When viewed from a clinical perspective, few of the common pediatric rheumatic diseases appear to be genetically determined. Family histories are rarely positive for diseases such as juvenile idiopathic arthritis (JIA), and a Mendelian pattern of inheritance would suggest an alternative diagnosis. Pediatric rheumatic diseases share this scenario with autoimmune diseases in general, where the absence of a family history of the specific disease is common, yet a family history of autoimmunity in its various forms is frequent. An exception is spondyloarthritis, which in some families can follow inheritance of human leukocyte antigen (HLA)-B27, although overall penetrance is less than 20%. There is also an expanding list of rare disorders that are often autoinflammatory in nature, caused by single gene defects and inherited in a Mendelian fashion or as a consequence of a new mutation.
The past decade has witnessed remarkable advances in our understanding of the human genome, its variability, and the effects of variants on health and disease. Genetic variability contributes not only to a primary predisposition but also to phenotypic differences, including age of onset and extent and severity of disease, and applies to various forms of JIA and other complex genetic conditions in rheumatology. The well-recognized HLA associations for most of these diseases provide an indication of their genetic nature and involvement of the immune system in pathogenesis. However, although HLA genes may be a necessary part of genetic predisposition, it is now clear that multiple genes outside the major histocompatibility complex (MHC) contribute to risk in a given individual, and there are environmental contributions.
High-throughput technology, including next-generation sequencing, allows for rapid screening of known DNA polymorphisms, routine sequencing of the entire expressed genome (whole exome sequencing), and monitoring the expression of virtually every RNA molecule. The technology is enabling unprecedented discovery of the genetic basis of disease. Understanding how DNA polymorphisms alter gene expression and function (functional genomics) with these basic tools, together with comprehensive and integrative systems biology approaches, will foster a better understanding of human diseases and how to treat them optimally. Here we provide an introduction to the genome with respect to genes, noncoding DNA, and genomic variability, before discussing genetic components of pediatric rheumatic diseases and functional genomic approaches to their understanding.
The Human Genome
Organization and Content
The genome can be defined as an individual’s (or cell’s) total genetic information, and genomics as the science of mapping, sequencing, and analyzing this information. The sequence of the human genome provides the genetic instructions for human physiology. The first draft of the human genome sequence was reported in 2001, followed by a full assembly in 2004. A complete description of The Human Genome Project and its remarkable achievements can be found at the National Human Genome Research Institute ( www.nhgri.nih.gov/HGP/ ).
Protein Coding and Nonprotein Coding DNA
Human genetic information consists of approximately 3.1 billion base pairs of nuclear DNA organized into three components: 22 paired autosomal chromosomes and 2 sex chromosomes. It is striking that less than 2% of our DNA encodes protein (exons), ribosomal RNA (rRNA), or transfer RNA (tRNA). Another 37% contains sequences around or related to genes, such as introns, untranslated regions (UTRs), and pseudogenes. A significant proportion of genetic material is aggregated into repetitive sequences that contribute to the familiar banding pattern that characterizes the morphology of chromosomes. For many years it was thought that much of the noncoding genome was composed of “junk” DNA with little functional significance. However, there has been a transformation in our understanding due in part to the ENCODE Project ( http://www.genome.gov/Encode/ ) intended to produce an Encyclopedia of Functional DNA Elements . It is now estimated that 80% of our genome has some function, including transcription factor binding sites and structural elements for histone binding and chromatin formation, with a great deal of noncoding DNA controlling the complexities of gene regulation.
Protein coding DNA encompasses about 21,500 genes that provide the essential information for all proteins in the human body. However, because a single mRNA can be alternately spliced and proteins can be modified posttranslationally (e.g., proteolytic processing, glycosylation, phosphorylation, acetylation), more than one molecular species of a protein from a single gene can exist. This contributes to the inherent complexity of proteome analysis (discussed later in this chapter). There is both qualitative and quantitative variation between different cell types in the genes expressed. For example, a metabolically complex organ such as the liver may express 15,000 genes, many of which are important for hepatic function; the synovium may well express fewer proteins, varying with stage of development and appropriateness for the function of the tissue. The encyclopedia remains to be completed because many cell types and physiological states of these cell types have yet to be investigated.
