There are many challenges to conducting valid epidemiological research of work-related musculoskeletal disorders and interpreting reports describing the results. In particular, these concern the basic study design, selection of subjects, measurement of exposure and outcome, control of confounding and the limitations of workers’ compensation data systems. Researchers and people interested in the research results need to be aware of the major potential problems and pay careful attention to them when designing, conducting and using the results of such research.
The design and conduct of a study importantly affect the validity and usefulness of the results produced by that study. Where the design and conduct are not ideal, bias usually results. This is the fundamental challenge of the design, conduct and interpretation of epidemiological research. Bias does not imply a conscious misrepresentation of study results, but describes the situation where the study results differ from the truth for systematic reasons, that is, for reasons related to the design and conduct of the study rather than simply due to chance. Epidemiological research involving musculoskeletal conditions is particularly vulnerable to many of the methodological problems that can arise, especially when the exposures of interest are occupational. Fortunately, valid and useful study findings can be obtained and used, if the investigator and the reader are aware of the potential problems and pay careful attention to them. This article aims to describe the most important challenges faced by researchers conducting epidemiological research involving musculoskeletal conditions in the occupational setting and to consider some of the potential solutions, using real examples to illustrate the issues.
Issues related to study type
A range of study types can be used in epidemiology, and probably all have been used at some time or another in studies of occupational musculoskeletal disorders. Randomised controlled trials, which involve randomly allocating study subjects to two or more different exposures, usually provide the highest level of evidence from a single study, as they minimise issues of selection bias and confounding. However, they are not commonly conducted in the occupational setting because of the practical and sometimes ethical difficulties in randomising some workers to one exposure and others to a different exposure. Allocating one worker to an exposure different from that of a fellow worker is practically difficult because it is hard to have different equipment, different workplace design or different tasks at the same workplace, and also because it is hard to prevent one worker being exposed in some form to the intervention allocated to another worker. This cross-contamination of allocated exposures diminishes the difference between study groups, making it harder to detect any difference in effect, if one exists. Ethical issues arise because workers should always be provided with a safe working environment, and if there is good reason to believe that a new approach would be safer, all workers should receive the benefit of that new approach. This should not be a problem, if there is no definitive evidence that the new approach is beneficial (which presumably would be the case if it is the subject of study), but commonly there is an informed suspicion about the benefits or otherwise of an exposure, and workers could understandably want the opportunity to benefit from that exposure, especially if they see that a fellow worker has that opportunity. One approach that can be used to overcome the practical issues is to use a cluster-randomised design, which involves randomising the work setting rather than the individual worker. All workers within a workplace therefore have the same exposure. A recent example of this was a study of the use of core stabilisation exercises compared with the traditional sit-up training in United States soldiers. The unit of randomisation was the company, with all soldiers in the company assigned to the same intervention (core stabilisation or sit-up training). The study found no important difference in the apparent incidence of musculoskeletal injuries in the two exercise groups .
Cohort studies involve identifying a group of subjects with a common exposure and following them over time to determine the incidence of the condition of interest, this incidence usually being compared with another group of subjects with a different exposure. This design is commonly used in occupational musculoskeletal studies to examine incidence, to identify problem exposures and to assess the effectiveness of interventions. Cohort studies are particularly prone to problems involving selection, measurement of the outcome (the disorder of interest) and confounding. If these problems are addressed well, a cohort study can provide strong evidence regarding the study question of interest.
Case-control studies require the identification of a well-defined study base (a group of people in a given place over a given time), identification of those with the disorder of interest (the cases) and those without the disorder of interest (the controls or referents) and the measurement and comparison of exposure proportions in these cases and controls. Case-control studies are used in occupational musculoskeletal research, but are prone to selection problems involved in the study base and selection of appropriate controls and to difficulties in making an unbiased measurement of exposure. Case identification is difficult in musculoskeletal disorders because there are few sources of information (such as registers) that allow identification of all the cases or an unbiased sample selected from them. Where this issue can be overcome, and the valid measurement of exposure achieved, case-control studies can also provide useful information about the role of work factors in musculoskeletal disorders.
