Abstract
Many examinees approach medical statistics with a lot of apprehension. This is justified in most circumstances as we do not regularly practise statistics, nor do we study it on a regular basis. Examiners are not different and appreciate this very well. You should remember that when you are asked questions related to medical statistics you are not expected to demonstrate the knowledge of a statistician. The examiners simply wish to satisfy themselves that as an inquisitive orthopaedic surgeon you understand the basic statistical concepts well enough to be able to scrutinize the published orthopaedic evidence. It is very unlikely that you will be asked esoteric questions (unless you do really well). Statistics of direct relevance (for example NJR survival analysis) are very popular with examiners and are frequently asked. Of the six basic science viva questions most of you would probably be asked at least one question related to Medical Statistics.
Introduction
Many examinees approach medical statistics with a lot of apprehension. This is justified in most circumstances as we do not regularly practise statistics, nor do we study it on a regular basis. Examiners are not different and appreciate this very well. You should remember that when you are asked questions related to medical statistics you are not expected to demonstrate the knowledge of a statistician. The examiners simply wish to satisfy themselves that as an inquisitive orthopaedic surgeon you understand the basic statistical concepts well enough to be able to scrutinize the published orthopaedic evidence. It is very unlikely that you will be asked esoteric questions (unless you do really well). Statistics of direct relevance (for example NJR survival analysis) are very popular with examiners and are frequently asked. Of the six basic science viva questions most of you would probably be asked at least one question related to Medical Statistics.
What kind of statistics question you get asked in the viva would depend on the stage of your viva. If your Basic Science viva commences with a statistics question this would be a ‘settling’ question designed to get you at ease. Remember when you answer your question to remain to the point, but whenever you can feel free to use buzzwords designed to show your familiarity and also to tempt the examiner onto your comfort zone.
Below are examples through which we aim to demonstrate what kind of questions you are likely to be asked and how to address them. The answers are deliberately but thoughtfully expanded to better understand the subject around that particular question and to help address potential follow-up questions.
Structured oral examination question 1
EXAMINER: What do these lines represent (Figure 30.1)?
Figure 30.1 Bell-shaped curve normal distribution.
CANDIDATE: These are all bell-shaped curves of a ‘Normal’ distribution. The x-axis represents a variable (let it be weight, height, Hb level, Na level, hip score, knee score, etc.) and the y-axis represents the frequency of that variable.
EXAMINER: Why do you say this is ‘Normal’?
CANDIDATE: A normal distribution of data is one in which the majority of data are relatively similar, occurring within a small range of values such as height, weight, Hb level, K, Na, etc. Plotting normally distributed data results in a bell-shaped graph as shown here (Figure 30.1).
EXAMINER: What is the significance of this bell-shaped curve?
CANDIDATE: All normal distributions are symmetric and have bell-shaped curves with a single peak. They share important features that make statistical calculation and testing easy and reproducible. Two constant features of normally distributed curve are the mean, where the peak of the density occurs, and the standard deviation, which indicates the spread or girth of the bell curve. Although different variables yield different normal distributions, they all satisfy the 68–95–99.7% rule. Sixty-eight of the observations fall within 1 SD of the mean, 95% of the observations fall within 2 SD of the mean and 99.7% of the observations fall within 3 SD of the mean. Moreover, in normally distributed data, the mean, median and mode are all the same value and coincide with the peak of the curve (Figure 30.2).
Figure 30.2 Normal distribution curve.
EXAMINER: Why is it important to know whether your data are normally distributed or not?
CANDIDATE: So that we can choose the best way to present, analyze and test our findings. For example, I would use mean and SD to present my normally distributed data in contrast to mode, median and range in non-normally distributed data. I would use parametric tests (such as t-test, analysis of variance (ANOVA)) in comparing my findings if data were normally distributed, but I would use non-parametric tests (such as Wilcoxon rank test, Mann–Whitney U tests) if I was dealing with non-normally distributed data.
Authors note 1: Describing data
Data are the building stones for any study and it is expected that all candidates are confident in understanding and describing data. In our experience most candidates are; however, there is some confusion about the pros and cons of various methods of presenting data. In this section, we summarize some important aspects.
