PROMIS, the Patient-Reported Outcomes Measurement Information System, is opening new possibilities to explore and learn how patient (or proxy) self-report of core symptoms and health-related quality of life can meaningfully advance clinical research and patient care. PROMIS leverages Item Response Theory to agnostically assess, across diseases and conditions or clinical settings, numerous universally applicable core “domains” of health (symptoms and functioning) from the patient perspective. Importantly, PROMIS is enabling the testing and adoption of computerized adaptive testing, which holds great potential to minimize patient burden while maximizing accuracy.
Key points
- •
The Patient-Reported Outcomes Measurement Information System (PROMIS) implements modern measurement theory and techniques to advance self- and proxy assessment of symptoms and health-related quality-of-life concepts.
- •
PROMIS enables improved measurement precision with less respondent burden by embracing item-response theory and computer-adaptive testing.
- •
PROMIS focuses on measuring universally relevant domains of health and disease to allow agnostic assessments across diseases and clinical settings facilitating meaningful cross-disease comparisons.
- •
By standardizing patient-reported outcome assessments, PROMIS supports the accumulation of data across settings, which enables meta-analysis and increases the amount of information that can be brought to the interpretation of the scores.
PROMIS ® (a registered trademark of the US Department of Health and Human Services)—the Patient-Reported Outcomes Measurement Information System—is helping to facilitate an evolution in the science of patient/person self-assessment of experiences during health or disease. PROMIS represents a cooperative research program involving multiple academic medical centers, private research organizations, and numerous Institutes across the National Institutes of Health (NIH). It was designed to bring the most advanced measurement science to the development, evaluation, and standardization of item banks to measure patient-reported outcomes (PROs) of health-related quality of life (HRQL) across medical conditions. This system is the result of adopting and implementing concepts like item response theory (IRT) and standard setting (bookmarking), commonly used in educational testing, to measure and interpret health-related outcomes. PROMIS measures are applicable to be used as primary, secondary, or exploratory outcome measures in both adult and pediatric clinical research as well as to provide assessments of HRQL in patient care settings. This evolution in the assessment of PROs is occurring in many fields of medicine, not the least of which is rheumatology.
The PROMIS initiative was one of the efforts of the NIH Roadmap (later Common Fund) initiative in 2004 designed to re-engineer the clinical research enterprise. The funding announcement laid out a new vision by noting that, “ The clinical outcomes research enterprise would be enhanced greatly by the availability of a psychometrically validated, dynamic system to measure PROs efficiently in study participants with a wide range of chronic diseases and demographic characteristics .” As noted, it established a collaborative working group between NIH and individual research teams throughout the United States to develop a measurement system and take it through various stages of growth and maturation (see later discussion, under New Science of Patient-Reported Outcomes).
Funding for this greater than 10-year initiative resulted from the recognition at the time that there was no common PRO language and no national standardized set of PRO instruments. Rather, what existed was a “Tower of Babel” approach for assessing PROs across diseases such as rheumatoid arthritis (RA), psoriatic arthritis, or systemic lupus erythematosus (SLE). Certainly, disease-specific outcome measures, especially those that include patient self-assessments, have been demonstrated to be useful for studies within the population in whom they were developed. However, such specificity has hampered the ability to easily and meaningfully compare the level of symptoms and other burdensome aspects of compromised health that make up “health-related quality of life” from one disease to another. Arguably, this lack of a common PRO language also hinders the ability to integrate and synthesize valuable PRO data into a better understanding of common pathogenic mechanisms that drive disease and adversely impact health. Moreover, a common language is required to assess PROs for patients with multiple chronic conditions.
New science of patient-reported outcomes
Robust qualitative and quantitative studies, using a “mixed methods approach,” are essential components for PRO development and validation according to modern measurement principles and current standards. Despite this, few of the legacy or traditional PROs currently used in clinical medicine, including rheumatology, have been developed with this degree of rigor and attention, especially the inclusion of input from those living with and impacted by the conditions under study during the instruments’ development. Many of these fundamental principles, especially those that relate to establishing the content validity of a developing PRO instrument, have been delineated in the US Food and Drug Administration’s (FDA) PRO Guidance Document. Those principles, illustrated in Fig. 1 , require a series of iterative steps to ensure thorough psychometric and clinically focused validation.

