Meta-analysis was introduced and promoted on the basis of approximated assumptions, leading to straightforward application and wide dissemination among users of diverse statistical knowledge. However, the credibility of meta-analysis results is inextricably linked to the plausibility of the underlying assumptions. Accumulated evidence from simulation studies has highlighted the shortcomings of the approximated assumptions in certain settings. , This column introduces the readers to 2 schools of meta-analysis models, the 2-stage and the 1-stage. We discuss the advantages and disadvantages of these models and exemplify their application using a real-life meta-analysis.
Two-stage meta-analysis
The reader is already familiar with this model, probably not under this nomenclature. The data of this model comprise calculated effect measures , such as odds ratio in logarithmic scale (log OR) for a binary outcome or mean difference for a continuous outcome, alongside their standard errors for each study. During the first stage, the systematic review authors usually extract the raw aggregate outcome data from the study reports, convert them into the effect measure of interest (eg, log OR), and calculate the corresponding variance, <SPAN role=presentation tabIndex=0 id=MathJax-Element-1-Frame class=MathJax style="POSITION: relative" data-mathml='si2′>π 2πsi2
s i 2
, for each study <SPAN role=presentation tabIndex=0 id=MathJax-Element-2-Frame class=MathJax style="POSITION: relative" data-mathml='i’>πi
i
. Then, in the second stage, these data are synthesized using the inverse-variance approach under a meta-analysis of fixed or random effects. Mantel-Haenszel and Peto OR are special cases of the 2-stage fixed-effect meta-analysis for binary outcomes advocated for synthesizing studies with sparse outcomes.
Typically, in the 2-stage meta-analysis, the estimated within-study variances are assumed to approximate the population variances, <SPAN role=presentation tabIndex=0 id=MathJax-Element-3-Frame class=MathJax style="POSITION: relative" data-mathml='σi2′>π2πΟi2
Ο i 2
, and hence, they are treated as fixed ; that is, the uncertainty in estimating the parameter <SPAN role=presentation tabIndex=0 id=MathJax-Element-4-Frame class=MathJax style="POSITION: relative" data-mathml='σi2′>π2πΟi2
Ο i 2
is ignored. The same holds for the estimated between-study variance, <SPAN role=presentation tabIndex=0 id=MathJax-Element-5-Frame class=MathJax style="POSITION: relative" data-mathml='τ2′>π2Ο2
Ο 2
, under the random-effects meta-analysis. Because the inverse-variance approach contains at least 1 of these variances ( <SPAN role=presentation tabIndex=0 id=MathJax-Element-6-Frame class=MathJax style="POSITION: relative" data-mathml='si2′>π 2πsi2
s i 2
in the fixed-effect meta-analysis, but <SPAN role=presentation tabIndex=0 id=MathJax-Element-7-Frame class=MathJax style="POSITION: relative" data-mathml='si2′>π 2πsi2
s i 2
and <SPAN role=presentation tabIndex=0 id=MathJax-Element-8-Frame class=MathJax style="POSITION: relative" data-mathml='τ2′>π2Ο2
Ο 2
in the random-effects meta-analysis), the meta-analysis weights are also treated as fixed. These approximations greatly simplify the synthesis of the studies, but they can compromise the credibility of the results when the necessary conditions are not fulfilled.
One-stage meta-analysis
The 1-stage meta-analysis has received more attention lately for being more flexible and performing better than the 2-stage model when approximations are difficult to defend. The data for the 1-stage model constitute raw aggregate outcome data for each arm of every study. Then, a regression is conducted that aligns with the exact distribution of the outcome data. For instance, for binary outcomes, the extracted data are aggregate, comprising the number of events for the experimental and control arms, which follow a binomial distribution for the corresponding number of randomized participants. Then, a mixed-effects logistic regression with a logit link function is performed, corresponding to a random-effects meta-analysis for log OR. Hence, all studies are synthesized in a single stage, avoiding any approximate assumptions about the effect estimates and their standard error. Jackson et al examined various 1-stage random-effects meta-analysis models for OR on the basis of the family of generalized linear mixed models as viable alternatives to the traditional 2-stage random-effects meta-analysis.