Data in general are divided into:
1. Categorical data: the objects being studied are grouped into categories based on some qualitative trait; hence, they are also called qualitative data. This is furthered classified into:
a. Nominal: categories without order, e.g. smoking status, living status, marital status, etc.
b. Ordinal: categories with order, e.g. social status, ficat grading, and pain score (mild, moderate, severe), etc.
Either type can be binary (i.e. two categories only e.g. live or dead; married or unmarried; smoker or non-smoker) or not binary.
2. Measurement data: the objects being studied are measured based on some quantitative trait. Hence, they are also called quantitative data. This is further classified into:
a. Discrete: only certain values are possible (there are gaps between the possible values), e.g. number of admissions to an orthopaedic ward, number of spinal metastases, number of patients transfused.
b. Continuous: theoretically, any value within an interval is possible with a fine enough measuring device, e.g. age, height, weight, blood loss.
The type(s) of data collected in a study determine the type of presentation and statistical analysis used. Categorical data are commonly summarized using percentages or proportions and tested using Chi-square or Fisher exact tests. Summarizing measurement data depends on whether the data are normally distributed or not. If they are normally distributed they can be summarized using means and standard deviation and tested by parametric tests. If data are not normally distributed, they can be summarized using mode, median, range and tested by non-parametric tests (Table 30.1). It is not expected from candidates to know the details of these tests and when to use them. It is acceptable to use a statistician’s help to design a study. However, it boosts your answers if you have basic knowledge about the common ones (highlighted in the table).
There are several ways to check data for normality. Plotting normally distributed data produces a symmetrical bell-shaped curve. If the curve is not symmetrical, the data are probably not normally distributed. Alternatively, data can be formally tested using the Kolmogorov–Smirnov test or Shapiro–Wilk’s test for normality. The latter tests are too sensitive to sample size and may show data as not normally distributed at the time the violation for normality is not substantive; therefore, graphical methods are generally preferable.
Data can also be presented as risk ratio (RR) or relative risk. These are just ways of expressing chance in numbers. For example: 24 people skiing down a slope, 6 fall:
The risk = number of events of interest (falls) / total number of observations = 6/24 = 0.25
The odds = number of events of interest (falls) / number without the event (no falls) = 6/18 = 0.33
Relative risk or risk ratio (they mean the same thing and are both abbreviated as RR) is simply the risk of the event in one group divided by the risk of the event in the other group. RR value of 1 means no difference between the two groups.
The risk difference (RD) is the risk difference between two groups. Sometimes this is called the absolute risk reduction and it is equal to risk on treatment – risk on control. RD value of 0 means no difference between the two groups.
The number needed to treat (NNT) is how many people would need to be treated for one extra patient to be helped and it is the inverse of RD.
The odds ratio (OR) is simply the odds of the event occurring in one group divided by the odds of the event occurring in the other group. OR value of 1 means no difference between the two groups.
There are pros and cons of using the above ratios. Odds ratios are hard to understand and are often misinterpreted. Risk difference (and derivatives like NNT) is more immediately useful than relative risk and odds ratio. The odds and risk ratio are equally variable whereas the risk difference varies more widely and less consistently. They do not change behaviour when the event of interest is reversed and work well in small samples and for rare events.
Table 30.1 Statistical tests.
Testing | Normal distribution | Non-normal distribution | |
---|---|---|---|
Ordinal, measurement data | Nominal data | ||
Data description – central tendency (spread) | Mean (SD) | Median (range) | Proportion |
Intervention in two different groups (such as placebo vs. treatment) | Unpaired t-test | Mann–Whitney U test | Chi-square (Fisher’s test for sample samples of < 5) |
Intervention in the same or matched group (such as Oxford hip or knee scores before and after joint replacement) | Paired t-test | Wilcoxon signed rank test | McNemars’s test |
More than two interventions in different groups (such as pain scores before and after two different non-steroidal anti-inflammatories) | ANOVA | Kruskal–Wallis test | Chi-square test |
More than two interventions in the same or matched groups (such as pain scores before and after two different non-steroidal anti-inflammatories) | Repeated-measures ANOVA | Friedman test | Cochrane Q test |
Quantifying association between two variables (e.g. recurrent falls and number of fractures) | Pearson correlation | Spearman correlation | Contingency coefficients |
Regression analysis † (one variable) | Simple linear or non-linear regression | Non-parametric regression | Simple logistic regression |
Regression analysis (several variables) | Multiple linear or non-linear regression | Multiple logistic regression |
† Regression analysis involves modelling and analyzing several variables, when the focus is on the relationship between a dependent variable (for example, death) and one or more independent variables (for example, age, weight, comorbidities, haemoglobin level, etc.). It helps to understand how the dependent variable changes when any one of the independent variables is varied, while the other independent variables are held fixed.