Even though PROMIS began years before the release of this PRO guidance, these fundamental principles for the development and validation of PROs shepherded the maturation of PROMIS from its beginning into what it is today. In fact, PROMIS has established a maturity model to standardize this process for extant, and future, PROMIS measures ( http://www.nihpromis.org/Documents/PROMISStandards_Vers%202.0_MaturityModelOnly_508.pdf ).
One truly unique feature of PROMIS is that it is based in IRT. IRT was developed in the educational testing fields to measure academic content in a standardized manner that would enable the enhancement of measurement precision at all levels of ability. Although grounded in sophisticated mathematical models and principles, simply stated, IRT allows patients and items (ie, questions) to be placed on the same metric. As seen in Fig. 2 , based in this case on participants’ self-reports of their physical functioning (PF), the items and the participants likely to respond to the items throughout the scale can be ordered along a continuum by their levels of difficulty from low to high functioning. In educational testing whereby items have a “correct” answer, the difficulty of items refers to how challenging the content is. In the health care setting, item difficulty may refer to how difficult a task is (eg, in the case of PF) or how “intense” or “hard to endorse” a symptom is. For example, respondents with high levels of depressive symptoms would be likely to respond “always” to the item, “I felt hopeless.”

IRT also enables computer-adaptive testing (CAT), which is a distinguishing feature between a measure developed with classical test theory and IRT. As an example, the PROMIS adult PF bank currently includes 124 items. To obtain a score with such a questionnaire in a classic testing setting, patients/participants would be required to answer all 124 questions. However, with IRT and CAT, an accurate estimate of one’s score on the PROMIS PF scale can be obtained by answering only a fraction of questions. CAT algorithms take advantage of this feature by administering items that are targeted at a respondent’s individual level of the trait being measured. This adaptive process is depicted in Fig. 3 . Based on how a participant answers a question, a computer-driven, mathematical algorithm selects the next best question. Using the example in Fig. 2 of the PROMIS PF bank, it would make no sense to ask a person who reports being able to run 2 miles without difficulty whether he or she is able to get in and out of bed. Therefore, the respondent is not asked questions for which the answer is already likely known.

When a predetermined stopping criterion is met (eg, a certain level of precision around the score is obtained), the CAT stops and calculates a score ( Fig. 4 ) with an explanation of what the score means. How this information is displayed to patients, researchers, and health care providers is an area of active research. Nonetheless, a real-time assessment that can be easily accomplished and incorporated into electronic medical records records is an attractive option. CAT can result in a substantial reduction in the time and burden of capturing patient-important information. In addition to CAT versions, all PROMIS measures are available in more traditional “static” short forms. Because of the development of the short forms through IRT, scores are obtained on short forms on the same mathematical metric as those obtained through CAT. Fig. 4 can also be used to display results from PROMIS short forms.

The metric used in PROMIS is the T score. Fig. 5 is an idealized example of a normal (ie, Gaussian) distribution of this score with a general population mean of 50 and standard deviation of 10 points. It is idealized because PROMIS measures do not necessarily exactly follow this distribution along a domain, but neither do other PRO instruments. In terms of scores, this means that values from 40 to 60 would cover 68% of the population, while scores from 20 to 80 capture 99.7%. With the explosion of “OMICs” in all facets of scientific research, PROMIS represents the contribution that “PROomics” into the OMIC family could add to the future of personalized, person-centric medicine. Integration of PROs with traditional (eg, genomics, epigenomics, metabolomics, microbiome) biomarkers of disease can lead to a better understanding of how disease mechanisms impact the domains of health, such as pain, PF, fatigue, or social interaction, and will ultimately improve the quality and generalizability of clinical research and patient care.
New science of patient-reported outcomes
Robust qualitative and quantitative studies, using a “mixed methods approach,” are essential components for PRO development and validation according to modern measurement principles and current standards. Despite this, few of the legacy or traditional PROs currently used in clinical medicine, including rheumatology, have been developed with this degree of rigor and attention, especially the inclusion of input from those living with and impacted by the conditions under study during the instruments’ development. Many of these fundamental principles, especially those that relate to establishing the content validity of a developing PRO instrument, have been delineated in the US Food and Drug Administration’s (FDA) PRO Guidance Document. Those principles, illustrated in Fig. 1 , require a series of iterative steps to ensure thorough psychometric and clinically focused validation.
