54 How to Limit Bias in Experimental Research
All scientific studies are subject to experimental error, and it is the duty of the investigator to eliminate it where possible, or reduce its impact if it cannot be completely removed. Error may jeopardize the validity of research findings, which may result in useless or harmful treatments being recommended, a waste of limited research resources. Bias is a specific type of error that results in a consistently false result through a systemic flaw in the experiment′s methodology. It has been increasingly recognized that clinical and basic scientific research may be subject to significant biases that jeopardize their findings. 1, 2
In order to understand and reduce potential sources of error, an investigator requires a sound understanding of the experimental process (Fig. 54.1). Some study types are inherently more at risk of error than others. Inclusion and exclusion criteria are selected, or a suitable animal model, organ, tissue, cell line, or other biological material is chosen. An outcome measure is selected, and comparison of this outcome measure is made between study groups. The methodology of group allocation is critical to performing a sound experiment. The study results are analyzed, written up, and reported via posters, conference presentations, and papers. These are then disseminated to other researchers via journals and research databases. These results may then be further assimilated by meta-analysis.

54.1 New Techniques in Musculoskeletal Research
Jargon Simplified: Finite Element Analysis
Finite element analysis is a technique that is used to examine the internal stresses, strains, and deformation of materials under loads. It can be applied to simple homogenous materials, or complex heterogeneous biological tissues. Each structure is composed of a multitude of smaller elements that may be rectangles, cubes, or tetrahedrons. They are linked by “nodes” at their edges. The properties of each element and their influence on their neighbor is calculated for the overall structure. This requires intensive computational power for complex materials. Specific biological tissues can be modeled through the creation of a “mesh” from three-dimensional imaging such as computed tomography. The results predicted by the models can be compared to in vitro testing of the tissue under controlled conditions as part of the validation process.
Musculoskeletal research has been enhanced by the development of new techniques to explore biochemical processes, genes, and proteins. New imaging techniques have allowed more detailed structure of cellular and extracellular structure. These new techniques are subject to experimental error, and the investigator should recognize the potential sources of error. Biomechanical research also regularly investigates the material properties of tissues and implants. Design and testing of novel implants requires techniques to evaluate strength, fatig-ability, and wear in both in vitro and in vivo scenarios. Finite element analysis, while offering myriad new possibilities to understand loading and stress distribution in biological tissues and implants, has specific sources of error and bias that must be recognized.
Techniques in molecular biology and biomechanics are constantly evolving, and it is impossible to provide complete coverage of each of them, along with specific biases. The aim of this chapter is to discuss the types of bias that may occur in research, with particular reference to musculoskeletal research. It will also discuss techniques to reduce these through study design and risk assessment. Using this knowledge, researchers will be able to reduce the influence of bias through careful planning of their research and experiments. They will also be able to analyze new techniques for potential sources of bias.
54.1.1 Key Concepts: Types of Experimental Error
Random error occurs when there is chance variation between individuals or specimens. Biological processes may be influenced by myriad factors, and no two specimens or sets of environmental conditions are identical. These errors can only be reduced by the ubiquitous techniques of increasing repetition, multiple samples, and averaging of results. Statistical techniques exist that may be used to decide whether a difference between groups is a result of chance or represents a real effect. Random errors are generally reduced by increasing the sample size. Sample sizes are limited, however, by experimental design, technical, time, and funding considerations. A researcher can predict the sample size required by performing a power calculation. A power calculation generally requires an estimate of the dispersion of the characteristic in the sample (such as standard deviation) and the minimal detectable difference expected to be measured. In situations where these are not able to established from existing research, a pilot study may be required.
Systematic error is otherwise known as bias. 3 While random errors can be reduced by repeated experiments, biased studies can never be improved by repetition or sample size. Bias is the tendency of an experiment to produce a finding that is not consistent with the actual situation, through the methodology of performing the experiment. There are five main types of bias and they are influential at different stages of the research path.
