Introduction
The accuracy of tooth segmentation in intraoral scans is crucial for performing virtual setups and appliance fabrication. Hence, the objective of this study was to estimate and compare the accuracy of automated tooth segmentation generated by the artificial intelligence of dentOne software (DIORCO Co, Ltd, Yongin, South Korea) and Medit Ortho Simulation software (Medit Corp, Seoul, South Korea).
Methods
Twelve maxillary and mandibular pretreatment dental scan sets comprising 286 teeth were collected for this investigation from the archives of the Department of Orthodontics, Faculty of Dentistry, Alexandria University. The scans were imported as standard tessellation language files into both dentOne and Medit Ortho Simulation software. Automatic segmentation was run on each software. The number of successfully segmented teeth vs failed segmentations was recorded to determine the success rate of automated segmentation of each program. Evaluation of success and/or failure was based on the software’s identification of the teeth and the quality of the segmentation. The mesiodistal tooth width measurements after segmentation using both tested software programs were compared with those measured on the unsegmented scan using Meshmixer software (Autodesk, San Rafael, Calif). The unsegmented scans served as the reference standard.
Results
A total of 288 teeth were examined. Successful identification rates were 99% and 98.3% for Medit and dentOne, respectively. Success rates of segmenting the lingual surfaces of incisors were significantly higher in Medit than in dentOne (93.7% vs 66.7%, respectively; P <0.001). DentOne overestimated the mesiodistal width of canines (0.11 mm, P = 0.032), premolars (0.22 mm, P < 0.001), and molars (0.14 mm, P = 0.043) compared with the reference standard, whereas Medit overestimated the mesiodistal width of premolars only (0.13 mm, P = 0.006). Bland-Altman plots showed that mesiodistal tooth width agreement limits exceeded 0.2 mm between each software and the reference standard.
Conclusions
Both artificial intelligence-segmentation software demonstrated acceptable accuracy in tooth segmentation. There is a need for improvement in segmenting incisor lingual tooth surfaces in dentOne. Both software programs tended to overestimate the mesiodistal widths of segmented teeth, particularly the premolars. Artificial intelligence-segmentation needs to be manually adjusted by the operator to ensure accuracy. However, this still does not solve the problem of proximal surface reconstruction by the software.
Highlights
- •
Automatic tooth segmentation using artificial intelligence was tested.
- •
Both software packages showed a high success rate in tooth segmentation.
- •
Despite high success rates, both software showed some limitations.
Diagnostic setup is considered a crucial step in orthodontic diagnosis and treatment planning that allows the clinician to visualize the treatment results, compare different treatment options, and discuss the proposed treatment plan with the patient. Traditionally, diagnostic setup was performed manually on plaster casts by separating the teeth from each other and their base. However, this process is both time-consuming and laborious.
Currently, the use of plaster casts is largely replaced by 3-dimensional (3D) digital casts. This reduces the need for physical storage space and allows easier archiving. Successive technological innovations allowed the use of computer software to perform virtual tooth setup on 3D digital casts, which has been shown to be as reliable as the physical manual setup. More importantly, sequential virtual setups, in which teeth are moved gradually, can be used for the fabrication of clear aligners.
Similar to a physical setup, the first step in performing a virtual setup is the segmentation of the individual teeth to allow the manipulation of each tooth separately. With the advent of artificial intelligence (AI) in dentistry, AI technology was used in automating virtual tooth segmentation. The accuracy of tooth segmentation is crucial for subsequent virtual setup or model analysis. The determination of the gingival margin has been found to be a challenge for AI tooth segmentation. , In addition, reproducing the interproximal areas in the virtual models has been shown to be rather difficult because of the close contact between neighboring teeth, especially in the case of crowding. As a consequence, this may result in shorter dental arch lengths on virtual setups than on physical plaster model setups.
