The purpose of this article is to place these genetic discoveries in the context of current and future therapeutic strategies for patients with RA. More specifically, this article focuses on (1) a brief overview of genetic studies, (2) human genetics as an approach to identify the Achilles heel of disease pathways, (3) humans as the model organism for functional studies of human mutations, (4) pharmacogenetic studies to gain insight into the mechanism of action of drugs, and (5) next-generation patient registries to enable large-scale genotype-phenotype studies.
Despite decades of research, the biologic pathways that initiate rheumatoid arthritis (RA) are unknown. Without knowing the specific pathways that lead to RA, it is very difficult to develop novel therapies. Because genetic mutations are inherited before disease onset, human genetics provides prima facie evidence that a pathway is important in pathogenesis. Moreover, because human genetic strategies can be applied genome wide, they offer an unbiased search of the human genome for an insight into RA pathogenesis.
Since the 1970s, more than 20 RA risk loci have been identified ( Table 1 ). The first locus associated with RA risk was the major histocompatibility (MHC) locus, identified by mixed lymphocyte cultures between patients and controls with RA. Subsequently, Gregersen and colleagues advanced the hypothesis that the multiple RA risk alleles within the HLA-DRB1 gene share a conserved amino acid sequence. This is now widely known as the “shared epitope” hypothesis, and the risk alleles are known as shared epitope alleles. With the sequence of the human genome and improved understanding of human genetic diversity came many additional genetic discoveries. Between 2003 and 2005, common alleles within the PADI4 , PTPN22 , and CTLA4 genes were found to be reproducibly associated with risk of RA. Genome-wide association studies (GWASs), which in the contemporary form test hundreds of thousands of single nucleotide polymorphisms (SNPs) across the genome, have been systematically performed in large case-control collections. These GWASs have identified more than 20 common alleles (where an allele is 1 of the 2 base pairs of an SNP) that confer a 10% to 20% increase in disease risk per copy of the risk allele. Collectively, these risk alleles explain approximately 15% to 20% of the overall disease burden.
SNP | Locus | Candidate Gene | OR | Allele Frequency | References |
---|---|---|---|---|---|
rs3890745 | 1p36.2 | TNFSF14 | 0.920 | 0.320 | Raychaudhuri et al, 2008 |
rs2240340 | 1p36.13 | PADI4 | 1.40 | 0.373 | Suzuki et al, 2003 |
rs2476601 | 1p13.2 | PTPN22 | 1.750 | 0.100 | Begovich et al, 2004 |
rs11586238 | 1p13.1 | CD2 , IGSF2 , CD58 | 1.120 | 0.227 | Raychaudhuri et al, 2009 |
rs7528684 | 1q23.1 | FCLR3 | 1.2 | 0.35 | Kochi et al, 2005 |
rs12746613 | 1q23.2 | FCGR2A | 1.100 | 0.124 | Raychaudhuri et al, 2009 |
rs3766379 | 1q23.3 | CD244 | 1.31 | 0.53 | Suzuki et al, 2008 |
rs10919563 | 1q31.3 | PTPRC | 0.900 | 0.132 | Raychaudhuri et al, 2009 |
rs13031237 | 2p16.1 | REL | 1.207 | 0.340 | Gregersen et al, 2009 |
rs934734 | 2p14 | SPRED2 | 1.13 | 0.51 | Stahl et al, 2010 |
rs10865035 | 2q11.2 | AFF3 | 1.140 | 0.460 | Barton et al, 2009 |
rs7574865 | 2q32.3 | STAT4 | 1.320 | 0.180 | Remmers et al, 2007 |
rs1980422 | 2q33.2 | CD28 | 1.100 | 0.238 | Raychaudhuri et al, 2009 |
rs3087243 | 2q33.2 | CTLA4 | 1.136 | 0.560 | Plenge et al, 2005 |
rs13315591 | 3p14 | PXK | 1.13 | 0.08 | Stahl et al, 2010 |
rs874040 | 4p15 | RBPJ | 1.18 | 0.30 | Stahl et al, 2010 |
rs6822844 | 4q27 | IL2/IL21 | 1.389 | 0.710 | Zhernakova et al, 2007 |
rs6859219 | 5q11 | ANKRD55 | 0.85 | 0.22 | Stahl et al, 2010 |
rs26232 | 5q21 | C5orf13 | 0.93 | 0.32 | Stahl et al, 2010 |
rs2395175 (and others) | 6p21.32 | MHC | 1.000 | 0.021 | Gregersen et al, 1987 |
rs548234 | 6q21 | PRDM1 | 1.100 | 0.322 | Raychaudhuri et al, 2009 |
rs10499194 | 6q23.3 | TNFAIP3 | 1.220 | 0.220 | Plenge et al, 2007 |
rs6920220 | 6q23.3 | TNFAIP3 | 1.333 | 0.610 | Thomson et al, 2007 |
rs5029937 | 6q23.3 | TNFAIP3 | 1.34 | 0.04 | Orozco et al, 2009 |
rs394581 | 6q25.3 | TAGAP | 0.930 | 0.286 | Raychaudhuri et al, 2009 |
rs3093023 | 6q27 | CCR6 | 1.11 | 0.43 | Stahl et al, 2010 |
rs10488631 | 7q32 | IRF5 | 1.25 | 0.10 | Stahl et al, 2010 |
rs2736340 | 8p23.1 | BLK | 1.122 | 0.243 | Gregersen et al, 2009 |
rs2812378 | 9p13.3 | CCL21 | 1.100 | 0.355 | Raychaudhuri et al, 2008 |
