Randomized Controlled Trials
Heng An Lin, MBBS, MMEd(Ortho), FRCSEd
Patrick D.G. Henry, MD, FRCSC
David Wasserstein, MD, MSc, MPH, FRCSC
Dr. Henry or an immediate family member is a member of a speakers’ bureau or has made paid presentations on behalf of CONMED Linvatec. Neither of the following authors nor any immediate family member has received anything of value from or has stock or stock options held in a commercial company or institution related directly or indirectly to the subject of this chapter: Dr. Lin and Dr. Wasserstein.
Keywords: level of evidence; prospective; randomized controlled trials; study design
INTRODUCTION
The practice of orthopaedic surgery has evolved tremendously over the last two decades, with an emphasis on evidence-informed care. Critical appraisal of the literature is an inherent challenge in this environment, primarily due to the sheer volume and heterogeneity of published literature quality.1,2,3,4 This necessary skill requires appropriate interpretation of the data, which may not always be concordant with the authors’ conclusions. Readers must also recognize the benefits and limitations of each study, and this starts with an understanding of the study design. In the world of medical evidence, no type of study has achieved a greater reverence than a well-designed, well-executed, randomized controlled trial.
Randomized controlled trials (RCTs) are a form of prospective, analytical study that allows for the comparison of therapeutic options. When well designed and executed, the RCT minimizes bias in comparison to other study designs, and thus, the evidence it produces is considered “level 1,” based on the Centre for Evidence-Based Medicine Level of Evidence Classification.5 However, the proper design and execution of RCTs can be tedious, time consuming, and involve high cost. A study labeled as an “RCT” alone is not enough to ensure it has achieved this highest level of quality.
This chapter will outline the fundamental characteristics of the RCT, using landmark studies from the Orthopaedic literature as case examples.
UNDERSTANDING RANDOMIZED CONTROLLED TRIALS
An RCT is a prospective study design typically used to investigate the effectiveness of an intervention on a primary outcome of interest for a particular study population. For the trial, the study population is divided into at least two groups for comparison, each group receiving a different treatment. The process by which patients are distributed into the groups is called “randomization.” Randomization is the allocation of included patients into groups “at random,” rather than selecting patients for the groups by a particular feature or characteristic that they share. There are several different RCT designs including parallel, crossover, and factorial RCTs.
The most common type of RCT is the parallel design (Figure 1). In the parallel design, the study population is divided into groups (by the process of randomization), with each subject in each group exposed to only one intervention. Typically, one of these interventions is a new drug or treatment that is being tested, and the other group is considered the “control” group and is treated with either no treatment or another treatment that is considered the benchmark. The groups are then compared to determine whether one intervention is superior at achieving a particular outcome of interest. For example, Moseley JB et al (NEJM 2002)6 randomized 180 patients in a parallel design to arthroscopic débridement, arthroscopic lavage, or placebo surgery, in the management of
knee osteoarthritis. They found no difference in knee-specific pain scores or functional improvement in walking, bending, or climbing stairs.
knee osteoarthritis. They found no difference in knee-specific pain scores or functional improvement in walking, bending, or climbing stairs.
In a crossover design (Figures 2 and 3), two or more groups both receive the same intervention(s), but at different time periods. In this case, the subjects serve as their own controls. In the Denosumab Adherence Preference Satisfaction study (Freemantle et al, Osteoporosis Int 2012),7 250 postmenopausal women across 25 North American centers were given alendronate then denosumab, or denosumab then alendronate, over successive 12-month periods for each of the treatment. The similarities of the effectiveness of the drugs allowed the investigators to elucidate practical outcomes, like adherence, using the crossover design RCT.
Another major advantage of the crossover design is the avoidance of confounders (eg, age or sex) within the study and control groups, because the same people make up each group. This leads to statistical efficiency, meaning fewer patients (smaller sample size) are required. However, the disadvantages of crossover RCTs include a longer study duration, difficulty in managing dropout who had completed only a single evaluation phase, the possibility of unblinding when the participants noticed significant differences between the treatment phases, and the possibility of carryover effects between evaluation phases, especially if the “washout” phase is insufficient. Due to its nature, this design is almost impossible to carry out when testing surgical interventions and is therefore used more extensively in medical specialties.
“Crossover design” should not be confused with “treatment crossover,” which is the switching from one intervention arm to another and is common to all RCT designs. For example, in the Spine Patient Outcomes Research Trial (SPORT),8,9 245 patients were assigned to the standard open diskectomy group and 256 to the nonsurgical group. By the end of 1 year, 41% of those assigned to the nonsurgical arm had undergone surgery and 43% of patients assigned to surgery did not receive it. Only 59% of the patients assigned to the surgical arm had surgery performed on them by the end of 4 years.
