Abstract
In rheumatic diseases, classification criteria have been developed to identify well-defined homogenous cohorts for clinical research. Although they are commonly used in clinical practice, their use may not be appropriate for routine diagnostic clinical care. Classification criteria are being revised with improved methodology and further understanding of disease pathophysiology, but they still may not encompass all unique clinical situations to be applied for diagnosis of heterogenous, rare, evolving rheumatic diseases. Diagnostic criteria development is challenging primarily due to difficulty for universal application given significant differences in the prevalence of rheumatic diseases based on geographical area and clinic settings. Despite these shortcomings, the clinician can still use classification criteria for understanding the disease as well as a guide for diagnosis with a few caveats. We present the limits of current classification criteria, their use and abuse in clinical practice, and how they should be used with caution when applied in clinics.
Introduction
Rheumatology is not a field of black and white, but a specialty full of gray. Multisystem clinical syndromes and diseases in rheumatology attract clinicians and researchers who seek to unify different shades of “gray” into a single diagnosis or classification criteria. While understanding of the pathophysiology in each disease has advanced, single laboratory tests with high sensitivity and specificity sufficient to make a diagnosis still do not exist for most of the rheumatic diseases. As opposed to a positive blood culture in infectious disease suggestive of bacteremia or a fasting blood glucose in endocrinology suggestive of diabetes mellitus, even the most common and well-studied clinical conditions in rheumatology such as rheumatoid arthritis (RA) can have significant diagnostic uncertainty of so-called seronegativity up to 30% of the time . Despite making significant technological advances with diagnostic tests such as anti-cyclic citrullinated peptides (CCPs), diagnosis is still imperfect given the lack of 100% specificity for RA and, even worse, sensitivity . This diagnostic uncertainty has led to the development of multiple sets of disease classification criteria for use in research on disease characterization, epidemiology, prognosis, and design of clinical trials for therapeutic investigation . Although designed for clinical research, classification criteria are used and abused in clinical practice for patient care. This article will help define both classification and diagnostic criteria, and describe limitations of current classification criteria and how their use in clinical practice, while not sufficient alone for diagnosis, can be an aid or aide-mémoire in making a diagnosis.
Statistical principles
Prior to further discussion of classification and diagnostic criteria, a review of certain statistical principles is necessary to clarify differences between classification and diagnostic criteria. Sensitivity is the percentage of true positives with the disease. A highly sensitive test is useful for ruling out a disease with a negative test but not necessarily ruling in the disease. Conversely, specificity is the percentage of true negatives without disease, and it is useful for ruling in a positive test (if high specificity) but not necessarily ruling out a disease. In the setting of a highly sensitive and specific test, whereas sensitivity is easily understood (if you do not have the test positive, then the disease is not present), specificity leads to confusion because, rather than the focus being on having the disease, the focus is on not having the disease . Highly specific tests have low false-positive rates, and highly sensitive tests have low false-negative rates. For instance, anti-CCP antibodies have been shown to have a high, >90%, specificity for RA in established RA cohorts, whereas it has a moderate sensitivity of 66% . For knowing the true clinical applicability of sensitivity and specificity for a given test, the population in which it is studied or developed is important. For example, CCP is useful for ruling in RA in subjects with polyarthritis secondary to its high specificity in this particular population . Without knowing the population in which CCP specificity is attributed to, the meaning of the specificity is lost. For example, CCP is positive in many types of non-inflammatory arthritis including infections . Therefore, the sensitivity and specificity of any diagnostic or classification criteria are dependent on the reference gold standard used for its development as well as target population it is intended for. For example, the 2010 American College of Rheumatology (ACR)/European League Against Rheumatism (EULAR) RA classification criteria were developed for use on early RA cohorts and therefore not intended to be used on burned-out deforming nodular RA.
