Abstract
In recent years, there has been increasing interest in studying causal mechanisms in the development and treatment of back pain. The aim of this article is to provide an overview of our current understanding of causal mechanisms in the field. In the first section, we introduce key concepts and terminology. In the second section, we provide a brief synopsis of systematic reviews of mechanism studies relevant to the clinical course and treatment of back pain. In the third section, we reflect on the findings of our review to explain how understanding causal mechanisms can inform clinical practice and the implementation of best practice. In the final sections, we introduce contemporary methodological advances, highlight the key assumptions of these methods, and discuss future directions to advance the quality of mechanism-related studies in the back pain field.
Introduction
Experiencing back pain without knowing its cause can be highly distressing for the patient. Disappointingly, research so far has failed to provide robust causal explanations to mitigate these uncertainties. Poor understanding of causal factors has stifled the development of effective treatments. This is because treatment targets have only been informed by assumptions or implicit theories rather than empirical evidence for well-defined causal mechanisms. For the past 16 years, landmark papers in the field have repeatedly highlighted the paucity of evidence on the causes of low back pain . This is despite back pain researchers ranking research into the mechanisms and causes of low back pain as one of the highest research priorities to advance the field .
By understanding causal mechanisms in the clinical course of back pain, we can identify important factors that can be targeted in treatment. Although pain and disability are important outcomes for the patient, they are not the typical targets of treatments. Treatments are devised to target hypothesised intermediary factors (or mediators) that are causally linked to patient-relevant outcomes such as pain and disability. In other words, treatments generally exert their effects on pain and disability through indirect pathways, which we refer to as ‘causal mechanisms’. In a recent mechanism evaluation study, Fordham et al. showed that cognitive behavioural therapy (exposure) reduced disability (outcome) through changes in self-efficacy, fear avoidance and physical functioning (mediators) but not through improvements in mental functioning . This information gained from a mechanism evaluation is particularly useful in understanding how treatments work or why they fail.
By understanding causal mechanisms, treatments can be adapted and refined to improve their clinical efficacy and facilitate implementation. Recently, there has been increasing interest in generating evidence for causal mechanisms in the back pain field . The aim of this article is to provide an overview of current understanding of causal mechanisms in the clinical development and treatment of back pain. This article is divided into five sections (1. Key concepts and terminology; 2. Overview of mechanism evaluations in the field; 3. Application to clinical practice and implementation; 4. Methodological considerations; 5. Future directions).
Key concepts and terminology
Many empirical studies in health research aim to establish whether an exposure causes an outcome . For example, a randomised trial might aim to establish whether an exercise programme (exposure) causes less disability (outcome). However, merely establishing a single causal association is often insufficient to explain how or why an exposure causes an outcome . This approach to understanding causation is often criticised as the ‘black-box’ approach because the underlying causal mechanisms are unknown . In the above example, there is no explanation for how an exercise programme causes less disability. To overcome this limitation, we can use certain design characteristics and analyses in clinical studies to evaluate causal mechanisms and help explain how or why a causal association occurs .
A causal mechanism is the pathway that links an exposure (which could be a treatment or observed variation in clinical exposure) to an outcome . To identify a causal mechanism, a theoretically plausible intermediate variable (often called a mediator) that lies on the causal path between the exposure and outcome needs to be identified. Then, mediating effects (or indirect effects) caused by that selected mediator can be estimated, often by conducting a form of mediation analysis .
Mediation analysis can quantify causal mechanisms by decomposing the causal effect of the exposure on the outcome (total effect) into indirect and direct effects. The indirect effect is the causal effect of the exposure on the outcome that is channelled through the selected mediator , and the direct effect encompasses all other unspecified mechanisms. Quantitatively, the total effect is the sum of the indirect and direct effects, hence the term ‘effect decomposition’. By decomposing the total effect into indirect and direct effects, we can gain insight into why an exposure causes an outcome. Importantly, mediation analyses are causal analyses. Thus, it is always necessary to consider the role of confounders in these analyses.
