Abstract
Mediation analysis is a useful research method that potentially allows identification of the mechanisms through which treatments affect patient outcomes. This chapter reviews the theoretical framework, research designs and statistical approaches used in mediation analysis. It describes what can be learnt from previous mediation research, much of which has investigated mediating factors of psychosocial interventions in other health conditions. It also summarises the few treatment-mediation studies of psychosocial interventions conducted in back pain.
This chapter shows that there is emerging evidence about the role of some psychological factors as potential treatment mediators, such as self-efficacy and catastrophising. Mediation analysis can equally be applied to non-psychological factors. Pre-planned and appropriately conducted mediation analysis in adequately powered clinical trials would be a step forward in understanding treatment effects in back pain and improving patient management.
Introduction
A recurring conclusion of high-quality systematic reviews and randomised controlled trials (RCTs) in the field of back pain is that most treatments show modest effects compared to natural course and small or no differences between the effectiveness of different interventions . This leads to equivocal and sometimes contradictory messages in clinical practice guidelines and frustration in clinicians attempting to provide evidence-based care for their patients. In part, the underwhelming results reported by these studies are likely due to incomplete understanding of what factors might be necessary to be included in interventions to help influence outcome. Studies have examined the physical , psychological and social aspects of interventions to try to identify these factors. To date however, satisfying answers remain elusive.
Mediation analysis offers a method of testing theories regarding the causal links between a predictor and an outcome. The establishment of causal mechanisms as opposed to simply associative links is critical to the understanding of the processes of treatment effect. Mediation analysis can be applied to data from various types of study designs: cross-sectional surveys, clinical registries, longitudinal cohorts and randomised and non-randomised clinical trials. While different study designs impose different restrictions on the explanatory power of mediation analysis, this flexibility makes mediation analysis a useful supplement to other, more commonly used, methods of analysis.
Mediation analysis tests whether the influence of a predictor or treatment on an outcome occurs via change in a particular intermediate variable, the mediator. For example, a treatment could aim to influence fear-avoidant behaviours, which if successfully changed could be responsible for change in outcomes such as disability or pain intensity. Where the predictor variable is a ‘clinical feature’ associated with a condition, such as pain intensity or psychological distress, mediation analysis helps us understand the pathway between it and the outcome of interest. This information can be very useful in identifying factors that should be targeted in treatment. Where the predictor is a ‘specific treatment’, such as allocation to a particular treatment arm in an RCT, mediation analysis provides insight into whether the effectiveness or ineffectiveness of a treatment is consistent with theories regarding its mechanism.
Currently in the management of back pain, many interventions are based on imprecise theoretical rationales, rather than empirically derived hypotheses. It is potentially very useful to disentangle factors merely associated with outcome from those that could potentially help to explain treatment effects. Making distinctions between causes, consequences and epiphenomena is vital, as treatments that target factors that are not modifiable or influential are unlikely to be successful. For example, anxiety has been shown to be predictive of poor recovery from low back pain (LBP) and is commonly associated with pain. However, a recent mediation study showed that the relationship between pain and disability in patients with LBP is not mediated by anxiety. This suggests that a treatment designed to only target anxiety in LBP patients would be unlikely to have an important effect on pain-related disability.
Identifying the mechanism of action of a particular treatment offers the opportunity to optimise its effectiveness. Investigation of the relationship between an intervention and its effect via mediation analysis can provide information as to whether and to what extent the hypothesised action is real. This information can be used to modify the intervention in order to target the appropriate mediating factor more directly and enhance the capacity of the treatment to reach its full potential effect.
To date, relatively few mediation analyses have been conducted in back pain research despite such studies being potentially capable of providing important insight into questions relevant to the field. The aim of this article is to introduce the theory and practice of mediation analysis and discuss some of the issues involved with study design, conduct and interpretation.
What is a mediator?
It is important to define what we mean by the term ‘mediator’ along with some other, related terms, as these terms can have slightly different meanings in different fields and this can be a source of confusion.