EXAMINER: OK, what about these two curves (Figure 30.3), are they also bell-shaped?
Figure 30.3 Skewed curves.
CANDIDATE: Not quite, they are skewed curves, they are not symmetric, and the tail is larger on the right for the first image and larger on the left for the second image, respectively called right-skewed and left-skewed data.
EXAMINER: What is the significance of skewed data and where is the mean in these images?
CANDIDATE: They are not symmetrical, therefore the mean is pulled by the outliers, so the mean lies toward the direction of skew (the longer tail) relative to the median. Hence the mean is larger than the median for right-skewed data and smaller than the median in left-skewed data.
EXAMINER: What value would you use to describe this set of data?
CANDIDATE: I would use median and interquartile range.
EXAMINER: OK, what is this chart (shown in Figure 30.4)?
Figure 30.4 Box plot, Oxford hip score.
CANDIDATE: It is a box plot. It compares the Oxford hip score changes between distressed and non-distressed groups of patients from preoperative level to 5 years after surgery. This is another way to present findings graphically. It shows that in both groups of patients, surgery resulted in improvement in Oxford hip score and also that there were more outliers in the non-distressed group compared to the distressed group, postoperative improvement was maintained at 5 years of follow-up and the distressed group appears to have made a comparable if not a slightly larger gain compared to the non-distressed group. This may be a better way to present skewed data graphically.
EXAMINER: What are the different lines?
CANDIDATE: The width of the box shows the interquartile range, the line in the middle is the median value, the whisker shows the lowest and the highest values and the circles are the outliers.
CANDIDATE: A value that is much larger or smaller than the rest of the data.
EXAMINER: Do you know how you can transform non-parametric data to more normal-looking data?
CANDIDATE: No.
Authors’ note 2
There are several ways to present data numerically and graphically. There is no perfect way and each one has pros and cons. Most candidates are familiar with bell-shaped curves, bar and pie charts. A box plot is another important and common way to present data and has been featured in the exam (Figure 30.5). The middle of the box plots represents the median and the sides (or the bottom and top of the box) are always the first and third quartiles. The ends of the whiskers can represent several possible alternative values, such as the minimum and maximum of all of the data, one standard deviation above and below the mean of the data or the 9th percentile and the 91st percentile. Outliers may be plotted as individual points.
Figure 30.5 Box plot.
Figure 30.6 compares the box plot to the bell-shaped curve for better understanding.
Figure 30.6 Box plot versus bell-shaped curve.
Structured oral examination question 2
EXAMINER: Can you tell me how you would design a clinical trial?
Authors’ note 3
Frequently, candidates make the mistake of jumping straight onto a randomized controlled trial, the question is designed to see if you have an overall concept of designing a trial and whether you might have any practical experience.
CANDIDATE: Design of a clinical trial would begin with a clinical question that might arise out of a clinical context. In the first instance I would conduct a literature search utilizing the PICO principle [a buzzword that would impress the examiner, PICO stands for Patients, Intervention, Comparison, Outcome]. I would first search for the highest level of evidence in systematic reviews and meta-analyses. If there are none I would search for primary trials and so on [thus demonstrating that you are familiar with level of evidence].
If my search indicates that there is lack of evidence with regard to my clinical question I would proceed to designing a clinical trial. The design of the trial would depend on my underlying clinical question.