rs951005 | 9p13.3 | CCL21 | 0.87 | 0.15 | Stahl et al, 2010 |
rs3761847 | 9q33.1 | TRAF1 | 1.100 | 0.440 | Plenge et al, 2007; Kurreeman et al, 2007 |
rs2104286 | 10p15.1 | IL2RA | 0.92 | 0.28 | Thomson et al, 2007; Kurreeman et al, 2009 |
rs706778 | 10p15.1 | IL2RA | 1.11 | 0.40 | Stahl et al, 2010 |
rs4750316 | 10p15.1 | PRKCQ | 0.910 | 0.183 | Raychaudhuri et al, 2008; Barton et al, 2008 |
rs540386 | 11p12 | RAG1 , TRAF6 | 0.920 | 0.144 | Raychaudhuri et al, 2009 |
rs1678542 | 12q13.3 | KIF5A | 0.890 | 0.351 | Barton et al, 2008; Raychaudhuri et al, 2008 |
rs4810485 | 20q13.12 | CD40 | 0.910 | 0.231 | Raychaudhuri et al, 2008 |
rs3218253 | 22q12.3 | IL2RB | 1.110 | 0.730 | Barton et al, 2008 |
The purpose of this article is to place these genetic discoveries in the context of current and future therapeutic strategies for patients with RA. More specifically, this article focuses on (1) a brief overview of genetic studies, (2) human genetics as an approach to identify the Achilles heel of disease pathways, (3) humans as the model organism for functional studies of human mutations, (4) pharmacogenetic studies to gain insight into the mechanism of action of drugs, and (5) next-generation patient registries to enable large-scale genotype-phenotype studies.
Brief overview of human genetics: from SNP to causal allele
There are approximately 10 million common SNPs in the human genome. A fundamental challenge in human genetics is to systematically test each of these 10 million common SNPs for its role in disease. Advances in genomic technology have made this feasible. Contemporary GWASs test several hundred thousand SNPs across the entire human genome, most of which are common (minor allele frequency >5%) in the general, healthy population. To test the remaining more than 9 million common SNPs, the GWAS approach relies on the correlation structure of nearby SNPs. That is, 9 of 10 SNPs are highly correlated, and testing 1 SNP serves to tag the remaining 9 nearby SNPs. This concept is known as linkage disequilibrium (LD).
But the properties of LD that make it powerful for gene mapping also underscore the challenges that remain once an SNP is associated with disease risk; it is unknown if the SNP genotyped (and associated with risk in the genetic study) is the actual causal allele or whether the genotyped/associated SNP is simply in LD with the causal allele. Here causal allele is the single genetic mutation that is responsible for disrupting gene function and giving rise to the phenotype of interest. Given the sheer number of common alleles, the genotyped/associated SNP is most likely just a proxy for the actual causal allele. An example of the correlation structure of an RA risk locus is shown in Fig. 1 .

There is often more than 1 gene in the region of LD that harbors the genotyped/associated SNP, which makes it difficult to pinpoint definitively which gene is the causal gene. On the other hand, there may be no nearby gene in the region of LD. Here causal gene is the single gene that is altered by a mutation to give rise to the phenotype of interest (eg, risk of RA). For convenience, the best biologic gene, based on its known function, is often nominated as the “causal gene.” Fig. 1 illustrates that there are 3 genes in a region of LD at the locus on chromosome 9, 2 of which are very strong biologic candidate genes: TRAF1 (encoding tumor necrosis factor (TNF) α receptor–associated factor 1) and C5 (encoding complement component 5). Thus, this locus is referred to as the TRAF1-C5 RA risk locus.
For most of the 20 RA risk alleles shown in Table 1 , the causal mutation and the causal gene are yet to be identified. Outside of the MHC, the 1 exception is PTPN22 in which the associated mutation alters protein structure and function. Although it may be reasonable to nominate the most likely biologic candidate gene to be the causal gene, direct evidence is not yet available.
There are at least 2 reasons why it is important to identify (or “fine map”) the causal mutation. First, knowing the causal mutation helps guide functional studies. For drug discovery, it is crucial to understand if the risk allele is a gain-of-function or loss-of-function allele. Second, knowing the causal allele provides more accurate estimates of risk that could facilitate disease prediction. If the associated SNP is highly but not perfectly correlated with the causal allele, then risk estimates will be deflated.