Mediators, also known as intermediate variables or indirect effects , are variables that help explain how a treatment might work and are by definition on the pathway between predictor and outcome . As illustrated in Fig. 1 , the total effect of treatment on outcome can be separated into the direct effect and indirect (mediated) effect. Mediated effects differ from the direct effect of treatment in that the mediated effect combines the paths from the treatment to the mediator and from the mediator to the outcome. Mediators are modifiable and change during the course of, or in response to, treatment. For example, Fig. 1 shows that an intervention designed to reduce disability in back pain patients may work by reducing their levels of fear avoidance. The intervention causes fear avoidance to reduce, which results in improved function.

It is important to note the distinction between a mediator and a confounder. Confounders may explain the relationship between two variables but are not on a supposed causal pathway between them . For example, in an observational study including different treatment options, patients referred for an exercise programme may be younger whereas those offered pain relief may be older. Functional outcome may turn out to be better in those offered the exercise programme. However, age is not a mediator of treatment effect as it cannot be changed by treatment, even though it may be causally associated with function. In this case, age is a confounder due to the unequal distribution of age between the two groups.
It is equally important to distinguish between a mediator and a treatment-effect modifier or moderator variable. A moderator is a baseline characteristic that stratifies treatment effect (i.e., identifies for whom a treatment is likely to have the most effect) but is not on a supposed causal pathway. For example, status on a prediction rule at baseline has been reported to predict outcome in response to manual therapy. This does not necessarily mean that the features measured by the rule (e.g., hip range of motion, fear–avoidance beliefs and duration of symptoms) ‘cause’ the person’s disability or are all addressed by the treatment.
It is important to note that while Fig. 1 denotes a single-mediator model, this is unlikely to be the case in reality. It is much more likely that several mediators exist on a pathway between the predictor or treatment and the outcome (e.g., confidence in their clinician or the prescription of pain medication might help to reduce the patient’s fear avoidance, leading to an improvement in functioning). However, for simplicity, we only discuss examples of single-mediator models in this chapter.
Mediation across different study designs
Regardless of the way mediation is defined or how it is statistically analysed, it is described using causal language . The concept of causality has a number of facets, but in mediation analysis, those most commonly discussed are temporality, consistency and dose–response . It should be noted, however, that although addressing these facets allows us to be more confident that a causal association is present, this can never be fully established .
Temporality is the sequence in which variables change. In order for one variable to be potentially causing another, it must precede the proposed outcome in time . It has been suggested that establishing temporality is less complicated in the context of an RCT compared to observational study designs. This is because an RCT has a clear ‘start’ and ‘end’ point to the intervention and any given variable that changes during or in response to treatment, and is associated with that treatment, is a potential mediator . However, in order to truly establish a mediating pathway, we also need to consider how many assessments are required to establish temporality and when these assessments should take place. For example, we might expect an intervention to have an effect on patients very quickly so that we need to assess them early on to pick up any change, or perhaps we expect the intervention will take some time to have an impact so that we need to carry out assessments over a longer period of time. The more assessments that are included, the more confident we can be about the order in which variables change , although this does require the use of increasingly complex modelling procedures. It also increases patient burden as they will have to complete a larger number of questionnaires, examination procedures and/or biomedical tests.
Consistency is the observation of similar findings across multiple studies . As with other study designs, the replication of findings from mediation studies in different settings and samples increases confidence in their accuracy. Dose–response is the observation of increasing or decreasing effect on outcome depending upon the level of (change in) the mediator . These two aspects indicate that establishing mediating effects in different study designs, in different contexts and in different populations provides more convincing evidence for specific mediating factors.
Most research examining mediation has been performed in cross-sectional studies and therefore is of limited usefulness because temporal precedence cannot be established . However, finding an association between the potential mediator and the outcome might be an important first step in establishing consistency. Studies that include longitudinal analyses are much more useful from a mediation perspective as they allow the investigation of associations between variables over time, helping to establish temporality. Longitudinal designs can also provide some information on dose–response by investigating whether there is an association between the magnitude of change in the potential mediator and magnitude of change in the outcome. However, neither design allows causal mechanisms to be established. Clinical trial designs have several advantages over observational study methods for providing evidence for causality and reducing bias , such as active manipulation of the potential mediator, which lends more credence to any mediating effects found in the treatment arm (yet not in a control arm) and also helps to best establish dose–response. These factors help bring us closer to our goal in mediation: that of finding a causal pathway that explains the link between the predictor (independent) variable (in this case, the intervention) and the outcome (dependent) variable.