EXAMINER: Tell me more about the level of evidence (Table 30.2).
Table 30.2 Level of evidence.
Level | Intervention | Prognosis | Diagnosis | Economic and decision analyses | |
---|---|---|---|---|---|
1 | a | Systematic review (SR) of randomized controlled trials (RCT) with homogeneous findings | SR (with homogeneity) of inception cohort studies | SR (with homogeneity) of Level 1 diagnostic studies | SR (with homogeneity) of Level 1 economic studies |
b | Individual RCT with narrow confidence interval (CI) | Individual inception cohort study with > 80% follow-up | Validating cohort study with good reference standards | Analysis based on clinically sensible costs of alternatives; systematic review(s) of the evidence; and including multiway sensitivity analyses | |
c | All or none studies | All or none case-series | Absolute SpPins and SnNouts | Absolute better-value or worse-value analyses | |
2 | a | SR (with homogeneity) of cohort studies | SR (with homogeneity) of either retrospective cohort studies or untreated control groups in RCTs | SR (with homogeneity) of Level >2 diagnostic studies | SR (with homogeneity) of Level >2 economic studies |
b | Individual cohort study (including low quality RCT; e.g. < 80% follow-up) | Retrospective cohort study or follow-up of untreated control patients in an RCT | Exploratory cohort study with good reference standards | Analysis based on clinically sensible costs or alternatives; limited review(s) of the evidence, or single studies; and including multiway sensitivity analyses | |
c | ‘Outcomes’ research; ecological studies | Outcomes research | Audit or outcomes research | ||
3 | a | SR (with homogeneity) of case-control studies | SR (with homogeneity) of 3b and better studies | SR (with homogeneity) of 3b and better studies | |
b | Individual case-control study | Non-consecutive study; or without consistently applied reference standards | Analysis based on limited alternatives or costs, poor-quality estimates of data, but including sensitivity analyses incorporating clinically sensible variations | ||
4 | Case series | Case series | Case-control study, poor or non-independent reference standard | Analysis with no sensitivity analysis | |
5 | Expert opinion | Expert opinion | Expert opinion | Expert opinion |
1. A complete assessment of the quality of individual studies requires critical appraisal of all aspects of the study design.
2. A combination of results from two or more prior studies.
3. Studies provided consistent results.
4. Study was started before the first patient enrolled.
5. Patients treated one way (e.g. with cemented hip arthroplasty) compared with patients treated another way (e.g. with cementless hip arthroplasty) at the same institution.
6. Study was started after the first patient enrolled.
7. Patients identified for the study on the basis of their outcome (e.g. failed total hip arthroplasty), called ‘cases’, are compared with those who did not have the outcome (e.g. had a successful total hip arthroplasty), called ‘controls’.
8. Patients treated one way with no comparison group of patients treated another way.
This chart was adapted from material published by the Centre for Evidence-Based Medicine, Oxford, UK. For more information, please see www.cebm.net
CANDIDATE: Each published (or unpublished) study is considered as an evidence. The importance of this evidence is based on the quality of that particular study. The general principle of the hierarchy is that controlled studies are generally better than uncontrolled ones, prospective are generally better than retrospective, and randomized are generally better than non-randomized studies [1]. Over the last three decades, several systems have emerged to assign a hierarchy for studies based on the aforementioned principles. Examples of these systems are the Oxford Centre for Evidence-Based Medicine (OCEBM) [2], the Scottish Intercollegiate Guidelines Network (SIGN) [3] and the Journal of Bone and Joints Surgery Levels of Evidence [1]. I am familiar with the OCEBM, where the highest level of interventional studies is systematic reviews and meta-analysis of randomized controlled studies with a high homogeneity and the lowest is the expert opinion.
Authors’ note 4
Levels of evidence (LOE) are designed to help busy clinicians, researchers, or patients to find the likely best evidence in the shortest possible time. Table 30.1 showed the LOE produced by OCEBM. These levels of hierarchy vary among different systems and over time. They are not absolute. Sometimes ‘lower-level’ evidence from an observational study with a dramatic effect provides stronger evidence than a ‘higher-level’ study such as a systematic review of few studies leading to an inconclusive result. There are several examples in orthopaedic practice when a case series (level IV evidence) changed practice significantly. Charnley hip replacement and Ponseti’s treatment of clubfoot are classical examples [4].