Noncoding RNA
Regions of nonprotein coding DNA can be transcribed into noncoding RNAs (ncRNAs) that execute a number of important biological functions. A comprehensive view of this rapidly expanding area of biology is beyond our scope, but a few examples of how ncRNAs function can be instructive ( Fig. 5-1 ). Major classes of regulatory ncRNAs include long noncoding RNA (lncRNA), microRNA (miRNA), small nuclear RNA (snRNA), small nucleolar RNA (snoRNA), self-splicing RNA (ribozymes), and telomerase RNA. Long noncoding RNAs are generally less than 200 nucleotides (nt) in length and are autonomously transcribed from diverse areas of the genome, including intergenic, intronic, and regulatory regions. They hybridize with other species of RNA or DNA and can bind proteins to form scaffolds in chromatin structure, bringing into proximity genes that may be coordinately transcribed despite being distant from one another in the genome. Noncoding RNAs can act in cis or in trans and are crucial regulators of cell differentiation, organ development, and disease processes. MicroRNAs are generated from primary miRNA (pri-miRNA) transcripts of 80 or more nt in length, after enzymatic processing by Drosha and Dicer. Many pri-miRNAs are generated from introns of protein coding genes and can contain more than one miRNA. MicroRNAs bind to mRNA via complementary sequences at their 5′ end and reduce protein expression, either by facilitating rapid mRNA decay, or through translational inhibition. Small noncoding RNAs complex with proteins to form snRNPs (many of which can be targets of autoantibodies such as anti-Sm in lupus) and the spliceosome complex that removes introns from pre-mRNAs in the nucleus, whereas snoRNAs modify rRNA in the nucleolus. Telomerase RNA complexed with protein provides a scaffold and template for telomeric DNA synthesis, thus contributing to genomic stability. This list of ncRNA species, although incomplete, emphasizes the varied and important functions of the noncoding genome. A greater understanding of the role of ncRNA in normal function will lead to a better appreciation of its role in disease.

Sequence Variation
The Human Genome Project provided a reference genome. However, to characterize genetic influences on disease susceptibility, severity, and response to medications, it was necessary to map sequence variation. The 1000 Genomes Project used DNA sequences obtained from geographically distinct populations around the world to catalog 38 million single nucleotide polymorphisms (SNPs), including 1.4 million short insertions and deletions (indels), and 14,000 large deletions. SNPs are often characterized by the frequency of the variant in a population, with common alleles being present in more than 5% of individuals, uncommon in 0.5-5%, and rare in less than 0.5%. About 10 million of the 38 million human SNPs identified (26%) are classified as common . Although the genome and genome structures are broadly the same for all persons (99.9% identity), it is fundamental to the understanding of human diversity and disease susceptibility to recognize that variability is substantial among individuals and can influence health and disease.
The 1000 Genome dataset has been used to provide an estimate of likely pathogenic candidate genes by focusing on rare variants in evolutionarily conserved positions. It is predicted that on average, each person carries between 130 and 400 DNA variants that change a protein’s sequence (non-synonymous coding variants), with 2 to 5 of these variants likely to damage protein function, as well as between 10 and 20 complete loss-of-function variants due to premature stop codons (stop-gains), frameshifts or indels in coding sequence, or disruptions in critical mRNA splice sites. These predicted pathological variants, although they may only alter a single expressed gene product, can have effects ranging from deleterious (including fatal) to neutral, or sometimes even result in a gain of function. Clearly, the nature and site of the change (e.g., coding regions, regulatory regions) is important in this regard and can be reflected in changed phenotypes and disease. Although variability is local, the impact may be devastating for the patient. The complement deficiencies are examples of individual gene variability of relevance to autoimmunity; the chromosome 22 deletion associated with JIA is another such example, which, like trisomy 21 (Down syndrome), may also have considerable effects on the expression of a large number of genes.