In a factorial design RCT, it is possible to study several intervention arms within the study population at the same time.10 In a simple 2 × 2 factorial design RCT (Figure 4), there are two permutations to each of the two intervention arms (ie, yes/no). Hence, individual participants can be randomized to receive a single treatment, both treatments, or no treatment (control/placebo). The effect of each intervention can be compared with one another, with the control/placebo, as well as with a combination of treatments. This is the only design that allows the testing for interactions between different treatments.11 In Fluid Lavage of Open Wounds (FLOW) study (FLOW group, NEJM, 2015),12 2,551 patients with open fractures from across 41 centers in North America, Norway, Australia, and India were centrally randomized into one of the six treatment arms, in permutations of cleansing solution (either soap or normal saline) and pressure (high pressure, low pressure or gravity)—known as a 2 × 3 factorial RCT design. The rate of revision surgery was similar regardless of pressure, although the soap solution group had a higher revision surgery rate compared with that of normal saline.
RANDOMIZATION AND ALLOCATION
Randomization is the process of allocating subjects (patients) into groups within a study, and this process aims to reduce selection bias. Selection bias is the error introduced when the criteria used in selecting the subjects do not correctly
represent the target population of interest being evenly distributed in both (or all) groups.13 While it might seem more reliable to take a study population and go through each patient one by one and try to “match” each person up with someone with similar characteristics and place them in opposite groups, it is impossible to find every characteristic about each patient. Randomization increases the likelihood of a balanced profile of subjects within each of the groups, including both known and unknown demographic, confounding, or prognostic factors,14 such that the groups only differ in the interventions to be compared. The theoretical balancing of unknown confounding factors is a significant advantage of the RCT over matched, nonrandomized prospective studies where the likelihood of unknown confounders is higher—and this may bias the results.
represent the target population of interest being evenly distributed in both (or all) groups.13 While it might seem more reliable to take a study population and go through each patient one by one and try to “match” each person up with someone with similar characteristics and place them in opposite groups, it is impossible to find every characteristic about each patient. Randomization increases the likelihood of a balanced profile of subjects within each of the groups, including both known and unknown demographic, confounding, or prognostic factors,14 such that the groups only differ in the interventions to be compared. The theoretical balancing of unknown confounding factors is a significant advantage of the RCT over matched, nonrandomized prospective studies where the likelihood of unknown confounders is higher—and this may bias the results.
Randomization can be achieved in several ways, including simple randomization, block randomization, stratified randomization, and covariate adaptive randomization.14
In simple randomization, subjects are allocated based on a single algorithm of random assignment. The classic example is a flip of the coin. Modern techniques utilize a random computer-generated number, and allocation is based on an odd or even number. Although it is easy to implement, simple randomization does not control the number of patients allocated to each group and can lead to imbalance of the group sizes.
Block randomization (Figure 5) is designed to prevent this problem by randomizing patients in “blocks.” A block consists of a predetermined number of subjects. The number of subjects within the block is determined by the number of groups in the study. For a study with two groups, the number of subjects within the block should be in multiples of 2; multiples of 3 with 3 groups, etc. Within each block, subjects are randomized and allocated to intervention groups, which ensures balanced numbers. Block randomization is most useful when dealing with a small sized cohort (Figure 6).
![]() FIGURE 5 Diagram of block randomization. Each Block will consist of participants in multiples of number of groups. |
As stated above, randomization helps balance confounding factors, such as underlying medical problems for example. However, simple and block randomizations may still result in groups of patients with very different baseline characteristics (also called “covariates” when these characteristics are used in part of the statistical analysis). Thus, despite randomizing, an investigator may still end up with two differing groups of patients, one relatively healthy compared with the other, or older than the other, etc. The risk of this occurring is greater with smaller samples.
Stratified randomization is a technique that can be employed to further balance covariates among groups. Blocks are created based on important covariates, such as sex, or the presence of a comorbidity of interest. Randomization and allocation then occur within the block, ensuring balance of randomization based on the covariate(s) of interest. However, there are disadvantages to this technique. First, implementation of this process can be tedious and difficult, as once the number of covariates increases, there will be many separate blocks. Second, the covariates of interest need to be identified prior to randomization, which may not always be apparent right from the start of a prospective trial. Third, it still does not account for unknown covariates.
