Key Points
- •
Brain-computer interfaces use electrical activity recorded from electrodes on the scalp or surgically implanted in the brain.
- •
Brain-computer interfaces for rehabilitation and assistive devices strive to replace, supplement, or restore lost function.
- •
Significant challenges remain for brain-computer interfaces to achieve their full potential and practicality.
This chapter provides an overview of prior and prospective work related to the control of assistive robotics and prostheses via direct measurements of brain activity. Specifically, this chapter focuses on brain-controlled robotic devices that replace , supplement , or restore lost motor function. The chapter does not include brain-controlled robotic devices that have been developed for rehabilitation or therapeutic applications, which are included in Chapter 6 . The present chapter introduces noninvasive and invasive brain signal–recording modalities, common brain-actuated control signals, and a survey of applications in assistive robotics and prosthetics. The chapter concludes with a discussion of current challenges and future prospects of these technologies.
Brain-Computer/Brain-Machine Interfaces
A brain-computer interface (BCI) is a system that directly translates neuronal signals into actionable inputs for an external device, as shown in Fig. 10.1 . The term “brain-computer interface” is generally synonymous with brain-machine interface, with the terms emerging from the noninvasive and invasive research communities, respectively. This general field of research originated as a means to help severely disabled individuals (e.g., suffering from late-stage amyotrophic lateral sclerosis [ALS] or brain stem stroke) communicate and perform tasks of daily living. Although the field has since evolved to include other nonmedical applications, there is still significant focus on medical applications of BCI.

Because the original impetus for BCIs was to restore, replace, or supplement lost function, much of the early work was focused on controlling motor prostheses, orthotics, and assistive devices. Ideally, the ultimate objective is to completely restore natural motor function that has been lost by the user. However, approaching such naturalistic and transparent control in practice requires precise measurement of large-scale neural activity that can currently only be achieved via invasive measurements of brain activity (e.g., surgical implantation of high-density electrodes into the brain).
Due to the inherent risks of surgical implants, much of the research has been conducted in animal models (primarily nonhuman primates ) and, in special cases, on humans undergoing surgical procedures for intractable epilepsy and glioma removal. Additionally, there have been a limited number of long-term human implants exclusively for BCI research. In contrast, current modalities for noninvasive measurement of brain activity cannot provide sufficient fidelity to achieve natural, refined control of a motor prosthetic. Nevertheless, the ability to control assistive devices with as few as 2 to 3 degrees of freedom (DoFs) (e.g., wrist rotation and hand grasp) has the potential to greatly improve the user’s performance on activities of daily living.
Broadly, there are two classes of brain activity measurements that are relevant for such applications: (1) electrophysiologic and (2) hemodynamic. Hemodynamic activity can be measured with functional magnetic resonance imaging, positron emission tomography, and functional near-infrared imaging. Because of the comparatively slow hemodynamic response on the order of 1 to 2 seconds and the impractical size and cost of the devices for independent daily use (in the case of functional magnetic resonance imaging and positron emission tomography), these modalities are generally not well suited for real-time robotic control applications.
Conversely, electrophysiologic measurements of brain activity provide response times on the order of milliseconds and can be designed to be portable or fully implantable, making them suitable for real-time control and independent daily use. The established modalities for invasively and noninvasively acquiring electrical brain activity for BCI applications are described next.
Invasive Recordings
There are three general categories of implanted electrodes, characterized by the method of implantation, depth of penetration, and size of the recording contacts. The most commonly used types of implanted electrodes are shown in Fig. 10.2A–C .

Microelectrodes
Microelectrodes are penetrating electrodes designed to record from individual neurons, commonly referred to as single units . Most modern microelectrodes are configured as arrays of needlelike probes with electrodes along the shafts of each probe. The electrodes generally have surface areas and spacings on the order of 1 to 10 µm and are fabricated using silicon substrates. Individual microelectrode arrays are typically designed for sampling tens of hundreds, and potentially thousands, of neurons simultaneously.
Ideally, each microelectrode contact will measure action potentials from a single neuron. In practice, only a subset of contacts will yield clean recordings of action potentials from individual neurons. Otherwise, contacts can also measure local field potentials, representing a superposition of electrical activity from surrounding neurons.
The acquisition of action potentials from a population of neurons in the motor cortex can precisely determine movement intentions based on a phenomenon known as population coding . Put simply, individual neurons in the motor cortex have been shown to have firing rates that follow a cosine tuning curve, in which the firing rate of each neuron maximally corresponds to a preferred direction of movement and minimally corresponds to movements in the opposite direction (i.e., 180 degrees), with intermediate directions following a cosine curve (as shown in Fig. 10.3A–B ). By sampling a sufficient number of neurons in the motor cortex, shown to be as few as 10 to 100, continuous motor movements can be accurately reconstructed by taking the vector sum of the predicted movement directions for each neuron in the population as prescribed by the predetermined tuning curves ( Fig. 10.3C ). Because the resulting control signal represents a fairly direct mapping of the measured neuronal function, this approach yields naturalistic control of motor prostheses.