Throughout the human genome there is a correlation structure linking genetic variation of different loci. Consequently, knowing the genotype at one locus can provide information about the genotype at a second locus. This correlation between variants at different loci is termed linkage disequilibrium (LD). Preceding the 1000 Genomes Project, an international collaborative effort known as the HapMap Project was undertaken to map LD in the human genome ( http://hapmap.ncbi.nlm.nih.gov.easyaccess2.lib.cuhk.edu.hk/index.html.en ). The HapMap defines regions of LD throughout the genome and identifies SNPs that “tag” these haplotype blocks. The MHC where HLA proteins are encoded is the most comprehensively documented area of LD, with very large haplotype blocks. Specific tag SNPs serve as markers in genome-wide association studies representing large stretches of DNA with highly correlated structure. This facilitates genome-wide testing by limiting the number of SNP genotypes necessary to obtain maximum coverage. The HapMap data has accelerated the search for genes involved in common human diseases, including autoimmune disorders such as JIA and lupus.
The ENCODE project has revealed remarkable insights into gene regulation controlled by nonprotein coding regions of the genome, with implications for human disease. Many regulatory elements at great distance from each other in the linear DNA sequence are physically associated with one another and with expressed genes in live cells. These regulatory regions are statistically associated with sequence variants linked to human disease and will therefore inform our understanding of the consequences of this variation. Together, knowledge of human genome structure and the continued development of functional annotations will potentially improve the power to detect pathological noncoding variants and our understanding of disease mechanisms.
The Epigenome
Epigenetics refers to stably heritable phenotypes resulting from changes in chromosome structure not due to alterations in DNA sequence. Epigenetic changes generally result in structural adaptations of chromosomal regions so as to register, signal, or perpetuate altered activity states. Epigenetic regulation of gene expression occurs through multiple mechanisms, including DNA methylation, histone modifications, and noncoding RNAs ( Fig. 5-2 ).

DNA methylation is the most commonly studied epigenetic mark in the mammalian genome, and involves enzymatic addition of a methyl group by DNA methyltransferases to cytosine residues adjacent to guanosine (CpG). This typically occurs in long stretches of cytosine-guanine repeats referred to as CpG islands , and is often found at or near transcription start sites. These modifications can suppress the binding of transcription factors to gene promoters, alter chromatin structure, or modify methylation-specific regulatory factors, and together play a role in controlling gene expression. The mammalian genome is packaged into nucleosomes, which consist of genomic DNA wrapped around histone octamer scaffolds forming the basic units of chromatin. Chromatin structure is dynamic and serves to regulate access to DNA in response to a variety of signals. The higher order structure of chromatin is dictated not only by enzymatic DNA methylation, but also through posttranslational biochemical modification of histone proteins. Histone modifications are often near the amino terminal end of the protein, which protrudes from the nucleosome structure, as well as within its globular body, where they can directly affect interaction with DNA. In addition to DNA and histone modifications, noncoding RNAs can also regulate the dynamics of the mammalian gene expression and various physiological functions including cell division, differentiation, and apoptosis. Together the features that comprise the epigenome can be thought of as layers controlling gene expression and cellular function, without altering the underlying DNA sequences. Mechanisms controlling their heritability from one cell to its progeny, and in some cases from an entire organism to its offspring, are poorly understood but important areas of investigation. Efforts to comprehensively map epigenetic modifications across the genome are currently under way.
Functional Genomics
In its broadest sense, functional genomics refers to the study of how information encoded in the DNA sequence results in the phenotype of the organism. Understanding the mechanisms that enable genotypes to be translated into phenotypes encompasses what Francis Crick referred to in the 1950s as the “central dogma of molecular biology.” Although the flow of information from DNA to RNA to protein remains a central tenet, it is not entirely unidirectional, and it is becoming increasingly clear that RNA has many biological functions that do not require its information to be translated into protein. In the context of disease, the concept of functional genomics extends to understanding how genetic differences, both common and uncommon variants as well as mutations, lead to altered phenotypes that manifest as autoimmunity or autoinflammation.