In summary, while mediating factors can be explored using a variety of study designs, RCTs have the potential to provide the most robust answers about how treatments work. However, observational studies and other research designs may be useful in developing testable and empirically based hypotheses about treatment mediation. Identification of causal mechanisms is strengthened by the plausibility of mechanisms based on previous evidence, consistency of findings with those from preceding studies and the demonstration of dose–response.
Action Theory and Conceptual Theory
Action Theory and Conceptual Theory provide a framework for understanding and interpreting mediation analyses in controlled trials. Action Theory refers to whether the intervention potentially causes significant change in the mediator, and Conceptual Theory refers to whether the outcome of interest is affected by change in the mediator (see Fig. 1 ) . Action Theory addresses the question of whether the intervention is actually effective according to its theorised mechanism . Conceptual Theory provides information regarding whether the rationale upon which the treatment is based is valid. Chen explains that a weak (or absent) relationship between the intervention and the mediator would suggest that the intervention is not being implemented in the optimum way (Action Theory), whereas if the relationship between the mediator and outcome was weak or non-existent, this would suggest the theory or concept underlying the intervention is the problem (Conceptual Theory). Thus, the relationships (or lack thereof) observed in both parts of the model provide useful and interpretable information.
What is a mediator?
It is important to define what we mean by the term ‘mediator’ along with some other, related terms, as these terms can have slightly different meanings in different fields and this can be a source of confusion.
Mediators, also known as intermediate variables or indirect effects , are variables that help explain how a treatment might work and are by definition on the pathway between predictor and outcome . As illustrated in Fig. 1 , the total effect of treatment on outcome can be separated into the direct effect and indirect (mediated) effect. Mediated effects differ from the direct effect of treatment in that the mediated effect combines the paths from the treatment to the mediator and from the mediator to the outcome. Mediators are modifiable and change during the course of, or in response to, treatment. For example, Fig. 1 shows that an intervention designed to reduce disability in back pain patients may work by reducing their levels of fear avoidance. The intervention causes fear avoidance to reduce, which results in improved function.
It is important to note the distinction between a mediator and a confounder. Confounders may explain the relationship between two variables but are not on a supposed causal pathway between them . For example, in an observational study including different treatment options, patients referred for an exercise programme may be younger whereas those offered pain relief may be older. Functional outcome may turn out to be better in those offered the exercise programme. However, age is not a mediator of treatment effect as it cannot be changed by treatment, even though it may be causally associated with function. In this case, age is a confounder due to the unequal distribution of age between the two groups.
It is equally important to distinguish between a mediator and a treatment-effect modifier or moderator variable. A moderator is a baseline characteristic that stratifies treatment effect (i.e., identifies for whom a treatment is likely to have the most effect) but is not on a supposed causal pathway. For example, status on a prediction rule at baseline has been reported to predict outcome in response to manual therapy. This does not necessarily mean that the features measured by the rule (e.g., hip range of motion, fear–avoidance beliefs and duration of symptoms) ‘cause’ the person’s disability or are all addressed by the treatment.
It is important to note that while Fig. 1 denotes a single-mediator model, this is unlikely to be the case in reality. It is much more likely that several mediators exist on a pathway between the predictor or treatment and the outcome (e.g., confidence in their clinician or the prescription of pain medication might help to reduce the patient’s fear avoidance, leading to an improvement in functioning). However, for simplicity, we only discuss examples of single-mediator models in this chapter.
Mediation across different study designs
Regardless of the way mediation is defined or how it is statistically analysed, it is described using causal language . The concept of causality has a number of facets, but in mediation analysis, those most commonly discussed are temporality, consistency and dose–response . It should be noted, however, that although addressing these facets allows us to be more confident that a causal association is present, this can never be fully established .