To illustrate the above concepts, the following section outlines examples of the application of mediation analyses to cohort studies and randomised trials. A graphical representation of the above concepts is also shown in Fig. 1 with accompanying definitions in Table 1 .
Terms | Definitions |
---|---|
Exposure | A variable that is thought to exert an effect on the outcome through selected mediators and/or through a direct causal pathway. In randomised trials, this is often the random allocation of participants to selected groups (e.g. intervention vs control). In cohort studies, this is often an observed variable that is not experimentally manipulated (e.g. pain intensity, smoking). |
Mediator | A selected intermediate variable that is thought to channel the causal effect of the exposure onto the outcome. In other words, a variable that is causally affected by the exposure, which then causally affects the outcome. |
Outcome | A key variable that is hypothesised to be influenced by the exposure through a selected mediator or any other unspecified mechanism(s). This is often the primary outcome of a randomised trial or cohort study (e.g. pain-related disability). |
Confounder | A covariate that could induce a spurious (non-causal), association between exposure and mediator, exposure and outcome, and mediator and outcome. In cohort studies, independent or mutual sets of confounders may exist for all three effects (indirect, direct and total). In randomised trials, a set of confounders may only exist for the mediator–outcome effect. |
Hypothetical examples
Mediation analyses are often applied to both cohort studies and randomised trials, each addressing different research questions. When mediation analyses are applied to cohort studies, the aim is to identify causal pathways involved in the onset or sequela of a health condition. For example, the transition from acute back pain (exposure) to ongoing disability (outcome) may be mediated by reduced levels of physical activity (mediator). Mechanism evaluations in these instances can support a hypothesised causal mechanism, or perhaps more importantly, identify where the causal mechanism breaks down. For instance, acute pain may reduce physical activity, but reduction in physical activity may not be the cause of ongoing disability. Quantifying these mechanisms can provide insight into the factors that drive poor (or better) outcome, which can then inform treatment targets (explained in subsequent sections).
When mediation analyses are applied to randomised trials, the aim is to understand why an intervention is, or is not, effective . For example, an exercise intervention (exposure) may partly exert its effect on disability (outcome) by reducing depressive symptoms (mediator) . Mediation analyses are also particularly useful for explaining why treatments fail. In randomised trials with null findings, a mediation analysis can identify whether it was the intervention that failed to influence the mediator or whether that mediator failed to influence the outcome, or both. In the above example, the exercise intervention may successfully reduce depressive symptoms, but shift in depressive symptoms (caused by the intervention) may not cause a reduction in disability. This could be because of an insufficient magnitude of change in depressive symptoms or simply because depressive symptoms do not cause changes in disability. Understanding where the causal path breaks down by using this approach can be useful to guide the adaptation of treatments (explained in subsequent sections).
Key difference between mediation analyses of randomised trials and cohort studies
From a methodological perspective, the key difference between mechanism evaluations of randomised trials and those of cohort studies is how the exposure variable is defined. In randomised trials, the exposure is the random allocation of participants to groups (e.g. exercise vs usual care), whereas in cohort studies, the exposure is usually something that cannot be experimentally manipulated (e.g. smoking vs no smoking). This difference has important repercussions for the internal validity of the mechanism evaluation . A key assumption for accurately identifying causal mechanisms is the ‘no-confounding’ assumption . To meet this assumption, all pathways in the model ( Fig. 1 ) should be free of confounding. In a cohort study, despite adjusting for known confounders in all three paths, the assumption may still be unmet because of unknown or unmeasured confounders . In contrast, in a randomised trial (assuming perfect adherence, complete follow-up and adequate concealment), the treatment–outcome effect and treatment–mediator effects are free from confounding because randomisation eliminates the effects of known and unknown confounders. However, even in randomised trials, the path from the mediator to the outcome could still be confounded, even if known confounders are adjusted for. Recent analytical advances have developed sensitivity analyses that can provide insight into how unmeasured confounding influences mechanistic effects .