It is essential to appreciate that LOE are not recommendations for or against certain treatments and several factors must be considered when applying best evidence in practice. These include but are not limited to:
i. Is your patient sufficiently similar to the patients in the studies you have examined?
ii. Does the treatment have a clinically relevant benefit that outweighs the harms (e.g. medicine X reduces blood loss by 50 ml but may be clinically irrelevant)?
iii. Is another treatment better (e.g. a systematic review might suggest that surgery is the best treatment for back pain, but if exercise therapy is useful, this might be more acceptable to the patient than risking surgery as a first option) [5]?
EXAMINER: Suppose that you are trying to test a new treatment? Literature search revealed no useful information.
CANDIDATE: Ideally, I would like to design a randomized controlled trial (RCT) to compare my new treatment to the current (or any another treatment). It is the most rigorous way of determining whether a cause–effect relation exists between treatment and outcome. Good RCT design must ensure [6]:
1. Random allocation to treatment groups.
2. Patients and trialists remain unaware of which treatment was given until the study is completed: this is called ‘concealment’.
3. The number of participants is optimum to show a clinically relevant difference.
4. The two groups of treatment are treated identically except for the experimental treatment.
There are two types of RCT; explanatory and pragmatic. It can be debated which one is better in this scenario.
EXAMINER: What is the difference between the two types?
CANDIDATE: Explanatory trials generally measure efficacy – the outcomes of treatments under ideal conditions. For example, if I was testing a new type of cement to help reduce revision rates, I would design my study in such a way that I remove or minimize the effect of any factors that could influence the revision rate. For example, all operations will be done by surgeon X, in centre X using implant X, at room temperature X and using a standardized cementing technique, etc. This design would be likely to show me the real effect of the new cement on revision rate. However, in reality the above design is neither practical nor desirable because we would want to see the tested intervention (the new cement here) work similarly in every centre, for every surgeon and with every implant. Hence the pragmatic trials are more popular. They measure effectiveness – the benefit the treatment produces in routine practice [7].
EXAMINER: How do you randomize?
CANDIDATE: There are many ways of randomization. Ideally this should be performed by a centralized computerized system.
CANDIDATE: The advantage of randomization is to avoid bias by equally distributing the known and unknown patient variables (that potentially affect the outcomes) so that the difference in treatment effect is most likely to be due to the difference in the intervention.
EXAMINER: What do you mean by bias?
CANDIDATE: Bias is a tendency to deviate from the true value due to an error in the study design and thus over- or underestimate the true value of the treatment effect. Examples of bias include selection bias, allocation bias, assessment bias, performance bias, etc.
CANDIDATE: I would perform a statistical test and if the P value is < 0.05 this would suggest that there is less than 5% chance that the observed difference in treatment effect is purely due to chance, therefore I would consider the result to be statistically significant.
EXAMINER: Would you change your practice based on the results of a P value? Can you imagine a situation where the P value is > 0.05 but you might want to reconsider the intervention?
CANDIDATE: Yes, where there is a possibility of a type II error.
EXAMINER: What is a type II error?
CANDIDATE: Where the sample size of the trial might be inadequate and therefore even if there was a significant difference between the intervention and the control group this would not be evident in the statistical test.