Several software packages that can be used to perform virtual setups have been introduced over the past years. Some of the more commonly used ones are OrthoCAD (Align Technology, San Jose, Calif), Ortho Studio (Maestro 3D Dental Studio; AGE Solutions, Pontedera, Italy), OrthoAnalyzer (3Shape, Copenhagen, Denmark), and uDesign (uLab systems, Inc, Memphis, Tenn). Medit Ortho Simulation (Medit Corp, Seoul, South Korea) is a free commercially available software that uses AI to automatically segment teeth and perform virtual setups. Recent research has shown that virtual setups performed using Medit software were less time-consuming than manual setups. Nonetheless, the authors noted that the mesiodistal (MD) width of the teeth was reduced because of the inability of the software to capture the contact areas correctly during automatic segmentation. Another free commercially available software is dentOne (DIORCO Co, Ltd, Yongin, South Korea), which can be used for automatic tooth segmentation and alignment for the fabrication of aligners. However, to our knowledge, its performance has not been previously evaluated in the published literature. In addition, no published research has compared the accuracy of automated tooth segmentation using the abovementioned commercially available software packages.
Hence, this study aimed to estimate and compare the accuracy of automated tooth segmentation using Medit Ortho Simulation and dentOne software in terms of the success and/or failure of the segmentation. In addition, the accuracy of MD tooth width after segmentation by both software was determined in reference to the unsegmented scans.
Material and Methods
This in silico study of AI was approved by the institutional review board of the Faculty of Dentistry, Alexandria University (IORG:0008839, Ethics Committee no. 0717-06/2023).
The sample size was calculated assuming a 5% α error and 80% study power. According to Im et al, the comparison between an automated and a semiautomated tooth segmentation software yielded success rates of 87.86% and 97.26%, respectively. The required sample size was 148 pairs on the basis of differences between 2 paired proportions using the McNemar test. This was increased to 165 pairs to compensate for possible nonreads by either software package and to account for uneven clustering of teeth within the subjects. This sample requires approximately 12 digital dental models. The sample size estimation was done using the online sample size calculator Statulator.
The pretreatment orthodontic records of patients treated at the Department of Orthodontics, Faculty of Dentistry, Alexandria University, were screened for eligibility by the principal investigator. The inclusion criteria for record selection included patients aged >14 years, permanent dentition, and the availability of digital dental models that are free from any defects in the teeth and/or the gingiva. All the digital dental models were obtained using a CEREC Omnicam intraoral scanner (Dentsply Sirona, Bensheim, Germany) and were exported in standard tessellation language (STL) file format. Digital dental models that had missing or supernumerary teeth, multiunit fixed prosthodontics, or partially erupted teeth were excluded.
All the eligible dental scans were then deidentified and coded by the principal investigator before the commencement of experimental procedures. All the records in the department are collected after informed consent from the patients and their guardians, covering the use of their anonymized records for research purposes.
The intraoral scans were imported in STL file format into dentOne software and Medit Ortho Simulation software. The digital scans were then oriented in both software packages, a preliminary step required in the workflow of each software before further processing. One researcher (Y.M.Y.) executed the automatic segmentation of the digital scans in both Medit and dentOne software. The accuracy of tooth segmentation was evaluated in each respective software interface.
To evaluate MD tooth width, the segmented models were exported as STL files, and the unsegmented scans were imported into Meshmixer software (Autodesk, San Rafael, Calif), in which the MD width of each tooth was measured using the analysis tool. The MD tooth width was measured along the central groove from the mesial to the distal contact points of the posterior teeth and from the mesial to the distal line angles on the palatal or lingual surfaces of the incisors and canines ( Fig 1 ). Measurements were performed on both the segmented scans and the unsegmented scans in Meshmixer. Unsegmented scans served as the reference gold standard. One researcher (M.A.T.) performed all the MD measurements. Tooth size-arch length discrepancy was measured according to the Lundström segmental analysis method.

To evaluate the success and/or failure of automated segmentation by each software package, the following parameters were determined:
- 1.
Identified vs unidentified: the tooth was considered identified if AI numbered the tooth. Teeth that were not numbered were considered unidentified ( Fig 2 ).
Fig 2
Failure of tooth identification by the software: A, Failure to identify teeth number 25 and 26, and wrong tooth order in Medit Ortho Simulation; B, Failure to identify tooth number 26 in dentOne software.
- 2.