The importance of noncoding or regulatory DNA in disease is underscored by the observation that approximately 93% of SNPs identified by genome-wide association studies (GWAS) of common complex genetic diseases or traits lie within in these DNA regions, whereas less than 5% are in coding DNA. This distribution is not disproportionate because only about 2% of the genome encodes exons. Nevertheless, it emphasizes the need to better understand the effects of common SNPs on gene regulatory networks, sometimes acting at great distances across the genome. This is a challenge for modern biology that requires innovative systems biology and bioinformatics approaches.
Transcriptomics
Transcriptome refers to the set of all RNA molecules from protein coding (mRNA) to noncoding RNA, including rRNA, tRNA, lncRNA, pri-miRNA, and others. Transcriptome may apply to an entire organism or a specific cell type. Methods to comprehensively and systematically interrogate the expression of virtually all RNA species have been developed and complement global approaches to studying genome sequence, structure, and its variability, which was described previously in the chapter. Microarray (or “chip”) technology, and more recently high throughput next generation (NextGen) DNA sequencing, has made assessing the transcriptome a routine laboratory practice.
Methods to Assess the Transcriptome
Microarray-based platforms are frequently used to assess comprehensively the relative or absolute abundance of individual RNA transcripts. DNA oligonucleotide arrays use short oligomers with perfect and single-base pair mismatched oligonucleotides to provide a measure of specificity. For analyzing mRNA, samples are reverse transcribed, and then complementary RNA (cRNA) or complementary DNA (cDNA) containing fluorescent-labeled nucleotides is synthesized. The product is hybridized to the chip, and the fluorescent signal intensity, which is proportional to the abundance of the particular mRNA species in the original sample, is measured at each location on the chip using a high-resolution scanner. Multiple vendors sell microarrays that are designed to provide virtually genome-wide interrogation of known genes or exons. Microarrays for measuring the abundance of noncoding RNAs (lncRNA, miRNA, etc.) are also available.
Sequencing-based approaches to gene expression analysis (e.g., RNA-Seq) utilize ultra-high-throughput DNA sequencing methodology. Basically, purified RNA is broken into conveniently sized fragments, converted into cDNA, and then sequenced using random primers. The transcriptome is assembled using a reference genome, and then various bioinformatics tools for further analysis. The number of reads of a given sequence is directly proportional to the expression level and provides an absolute measure of expression. The initial steps of RNA isolation and selection can be modified to preferentially measure protein coding mRNA or small RNA species (miRNA), or remain unbiased if comprehensive transcriptome analysis is desired. RNA sequencing (RNA-Seq) provides certain advantages over DNA oligonucleotide microarrays, including broader transcriptome coverage with the detection of rare or novel transcripts, alternatively spliced forms, and allele-specific expression. In addition, RNA-Seq can provide better quantitation over a broader dynamic range with reduced noise, enabling more subtle changes to be quantitated reliably. A detailed comparison of the strengths and limitations of these approaches to transcriptome analysis is worthwhile before committing significant time and resources to these projects.
Data Analysis
Many aspects of data analysis are specific to the methods used to evaluate the transcriptome, and thus will not be considered here. Nevertheless, a few important points should be considered. Issues of experimental design and quantity, quality, and efficient processing of samples are paramount to obtaining sufficient power to detect statistically significant and biologically meaningful differences. Delayed processing or exposure of cells to heat or cold stress before RNA stabilization can dramatically change gene expression patterns. Estimates of sample sizes depend on the questions being asked, the complexity of the sample (i.e., number of cell or tissue types represented), and variability between samples. Given the issues inherent in the analysis of data generated from tens of thousands of features, conservative P value interpretation and/or multiple testing corrections are often necessary. Several software packages are available for identifying differentially expressed RNAs across multiple samples, recognizing clusters with similar expression, identifying pathways with functional significance, and estimating overall similarity and differences between patterns in complex samples. It is important to recognize that the relative abundance of individual mRNA species does not always correlate with the abundance of the encoded protein. The turnover of many proteins is tightly and specifically regulated. In addition, in complex samples such as tissue, whole blood, or peripheral blood mononuclear cells (PBMCs), where several cell types are represented, increases or decreases in the expression of individual genes may represent differences in the abundance of cell populations.