Cross-sectional studies have been commonly used in epidemiological research of occupational musculoskeletal conditions, typically because this type of study is reasonably easy to undertake and usually provides a quick result. Unfortunately, the problems that commonly arise in terms of selection, measurement and confounding mean that many of the studies have provided weak and sometimes misleading evidence on a particular topic. Most importantly, at the time of measurement, the affected workers may have stopped working or moved jobs, leaving only a survivor population or a workplace where the affected workers have moved to low-exposure jobs and the non-affected workers remain in high-exposure jobs, leading to paradoxical findings . Notwithstanding this, many such studies have suggested links between occupational exposures and musculoskeletal disorders that have been able to be investigated in more definitive studies.
In recent years, systematic reviews (often including meta-analysis) that consider the relationship of occupational exposures to various musculoskeletal disorders have been published, as well as guides to the conduct and reporting of systematic reviews . When conducted well, and when the studies included in the reviews are of good quality, systematic reviews can provide powerful evidence regarding an exposure–disorder relationship, or beneficial intervention, by summarising the evidence from all relevant studies. However, systematic reviews are subject to biases just as other study approaches are. These are well described in a number of published sources . Most importantly, the evidence provided by systematic reviews relies crucially on the quality of the studies they include, and if good evidence does not exist, a systematic review is unlikely to provide any worthwhile information. Using a poorly focussed question, not identifying all relevant studies, selective publication of studies with particular findings resulting in only a subset of conducted studies being available for inclusion (publication bias), the inclusion of poor-quality studies, and combination of studies that really were investigating different study questions or populations with different exposure–outcome relationships (heterogeneity) can all detract from the validity of a systematic review. Systematic reviews were developed initially to summarise data from randomised controlled trials, but have increasingly been used for observational studies, making it more difficult to be confident that the studies are of appropriate methodological quality; this is because these studies are more susceptible to confounding and selection bias than trials. Publication bias is also harder to exclude because, unlike for trials, there are no registers of observational studies.
Inherent in the consideration of study type is whether the study is likely to provide information primarily related to association (the occurrence of one factor with another) or to causation (a clear causal connection between presence of a harmful or beneficial risk factor and the later occurrence or prevention of an outcome). Except in descriptive studies, which provide information on incidence or prevalence, epidemiological research aims to provide information on causation. Due to their design and the limitations commonly associated with them, different study types provide information of varying strength in terms of causality. In most instances, cross-sectional studies should only be viewed as providing information on association, whereas randomised controlled trials commonly provided information suggestive of causation (or lack of causation). Cohort studies and case-control studies are typically designed to provide information suggestive of causation, but the strength of evidence provided by a single such study is usually insufficient to strongly support causation. Systematic reviews can assist by combining information from single studies, with consistent findings providing stronger evidence of a causal connection between risk factor and outcome. However, as mentioned, the strength of their evidence is reliant on the methodological quality of the studies they summarise.
Issues related to selection methods
Selection bias arises when some aspect of the process of selecting or monitoring subjects causes the measure of effect or occurrence to be wrong. The main causes of this are that the study subjects do not properly represent the population from which they come, comparison groups differ in important ways apart from exposure and subjects drop out during the study.
Studies of the prevalence or incidence of a condition aim to provide information on how common a problem is, or how commonly it occurs. Such studies are typically based on a sample of a population rather than a whole population. The selection of subjects therefore must be conducted in such a way that ensures the sample is representative of the whole population. Typically, this is done by taking a random sample of a population, although non-participation by selected subjects often potentially undermines representativeness of the selection. For example, a recent study of occupational musculoskeletal exposures in women selected subjects from a random sample of telephone listings, but, after mailing 1300 letters and identifying 460 eligible subjects, only 123 people actually participated in the study, and the relative lack of Hispanic, low-income, young and blue-collar women suggested that the study sample probably was not representative of the population in which the study was based. Whether this produced biased results was not clear, but the results would clearly have to be interpreted with these potential biases in mind . Approaches to sampling, which are not random, are even more likely to introduce the possibility of obtaining a non-representative sample, with the associated risk that the results will be biased. For example, a study of musculoskeletal disorders in Nigerian nurses had an 80% response rate, but did not send the questionnaires to a random sample of potential subjects, making it difficult to determine to which population the study results were applicable .