54.1.2 Key Concepts: Main Types of Bias
Selection bias: Systematic error introduced in choosing the experimental population and dividing it into groups
Performance bias: Systematic error introduced carrying out the experiment
Measurement bias: Systematic error introduced assessing outcome
Analytical and interpretation bias: Systematic error introduced during the analysis of data and interpretation of results
Publication bias: Systematic error introduced during the submission and presentation of the findings
54.2 Types of Bias
54.2.1 Selection Bias
Selection bias occurs where experimental subjects or specimens are divided into different intervention groups. The optimum situation would for each group to be completely identical apart from the characteristic of being deliberately altered between groups. This could refer to animal model age and gender or cell line characteristics. Confounding is a particular result of selection bias, where a second, unmeasured characteristic is linked with group selection and may also influence a group to have an association with the outcome measure. In animal models that require the induction of a disease state or performance of a surgical procedure, knowledge of the subsequent study group may influence the researcher performing this step, thereby introducing bias.3
An experiment may also be planned on tissue obtained from live patients (such as ligament, tendon, cartilage, or bone). In such cases, other forms of selection bias may occur, similar to other clinical studies. Sample bias is a form of selection bias that results from the sample population having different characteristics from a target population such as age, gender, or comorbidity. Sample bias is one of the most common biases present in basic science research, where the tissue or model is not adequately representative of the in vivo process and the generaliz-ability of results is limited.
Referral bias may occur if only a proportion of those in the target population, meeting the inclusion criteria, are considered for the research study. Certain groups of patients may be more likely to participate in research than others, and this may introduce participant bias where the results will have a tendency to reflect the situation in those more likely to participate in research. Some of these effects may be negligible, but often they are unknown and inestimable.
Selection bias can also result from loss to follow-up or post hoc exclusion of subjects. In basic science research, this may occur where a technique does not work as planned in a particular group. If these results are not included, the results may be biased toward or away from the group in which the technique worked.
Reducing Selection Bias
Control of selection bias is easier in prospective experiments where the target population or specimen specification can be tightly controlled to ensure uniformity between group. In retrospective studies, or studies where experimentation is planned on tissue obtained from patients during a study, selection bias is primarily controlled through rigorous study design and participant selection. In animal studies, the specimens should be of the same age and gender at the time of the study. If the disease process is to be induced prior to intervention, this should be performed without knowledge of the subsequent intervention group.
Randomization is one of the most powerful methods of reducing selection bias. It can reduce the effect of unequal distribution of known and unknown characteristics between groups. As such, it is also effective in reducing random error. Practically, this can be performed using many techniques that include sealed envelopes and computerized randomization services. The researcher should be unaware of the randomization sequence. Randomization should occur at the time of intervention. The use of quasirandomization techniques based on study sequence or day of week (among others) offer less protection against bias. Block randomization can be used to ensure that groups remain balanced at the end of a predetermined number of participants (i.e., six or eight) to assist in resource utilization. This may be important if a procedure- or time-intensive assay was required by the protocol. Stratification and minimization are techniques used in the randomization procedure to account for known confounding characteristics, where it is extremely desirable to ensure equal distribution between groups. Decisions regarding stratification and minimization should be taken during the development of the protocol if particularly powerful confounders are recognized. The advice of biostatistician is recommended for these more advanced techniques of randomization because they can have significant implications on power calculations (as studies require to be powered to demonstrate differences between subgroups).
Jargon Simplified: Allocation Concealment
Allocation concealment is the practice of protecting the randomization sequence from prior knowledge to the participants or researchers. This could result from knowledge of random number tables used to generate the sealed envelopes. For this reason, the use of external randomization techniques (which are more accessible in days of Internet access) or sequential numbering of sealed envelopes to prevent tampering with the sequence are used to prevent selection bias.