Even though PROMIS began years before the release of this PRO guidance, these fundamental principles for the development and validation of PROs shepherded the maturation of PROMIS from its beginning into what it is today. In fact, PROMIS has established a maturity model to standardize this process for extant, and future, PROMIS measures ( http://www.nihpromis.org/Documents/PROMISStandards_Vers%202.0_MaturityModelOnly_508.pdf ).
One truly unique feature of PROMIS is that it is based in IRT. IRT was developed in the educational testing fields to measure academic content in a standardized manner that would enable the enhancement of measurement precision at all levels of ability. Although grounded in sophisticated mathematical models and principles, simply stated, IRT allows patients and items (ie, questions) to be placed on the same metric. As seen in Fig. 2 , based in this case on participants’ self-reports of their physical functioning (PF), the items and the participants likely to respond to the items throughout the scale can be ordered along a continuum by their levels of difficulty from low to high functioning. In educational testing whereby items have a “correct” answer, the difficulty of items refers to how challenging the content is. In the health care setting, item difficulty may refer to how difficult a task is (eg, in the case of PF) or how “intense” or “hard to endorse” a symptom is. For example, respondents with high levels of depressive symptoms would be likely to respond “always” to the item, “I felt hopeless.”
IRT also enables computer-adaptive testing (CAT), which is a distinguishing feature between a measure developed with classical test theory and IRT. As an example, the PROMIS adult PF bank currently includes 124 items. To obtain a score with such a questionnaire in a classic testing setting, patients/participants would be required to answer all 124 questions. However, with IRT and CAT, an accurate estimate of one’s score on the PROMIS PF scale can be obtained by answering only a fraction of questions. CAT algorithms take advantage of this feature by administering items that are targeted at a respondent’s individual level of the trait being measured. This adaptive process is depicted in Fig. 3 . Based on how a participant answers a question, a computer-driven, mathematical algorithm selects the next best question. Using the example in Fig. 2 of the PROMIS PF bank, it would make no sense to ask a person who reports being able to run 2 miles without difficulty whether he or she is able to get in and out of bed. Therefore, the respondent is not asked questions for which the answer is already likely known.
When a predetermined stopping criterion is met (eg, a certain level of precision around the score is obtained), the CAT stops and calculates a score ( Fig. 4 ) with an explanation of what the score means. How this information is displayed to patients, researchers, and health care providers is an area of active research. Nonetheless, a real-time assessment that can be easily accomplished and incorporated into electronic medical records records is an attractive option. CAT can result in a substantial reduction in the time and burden of capturing patient-important information. In addition to CAT versions, all PROMIS measures are available in more traditional “static” short forms. Because of the development of the short forms through IRT, scores are obtained on short forms on the same mathematical metric as those obtained through CAT. Fig. 4 can also be used to display results from PROMIS short forms.
The metric used in PROMIS is the T score. Fig. 5 is an idealized example of a normal (ie, Gaussian) distribution of this score with a general population mean of 50 and standard deviation of 10 points. It is idealized because PROMIS measures do not necessarily exactly follow this distribution along a domain, but neither do other PRO instruments. In terms of scores, this means that values from 40 to 60 would cover 68% of the population, while scores from 20 to 80 capture 99.7%. With the explosion of “OMICs” in all facets of scientific research, PROMIS represents the contribution that “PROomics” into the OMIC family could add to the future of personalized, person-centric medicine. Integration of PROs with traditional (eg, genomics, epigenomics, metabolomics, microbiome) biomarkers of disease can lead to a better understanding of how disease mechanisms impact the domains of health, such as pain, PF, fatigue, or social interaction, and will ultimately improve the quality and generalizability of clinical research and patient care.
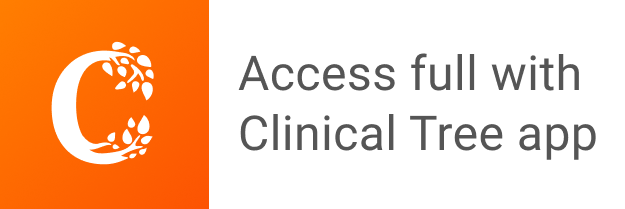