A fundamental aspect of any epidemiological study is that the study groups being compared are similar in all important aspects apart from the exposure of interest. In practice, this can be very difficult to achieve, especially in occupational studies. When assessing whether an exposure increases (or decreases) the risk of developing a certain musculoskeletal disorder, a group of employed persons may be identified and their illness experience compared with that of the general population of a similar age and gender. This is a superficially attractive approach, but can be the source of important bias. Typically, depending on the type of occupation, employed persons are healthier than persons, who are not employed. Therefore, even if work has no effect on the health of the worker, it would be expected that an employed population will be healthier than the general population. A problem exposure in a workplace may therefore go unnoticed, or be under-recognised because the increase in risk resulting from the exposure is offset by the underlying lower risk of the disorder in the working population compared with the general population. This is an example of the so-called healthy worker effect . a phenomenon where fit people tend to be more likely to be employed or more likely to remain at work than people in the general population. Comparison of an employed population to the general population is therefore often not appropriate because the employed population would be expected to be healthier (i.e., to have a lower incidence and prevalence of most conditions) than the general population, irrespective of any problematic (or beneficial) exposures there might be in the workplace.
Therefore, rather than comparing to the general population, a specific working population could be used for comparison, but choosing an appropriate comparison population can be difficult. For example, what is the appropriate comparison population for a study of shoulder pain in factory workers on a production line or for a study of knee problems in miners? If the exposure of interest is factory production-line work or work as a miner, then clearly other factory production-line workers or miners, respectively, would not be appropriate comparison groups. Even workers in different occupations may have similar relevant exposures in their occupations. Hence, for example, factory production-line workers may be found to have the same incidence of new-onset shoulder pain as carpenters, but the incidence may still be much higher than in, say, office workers, because the causative exposure occurs in the production-line workers and the carpenters but not in the office workers. Presumably, office workers would be a more appropriate comparison group in that instance. This is one of the main reasons why office workers, managers or clerical officers are commonly used as the reference group for studies of musculoskeletal disorders in workers whose occupations entail exposure to considerable physical forces. Another complicating factor is that workers’ attributes may influence the occupation in which they work, and these attributes may be important determinants of their susceptibility to certain disorders. If those disorders are the focus of interest, that group may not be appropriate to use for comparison. The choice of comparison groups also needs to take into account how the study information will be used. Will it be useful to know, for example, that factory production-line workers have a higher incidence of new-onset shoulder pain than office workers? What intervention could usefully arise from this finding? In this instance, the office worker comparison group probably would be useful because, presumably, if such a study showed a higher incidence of shoulder injury in factory production-line workers, this would suggest that factory production-line workers should be studied more closely to determine which tasks are undertaken that increase the risk of shoulder pain. Having identified these tasks, they can be the focus of interventions to decrease the incidence of the shoulder problems.
Once a study has commenced, it is common for subjects to formally withdraw, no longer respond or be lost to follow-up. This can occur for a variety of reasons. These ‘dropouts’ are not of themselves a problem. They only matter if they introduce bias, and this should only occur, if those who drop out differ in an important way compared with those who do not drop out, and, particularly, if they drop out or change because of the exposure of interest. For example, in a cohort study of carpal tunnel syndrome in supermarket check-out operators, it would be a problem if those who developed symptoms changed jobs or left the company because they had developed symptoms, as this would lead to an underestimate of the incidence of the condition. However, if some subjects left the study because they moved away from the area or changed jobs, and these changes were not related to having developed symptoms or their likelihood of developing symptoms, then such loss of subjects should not introduce any bias. A clear illustration of this occurred in a study of the relationship between occupational physical activity and back pain. The study did find a clear relationship between the two, but also found that people who had a high physical load and subsequently developed back pain were more likely to change to jobs that had a lower physical load, compared with persons who had a high physical load but did not develop back pain, and compared with persons who did not have a high physical load at baseline . This issue was able to be identified because the study used a cohort design, but it would have lead to biased and misleading results if a cross-sectional approach had been used.