Identified teeth were evaluated for the quality of segmentation. The segmentation was considered adequate if the segmented scan included the entire tooth surface to the gingival margin. Faulty segmentations were classified according to the involved surface (occlusal, vestibular, and/or lingual) and whether the fault is a deficit (occlusal, vestibular, or lingual) or a surplus (vestibular and/or lingual), as shown in Figure 3 , A-J .
Fig 3
Classification of faulty segmentations in Medit Ortho Simulation: A, Occlusal deficit; B, Labial surplus; C, Labial deficit; D, Lingual surplus; E, Lingual deficit; and classification of faulty segmentations in dentOne: F, Occlusal deficit; G, Labial surplus; H, Labial deficit; I, Lingual surplus; J, Lingual deficit.
Because of the nature of the study, the operator could not have been masked during the assessment of each of the tested software packages. However, the operator was masked when measuring the MD dimensions of the teeth on the STL models.
Six randomly selected digital dental scans were reanalyzed by (M.A.T.) and (Y.M.Y.) to test intraexaminer and interexaminer reliability. Fleiss’ κ was calculated for categorical variables, and intraclass correlation coefficients (ICC) were calculated for MD tooth width measurements.
Statistical analysis
Frequencies and proportions were calculated for categorical variables to demonstrate success rates in which the scores “adequate” and “identified” were considered a success, whereas the scores “unidentified,” “deficit,” and “surplus” were considered failures. Success rates were compared between Medit and dentOne using the McNemar test of related proportions. Agreement between the 2 software packages was tested using Fleiss’ κ for categorical variables. Mean and standard deviation values were calculated for Medit, dentOne, and manual measurements and compared using repeated measures analysis of variance followed by post-hoc Bonferroni test. Bland-Altman plots and ICCs were both used to test the agreement of MD tooth width between the 3 methods. The significance level was set at P ≤0.05. All statistical analyses were conducted using Jasp software (version 0.17.3; Jasp Team 2023, Department of Psychological Methods, University of Amsterdam, Amsterdam, The Netherlands, www.jasp-stats.org/ ).
Results
Twelve maxillary and mandibular pretreatment dental scan sets comprising 286 teeth were analyzed. Tooth size – arch length discrepancy ranged from −5.83 to 5.50 mm, with a mean of 0.015 ± 3.190 in the maxillary scans, and −9.09 to 4.77 mm, with a mean of −0.448 ± 3.470 in the mandibular scan.
All the variables exhibited high reliability ( Supplementary Table I ). Fleiss’ κ for categorical variables ranged 0.973-0.999 for intraexaminer agreement and 0.906-0.999 for interexaminer agreement. ICC for the MD tooth width was 0.991 (95% confidence interval [CI], 0.993-0.989) and 0.992 (95% CI, 0.989-0.994) for intraexaminer and interexaminer agreements, respectively.
The success and/or failure of tooth segmentation in Medit and dentOne is depicted in Figure 4 and stratified by tooth type in Table I . Both software packages showed a high success rate in tooth segmentation. Success rates of Medit and dentOne in tooth identification were 99% (97.9%-100.0%) and 98.3% (95.8%-100.0%), respectively. An incidental finding was the wrong tooth number in Medit’s segmentation of 1 scan, in which 4 teeth were incorrectly numbered ( Fig 2 ).