Temporality is the sequence in which variables change. In order for one variable to be potentially causing another, it must precede the proposed outcome in time . It has been suggested that establishing temporality is less complicated in the context of an RCT compared to observational study designs. This is because an RCT has a clear ‘start’ and ‘end’ point to the intervention and any given variable that changes during or in response to treatment, and is associated with that treatment, is a potential mediator . However, in order to truly establish a mediating pathway, we also need to consider how many assessments are required to establish temporality and when these assessments should take place. For example, we might expect an intervention to have an effect on patients very quickly so that we need to assess them early on to pick up any change, or perhaps we expect the intervention will take some time to have an impact so that we need to carry out assessments over a longer period of time. The more assessments that are included, the more confident we can be about the order in which variables change , although this does require the use of increasingly complex modelling procedures. It also increases patient burden as they will have to complete a larger number of questionnaires, examination procedures and/or biomedical tests.
Consistency is the observation of similar findings across multiple studies . As with other study designs, the replication of findings from mediation studies in different settings and samples increases confidence in their accuracy. Dose–response is the observation of increasing or decreasing effect on outcome depending upon the level of (change in) the mediator . These two aspects indicate that establishing mediating effects in different study designs, in different contexts and in different populations provides more convincing evidence for specific mediating factors.
Most research examining mediation has been performed in cross-sectional studies and therefore is of limited usefulness because temporal precedence cannot be established . However, finding an association between the potential mediator and the outcome might be an important first step in establishing consistency. Studies that include longitudinal analyses are much more useful from a mediation perspective as they allow the investigation of associations between variables over time, helping to establish temporality. Longitudinal designs can also provide some information on dose–response by investigating whether there is an association between the magnitude of change in the potential mediator and magnitude of change in the outcome. However, neither design allows causal mechanisms to be established. Clinical trial designs have several advantages over observational study methods for providing evidence for causality and reducing bias , such as active manipulation of the potential mediator, which lends more credence to any mediating effects found in the treatment arm (yet not in a control arm) and also helps to best establish dose–response. These factors help bring us closer to our goal in mediation: that of finding a causal pathway that explains the link between the predictor (independent) variable (in this case, the intervention) and the outcome (dependent) variable.
In summary, while mediating factors can be explored using a variety of study designs, RCTs have the potential to provide the most robust answers about how treatments work. However, observational studies and other research designs may be useful in developing testable and empirically based hypotheses about treatment mediation. Identification of causal mechanisms is strengthened by the plausibility of mechanisms based on previous evidence, consistency of findings with those from preceding studies and the demonstration of dose–response.
Action Theory and Conceptual Theory
Action Theory and Conceptual Theory provide a framework for understanding and interpreting mediation analyses in controlled trials. Action Theory refers to whether the intervention potentially causes significant change in the mediator, and Conceptual Theory refers to whether the outcome of interest is affected by change in the mediator (see Fig. 1 ) . Action Theory addresses the question of whether the intervention is actually effective according to its theorised mechanism . Conceptual Theory provides information regarding whether the rationale upon which the treatment is based is valid. Chen explains that a weak (or absent) relationship between the intervention and the mediator would suggest that the intervention is not being implemented in the optimum way (Action Theory), whereas if the relationship between the mediator and outcome was weak or non-existent, this would suggest the theory or concept underlying the intervention is the problem (Conceptual Theory). Thus, the relationships (or lack thereof) observed in both parts of the model provide useful and interpretable information.
Mediation analysis: how is it done?
Review of statistical approaches to mediation analysis
A number of different approaches to conducting mediation analysis have been proposed. MacKinnon et al. identified 14 different methods, which they grouped into three different approaches: causal steps, difference in coefficients and product of coefficients.
- 1.
Causal steps’ approach
These methods seek to test different links in a chain of variables in which the order is assumed to be causal . They involve using multiple regression analysis to test each of the paths illustrated in Fig. 1 , and each path must be statistically significant. In the context of an RCT, the test of mediation occurs when the potential mediating factor is included in the model with the independent (treatment) and dependent (outcome) variables. If the relationship between treatment and outcome is no longer significant or is substantially reduced when the potential mediator is included, a mediation effect is identified . The contribution of the mediating variable to the relationship between the treatment and outcome is sometimes estimated by the proportional change that occurs in the relationship between treatment and outcome when the mediator is introduced to the model .