Key concepts and terminology
Many empirical studies in health research aim to establish whether an exposure causes an outcome . For example, a randomised trial might aim to establish whether an exercise programme (exposure) causes less disability (outcome). However, merely establishing a single causal association is often insufficient to explain how or why an exposure causes an outcome . This approach to understanding causation is often criticised as the ‘black-box’ approach because the underlying causal mechanisms are unknown . In the above example, there is no explanation for how an exercise programme causes less disability. To overcome this limitation, we can use certain design characteristics and analyses in clinical studies to evaluate causal mechanisms and help explain how or why a causal association occurs .
A causal mechanism is the pathway that links an exposure (which could be a treatment or observed variation in clinical exposure) to an outcome . To identify a causal mechanism, a theoretically plausible intermediate variable (often called a mediator) that lies on the causal path between the exposure and outcome needs to be identified. Then, mediating effects (or indirect effects) caused by that selected mediator can be estimated, often by conducting a form of mediation analysis .
Mediation analysis can quantify causal mechanisms by decomposing the causal effect of the exposure on the outcome (total effect) into indirect and direct effects. The indirect effect is the causal effect of the exposure on the outcome that is channelled through the selected mediator , and the direct effect encompasses all other unspecified mechanisms. Quantitatively, the total effect is the sum of the indirect and direct effects, hence the term ‘effect decomposition’. By decomposing the total effect into indirect and direct effects, we can gain insight into why an exposure causes an outcome. Importantly, mediation analyses are causal analyses. Thus, it is always necessary to consider the role of confounders in these analyses.
To illustrate the above concepts, the following section outlines examples of the application of mediation analyses to cohort studies and randomised trials. A graphical representation of the above concepts is also shown in Fig. 1 with accompanying definitions in Table 1 .
Terms | Definitions |
---|---|
Exposure | A variable that is thought to exert an effect on the outcome through selected mediators and/or through a direct causal pathway. In randomised trials, this is often the random allocation of participants to selected groups (e.g. intervention vs control). In cohort studies, this is often an observed variable that is not experimentally manipulated (e.g. pain intensity, smoking). |
Mediator | A selected intermediate variable that is thought to channel the causal effect of the exposure onto the outcome. In other words, a variable that is causally affected by the exposure, which then causally affects the outcome. |
Outcome | A key variable that is hypothesised to be influenced by the exposure through a selected mediator or any other unspecified mechanism(s). This is often the primary outcome of a randomised trial or cohort study (e.g. pain-related disability). |
Confounder | A covariate that could induce a spurious (non-causal), association between exposure and mediator, exposure and outcome, and mediator and outcome. In cohort studies, independent or mutual sets of confounders may exist for all three effects (indirect, direct and total). In randomised trials, a set of confounders may only exist for the mediator–outcome effect. |
Hypothetical examples
Mediation analyses are often applied to both cohort studies and randomised trials, each addressing different research questions. When mediation analyses are applied to cohort studies, the aim is to identify causal pathways involved in the onset or sequela of a health condition. For example, the transition from acute back pain (exposure) to ongoing disability (outcome) may be mediated by reduced levels of physical activity (mediator). Mechanism evaluations in these instances can support a hypothesised causal mechanism, or perhaps more importantly, identify where the causal mechanism breaks down. For instance, acute pain may reduce physical activity, but reduction in physical activity may not be the cause of ongoing disability. Quantifying these mechanisms can provide insight into the factors that drive poor (or better) outcome, which can then inform treatment targets (explained in subsequent sections).