A limitation of contemporary GWASs is that they only test common SNPs. For every common allele (defined as having an allele frequency of >5% in the general population), there is at least 1, and likely many more, rare alleles (frequency <5%). In addition, there are other forms of genetic variation besides SNPs, including copy number variants (in which a gene may be duplicated or deleted). Next-generation sequencing and genotyping technologies are required to identify and test rare variants and structural variants.
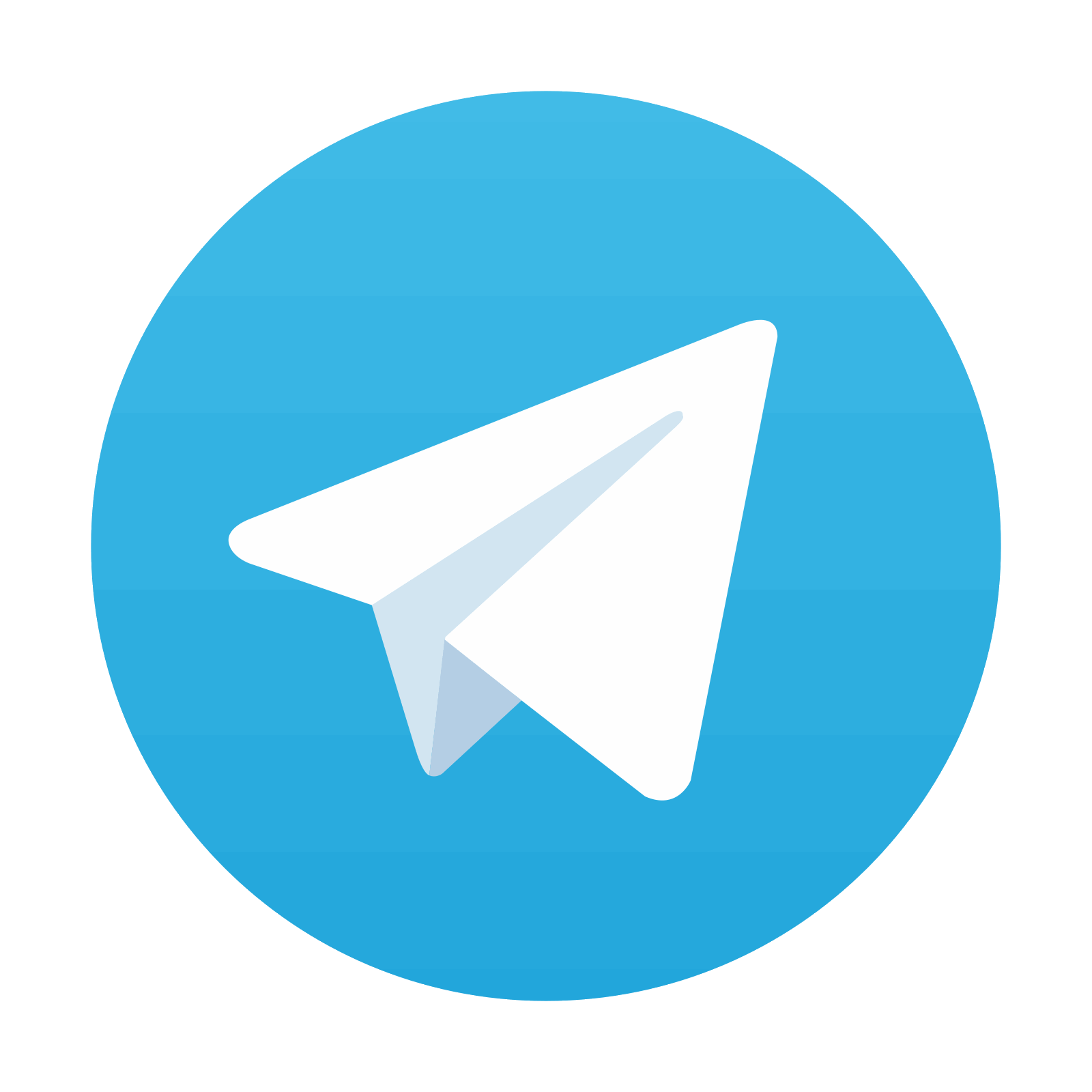
Stay updated, free articles. Join our Telegram channel

Full access? Get Clinical Tree
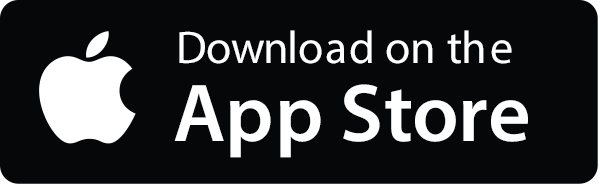