However, these approaches are criticised in the literature . The requirement for the treatment-outcome path to be statistically significant has been contested by a number of authors as it reduces the approach’s power to detect mediating effects and is highly dependent on sample size; hence, larger studies are more likely to show significant results . Further, only performing mediation analysis in studies where there is a treatment effect precludes research into why treatments ‘do not’ work.
Overall, the review by MacKinnon et al. of all 14 methods concluded that the causal steps’ approach was the least optimal test of mediation. Although some researchers still advocate their use in certain contexts (e.g., Ref. ), they appear to have been superseded by more sophisticated methods.
- 2.
Difference in coefficients’ approach
This approach examines the difference between regression coefficients before and after the inclusion of a potential mediating variable . Conceptually, this is similar to the causal steps’ approach but the statistical equations used are different (see MacKinnon et al. or Hayes for a detailed discussion). This approach has been found to have more power but MacKinnon’s review found the method to be still susceptible to Type II errors (a true mediation effect is missed) and useful only when testing a single mediating factor. As a single-mediator model is often too simplistic to suitably account for real-world situations, the usefulness of this approach is limited.
- 3.
Product of coefficients’ approach
This final approach involves multiplying the regression coefficients of the proposed mediating pathways (paths a and b in Fig. 1 ) to estimate the amount of variance in the outcome that is explained by the treatment through the mediator . Sobel extended this method to allow determination of its statistical significance, by dividing the product of coefficients by its standard error and comparing that value to a normal distribution.
However, the Sobel test assumes that the mediated effect is normally distributed , which often may not be the case. Several methods have been proposed to deal with this, (see Ref. ) the most promising of which is the statistical technique known as bootstrapping . Bootstrapping does not assume normal distribution and allows the generation of bias-corrected confidence intervals (CIs) around the mediation effect. It can also be applied to smaller samples , which helps to resolve the problem of inadequate power often encountered in mediation analysis studies. MacKinnon and colleagues argue that this approach should be the preferred method for investigating mediation effects.
Statistical methods for testing mediation
To perform the analyses described above, linear regression or structural equation modelling (SEM) techniques are most often used . Linear regression is simple to use and perhaps more familiar to researchers but makes strong assumptions about the data that are not always satisfied. For example, the assumption of no measurement error in the tools used to assess the variables of interest is difficult to sustain in the investigation of psychological factors where the variables being examined are latent and therefore not directly measurable. Measurement error is a particular problem when using regression analysis for mediation, as we are trying to establish whether change in a particular factor is responsible for change in the outcome. Measures need to be highly accurate when measuring change, as this involves at least two measurements, each with its own error .
Although more complex, SEM is viewed as a better choice of statistical technique for mediation analysis. SEM is a combination of regression analysis and factor analysis and, while making many of the same assumptions about the data, it handles the inclusion of several mediating factors more readily, it can include latent (unobserved) factors and it can account for measurement error . SEM also provides goodness-of-fit statistics that allow comparisons between tested models . This technique does however require larger sample sizes than traditional regression analysis .
More recent developments in mediation analysis methods include latent growth modelling (LGM), performed within SEM . This method allows the inclusion of several time points for the mediator and the outcome variable and also allows the modelling of the change that occurs within an individual (within-subject effects) rather than only change between different people (between-subject effects) .
Overall, this literature suggests that a product of coefficients approach performed using SEM, with bootstrapped CIs, is currently the most effective method for addressing the assumptions made when performing mediation analysis. However, while these more complex statistical techniques are necessary in order for us to gauge more accurately the mediating effect of particular variables, the results may be more difficult to interpret and more difficult to implement in clinical settings. It is important that the right balance is struck between the integrity of the analysis performed and its practical application.