Electrocorticography and Stereotactic Electroencephalography
Electrocorticography (ECoG) uses comparatively larger disk electrodes in rectangular arrays or strips placed on the cortical surface via a craniotomy. The standard clinical electrodes are 5 mm in diameter with 1 cm spacing between contacts, although micro-ECoG arrays also have been used with diameters and spacings on the order of tens of microns. Although ECoG does not penetrate the brain or record single unit activity, it can provide detailed information for decoding sensory, motor, and cognitive processes for a BCI. ECoG recording provide signals that are morphologically similar to local field potentials with spectral bandwidth of roughly 0 to 250 Hz. ECoG is used clinically for localizing and mapping as part of surgical planning for intractable epilepsy and intraoperatively for glioma removal. To minimize the extent of the required craniotomy, ECoG grid placement is typically localized to particular cortical areas associated with the patient’s pathology, typically on a single hemisphere. For clinical purposes, the number of implanted ECoG electrodes typically ranges from 16 to 128, depending on the nature and location of the pathology.
A related technology employed for epileptic seizure localization is stereotactic electroencephalography (sEEG). sEEG electrodes are positioned along a cylindrical shaft (∼1 mm in diameter), which is inserted deeper into the brain through small burr holes in the skull using stereotactic guidance. The surface area, number of electrodes implanted, and nature of the measured signals of sEEG for clinical purposes are roughly comparable to ECoG, although higher-density contacts and microwires can be used. Although, in contrast to ECoG, sEEG electrodes penetrate brain tissue, there is evidence that the procedures are as effective as ECoG and have improved surgical recovery outcomes compared with the craniotomy required for ECoG. Also, unlike the single, localized craniotomy for ECoG, the comparatively minute burr holes for sEEG electrodes allows for a broader but sparse sampling of both superficial and deeper brain structures. This creates a trade-off for investigating and utilizing specific brain regions and networks compared with the generally superior local cortical resolution provided by ECoG.
Although the traditional lower-frequency rhythms can be observed in intracranial recordings, one unique and commonly used feature of ECoG/sEEG signals for BCI control is broadband gamma activity. This activity manifests as roughly uniform fluctuations in the signal spectrum over the approximate range of 70 to 250 Hz, which resembles a broadband noise process and is not practically observable in scalp EEG, as illustrated in Fig. 10.4 . This activity has been shown to be highly correlated with a wide variety of cognitive and behavioral function. It is important to note that although high-frequency oscillations exist, broadband gamma is generally not considered a brain oscillation akin to lower-frequency gamma (∼40–70 Hz) or the traditional lower-frequency EEG bands below 40 Hz (i.e., delta, theta, alpha, beta).

Other Implanted Electrodes
One of the earliest demonstrations of invasive BCI control in humans was using neurotrophic electrodes. Neurotrophic electrodes consist of a 1- to 2-mm hollow glass cone attached to several gold conductive wires. The electrode is filled with trophic factors to encourage the growth of axons and dendrites into the cone. Unique features of neurotrophic electrodes are their ability to isolate and record from single or small groups of neurons and their biocompatibility for long-term implantation. However, compared with modern microelectrode arrays, neurotrophic electrodes have not been designed to conveniently sample larger numbers of neurons and have therefore not been widely adopted for BCI research.
More recently, an endovascular thin-film stent-electrode array was implanted in the superior sagittal sinus adjacent to the primary motor cortex of two participants with ALS. This sensor array, known as a stentrode , consists of 17 circumferential sensors on an 8 mm × 40 mm monolithic, self-expanding nitinol scaffold designed for minimally invasive intracranial delivery using catheter venography. The array is connected to a 50-cm flexible transvascular lead and inserted into an inductively powered wireless telemetry unit. For this feasibility study, the participants were able to successfully perform a typing task using attempted movements in combination with an eye tracker for cursor navigation.
Noninvasive Recordings
The primary modality for noninvasive electrophysiologic recordings of the brain is the scalp electroencephalogram (EEG). EEG is acquired via metallic electrodes placed directly on the scalp. EEG electrode diameters are generally on the order of centimeters, and the number of electrodes used can be anywhere from 2, giving a single bipolar channel, to 256 or even more for detecting subcortical activity and source localization. To provide conductivity through hair, the electrodes are either filled with electrolytic gel (i.e., wet electrodes) or have barbs designed to pass through hair (i.e., dry electrodes), as shown in Fig. 10.2D .