Tooth type | Medit | dentOne | P value † |
---|---|---|---|
Identification | |||
Incisor | n = 96 | n = 96 | |
Not identified | 1 (1.0%) | 0 (0.0%) | |
Identified | 95 (98.9%) | 96 (100.0%) | >0.999 |
Canine | n = 48 | n = 48 | |
Not identified | 0 (0.0%) | 1 (2.1%) | |
Identified | 48 (100%) | 47 (97.9%) | >0.999 |
Premolar | n = 94 | n = 94 | |
Not identified | 1 (1.1%) | 2 (2.1%) | |
Identified | 93 (98.9%) | 92 (97.9%) | >0.999 |
Molar | n = 48 | n = 48 | |
Not identified | 1 (2.1%) | 2 (4.2%) | |
Identified | 47 (97.9%) | 46 (95.8%) | >0.999 |
Total | n = 286 | n = 286 | |
Not identified | 3 (1.0%) | 5 (1.7%) | |
Identified | 283 (99.0%) | 281 (98.3%) | 0.727 |
Occlusal | |||
Premolar | n = 93 | n = 92 | |
Deficit | 8 (8.6%) | 4 (4.3%) | |
Adequate | 85 (91.4%) | 88 (95.7%) | 0.344 |
Molar | n = 47 | n = 46 | |
Deficit | 6 (12.8%) | 2 (4.3%) | |
Adequate | 41 (87.2%) | 44 (95.7%) | 0.219 |
Total | n = 140 | n = 138 | |
Deficit | 14 (10.0%) | 6 (4.3%) | |
Adequate | 126 (90.0%) | 132 (95.7%) | 0.077 |
Vestibular | |||
Incisor | n = 95 | n = 96 | |
Deficit | 0 (0.0%) | 6 (6.2%) | |
Adequate | 93 (97.9%) | 88 (91.7%) | 0.070 |
Surplus | 2 (2.1%) | 2 (2.1%) | |
Canine | n = 48 | n = 47 | |
Deficit | 1 (2.1%) | 3 (6.4%) | |
Adequate | 45 (93.8%) | 44 (93.6%) | >0.999 |
Surplus | 2 (4.2%) | 0 (0.0%) | |
Premolar | n = 93 | n = 92 | |