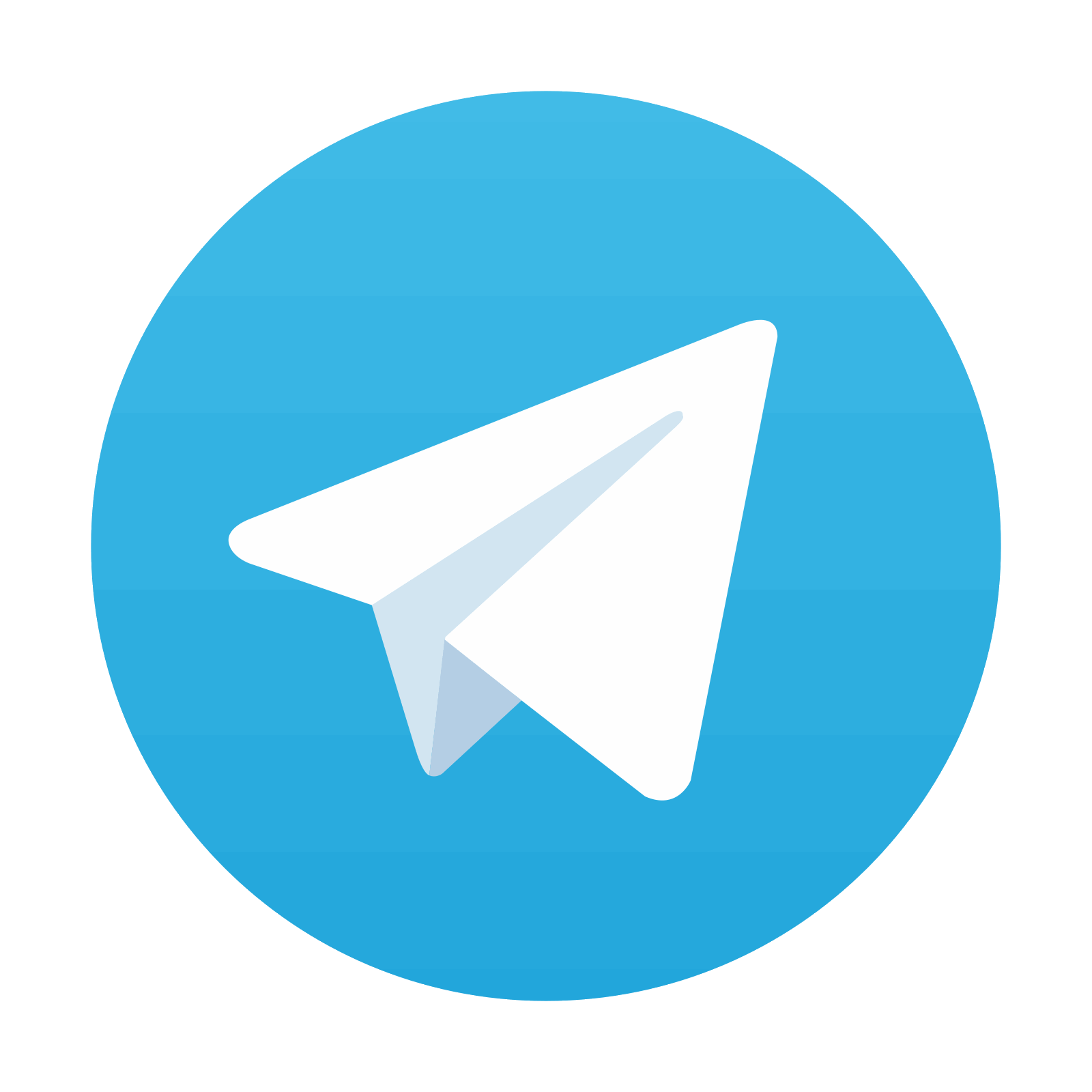
Stay updated, free articles. Join our Telegram channel

Full access? Get Clinical Tree
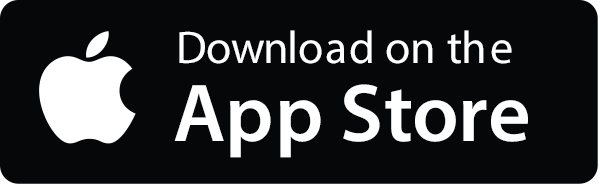

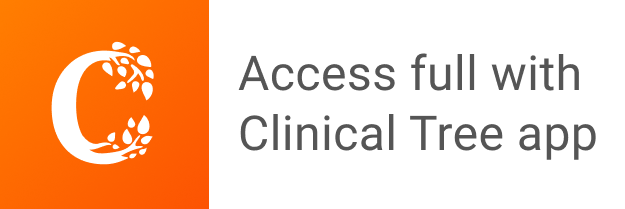