Sensitivity and specificity are on a continuum with an inverse relationship where perfect sensitivity (close to 100%) will lead to loss in specificity and vice versa. This is more evident in rheumatology where the sensitivity and specificity of any criteria depend on multiple disease variables . When one gold-standard test is used for diagnosis, as in acute gout or septic arthritis , both sensitivity and specificity can remain high. However, as the number of variables needed for a disease classification increase, that is, elevated C-reactive protein, number of swollen joints, and seropositivity, the specificity in classification criteria increases, but sensitivity decreases, and vice versa. The receiver operator curve (ROC) is the statistical and graphical description of this process showing the equilibrium between sensitivity and specificity . This same continuum is found when describing the sensitivity and specificity of any classification and/or diagnostic criteria .
Furthermore, in addition to the number of variables and continuum of sensitivity and specificity involved in the development of classification or diagnostic criteria, the ultimate performance of any classification or diagnostic criteria is highly dependent on the prevalence of the disease in the patient population being investigated . The principle of positive predictive value (PPV) illustrates this point. PPV is the proportion of true positives to the number of positive tests, and it is a measure of the accuracy or performance of a diagnostic test or, in the case of this discussion, diagnostic or classification criteria. Negative predictive value (NPV) is the opposite: a proportion of the number of true negatives to the number of negative tests. Both PPV and NPV are highly dependent on the prevalence of disease. For instance, the prevalence of Behçet’s disease in Turkey is almost 0.4% of the population, and in this population the international Behçet’s classification criteria have high sensitivity and specificity . In this population, the classification criteria can be used for diagnosis without significant numbers of false-positive classifications. However, if the same criteria were used outside of Turkey where Behçet’s is rare, while the sensitivity would remain the same, the specificity would decrease with increase in false positivity: the PPV of these classification criteria used for diagnosis would then plummet . With each change in individual population prevalence, the PPV of the test is dependent on the frequency of disease in the population being studied. The concept of frequency of the disease in a population affecting the utility of any criteria applies not only to geographical areas but also to clinical settings in which patients are being seen within the same geographical area. Be it a tertiary referral center for inflammatory myositis, a community rheumatology practice, or an acute care primary clinic, with each change in disease frequency, the usefulness of the test is determined by the PPV in that clinical setting or population.
Statistical principles
Prior to further discussion of classification and diagnostic criteria, a review of certain statistical principles is necessary to clarify differences between classification and diagnostic criteria. Sensitivity is the percentage of true positives with the disease. A highly sensitive test is useful for ruling out a disease with a negative test but not necessarily ruling in the disease. Conversely, specificity is the percentage of true negatives without disease, and it is useful for ruling in a positive test (if high specificity) but not necessarily ruling out a disease. In the setting of a highly sensitive and specific test, whereas sensitivity is easily understood (if you do not have the test positive, then the disease is not present), specificity leads to confusion because, rather than the focus being on having the disease, the focus is on not having the disease . Highly specific tests have low false-positive rates, and highly sensitive tests have low false-negative rates. For instance, anti-CCP antibodies have been shown to have a high, >90%, specificity for RA in established RA cohorts, whereas it has a moderate sensitivity of 66% . For knowing the true clinical applicability of sensitivity and specificity for a given test, the population in which it is studied or developed is important. For example, CCP is useful for ruling in RA in subjects with polyarthritis secondary to its high specificity in this particular population . Without knowing the population in which CCP specificity is attributed to, the meaning of the specificity is lost. For example, CCP is positive in many types of non-inflammatory arthritis including infections . Therefore, the sensitivity and specificity of any diagnostic or classification criteria are dependent on the reference gold standard used for its development as well as target population it is intended for. For example, the 2010 American College of Rheumatology (ACR)/European League Against Rheumatism (EULAR) RA classification criteria were developed for use on early RA cohorts and therefore not intended to be used on burned-out deforming nodular RA.
Sensitivity and specificity are on a continuum with an inverse relationship where perfect sensitivity (close to 100%) will lead to loss in specificity and vice versa. This is more evident in rheumatology where the sensitivity and specificity of any criteria depend on multiple disease variables . When one gold-standard test is used for diagnosis, as in acute gout or septic arthritis , both sensitivity and specificity can remain high. However, as the number of variables needed for a disease classification increase, that is, elevated C-reactive protein, number of swollen joints, and seropositivity, the specificity in classification criteria increases, but sensitivity decreases, and vice versa. The receiver operator curve (ROC) is the statistical and graphical description of this process showing the equilibrium between sensitivity and specificity . This same continuum is found when describing the sensitivity and specificity of any classification and/or diagnostic criteria .