The key problem in this area is that it is often difficult to find information about why people left, and hard to be confident what the effect might have been even if these reasons for leaving are known. An understanding of the potential effect of such losses can be gained by assuming that all the dropouts did, or did not have, the condition of interest. For example, in a postal survey of nurses, postal workers and office workers in New Zealand, the 12-month prevalence of musculoskeletal disorders was found to be 88%. However, the response rate was only about 58%. It might be expected that people with a musculoskeletal problem would be more likely to respond to a survey about musculoskeletal problems than people who do not have such problems, which would mean that the 88% was an overestimate of the true prevalence. To investigate the possible extent of this effect, the authors showed that even if all of the non-responders did not have a musculoskeletal problem, the prevalence would still have been 51% .
Study methods that minimise the number of dropouts obviously minimise the potential for bias arising from this source. The best example of this is probably the use of population databases that record information on the relevant disorder of interest. Little work is required by the investigators to obtain the information and it is rare that no information can be found regarding a subject, meaning that there are few dropouts. Unfortunately, most countries do not have population registers of musculoskeletal disorders, or do not allow the necessary linkage of personal data between registers. An example of where this was possible was a Swedish study of osteoarthritis of the knee and hip in construction workers. The outcome was defined as surgically treated osteoarthritis in construction workers because information on this surgery was available from the Swedish Hospital Discharge Register. Details of the study subjects were linked to the Register, allowing identification of subjects whose primary presenting problem was osteoarthritis and whose primary reason for admission was a first hip or knee replacement. Of the subjects who enrolled in the study, only 0.15% were excluded because they could not be found in any of the relevant official Swedish registers, and the hospital register had virtually complete national coverage .
A specific selection issue can arise in case-control studies when the cases and controls do not come from the same study base, or do not represent the population of cases and controls within the study base. In occupational musculoskeletal studies, this might occur when cases are identified from a hospital or clinic, as these sites usually do not contain all the cases, and the cases that are identified from these sites commonly are not representative of all the relevant cases. This problem is compounded if the controls are all obtained via a hospital or clinic rather than from the general community from which the cases come, and if a considerable proportion of the eligible controls do not participate in the study. A recent study of the relationship of occupation to the occurrence of supraspinatus tendon tears illustrates some of these issues. Cases were persons whose shoulder pain caused them to have a magnetic resonance imaging (MRI) scan at any of 14 radiology practices in two regions in Germany. Controls were a random sample of the population from the two regions. Forty-eight per cent of eligible cases and 54% of eligible controls agreed to participate. Were the participating cases representative of all cases? As only 48% of the eligible cases participated, and there may have been other radiology centres in the region, and not all people with supraspinatus tears develop pain, it is likely they were not. People who believed their shoulder injury was related to their work may have been more likely to agree to become involved in the study than those who did not have that suspicion. The controls were appropriately drawn from the general population from which it was presumed the cases arose, but there was little information on the controls who did not participate. Therefore, it is difficult to know whether they were representative of all controls. The study found a dose–response relation between length of time working with hands raised above the shoulder and the occurrence of supraspinatus tear causing pain. However, the information on case and control selection and participation suggests considerable scope for selection bias in the study, and illustrates the difficulties in conducting this sort of research in the real world .
Issues related to selection methods
Selection bias arises when some aspect of the process of selecting or monitoring subjects causes the measure of effect or occurrence to be wrong. The main causes of this are that the study subjects do not properly represent the population from which they come, comparison groups differ in important ways apart from exposure and subjects drop out during the study.