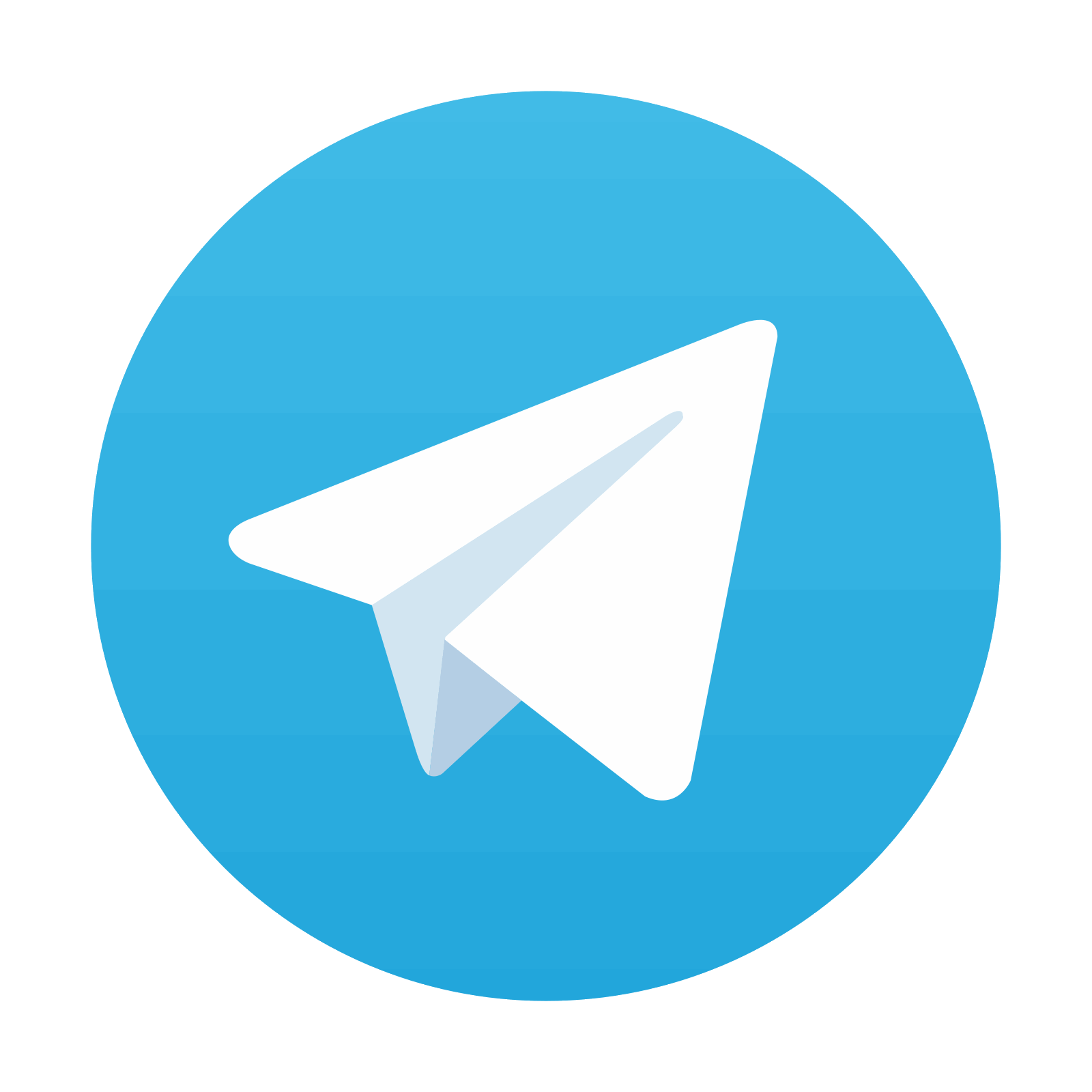
Stay updated, free articles. Join our Telegram channel

Full access? Get Clinical Tree
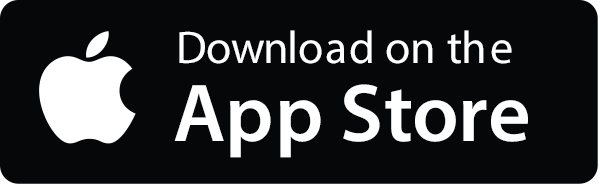