Validation
Depending on the nature of the study and the conclusions being drawn from the data, there may be a need for validation of RNA expression differences using a second approach. The quantitative real-time polymerase chain reaction (qPCR or real-time PCR) is most commonly used to measure individual complementary DNA that has been produced from the RNA sample. Either a DNA binding dye or fluorescent-labeled oligonucleotides are used to detect an increase in DNA product that accumulates in proportion to the amount of RNA in the original sample. Normalization to RNA species that do not change under the experimental conditions used (often referred to as housekeeping RNAs ) is critical to properly interpret the results.
Functional Genomics in Rheumatic Diseases
Functional genomics approaches have been used successfully to better understand the complexity and pathogenesis of complex rheumatologic diseases. Oligonucleotide microarrays revealed prominent granulopoiesis and type I interferon (IFN) response “signatures” in PBMCs from patients with juvenile and adult-onset systemic lupus erythematosus (SLE), highlighting a role for IFN-α in pathogenesis. The granulopoiesis signature led to the identification of granulocyte precursors that purified with PBMC in new-onset untreated patients. These studies highlighted the type I IFN signature as a potential biomarker for disease activity in SLE, and supported the development of therapeutic agents targeting the IFN axis. Antibodies against IFN-α are currently in clinical development.
In juvenile arthritis, PBMC gene expression differences distinguished patients with polyarticular juvenile rheumatoid arthritis (JRA, American College of Rheumatology [ACR] criteria) from healthy controls, and revealed possible differences between the polyarticular and pauciarticular subtypes and juvenile-onset ankylosing spondylitis (AS). Interestingly, differentially expressed genes in polyarticular JIA patients tended to normalize with response to treatment. Subsequent larger studies of untreated patients at disease onset have further distinguished the major subtypes of JIA, including oligoarticular, polyarticular, systemic, and enthesitis-related arthritis based on PBMC gene expression differences. Gene expression differences in active systemic JIA are quite profound and include evidence for interleukin (IL)-1 and IL-6 signaling, an erythropoiesis signature with overexpression of fetal hemoglobins, innate immune signaling, and downregulation of natural killer cell and T-cell networks.
Gene expression analyses have also revealed substantial heterogeneity in new-onset polyarticular JIA, with three subgroups reflecting varying strengths of three gene expression signatures. One signature (I), most likely from monocytes, correlated with the presence of autoantibodies (RF and anti-CCP) and was present in two groups of polyarticular JIA subjects but not the third. Another signature (III) with low CD8 expression was associated with reduced numbers of CD8 T cells and increased plasmacytoid dendritic cells. Signature III was almost exclusively found in one group of polyarticular JIA subjects, and many of the gene expression differences were consistent with biological effects of transforming growth factor-β (TGF-β). Using approaches like this together with genetic data, it may be possible to improve classification with a genomics and biomarker-based approach.
These studies emphasize that peripheral blood can be a rich source of information, both in terms of biomarkers and pathogenic mechanisms. In JIA this supports the concept that joint inflammation may be an end result of immune dysregulation, rather than simply a site where joint antigens drive a cross-reactive local inflammatory process. In addition, analysis of complex cell mixtures, such as those present in peripheral blood and synovial fluid, can provide useful information despite the complexity of the sample. It has been striking in these and other studies how small changes in RNA abundance can be powerful means of detecting differences in cell populations rather than simply upregulation or downregulation of genes. Development of bioinformatics methods for computational “deconvolution” of transcriptomic data has greatly facilitated interpretation of such studies. The comprehensive nature of transcriptomic approaches affords several advantages, including the ability to measure simultaneously multiple gene products in a pathway, which can be more sensitive and more specific than analyzing individual candidate genes or even cytokines presumed to be driving the signatures. Finally, regardless of the actual identities of the differentially represented transcripts, consistent differences between the groups being compared can serve as gene expression biomarkers that help distinguish disease subtypes, and equally importantly, disease states. Further development of biological correlates of active and inactive disease will enrich our clinical definitions and eventually provide a biological definition of remission. It may be possible with genetic and transcriptomic data to use an integrative approach to predict disease severity and outcome.