Studies of the prevalence or incidence of a condition aim to provide information on how common a problem is, or how commonly it occurs. Such studies are typically based on a sample of a population rather than a whole population. The selection of subjects therefore must be conducted in such a way that ensures the sample is representative of the whole population. Typically, this is done by taking a random sample of a population, although non-participation by selected subjects often potentially undermines representativeness of the selection. For example, a recent study of occupational musculoskeletal exposures in women selected subjects from a random sample of telephone listings, but, after mailing 1300 letters and identifying 460 eligible subjects, only 123 people actually participated in the study, and the relative lack of Hispanic, low-income, young and blue-collar women suggested that the study sample probably was not representative of the population in which the study was based. Whether this produced biased results was not clear, but the results would clearly have to be interpreted with these potential biases in mind . Approaches to sampling, which are not random, are even more likely to introduce the possibility of obtaining a non-representative sample, with the associated risk that the results will be biased. For example, a study of musculoskeletal disorders in Nigerian nurses had an 80% response rate, but did not send the questionnaires to a random sample of potential subjects, making it difficult to determine to which population the study results were applicable .
A fundamental aspect of any epidemiological study is that the study groups being compared are similar in all important aspects apart from the exposure of interest. In practice, this can be very difficult to achieve, especially in occupational studies. When assessing whether an exposure increases (or decreases) the risk of developing a certain musculoskeletal disorder, a group of employed persons may be identified and their illness experience compared with that of the general population of a similar age and gender. This is a superficially attractive approach, but can be the source of important bias. Typically, depending on the type of occupation, employed persons are healthier than persons, who are not employed. Therefore, even if work has no effect on the health of the worker, it would be expected that an employed population will be healthier than the general population. A problem exposure in a workplace may therefore go unnoticed, or be under-recognised because the increase in risk resulting from the exposure is offset by the underlying lower risk of the disorder in the working population compared with the general population. This is an example of the so-called healthy worker effect . a phenomenon where fit people tend to be more likely to be employed or more likely to remain at work than people in the general population. Comparison of an employed population to the general population is therefore often not appropriate because the employed population would be expected to be healthier (i.e., to have a lower incidence and prevalence of most conditions) than the general population, irrespective of any problematic (or beneficial) exposures there might be in the workplace.
Therefore, rather than comparing to the general population, a specific working population could be used for comparison, but choosing an appropriate comparison population can be difficult. For example, what is the appropriate comparison population for a study of shoulder pain in factory workers on a production line or for a study of knee problems in miners? If the exposure of interest is factory production-line work or work as a miner, then clearly other factory production-line workers or miners, respectively, would not be appropriate comparison groups. Even workers in different occupations may have similar relevant exposures in their occupations. Hence, for example, factory production-line workers may be found to have the same incidence of new-onset shoulder pain as carpenters, but the incidence may still be much higher than in, say, office workers, because the causative exposure occurs in the production-line workers and the carpenters but not in the office workers. Presumably, office workers would be a more appropriate comparison group in that instance. This is one of the main reasons why office workers, managers or clerical officers are commonly used as the reference group for studies of musculoskeletal disorders in workers whose occupations entail exposure to considerable physical forces. Another complicating factor is that workers’ attributes may influence the occupation in which they work, and these attributes may be important determinants of their susceptibility to certain disorders. If those disorders are the focus of interest, that group may not be appropriate to use for comparison. The choice of comparison groups also needs to take into account how the study information will be used. Will it be useful to know, for example, that factory production-line workers have a higher incidence of new-onset shoulder pain than office workers? What intervention could usefully arise from this finding? In this instance, the office worker comparison group probably would be useful because, presumably, if such a study showed a higher incidence of shoulder injury in factory production-line workers, this would suggest that factory production-line workers should be studied more closely to determine which tasks are undertaken that increase the risk of shoulder pain. Having identified these tasks, they can be the focus of interventions to decrease the incidence of the shoulder problems.