Due to the signal attenuation caused by the skull and scalp layers, noninvasive recordings are lower in amplitude (10–100 µV) and are more prone to movement and muscle artifacts compared with invasive recordings. The use of active electrodes, which contain preamplifier circuitry embedded in the electrode housing, can effectively boost the signal and mitigate certain noise and artifacts in the recordings.
EEG signals for direct BCI control can broadly be categorized as active or reactive , depending on whether the brain activity is primarily modulated by endogenous or exogenous means, respectively.
Active Control Signals
The most commonly used active control signals for robotic control applications are event-related desynchronization/synchronization (ERD/ERS) and sensorimotor rhythms (SMRs). These are more naturalistic control signals since they are generated from the motor cortex during actual and imagined movements.
SMRs are idling rhythms occurring in the alpha band of the motor cortex during rest, which can be observed in an estimated 80% of the population. To distinguish from unrelated alpha-band activity, these are often referred to as mu rhythms. A defining characteristic of these rhythms is that the amplitude is attenuated over the corresponding area of the motor cortex during continued actual or imagined movements. Related modulations can also be observed in the beta and gamma bands, although it is not clear whether these are contributed by distinct neural processes or are simply, in part, a by-product of the unique temporal morphology of mu rhythms. Users can learn to modulate SMR amplitudes continuously to achieve continuous, dimensional BCI control (e.g., imagine right-hand movement to move a cursor to the right). The temporal, spatial, and spectral characteristics and how they can be mapped to dimensional BCI control are illustrated in Fig. 10.5A .

ERD refers to the transient decrease in alpha-band power over the motor cortex associated with the onset of isolated movement or imagery, followed by the transient ERS increase as the motor activity returns to the resting state, as shown in Fig. 10.5B . Thus, this transient activity can be used as a discrete switch, for instance to activate/deactivate a hand grasp orthotic.
Other active control signals exist, such as alpha-band modulation during cognitive processes such as mental math or object rotation. However, although these modulations can be reliably detected in the EEG, they require the user to perform a mental task that is not directly related to the primary robotic control task. This can be unnatural, unintuitive, and distracting and is ultimately not an ideal control signal for a practical assistive device.
Although ERD/ERS and SMR represent the most natural and intuitive scalp EEG activity for dimensional control of robotic or assistive devices, these signals tend to be nonstationary, and significant task training may be required for users to reliably modulate these signals to achieve practical device control.
Reactive Control Signals
Reactive control signals result from predictable changes in brain activity generated from the user’s attention to specific sensory stimuli that are mapped to aspects of the control task. These predictable changes in brain activity are known as stimulus evoked potentials (SEPs). SEPs can either be transient, which are generally time-locked with the sensory stimulus and resolve on the order of a second, or steady state, which continue as long as attention to the stimulus is maintained.
One of the earliest scalp EEG BCIs is the P300 speller. It operates based on the brain’s response to a rare or novel sensory stimulus. This brain response is known as an event-related potential (ERP), which is a specific type of SEP that includes a cognitive component (opposed to a purely sensory reflex). In this case, the cognitive component is the recognition that the stimulus is rare or novel.
In a simple P300 speller paradigm, the user is presented with a matrix of flashing symbols on a computer monitor, for instance, similar to a visual keyboard. The user attends to the desired symbol as each symbol is flashed in a random sequence. The general concept is illustrated in Fig. 10.6 . Because the user does not know when the desired symbol will be flashed, an ERP is generated when the symbol is flashed while the user maintains fixed attention. Multiple ERPs must be accumulated over multiple stimulus sequences to reliably represent the characteristic brain response and determine the desired symbol. This process can be made more efficient via row/column flashing or a variety of other enhancements to the original paradigm. Although the original P300 speller paradigm was based on visual attention, auditory or tactile attention can also be utilized with decreased performance compared with visual paradigms.

Effectively, such paradigms provide discrete command selection, equivalent to a computer keyboard—albeit at a much slower rate (∼4–5 symbols per minute). These commands can be mapped to achieve discrete dimensional or goal-oriented control of a robot, for example.
To achieve continuous dimensional control, steady-state sensory evoked potentials can be used, the most practical being the steady-state visual evoked potential (SSVEP). Unlike transient evoked potentials resulting from a single stimulus event, the steady-state evoked potentials result from a repeating stimulus pattern, such as a blinking light. As such, rather than generating a transient EEG response, predictable, continuous repeating EEG patterns over the occipital cortex are generated with attention to the repeating stimulus patterns. These EEG patterns manifest as distinct spectral peaks in the frequency domain that can be reliably identified and tracked, in contrast to the time-domain techniques used to identify transient evoked potentials.
