There has been a recent increase in research evaluating treatment-based subgroups of non-specific low back pain. The aim of these sub-classification schemes is to identify subgroups of patients who will respond preferentially to one treatment as opposed to another. Our article provides accessible guidance on to how to interpret this research and determine its implications for clinical practice. We propose that studies evaluating treatment-based subgroups can be interpreted in the context of a three-stage process: (1) hypothesis generation–proposal of clinical features to define subgroups; (2) hypothesis testing–a randomised controlled trial (RCT) to test that subgroup membership modifies the effect of a treatment; and (3) replication–another RCT to confirm the results of stage 2 and ensure that findings hold beyond the specific original conditions. At this point, the bulk of research evidence in defining subgroups of patients with low back pain is in the hypothesis generation stage; no classification system is supported by sufficient evidence to recommend implementation into clinical practice.
For about 3 decades, the position adopted in evidence-based treatment guidelines has been that the source of pain cannot be determined for most patients (up to 90%) presenting to primary care with low back pain (LBP). Most guidelines recommend that such patients be assigned to the classification ‘non-specific LBP’ and be provided with generic treatment. Recently, there has been some reconsideration of this position and it has been suggested that it may be better to divide patients with non-specific LBP into treatment-based subgroups that inform the choice of specific treatment for that individual . Importantly, this is also the position adopted by many clinicians who use a subgroup approach to direct treatment .
Some subgroups are based to some extent on putative pathoanatomy , while others are based on clinical findings such as psychosocial characteristics (or yellow flags) or characteristic patterns of signs and symptoms . What unifies most schemes is an underlying belief that the effect of treatment will be greater when patients receive the specific treatment that matches their subgroup. Proponents of treatment-based subgroups argue that this approach offers the possibility of much larger treatment effects than are typically observed after applying generic treatments to all patients with non-specific LBP. The argument is that mean group treatment effects may be diluted by the inclusion of subgroups of LBP subjects for whom the treatment is not effective . If treatment-based subgroups could be reliably identified, it would represent an important advance in LBP treatment, and the pursuit of this goal has been identified as a priority for LBP researchers .
The aim of this article is to illustrate the key methodological issues in this area, provide clinicians with a better understanding of the literature in LBP and thus present implications for clinical practice and future research. We begin by defining some key concepts and then describe the process to identify and test the existence of LBP subgroups which respond differently to a treatment. We conclude with a brief summary of the state of evidence so far in relation to subgroups of subjects with LBP.
Key concepts
Treatment effect modification
The effect of treatment is the difference in outcome between the treatment and control groups. A system for treatment-based subgroups needs to reliably identify patients where the effect of treatment is consistently greater than it would be for the whole group. A characteristic that defines the subgroup, for example, gender or high pain intensity, is described as a treatment effect modifier. Subgroups may be defined by the presence of one or several effect modifiers.
The potential for treatment-based subgroups is often justified by reference to the variability of patient outcomes observed in clinical practice and also within the treatment arm of clinical trials. However, variability in treatment outcomes can arise for reasons other than treatment effect modification. For example, variability in outcomes can be due to patients having variable prognoses (regardless of treatment) or because of random variation in a patient’s response to treatment. Variability in outcome due to either of these reasons would not contribute to defining a subgroup of patients for which the effect of treatment is consistently greater. What is required is treatment effect modification where subgroups of patients reliably exhibit greater effects of treatment.
Distinguishing treatment effect modifiers and prognostic factors
It is important to distinguish between factors predictive of patient outcomes (prognostic factors) and those that predict treatment effects (treatment effect modifiers). Prognostic factors relate to the susceptibility of a patient’s condition to time, while treatment effect modifiers relate to the suscetibility of their condition to a specific treatment. An important point is that single-arm studies cannot quantify treatment effects (difference in outcomes between experimental and control groups) and so cannot identify treatment effect modifiers. Clinically, there may be value in identifying patients with good prognoses; this information may be used to reassure patients and can limit the implementation of unnecessary interventions. However, recognition of the difference between the two concepts is crucial.
Illustrative example: A single-arm study incorrectly interpreted as providing evidence of effect modification .
Predicting response of patients with neck pain to cervical manipulation . Tseng and colleagues conducted a prospective cohort study on 100 patients with neck pain, all of whom received cervical manipulation . Outcome was assessed with subjective global improvement or changes in pain rating and patients were classified as ‘responders’ or ‘non-responders’ based on these variables. The authors used regression analyses to identify baseline demographic and clinical characteristics associated with outcome. They reported that the following factors predicted the outcome: low disability score, bilateral symptoms, not performing sedentary work, feeling better while moving, not feeling worse when extending the neck and diagnosis of spondylosis without radiculopathy. Their conclusion, however, that these variables predict response to treatment (cervical manipulation) is not supported. While this study does enable us to identify factors associated with a favourable prognosis, we do not know that it is the effect of manipulation that drives the improved outcome for patients with these characteristics.
While prognostic factors and treatment effect modifiers may overlap in some instances , in other cases they do not . More importantly there are examples where the same factor predicts a favourable response to treatment although an unfavourable response to time. For example, in Stewart’s study of whiplash , high baseline pain predicted a greater response to exercise treatment (when compared with advice) but, by itself, high pain is an adverse prognostic factor for spinal pain . Accordingly, the use of single-arm studies to generate information on treatment effect modification is unwise .
Study design considerations
While there has been a sharp rise in the amount of research evaluating treatment-based subgroups, unfortunately, not all of it is methodologically sound. To establish that subgroup membership influences the effect of treatment, we need a design whereby patients are classified in one subgroup or another, and they receive the treatment or the control, represented by the four cells of a 2 × 2 table ( Fig. 1 a ). A well-known study that used this design is the Childs et al. trial, which reported that the effect of spinal manipulation was greater in those who were positive on a clinical prediction rule than in those who were negative. As shown in Fig. 1 b, the subjects in the trial could be divided into the four cells based upon the treatment they received and their rule status. A modified version of this approach compares the outcomes of patients who were randomised to receive treatment matched to their classification (subgroup) with patients who received treatment not matched to their classification. An example of this is the study of Long et al. where subjects with a directional preference were allocated to exercise in the matched direction, the opposite direction, or all directions. In this case the design can be represented in a 3 × 3 table ( Fig. 1 c); this more complex design is discussed further in a later section.