Furthermore, in addition to the number of variables and continuum of sensitivity and specificity involved in the development of classification or diagnostic criteria, the ultimate performance of any classification or diagnostic criteria is highly dependent on the prevalence of the disease in the patient population being investigated . The principle of positive predictive value (PPV) illustrates this point. PPV is the proportion of true positives to the number of positive tests, and it is a measure of the accuracy or performance of a diagnostic test or, in the case of this discussion, diagnostic or classification criteria. Negative predictive value (NPV) is the opposite: a proportion of the number of true negatives to the number of negative tests. Both PPV and NPV are highly dependent on the prevalence of disease. For instance, the prevalence of Behçet’s disease in Turkey is almost 0.4% of the population, and in this population the international Behçet’s classification criteria have high sensitivity and specificity . In this population, the classification criteria can be used for diagnosis without significant numbers of false-positive classifications. However, if the same criteria were used outside of Turkey where Behçet’s is rare, while the sensitivity would remain the same, the specificity would decrease with increase in false positivity: the PPV of these classification criteria used for diagnosis would then plummet . With each change in individual population prevalence, the PPV of the test is dependent on the frequency of disease in the population being studied. The concept of frequency of the disease in a population affecting the utility of any criteria applies not only to geographical areas but also to clinical settings in which patients are being seen within the same geographical area. Be it a tertiary referral center for inflammatory myositis, a community rheumatology practice, or an acute care primary clinic, with each change in disease frequency, the usefulness of the test is determined by the PPV in that clinical setting or population.
Classification criteria
Classification criteria are defined as a set of disease characteristics used to group individuals into a well-defined homogenous population with similar clinical disease features . Classification criteria are essential for understanding disease pathogenesis and assessing treatment response. Classification criteria increase the specificity for underlying disease by creating a homogenous population, while at times losing sensitivity on the ROC continuum.
Classification criteria are not designed to be used for clinical diagnosis or applied to individual patients but instead used to further research of the population. For instance, in 1990, the ACR produced many disease specific classification criteria aimed at furthering clinical research in disease specific states. The 1990 ACR vasculitis classification criteria were shown by Rao et al. to have particularly low PPV for a specific vasculitis diagnosis, <30%, with only 38/51(75%) of patients with vasculitis fulfilling the 1990 classification criteria for any type of vasculitis, low sensitivity. Furthermore, the criteria also showed a low specificity with 31/147 (21%) of control patients without vasculitis actually fulfilling these vasculitis classification criteria . Further study of the subsequent Chapel Hill Consensus Vasculitis criteria showed similar low sensitivity and specificity of classification criteria being applied as diagnostic criteria to individual patients in which the classification criteria were not designed to assess . Another study showed that 65.8% of patients with histopathologically proven vasculitis from a single center were classified according to the Chapel Hill Consensus Classification criteria , further showing the lack of clinical utility for the vasculitis classification criteria outside of clinical research studies. Similar to vasculitis, in knee osteoarthritis, Peat et al. examined the relationships between patient diagnosis, clinician diagnosis, and classification of knee osteoarthritis by the ACR criteria, and they found poor levels of agreement between patient or physician diagnosis with clinical classification of knee osteoarthritis . Classification criteria work best in the study of groups rather than the study, or care, of the individual patient .