Plausibility of approximations in orthodontic research and implications
The 2-stage model relies on normality approximation at the study level to justify calculating each studyβs effect size and variance at the first stage. , The formula to calculate the variance of most effect measures is based on the delta method that relies on asymptotic normality. Consequently, the estimated within-study variances are assumed to approximate the population variance and are treated as fixed. However, the included studies must be sufficiently large to defend asymptotic normality and allow the 2-stage meta-analysis to provide reliable results. In orthodontic research, the sample size requirements and the number of synthesized studies are often insufficient to demonstrate the normality approximation. , Failure to approximate normality at the first stage will implicate the quality of results in the second stage, increasing the risk of biased summary effect size and type I error inflation, with the latter also resulting from treating <SPAN role=presentation tabIndex=0 id=MathJax-Element-9-Frame class=MathJax style="POSITION: relative" data-mathml='si2′>π 2πsi2
s i 2
and <SPAN role=presentation tabIndex=0 id=MathJax-Element-10-Frame class=MathJax style="POSITION: relative" data-mathml='τ2′>π2Ο2
Ο 2
as spuriously fixed. Note that <SPAN role=presentation tabIndex=0 id=MathJax-Element-11-Frame class=MathJax style="POSITION: relative" data-mathml='τ2′>π2Ο2
Ο 2
requires a sufficient number of studies to be estimated accurately, and some estimators of <SPAN role=presentation tabIndex=0 id=MathJax-Element-12-Frame class=MathJax style="POSITION: relative" data-mathml='τ2′>π2Ο2
Ο 2
rely on normality approximations at the first stage.
Another issue of the 2-stage approach pertains to binary outcomes in which the estimated effect size (eg, log OR) and variance at the study level are inherently correlated. The 2-stage approach does not account for this correlation. Consequently, the summary effect may be underestimated or overestimated depending on whether the correlation is positive or negative. This inherent correlation may also manifest as spurious evidence of a small study effect. Using arbitrary corrections to address zero events is another well-known shortcoming of the 2-stage approach with implications for the credibility of the summary results. , The 1-stage approach avoids the issues related to such correlation and zero-event corrections. Jackson et al delve further into the discussion of hidden normality assumptions and juxtapose the 2-stage and 1-stage models.
The 1-stage model has been advocated when synthesizing small studies or sparse outcomes for relying on the exact distribution of the outcome data. , However, 1-stage models are also prone to shortcomings, including converging issues and computation requirements, making it impossible to recommend a single 1-stage model for general use. Jackson et al advised adopting a principled analysis, in which a specific 1-stage model constitutes the primary analysis, and a carefully selected set of other 1-stage models comprise the sensitivity analysis, including the 2-stage model. The involvement of a biostatistician is imperative to ensure proper design and execution of the principled analysis. Such an analysis plan is essential in the presence of small studies, in which normality approximations will likely not be met, rendering the 2-stage meta-analysis model potentially inappropriate.
Application to real data
We will illustrate the 2-stage (normal approximations) and 1-stage (exact binomial distribution) models for a random-effects meta-analysis on a binary outcome using the systematic review of 12 studies on implant failure in nonperforated vs perforated sinus membranes. The Table presents the raw outcome data for each arm in every study. Fewer events are better; hence, an OR of <1 favors nonperforated sinus membranes. Both arms have low events, ranging 0.00%-13.64% in nonperforated sinus membranes and 0.00%-23.81% in perforated ones. Furthermore, the sample size of studies is substantially variable (range, 32-1588). Both conditions do not favor normality approximation; thus, conducting a 2-stage model may yield potentially questionable results, and it would be important to compare the results with those of the 1-stage model. Both models were applied using the metafor R package (R software, version 4.3.3; R Core Team, Vienna, Austria): the rma.uni function was used for the 2-stage model, and the rma.glmm function with mixed-effects conditional logistic regression with an approximate likelihood (model = βCM.ALβ) was employed for the 1-stage model. A mixed-effects conditional logistic regression with an exact likelihood (model = βCM.ELβ) can also be employed; however, it can be computationally laborious (the model converged in 10.13 seconds in our example). The between-study variance was estimated using the maximum likelihood estimator in both models. The ggplot2 R package was used to create the forest plot.
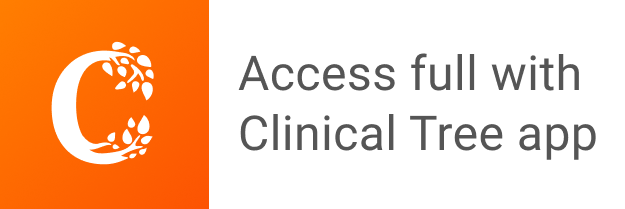