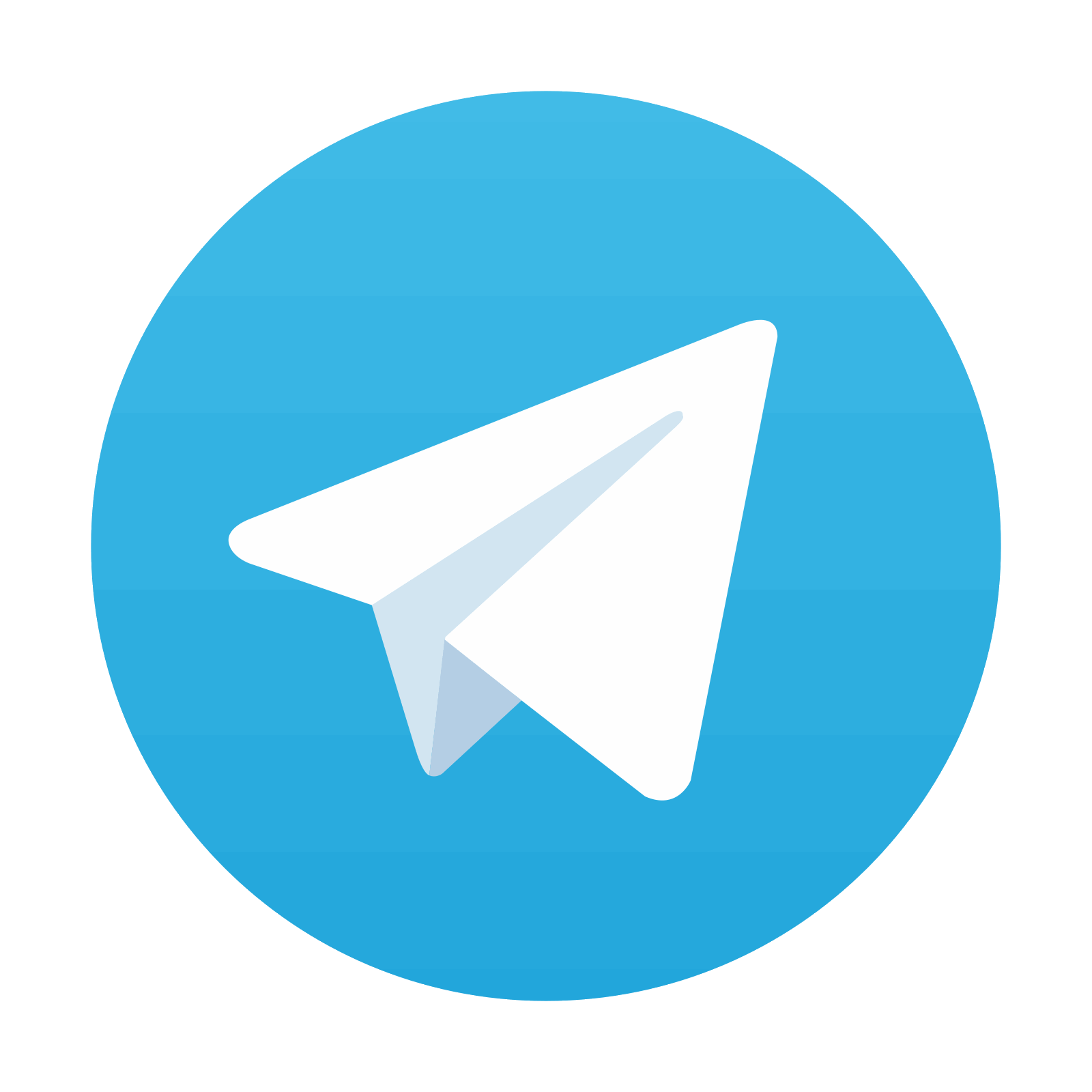
Stay updated, free articles. Join our Telegram channel

Full access? Get Clinical Tree
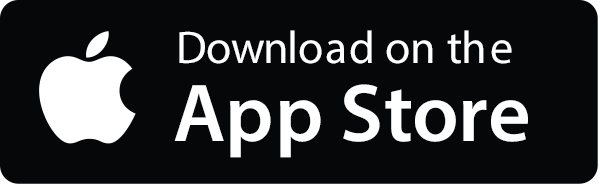

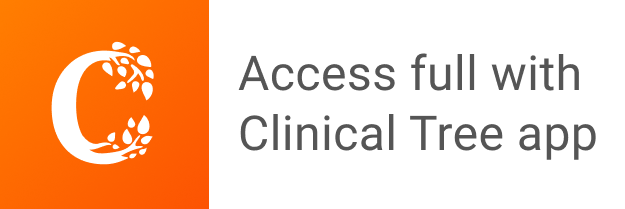