Once a study has commenced, it is common for subjects to formally withdraw, no longer respond or be lost to follow-up. This can occur for a variety of reasons. These ‘dropouts’ are not of themselves a problem. They only matter if they introduce bias, and this should only occur, if those who drop out differ in an important way compared with those who do not drop out, and, particularly, if they drop out or change because of the exposure of interest. For example, in a cohort study of carpal tunnel syndrome in supermarket check-out operators, it would be a problem if those who developed symptoms changed jobs or left the company because they had developed symptoms, as this would lead to an underestimate of the incidence of the condition. However, if some subjects left the study because they moved away from the area or changed jobs, and these changes were not related to having developed symptoms or their likelihood of developing symptoms, then such loss of subjects should not introduce any bias. A clear illustration of this occurred in a study of the relationship between occupational physical activity and back pain. The study did find a clear relationship between the two, but also found that people who had a high physical load and subsequently developed back pain were more likely to change to jobs that had a lower physical load, compared with persons who had a high physical load but did not develop back pain, and compared with persons who did not have a high physical load at baseline . This issue was able to be identified because the study used a cohort design, but it would have lead to biased and misleading results if a cross-sectional approach had been used.
The key problem in this area is that it is often difficult to find information about why people left, and hard to be confident what the effect might have been even if these reasons for leaving are known. An understanding of the potential effect of such losses can be gained by assuming that all the dropouts did, or did not have, the condition of interest. For example, in a postal survey of nurses, postal workers and office workers in New Zealand, the 12-month prevalence of musculoskeletal disorders was found to be 88%. However, the response rate was only about 58%. It might be expected that people with a musculoskeletal problem would be more likely to respond to a survey about musculoskeletal problems than people who do not have such problems, which would mean that the 88% was an overestimate of the true prevalence. To investigate the possible extent of this effect, the authors showed that even if all of the non-responders did not have a musculoskeletal problem, the prevalence would still have been 51% .
Study methods that minimise the number of dropouts obviously minimise the potential for bias arising from this source. The best example of this is probably the use of population databases that record information on the relevant disorder of interest. Little work is required by the investigators to obtain the information and it is rare that no information can be found regarding a subject, meaning that there are few dropouts. Unfortunately, most countries do not have population registers of musculoskeletal disorders, or do not allow the necessary linkage of personal data between registers. An example of where this was possible was a Swedish study of osteoarthritis of the knee and hip in construction workers. The outcome was defined as surgically treated osteoarthritis in construction workers because information on this surgery was available from the Swedish Hospital Discharge Register. Details of the study subjects were linked to the Register, allowing identification of subjects whose primary presenting problem was osteoarthritis and whose primary reason for admission was a first hip or knee replacement. Of the subjects who enrolled in the study, only 0.15% were excluded because they could not be found in any of the relevant official Swedish registers, and the hospital register had virtually complete national coverage .
A specific selection issue can arise in case-control studies when the cases and controls do not come from the same study base, or do not represent the population of cases and controls within the study base. In occupational musculoskeletal studies, this might occur when cases are identified from a hospital or clinic, as these sites usually do not contain all the cases, and the cases that are identified from these sites commonly are not representative of all the relevant cases. This problem is compounded if the controls are all obtained via a hospital or clinic rather than from the general community from which the cases come, and if a considerable proportion of the eligible controls do not participate in the study. A recent study of the relationship of occupation to the occurrence of supraspinatus tendon tears illustrates some of these issues. Cases were persons whose shoulder pain caused them to have a magnetic resonance imaging (MRI) scan at any of 14 radiology practices in two regions in Germany. Controls were a random sample of the population from the two regions. Forty-eight per cent of eligible cases and 54% of eligible controls agreed to participate. Were the participating cases representative of all cases? As only 48% of the eligible cases participated, and there may have been other radiology centres in the region, and not all people with supraspinatus tears develop pain, it is likely they were not. People who believed their shoulder injury was related to their work may have been more likely to agree to become involved in the study than those who did not have that suspicion. The controls were appropriately drawn from the general population from which it was presumed the cases arose, but there was little information on the controls who did not participate. Therefore, it is difficult to know whether they were representative of all controls. The study found a dose–response relation between length of time working with hands raised above the shoulder and the occurrence of supraspinatus tear causing pain. However, the information on case and control selection and participation suggests considerable scope for selection bias in the study, and illustrates the difficulties in conducting this sort of research in the real world .