In 2007, Johnson et al. reviewed the methodological properties of various classification criteria for the rheumatic diseases , and they emphasized that there are marked variation and significant deficiencies in methods used for criteria development, which affects the face validity and reliability of classification criteria. They noted that >50% of the classification criteria used in rheumatic disease have not even been based off of patient data sets, but instead on expert opinion alone . There were several other shortcomings noted in previous classification criteria including the lack of adequate control with only four criteria using controls with non-rheumatic disease, small numbers of patients, poor description of content validity and description of construct of disease, inclusions of limited patient populations, including community rheumatology practices rather than only referral centers, and lack of independent validation . Prospective longitudinal cohorts are the optimal cohort design for criteria development, including adequate control groups, with each criterion in the classification criteria investigated for psychometric properties . The population in which classification criteria are developed needs to be considered as, often, classification criteria are specific to the patient population being studied . Oftentimes, classification criteria are developed on populations from academic medical centers without including community practices, which might limit their use in community practices. Moreover, following the development of classification criteria, their use is not confirmed until validated in independent cohorts. Over time, with advancement in technology, methodological rigor, and better understanding of the disease, older classification criteria need to be updated. The subcommittee of quality of care – the classification and response criteria committee of the ACR and the EULAR, – has been active in classification criteria development and further validation of use in clinical trials .
With the efforts and support of ACR and EULAR in the last 5 years, newer classification criteria are being developed, which may perform better than some older versions in terms of sensitivity and/or specificity. Furthermore, with advancement in therapeutics (better risk–benefit profile of drugs) and the need for recognizing and treating early disease in order to change the natural history of the disease, there is a current trend in newer classification criteria development, particularly for both systemic lupus and RA, of having increased sensitivity. For instance, the 1987 ACR RA criteria had low sensitivity for definitive RA diagnosis in early disease, and subsequently the criteria were updated in 2010 with improved sensitivity in order to include patients with early-onset RA in clinical trials . However, with this increased sensitivity in lupus and RA classification criteria, there is a loss of specificity , leading to further limitation of their use for clinical diagnosis. Ideal diagnostic criteria need to have very high (almost perfect, 100%) sensitivity and specificity, ensuring the clinician confidence in the diagnosis. By increasing sensitivity, early disease is included in the population for the classification criteria. However, this increased sensitivity risks including both undifferentiated arthritis that does not progress to RA and subjects with incomplete lupus who do not develop systemic lupus. On the other hand, the inclusion of early disease gives the opportunity to develop newer therapeutics with improved outcomes and chance of cure. Moreover, the risk–benefit ratio of therapeutics needs to be considered when determining the sensitivity and specificity of criteria. For instance, in gout, the existing classification criteria have low specificity for early disease, and they should be used with caution in early disease when investigating agents with unclear safety profiles .
Furthermore, classification criteria change over time with development of new technology and longitudinal evaluation of disease. This process is most evident in the evolution of the classification criteria for systemic sclerosis (SSc). Originally, the 1980 ACR classification for SSc was shown to have a low sensitivity with only 34 out of 54 patients with a clinical diagnosis of SSc fulfilling the classification criteria; these criteria were designed for research studies, thus having high specificity but low sensitivity . A high specificity of classification criteria is essential for clinical research of disease to create homogeneity among the subjects, but the decreased sensitivity leaves the criteria without utility in patient care. Furthermore, stringent criteria are required, especially when cytotoxic treatment is necessary for severe disease, to ensure only uniform patient populations with disease are enrolled in clinical trials for therapeutic investigation. Over time and with increased understanding of disease, the SSc classification criteria have been updated to include extra-cutaneous manifestation of disease such as interstitial lung disease and pulmonary hypertension, they have also included advanced laboratory evidence of disease with SSc-related antibodies . With this improvement of understanding of disease and updated criteria, the criteria now have much improved sensitivity and specificity (up to 96% sensitive and 90% specific) . Given the advances in understanding the pathogenesis of disease, multiple older classification criteria, including vasculitis and myositis, are in the process of being updated along the same lines as the SSc criteria . These updated criteria are likely to have better sensitivity as well as specificity due to improved knowledge as compared to few decades ago.
Although traditionally classification criteria have high specificity, the 1975 Bohan and Peter classification criteria for polymyositis (PM) are an example where the classification criteria were too nonspecific, leading to the wrong diagnosis of PM in patients with metabolic myopathy, muscle dystrophies, and inclusion body myositis . These criteria only require a minimum of symmetrical proximal muscle weakness and abnormal serum skeletal muscle enzymes, myopathic electromyography (EMG), or biopsy for probable classification of PM. Given that PM often requires intensive and potentially toxic treatment with long courses of corticosteroids and additional immunosuppressive medications, clinicians should do due diligence of ruling out PM mimics before making the clinical diagnosis, rather than blindly applying the classification criteria for diagnosis .
