Introduction
This study aimed to clinically evaluate the accuracy of Dental Monitoring’s (DM) artificial intelligence (AI) image analysis and oral hygiene notification algorithm in identifying oral hygiene and mucogingival conditions.
Methods
Twenty-four patients seeking orthodontic therapy were monitored by DM oral hygiene protocol during their orthodontic treatment. During the bonding appointment and at each of 10 subsequent adjustment visits, a total of 232 clinical oral examinations were performed to assess the presence of the 3 oral hygiene parameters that DM monitors. In each clinical timepoint, the subjects took an oral DM scan and received a notification regarding their current oral status at that moment in time. Sensitivity, specificity, positive predictive value, and negative predictive value were calculated to evaluate AI and clinical assessment of plaque, gingivitis, and recession.
Results
A total of 232 clinical time points have been evaluated clinically and by the DM AI algorithm. For DM’s AI detection of plaque and calculus, gingivitis, and recession, the sensitivity was 0.53, 0.35, and 0.22; the specificity was 0.94, 0.96, and 0.99; and the accuracy was 0.60, 0.49, and 0.72, respectively.
Conclusions
DM’s oral hygiene notification algorithm has low sensitivity, high specificity, and moderate accuracy. This indicates a tendency of DM to underreport the presence of plaque, gingivitis, and recession.
Highlights
- •
Dental Monitoring’s (DM) oral hygiene notification algorithm has low sensitivity.
- •
The DM algorithm also exhibits high specificity and moderate accuracy.
- •
There is a tendency of DM to underreport the presence of plaque, gingivitis, and recession.
Compliance with oral hygiene instructions has been a war of attrition between orthodontists and their patients for decades. Recently, with the widespread presence of smartphones, oral health applications specifically designed for hygiene motivation during orthodontic treatment have become a common tool for remotely monitoring patients. Remote treatment monitoring, or telemonitoring, in orthodontics is a 2-way street requiring active participation from both orthodontists and patients and is becoming increasingly used in our digital world. Applications of telemonitoring include remote treatment planning and consultations, retention phase observation, and emergency mitigation. , An even more cutting-edge aspect of remote monitoring is called Artificial Intelligence Driven Remote Monitoring (AIDRM) because it involves the use of artificial intelligence (AI) image analysis.
Deep machine learning is the current paradigm of AI-based image analysis and is uniquely suited for image analysis because of the algorithm’s ability to learn from statistical and probabilistic examples rather than a set of rules established by humans. A specific type of machine learning called convolutional neural networks (CNNs) is now the predominant AI-based technique for analyzing medical images. CNN’s incorporation of spatial information and their invariance to translation make them uniquely suited to AI image analysis. CNNs have been successfully trained to identify plaque and gingivitis in digital images taken of human subjects’ dentition. ,
Dental Monitoring (DM) is an innovative new AIDRM technology that allows patients to take photographs or videos of their mouth at home that are then evaluated by a proprietary AI image analysis algorithm. Real-time alerts can then be sent to the patient and doctor regarding the specific conditions the AI detects. AIDRM involves collecting large amounts of data from the patients’ photographs from which the AI software is trained to identify specific characteristics and uses deep machine learning to improve the ability of its neural network to detect these attributes.
DM consists of 3 interconnected platforms: a smartphone application for patients, a patented tooth movement and oral health tracking AI algorithm, and a web-based Doctor Dashboard for the orthodontist to view treatment progress. Although analyzing a patient’s regularly scheduled oral scans, the AI algorithm can evaluate tooth movement, monitor oral hygiene, and detect appliance breakage. The DM software then sends specific notifications to the patient and the doctor to take the appropriate next steps on the basis of the conditions detected by the AI algorithm. When evaluating oral hygiene, the DM image analysis algorithm uses a specific set of metrics to identify the presence or absence of 3 important oral hygiene and mucogingival parameters: dental plaque and calculus (P&C), gingivitis, and recession. If the AI algorithm detects 1 of these conditions, it sends an automated notification to the patient and orthodontist regarding the current status of the patient’s oral health.
Several studies have evaluated the accuracy of DM’s tooth-tracking algorithm, its effects on orthodontic care, and patient acceptance of the technology. Overall, the tooth-tracking algorithm has been shown to be accurate enough for clinical acceptability. , Additional research demonstrated that DM can decrease the number of appointments for patients using Invisalign by approximately 35% and that most patients have a positive perception of remote monitoring with DM. , A very recent study investigating the effects of DM on the oral hygiene of orthodontic patients found significantly lower plaque and gingival index values after 6 months of treatment.
DM is poised to become a very powerful tool, allowing orthodontists to closely monitor oral hygiene and communicate with their patients regarding their oral health during orthodontic treatment, which is in addition to the possibility of in-office visit optimization by DM. However, to the best of our knowledge, the AI algorithm for DM’s oral hygiene protocols has not yet been clinically assessed. Therefore, this study aimed to be the first to evaluate the accuracy of DM’s AI image analysis system compared with the gold standard clinical examination regarding the detection of P&C, gingivitis, and recession.