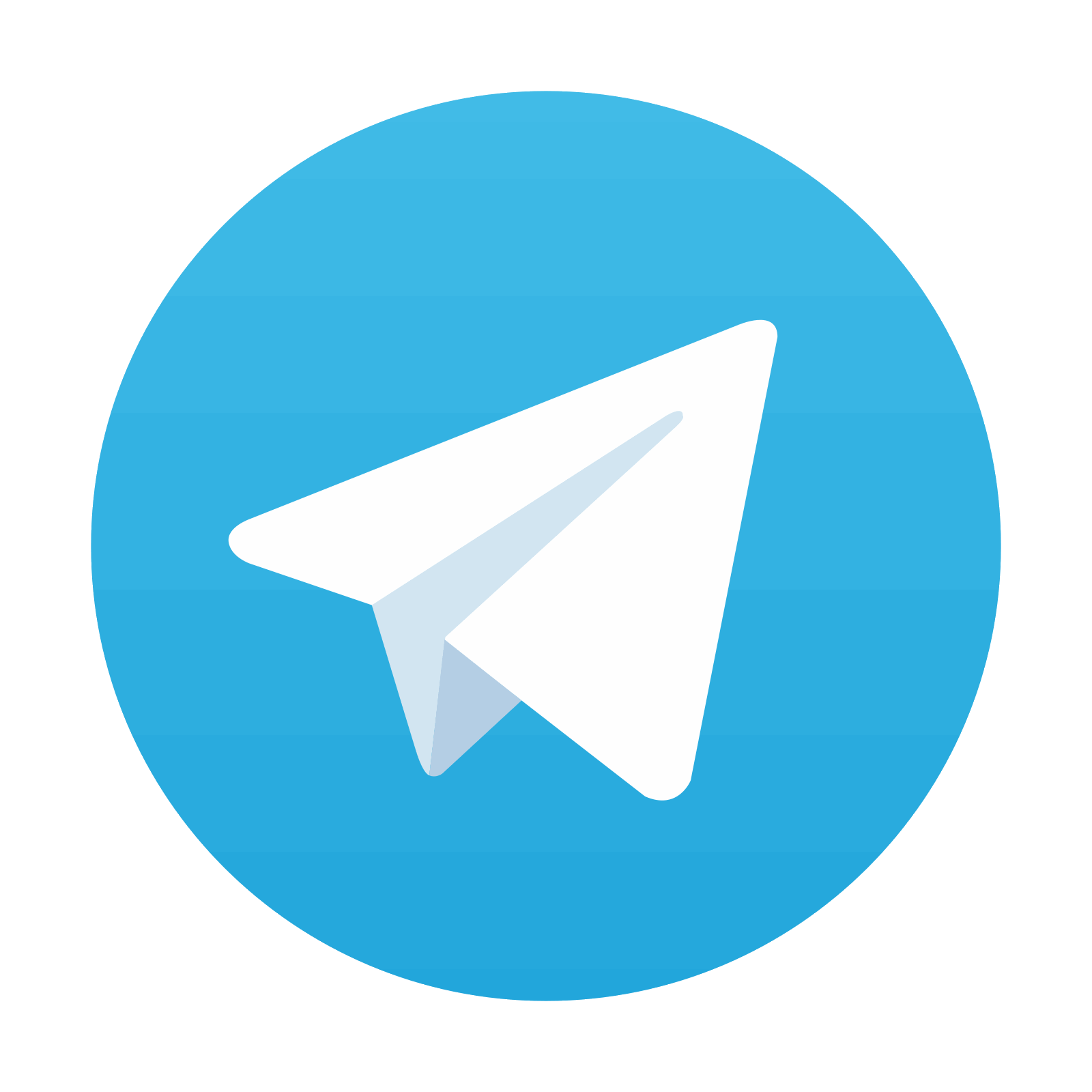
Stay updated, free articles. Join our Telegram channel

Full access? Get Clinical Tree
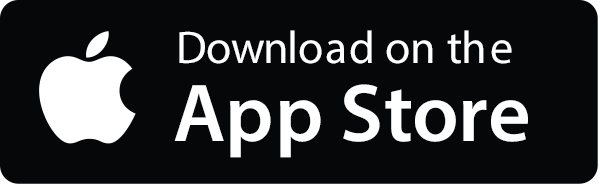