The remarkable progress in identifying genetic variants associated with susceptibility to common rheumatic diseases has outpaced our understanding of how these variants impact gene function and disease pathogenesis. Nevertheless, certain principles are beginning to emerge. Using an integrative approach, analyzing genetic data with gene expression as a quantitative trait locus (eQTL), common variants in interferon regulatory factor 7 ( IRF7 ) were shown to influence IFN-α production, with implications for SLE pathogenesis. Using a similar approach, eQTLs that control monocyte gene expression in response to lipopolysaccharide (LPS) were identified for networks involving IFN-β, IRF2 , and others. The eQTLs were significantly more often identified for genes identified by GWAS to be involved in susceptibility to autoimmune disease. Together these studies also highlighted the importance of cell-type–specific and condition-specific responses in establishing links between the genetic variants and immune disorders.
Proteomics
Proteome refers to the entire complement of proteins in a cell type or organism. Although methods to detect and measure the proteome of a cell or tissue have advanced significantly, difficulties remain. Current estimates suggest 21,500 proteins are encoded in the human genome, with the number expressed in any individual cell type being significantly smaller. However, the complexity of the proteome is increased substantially by posttranslational modifications such as glycosylation, phosphorylation, and proteolytic processing, and multiple translation products can derive from one differentially spliced mRNA. Comprehensive proteomic studies may need to consider subcellular localization and interacting partners. Proteins are also inherently more complex than DNA/RNA, with 20 amino acid building blocks rather than 4 primary nucleotides, and they cannot be copied or amplified in vitro. As a result, methods to assess the proteome are less comprehensive and considerably lower throughput than genomic techniques. In addition, because resolution of proteins on two-dimensional (2D) gels depends primarily on two parameters—relative molecular mass (M r ) and isoelectric point (pI)—there may be considerable overlap in a complex mixture containing thousands of cellular proteins. Methods such as mass spectroscopy (MS), which provides precise mass measurements, are highly sensitive and provide greater resolution than 2D gel separations but are expensive and difficult to automate.
Protein Identification by Mass Spectroscopy Peptide Fingerprinting
The identification of individual proteins separated from complex mixtures has become relatively routine. Single protein “spots” from 2D gel separations can be removed from the gel, proteolytically digested into peptide fragments, and subjected to MS analysis. In matrix-assisted laser desorption ionization, the time of flight provides highly precise fragment masses (fingerprints), which are matched against a database of calculated peptide fragment masses from in silico digested proteins based on the specificity of the protease.
It is also possible to obtain peptide sequence information using tandem MS (MS/MS or MS 2 ), where peptide ion fragments in a complex mixture are isolated in the machine due to their mass ( m/z ), and then fragmented in the gas phase. Because peptides will fragment in a sequence-dependent fashion, in most cases an unambiguous ordering of the amino acids can be obtained from the MS/MS spectrum. This technology has been instrumental in determining the sequences of complex mixtures of peptides derived from HLA class I and class II molecules.
Microarray-Based Methods
Protein or antigen microarrays are being used extensively to assess autoantibody profiles from patients with various autoimmune diseases. They offer much higher throughput with smaller sample sizes than traditional enzyme-linked immunosorbent assays (ELISAs) or fluorescence immunoassays. Typically, antigens are immobilized to planar surfaces and reacted with antibody-containing sera or plasma. Antibody-antigen complexes are then visualized with antihuman secondary antibodies conjugated to fluorophores or enzymes, followed by imaging and quantitation. Protein microarrays are more sensitive than conventional ELISAs and offer parallel screening for multiple autoantibodies. The utility of antigen microarrays for screening and discovery is limited by selection bias, because some a priori knowledge of relevant antigens is necessary. More recently, high-density arrays with thousands of non-preselected recombinant proteins have been developed and used to identify novel autoantigens in RA and other diseases.