The Moseley et al (NEJM 2002) trial6 utilized a block randomization with stratification RCT design. In the randomization process of the trial, the participants were stratified according to the severity of osteoarthritis (ie, the main covariate in the study). This was followed by a stratified allocation with fixed blocks of six, wherein patients were randomized to one of three treatment arms. The goal of stratification was to allow an even distribution of mild to severe knee osteoarthritis across the various treatment arms. This was an effective design for at the time what was a landmark trial. In retrospect, separating the groups entirely based on severity of arthritis may have provided more pertinent data, as it is only the group of patients with no or very mild osteoarthritis (OA) where contention still exists as to the efficacy of this particular intervention!15
Adaptive randomization also allows for consideration of covariates. In this method of randomization, each new subject is assigned sequentially to a group based on the specific covariates that they have, taking into consideration previous subject allocations.16
CONCEALMENT AND BLINDING
In addition to being “random,” group allocation should also be “concealed.” Allocation concealment refers to the technique where the next treatment allocation is concealed from the researcher randomizing and assigning the patient, until the moment of assignment. (Note that this is different than “blinding,” which has to do with either patient or researcher being kept unaware of the treatment group even after assignment.) Concealment reduces selection bias that might otherwise be caused by the investigators, as they might influence the patient treatment assignment. For example, in a trial comparing different grafts in anterior cruciate ligament reconstruction, a surgeon who favors bone-patella tendon-bone autograft may be influenced to assign his patients into this treatment group, as opposed to other graft options. Concealment prevents this surgeon from doing this.
Ideally, participants in an RCT should also be blinded. A “blinded trial” refers to a study where treatment allocation is kept secret for the entire duration of the trial. A “blinded” person does not know what treatment was received by each patient. Patients, healthcare providers, researchers, and outcome analyzers may all potentially be blinded depending on the intervention studied. A single-blinded trial conceals the allocation from the subjects; a double-blinded trial conceals allocation from the subjects and investigators; a triple-blinded trial conceals allocation from the investigators, subjects, and outcome assessors/analysts. Blinding reduces observer bias from the investigators, placebo and nocebo effect bias from the subjects, and bias of data analysis and interpretation by eliminating the possibility of that person (patient, provider, or outcome assessor) injecting their own preconceived notions into their role in the study.
The process of blinding is not easy and, in some cases, is not possible, particularly in surgery. In a review of RCTs published in the Journal of Bone and Joint Surgery (American) from 1988 till 2000,17,18 it was noted that there were more higher quality double-blinded trials on drugs as compared with surgical intervention. Though it is possible to blind the assessors and sometimes the patients in a surgical trial, surgeons cannot be blinded.
INCLUSION AND EXCLUSION CRITERIA
Ideal RCT cohort selection consists of participants from a relatively homogenous population—this will minimize confounders (ie, differences between the baseline characteristics in participants in each group that might affect the outcome). For example, the age of the target population is of importance as the physiology in a child differs greatly from that of an adult; therefore, narrowing the age criteria of a study to patients between the ages of 18 and 60 years would eliminate the chance that there would be more children in one group compared with the other. “Inclusion and exclusion criteria” help refine the target population. By adhering to strict criteria, it is possible to make the study population very homogenous, which will minimize bias and thus increase the “internal validity” of the study (ie, it increases the likelihood that the conclusions you make in your study are valid for that specified patient population).
However, if a population selected for inclusion is too narrow, then the study will lack external validity; meaning the results may not be applicable to many patients in real-life practice (as the typical clinical practice usually involves patients of various ages and medical profiles). Defining a narrow population can also lead to difficulty in patient recruitment and, subsequently, failure to complete a study or recruit enough patients to power the analysis. Finding the right balance in the selection criteria is challenging and depends on many factors, and this usually involves a good literature review and a seasoned team of investigators.
SETTING PRIMARY AND SECONDARY OUTCOME MEASURES
The primary outcome measure is the most important dependent variable which the study aims to evaluate. This must be identified prior to the start of the study to set a clear goal around which the study design can be developed. The remaining dependent variables that the authors are interested to look at, but are not part of the main hypothesis, are known as secondary outcome measures.
The primary outcome measure is typically used to calculate the sample size of the study. It must be set before the study which avoids a post hoc (ie, after study completion) selection of statistically significant secondary outcome. This is just one of the reasons authors are encouraged to register RCTs publicly before recruitment (eg, registration can be done on the website www.clinicaltrials.gov)—an almost mandatory step to facilitate eventual peer-review publication.