Diagnostic criteria
Diagnostic criteria are a conglomeration of signs, symptoms, or supportive tests used in routine clinical care to aid in a clinical diagnosis in an individual patient. Clinical diagnoses are used for two major purposes during the care of the individual patient: first, to guide medical care and, second, to help the patient gain an understanding of the disease process with prognosis. The most well-known diagnostic criteria in medicine are in psychiatry for the Diagnostic and Statistical Manual of Mental Disorders . As there was poor agreement among providers regarding patient’s psychiatric diagnosis, specific diagnostic criteria were developed to assist in patient care and diagnosis. The goal of diagnostic criteria is to have a high PPV of the diagnosis and high likelihood ratio, whereas the goal of classification criteria is to create a well-defined group of patients with the same underlying diagnosis.
Regarding the evolution of diagnostic criteria development, diagnostic criteria were initially developed to purely help guide the care of patients, but later they were translated for use in clinical research. Following the initial Jones diagnostic criteria development for rheumatic fever, clinical research realized that more specific criteria were needed to prevent misclassification of non-disease patients and exposing them to therapeutic agents with unclear evidence in clinical trials . As formal clinical research studies were developed, classification criteria soon followed to increase specificity for disease and minimize variation across study populations. Professional societies have focused on classification criteria development, whereas diagnostic criteria have not been updated.
Given the lack of optimal diagnostic criteria, the majority of rheumatic disease diagnoses are now made based on a complex decision making process by physicians – using a combination of symptoms, signs, and available diagnostic tests, and ruling out other competing diagnosis while considering knowledge about geographic disease prevalence affecting pretest probability to make a diagnosis. One particular difficulty in the development of diagnostic criteria is that the disease prevalence in the population (different geographical areas or clinic settings) significantly affects the specificity and PPV of any criteria. For instance, in a morning report at an academic medical center, a patient presenting with significant nausea, hepatomegaly, and transaminitis has a broad differential diagnosis requiring multiple diagnostic tests to evaluate the differential diagnosis (imaging, recurrent laboratory work, and liver biopsy). However, the same patient presenting in an endemic area for hepatitis A will be diagnosed and treated as such given the high prevalence of the disease in that area. These variations in disease frequency by geographic areas are especially true for some rheumatic diseases. For example, the prevalence of Takayasu arteritis in Asian countries is very different from that in USA, leading to a vast difference in performance characteristics especially the PPV of any criteria for diagnosis. There are also wide variations in rheumatic disease frequency in different clinical settings within the same geographic areas such as academic referral center as compared to community rheumatology practice or primary care physician. The same concept applies to differences in disease prevalence in individual races or ethnicities within a geographic area.
Moreover, the rarity and heterogeneity of many rheumatic diseases, given complex and variable clinic presentations, lack of gold-standard tests, and reliance of multiple clinical factors rather than few makes the development of diagnostic criteria extremely difficult in rheumatology. Due to these limitations, only few diagnostic criteria have been developed in rheumatology with the most recent diagnostic criteria being the recently validated 2010 ACR preliminary fibromyalgia syndrome (FMS) diagnostic criteria . However, these criteria have been criticized secondary to their lack of specificity . For instance, when the modified 2010 FMS criteria were applied to patients with cirrhosis, 53 of 193 patients (27%) met the diagnostic criteria for FMS syndrome . In addition, when the 2010 FMS diagnostic criteria were applied to 100 pregnant patients, 27 patients (27%) fulfilled the modified 2010 FMS criteria, whereas only 1 of 100 patients fulfilled the previous 1990 classification criteria, which were more specific . This example illustrates the difficulty of placing a complex decision made by a trained rheumatologist, who considers several factors simultaneously to make a clinical diagnosis, into a simple algorithm of diagnostic criteria.