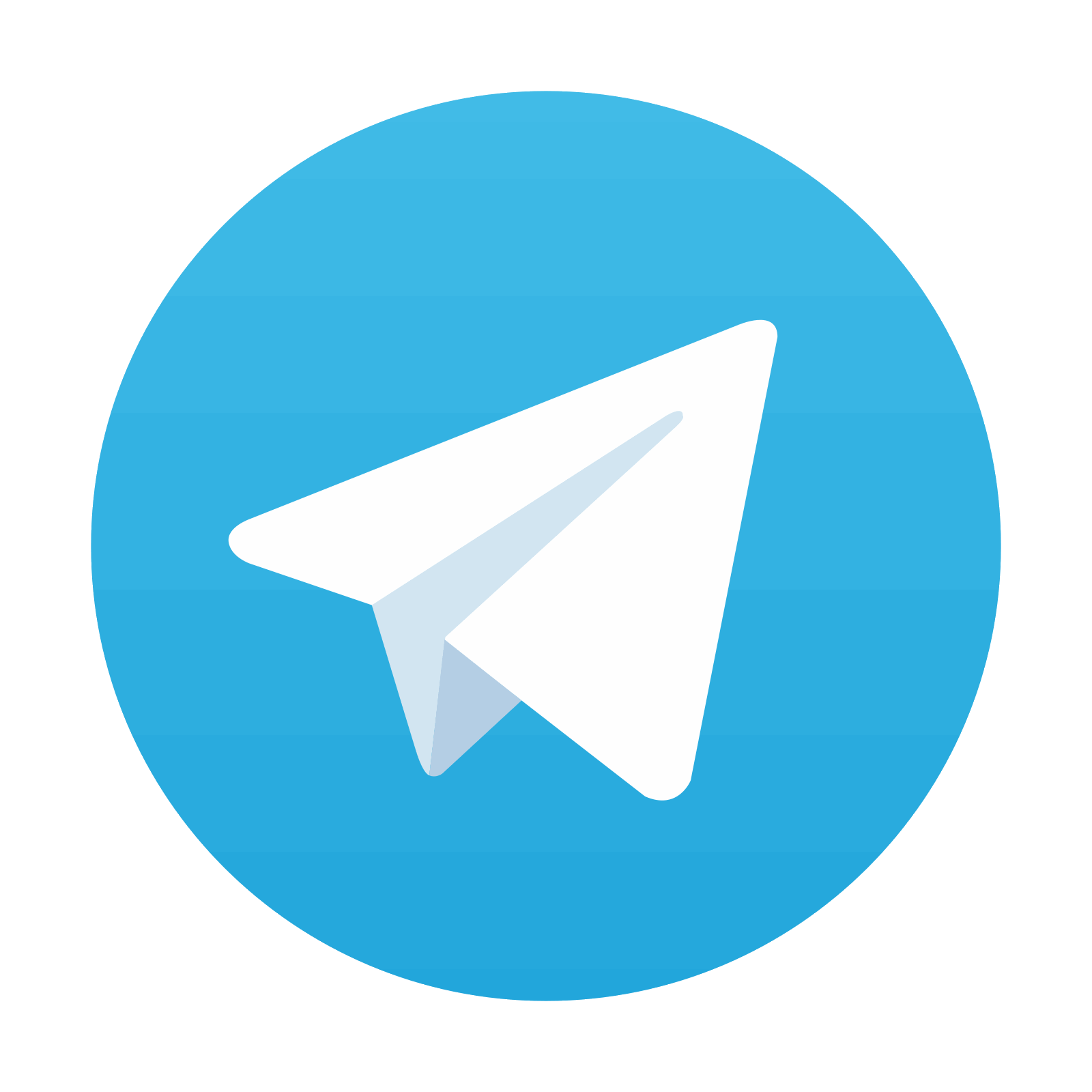
Stay updated, free articles. Join our Telegram channel

Full access? Get Clinical Tree
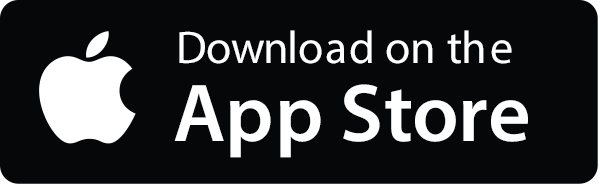

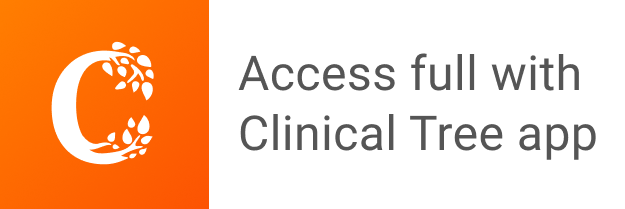