Secondary outcome measures should also be judiciously selected to minimize the probability of reporting falsely significant results. In any RCT, there are many data that can be potentially used as outcomes. However, when multiple variables are examined, the risk of false-positive findings is increased. Thus, the primary outcome is the most important, and secondary outcome findings should be interpreted with caution, especially when there are numerous secondary outcome measures.19
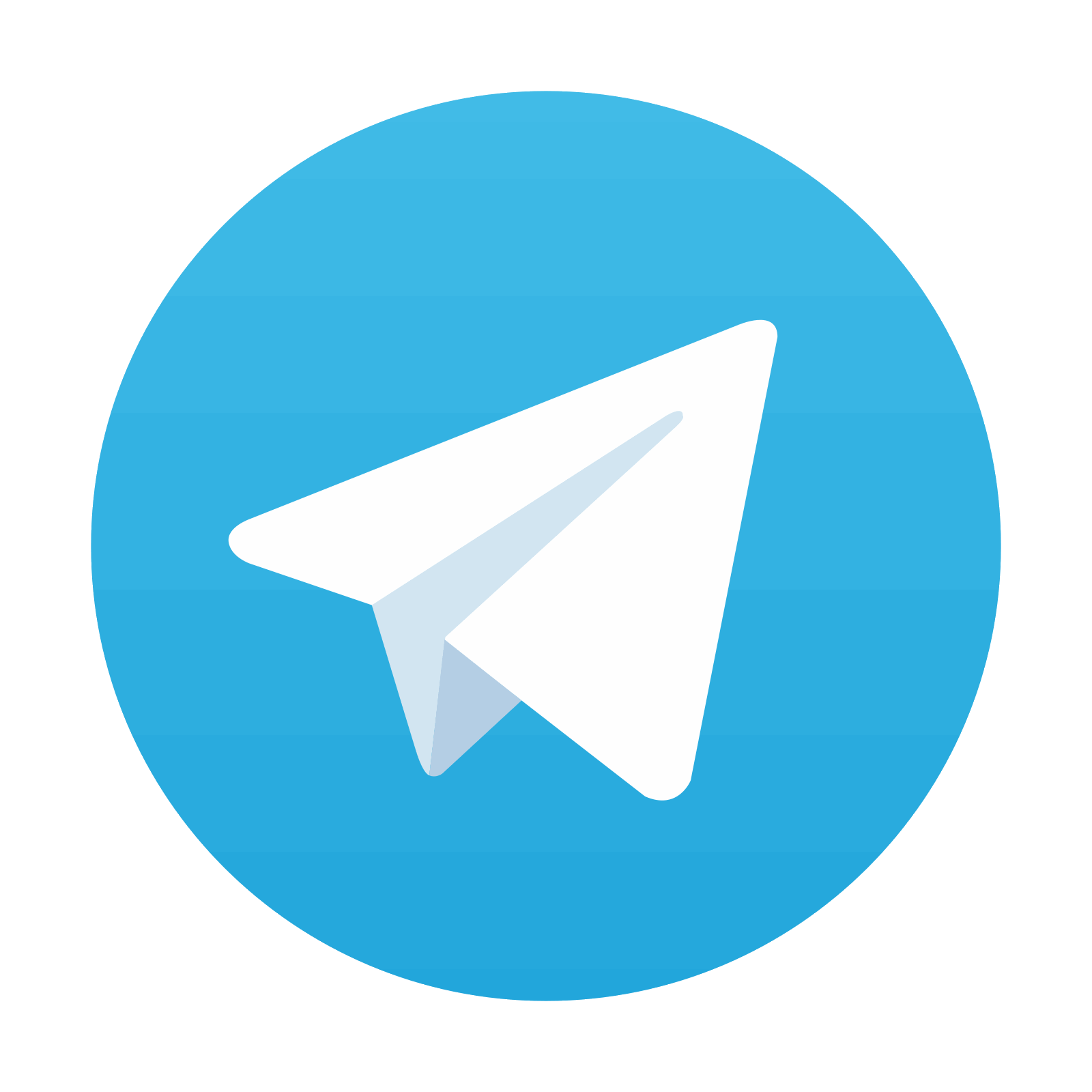
Stay updated, free articles. Join our Telegram channel

Full access? Get Clinical Tree
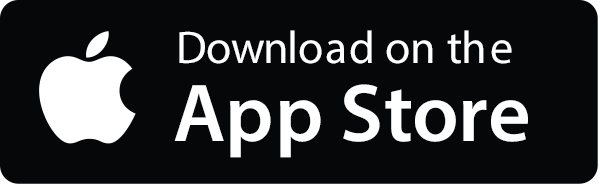