Deficit | 0 (0.0%) | 3 (3.3%) | |
Adequate | 93 (100.0%) | 89 (96.7%) | |
Surplus | 0 (0.0%) | 0 (0.0%) | 0.250 |
Molar | n = 47 | n = 46 | |
Deficit | 4 (8.5%) | 8 (17.4%) | |
Adequate | 43 (91.5%) | 38 (82.6%) | 0.277 |
Surplus | 0 (0.0%) | 0 (0.0%) | |
Total | n = 283 | n = 281 | |
Deficit | 5 (1.8%) | 20 (7.1%) | |
Adequate | 274 (96.8%) | 259 (92.2%) | 0.014 |
Surplus | 4 (1.4%) | 2 (0.7%) | |
Lingual | |||
Incisor | n = 95 | n = 96 | |
Deficit | 5 (5.3%) | 30 (31.2%) | |
Adequate | 89 (93.7%) | 64 (66.7%) | <0.001 |
Surplus | 1 (1.1%) | 2 (2.1%) | |
Canine | n = 48 | n = 47 | |
Deficit | 1 (2.1%) | 4 (8.5%) | |
Adequate | 46 (95.8%) | 43 (91.5%) | 0.625 |
Surplus | 1 (2.1%) | 0 (0.0%) | |
Premolar | n = 93 | n = 92 | |
Deficit | 4 (4.3%) | 2 (2.2%) | |
Adequate | 85 (91.4%) | 85 (92.4%) | 0.999 |
Surplus | 4 (4.3%) | 5 (5.4%) | |
Molar | n = 47 | n = 46 | |
Deficit | 3 (6.4%) | 2 (4.3%) | |
Adequate | 43 (91.5%) | 40 (87.0%) | 0.727 |
Surplus | 1 (2.1%) | 4 (8.7%) | |
Total | n = 283 | n = 281 | |
Deficit | 13 (4.6%) | 38 (13.5%) | |
Adequate | 263 (92.9%) | 232 (82.6%) | <0.001 |
Surplus | 7 (2.5%) | 11 (3.9%) |
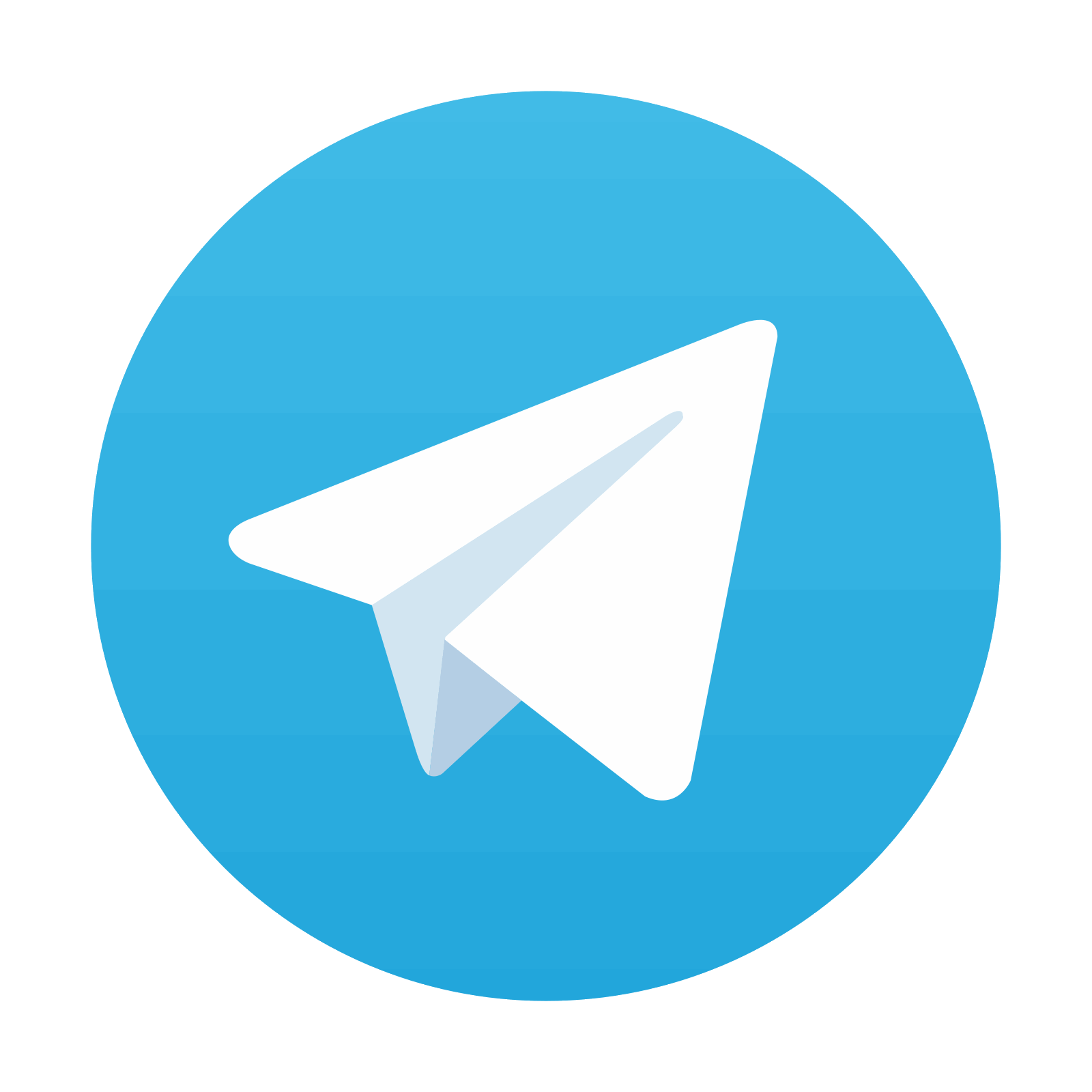
Stay updated, free articles. Join our Telegram channel

Full access? Get Clinical Tree
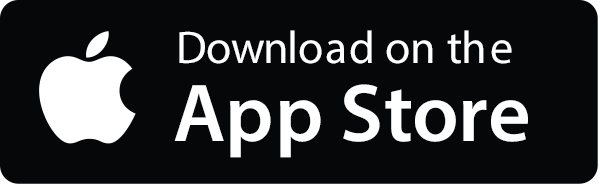

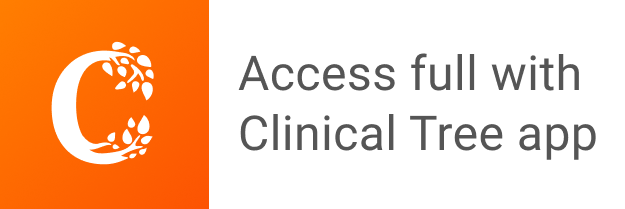