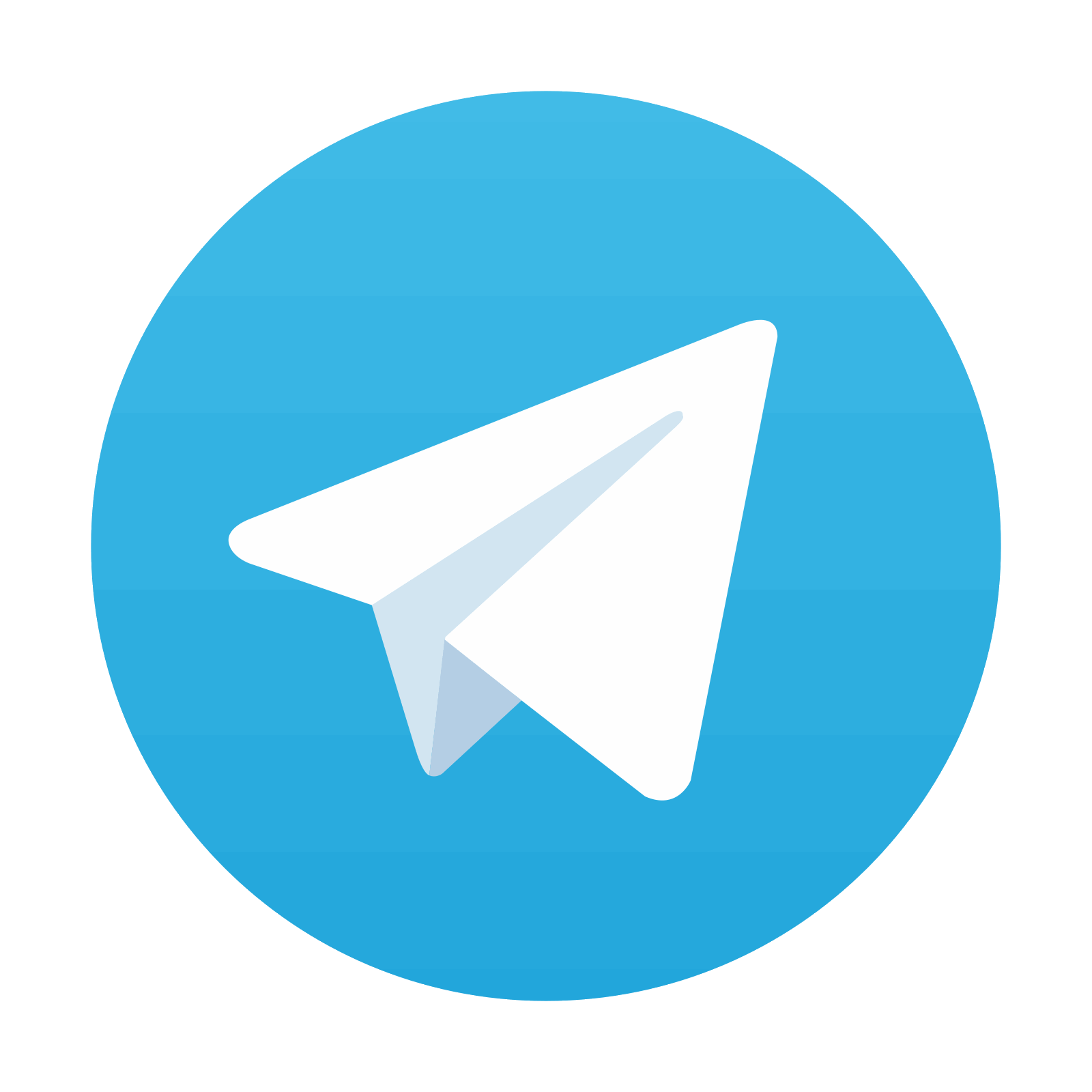
Stay updated, free articles. Join our Telegram channel

Full access? Get Clinical Tree
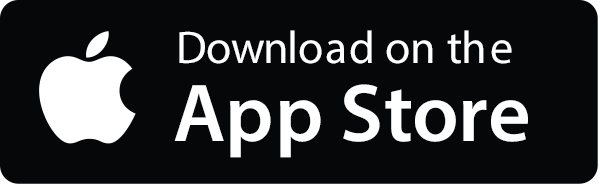