Material and methods
An in-vivo prospective clinical study was conducted at the University of Illinois Chicago’s orthodontic clinic. The study was approved by this academic institution’s Office of Human Subjects Protection (Institutional Review Board 2019-0326). Systemically healthy patients aged >14 years seeking orthodontic treatment with buccally bonded metal brackets were recruited from the patient population at the University of Illinois at Chicago. Patients were excluded from the DM group if they did not have reliable internet or access to a smartphone compatible with the DM application or had a physical limitation that impaired their ability to take oral DM scans. For a significance level, a = 0.05, power more than b = 0.80, and effect size = 1, and considering dropping out, 25 patients were recruited between September 2019 and November 2020. One patient was lost to follow-up during the intervening months, so 24 patients were included in the study group. Considering every patient will be followed up for 10 appointments and 10 independent time points will be obtained from every patient, we were expected to evaluate >200 clinical time points in this study.
A direct bonding technique was used with a standard 38% phosphoric acid etchant. All patients were instructed to have professional prophylaxis before initiating orthodontic treatment and presented at the bonding appointment with a clearance letter from their general dentist indicating that their teeth and gingiva were healthy enough to undergo comprehensive orthodontic treatment. Standard oral hygiene instructions were provided to all participants after the bonding appointment. An oral hygiene kit containing a toothbrush, toothpaste, floss threaders, floss, and interdental aid was provided to each patient, who was instructed to brush at least twice a day and to floss once a day.
The study group (n = 24) was followed for 10-13 months, the equivalent of 10 appointments. At the initial appointment, before bonding brackets, a full mouth clinical examination was performed to determine the presence or absence of P&C, gingivitis, or recession at any location in the mouth. The study participants were instructed on how to operate the DM-provided cheek retractors, ScanBox (Dental Monitoring, Paris, France), and smartphone application and then asked to take a baseline DM scan of their mouths with the assistance of the principal investigator (PI) to ensure proper operation of the device ( Figs 1 and 2 ). The participants then received an automated, individualized oral hygiene notification via their smartphone indicating whether the AI algorithm detected P&C, gingivitis, or recession anywhere in the oral cavity ( Fig 3 ). The web-based Doctor Dashboard receives the same notification as well. At each subsequent adjustment appointment, patients first had a full mouth clinical assessment to evaluate the presence of P&C, gingivitis, or recession at any location in the mouth. Then, immediately afterward, the patient took a DM 2-dimensional photographic scan of their mouths and received a notification on the basis of DM’s proprietary image analysis algorithm of the presence or absence of P&C, gingivitis, or recession. During the clinical examination, the PI assessed the patient’s oral hygiene condition using the same metrics as the AI image analysis software, which is based on the detection of the presence or absence of oral hygiene conditions ( Fig 3 ). A total of 232 clinical time points with their corresponding clinical examination and DM scan notification were evaluated in this study.



For all reliability calculations, 10 patients were chosen at random, and all measurements were reevaluated by the PI and by a separate examiner at 3 predetermined time points. For this clinical assessment, we reorganized DM’s hygiene and gingival indexes into a categorical binomial scale, with the PI recording a score of 0 to indicate the absence of P&C, gingivitis, or recession and a score of 1 to indicate the presence of the oral hygiene and mucogingival parameter. As the gold standard for assessing oral hygiene and mucogingival conditions is the clinical examination, the accuracy, sensitivity, specificity, positive predictive value (PPV), and negative predictive value (NPV) of DM’s AI-based image analysis software were estimated for each of the oral hygiene and mucogingival parameters.
Data distributions were investigated using the Shapiro-Wilk Normality test. Cohen’s kappa coefficient was calculated to evaluate intrarater and interrater reliability. Accuracy, sensitivity, specificity, PPV, and NPV and their corresponding 95% confidence intervals were calculated. Statistical significance was set at 0.05. The data were analyzed using SPSS software (version 28.0, IBM, Armonk, NY).
Results
Twenty-four subjects were included in the study group (8 males, 16 females; mean age, 24.8 ± 12.2 years). A total of 232 clinical time points have been evaluated clinically and by the DM AI algorithm. The Shapiro-Wilk test of normality showed that most of the variables were normally distributed. Intraclass correlation coefficient and Cohen’s kappa coefficient showed high intrarater (κ = 0.792) and interrater reliabilities (κ = 0.791).
Table I includes the crosstabulation for the clinical assessment and DM’s AI assessment of the patient regarding the presence or absence of P&C, gingivitis, and recession.
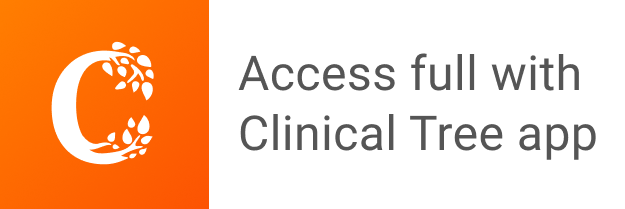