When mediation analyses are applied to randomised trials, the aim is to understand why an intervention is, or is not, effective . For example, an exercise intervention (exposure) may partly exert its effect on disability (outcome) by reducing depressive symptoms (mediator) . Mediation analyses are also particularly useful for explaining why treatments fail. In randomised trials with null findings, a mediation analysis can identify whether it was the intervention that failed to influence the mediator or whether that mediator failed to influence the outcome, or both. In the above example, the exercise intervention may successfully reduce depressive symptoms, but shift in depressive symptoms (caused by the intervention) may not cause a reduction in disability. This could be because of an insufficient magnitude of change in depressive symptoms or simply because depressive symptoms do not cause changes in disability. Understanding where the causal path breaks down by using this approach can be useful to guide the adaptation of treatments (explained in subsequent sections).
Key difference between mediation analyses of randomised trials and cohort studies
From a methodological perspective, the key difference between mechanism evaluations of randomised trials and those of cohort studies is how the exposure variable is defined. In randomised trials, the exposure is the random allocation of participants to groups (e.g. exercise vs usual care), whereas in cohort studies, the exposure is usually something that cannot be experimentally manipulated (e.g. smoking vs no smoking). This difference has important repercussions for the internal validity of the mechanism evaluation . A key assumption for accurately identifying causal mechanisms is the ‘no-confounding’ assumption . To meet this assumption, all pathways in the model ( Fig. 1 ) should be free of confounding. In a cohort study, despite adjusting for known confounders in all three paths, the assumption may still be unmet because of unknown or unmeasured confounders . In contrast, in a randomised trial (assuming perfect adherence, complete follow-up and adequate concealment), the treatment–outcome effect and treatment–mediator effects are free from confounding because randomisation eliminates the effects of known and unknown confounders. However, even in randomised trials, the path from the mediator to the outcome could still be confounded, even if known confounders are adjusted for. Recent analytical advances have developed sensitivity analyses that can provide insight into how unmeasured confounding influences mechanistic effects .
Overview of mechanism evaluations in the clinical course and treatment of low back pain
Causal mechanisms in the clinical course of low back pain
The clinical course of back pain typically follows a pattern where most patients experience significant improvements in pain and disability in the first 6 weeks from acute onset . Patients who continue to experience pain beyond 6 weeks are likely to suffer from significant levels of disability that adds to the burden of back pain . Mediation analysis can be used to identify the processes that lead to prolonged disability in patients with back pain. For example, mediation analyses of cohort studies have tested whether psychological variables underlie the development of prolonged disability . These studies were systematically reviewed and meta-analysed . From 12 studies that reported a total of 36 mediator models, reduced distress, reduced fear and increased self-efficacy were shown to mediate the pain–disability relationship. Contrary to the prevailing view , decreased catastrophising was not a mediator . Interestingly, the identified psychological mechanisms only explained a moderate proportion of the total effect, approximately between 20% and 33%. This implies that there are unexplained causal mechanisms that are yet to be identified (i.e. remaining direct effects accounted for a significant proportion of the total effect—67–80%). Perhaps these unexplained mechanisms are biological, such as neuro-inflammatory, immunological , and endocrine in nature that upregulate nociception. Establishing the causal pathways that link these constructs will provide more comprehensive explanations for why back pain causes ongoing disability.
Causal mechanisms in treating low back pain
In 2013, Mansell et al. systematically reviewed mediators of psychological interventions that aimed to reduce disability in patients with musculoskeletal conditions. Their review found that increased self-efficacy, reduced pain catastrophising, accurate pain beliefs, increased pain coping and increased psychological flexibility were important mediators. Since the publication of this review, more studies have investigated the causal mechanisms of various interventions for back pain. These studies along with those reviewed by Mansell et al. are summarised in Table 2 .
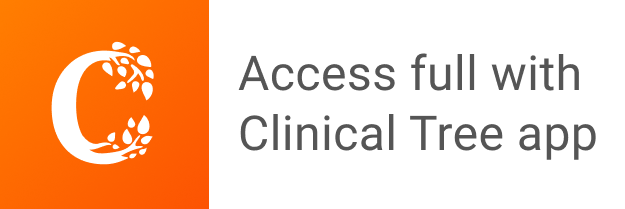