Protein-protein interactions can be mapped using genetic methods known as “yeast two-hybrid screens.” Using molecular biological tools, a known or “bait” protein can be expressed as a fusion product with the DNA-binding domain of a transcriptional activator. A different protein (“prey”) is expressed as a fusion product with the activation domain of the transcription factor. If the bait and prey interact when expressed in the yeast, the result is activation of transcription of a reporter gene that can easily be detected. This method can be used to study protein-protein interactions of known gene products or to screen entire libraries to discover interaction partners. Variations on this theme have been developed to detect RNA-protein and RNA-RNA interactions.
Methods to Study Gene Function Using Animal Models
To gain a clearer understanding of how a particular gene or its variants function, it is often desirable to turn to animal models. In this section we briefly describe commonly used strategies that have had an important impact on our understanding of disease mechanisms.
Transgenics
DNA introduced into the nuclei of fertilized embryos can incorporate into the host genome and be passed on to subsequent generations. When that DNA encodes a protein, the result is a transgenic animal. Use of foreign genomic DNA with intact regulatory regions can result in tissue- and cell-specific expression and regulation mimicking the pattern seen in the donor organism. Alternatively, cDNA under the control of a nonspecific housekeeping promoter that results in widespread overexpression can be used. Transgenesis is a powerful technique that has been used extensively since the late 1970s, but it has limitations. It usually results in overexpression of the gene of interest; consequently, additional controls need to be considered when interpreting the function of the newly expressed protein.
Targeted Gene Deletion (Knockout) and Knockin Approaches
The discovery and application of homologous recombination led to the production of targeted gene knockouts (KOs) in mice in the late 1980s. Briefly, a DNA construct containing a homologous portion of the gene of interest, but with a key region removed and a selection marker added, is introduced into stem cells derived from blastocysts. With homologous recombination, the gene with the key region removed and the selection marker added replaces the wild-type gene. Cells that have the marker are selected usually based on resistance to a drug, and then reintroduced into blastocysts that are then implanted into pseudopregnant female mice. The offspring contain some cells with the targeted (KO) gene and some with the wild-type gene (mosaics). Subsequent rounds of breeding usually result in germ-line transmission of the KO allele, and then generation of homozygous KOs. Depending on what portion of the gene has been targeted, there may be complete loss of expression or, alternatively, expression of a nonfunctional gene product.
It is often desirable to determine the effects of a gene deletion in selected cell types or tissues. This approach can be helpful to dissect complex phenotypes where the impact of the gene is different in different cell types. Cell- or tissue-specific gene deletion can be achieved using Cre-lox technology to create a conditional KO. In this case the targeted gene recombined into the genome contains the region of interest flanked by newly created loxP recognition sites (“floxed”). The floxed gene can be expressed and function normally. However, co-expression of Cre recombinase, a restriction enzyme that recognizes and cleaves loxP sites, will result in removal of the floxed region and creation of a gene KO, but only in the tissue where Cre is expressed. By driving Cre expression from a tissue-specific promoter, the conditional KO can be generated.
Knockins (KIs) are created in a similar fashion to KOs, except that instead of eliminating a key portion of the gene of interest, that region is replaced with a different coding sequence to generate a variant gene product. Conditional KIs can also be produced. These methods have been used to create disease models in which the effects of human gene mutations can be studied in rodents.
