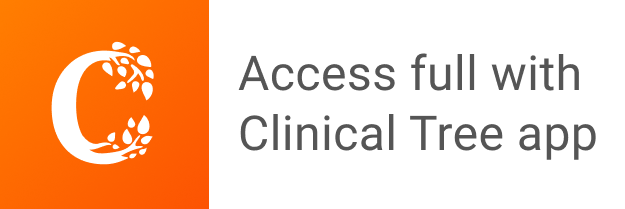