A typical SSVEP interface consists of a spatial array of individual lights or symbols on a screen, each concurrently repeating with a unique temporal flashing pattern and mapped to a control command, as shown in Fig. 10.6 . When the user focuses attention to a specific light/symbol, the brain activity unique to that symbol is detected and the associated command is executed. In contrast to transient evoked potentials, the command can be maintained with continued attention to the stimulus and can be readily changed by shifting attention to a different stimulus to execute the associated control command in a continuous fashion. This can enable joystick-like dimensional control of a robotic device with latency on the order of 0.5 to 1 seconds. It is also possible to achieve high communication rates with discrete selection via keyboard-like interfaces using SSVEP and related variants. As with transient responses, BCIs that utilize steady-state auditory and tactile (somatosensory) potentials, have also been developed.
Control paradigms that rely on visual evoked potentials have several limitations. Foremost, performance can drastically decrease with inability to control eye movements, which is the case for many patients with locked-in syndrome. Also, these interfaces often create a visual cacophony, can be unnatural and fatiguing, and can be distracting from the primary control task, which can make them inconvenient and impractical for long-term use.
Applications to Assistive Robotics and Prosthetics
Upper-Limb Prosthetics
Foundational work exploring the application of BCI for control of robotic arms was first performed in animal models. In 1999, Chapin et al. showed how rats could position a single-DoF robotic arm in real time using a BCI driven by neuronal population activity in the primary motor cortex and ventrolateral thalamus. Just a year later, Wessberg et al. demonstrated an open-loop BCI for real-time control of 3-DoF robotic arms, which better represent the complex arm movements needed to accomplish a reach-and-grasp task. In their approach, cortical neural ensemble activity, recorded from owl monkeys who received no feedback while completing the reach-and-grasp tasks, was used to predict 3D hand trajectories that controlled robotic movement. Further work succeeded in closing the loop for continuous neuronal control of robotic prostheses by nonhuman primates by introducing visual feedback, which resulted in stabilization of performance and more effective learning of neuromodulation.
Velliste et al. demonstrated embodied control, involving real-time interaction with the physical environment, of a 4-DoF prosthetic arm using cortical signals in a closed-loop system. Modulation of cortical signals represented not only the velocity of the end effector in Cartesian space, but also the aperture velocity of the gripper’s fingers. This BCI enabled monkeys to continuously control the prosthetic device to reach to a food target, grasp the food, and then feed themselves. Physical interaction with the environment using the robotic arm enabled displays of embodiment unseen in previous virtual cursor control experiements via behaviors inessential to task completion. For example, the monkey treated the prosthetic arm as if it was its own by moving the gripper to its mouth to lick it instead of only reaching to grasp the food target.
Initial results for BCI control of prosthetic devices by humans was demonstrated by Hochberg et al. in their report describing the first participant in a trial for BrainGate, a neuromotor prosthetic system consisting of a single cortically implanted, 96-channel sensor and external signal processors. In this work, a patient with tetraplegia was enabled to grasp and move objects using a robotic arm controlled via neuronal ensemble spiking activity.
A follow-up clinical trial of the BrainGate system, dubbed BrainGate2, showed how participants from the original BrainGate trial were able to use continuous neuronal ensemble control to operate a robotic arm for 3D reach-and-grasp movements. Using the BCI system, one participant with tetraplegia was able to manipulate and drink from a bottle without assistance for the first time in 14 years. The results from this work were achieved in participants whose intracortical arrays were implanted more than 5 years prior, which is promising evidence that the development of neural interfaces using chronically implanted intracortical sensors may be feasible.
Independent of the BrainGate trials, Collinger et al. demonstrated how a human with tetraplegia could control a 7-DoF prosthetic arm using an intracortical activity recorded from two 96-channel arrays implanted in the motor cortex. Continuous control was achieved by relating neural firing rates and movement velocity in each control dimension (3D translation, 3D orientation, 1D grasp) using a linear model, as previously demonstrated in nonhuman primate studies. The participant was able to achieve clinically significant improvements of function, as well as complex, coordinated movements, on par with that of able-bodied people, sustained over several weeks of testing. Fig. 10.7 shows the participant feeding herself using the robotic arm.
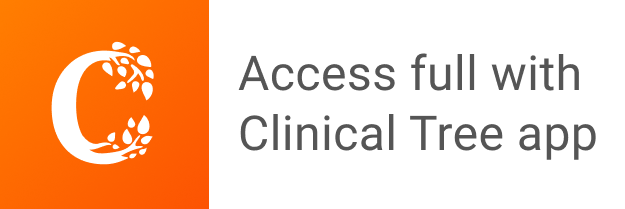