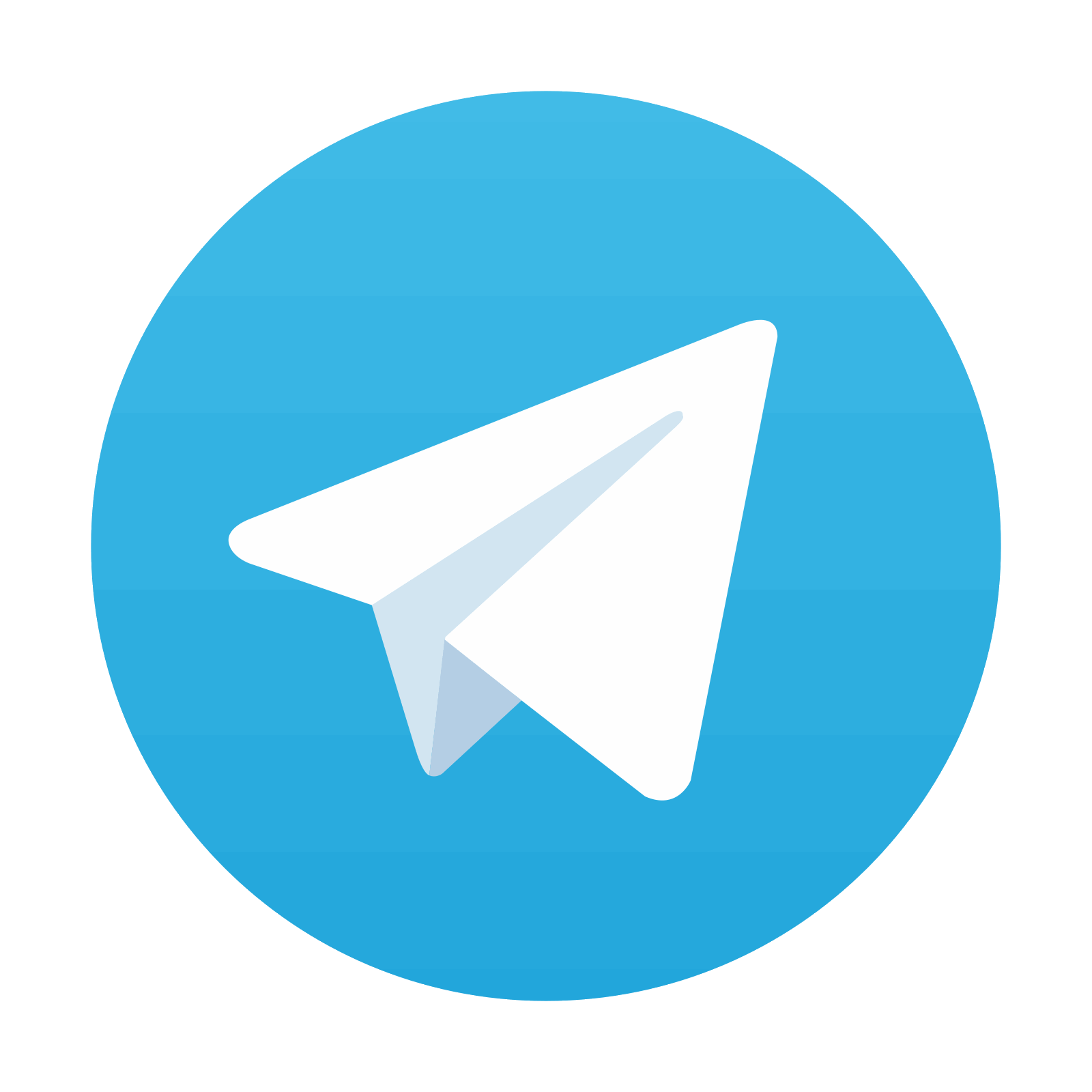
Stay updated, free articles. Join our Telegram channel

Full access? Get Clinical Tree
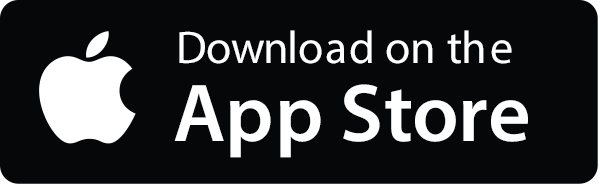
