Abstract
The idea of using brain computer interfaces (BCI) for rehabilitation emerged relatively recently. Basically, BCI for neurorehabilitation involves the recording and decoding of local brain signals generated by the patient, as he/her tries to perform a particular task (even if imperfect), or during a mental imagery task. The main objective is to promote the recruitment of selected brain areas involved and to facilitate neural plasticity. The recorded signal can be used in several ways: (i) to objectify and strengthen motor imagery-based training, by providing the patient feedback on the imagined motor task, for example, in a virtual environment; (ii) to generate a desired motor task via functional electrical stimulation or rehabilitative robotic orthoses attached to the patient’s limb – encouraging and optimizing task execution as well as “closing” the disrupted sensorimotor loop by giving the patient the appropriate sensory feedback; (iii) to understand cerebral reorganizations after lesion, in order to influence or even quantify plasticity-induced changes in brain networks. For example, applying cerebral stimulation to re-equilibrate inter-hemispheric imbalance as shown by functional recording of brain activity during movement may help recovery. Its potential usefulness for a patient population has been demonstrated on various levels and its diverseness in interface applications makes it adaptable to a large population. The position and status of these very new rehabilitation systems should now be considered with respect to our current and more or less validated traditional methods, as well as in the light of the wide range of possible brain damage. The heterogeneity in post-damage expression inevitably complicates the decoding of brain signals and thus their use in pathological conditions, asking for controlled clinical trials.
1
Introduction
Sensorimotor rehabilitation forms an important part of the care provided after brain injury, aiming to restore the frequent loss of motor control and increase independence and quality of life. Stroke is the leading cause of acquired disability in adults, and as such occupies a special place amongst the different types of brain injuries. For those people surviving a stroke, up to 80% are left with a residual deficit in fine motor control of the upper limb .
In light of the heterogeneity of symptom expression post-stroke, a large toolbox of training-oriented rehabilitation techniques has been developed. The majority of these approaches are based on theories of motor learning which assume that (a) motor re-learning is comparable to motor learning and (b) patients can learn . The most fundamental law of motor learning is “practice”, encompassing skill acquisition, motor adaptation, and decision-making. Key-features of successful practice are high numbers of repetitions, high intensity, sensory priming, variable practice, and last but not least the provision of feedback . Feedback facilitates the detailed appraisal of performance: it enforces the sensorial aspect in the sensorimotor loop. By highlighting the important features to the patient, it enhances active engagement as well as motivation. The latter is especially important, as no therapy will be effective when there is a lack of motivation to practice .
However, most of the common rehabilitation tools require a residual level of motor control to actually perform the required therapeutic tasks in order to have something to provide feedback on. For patients with severe deficits (little or no movement control), the current toolbox might therefore not be sufficient. Here, brain computer interfaces (BCI) hold promise for filling this gap. BCI records and decodes brain activity while performing or trying to perform motor and/or cognitive tasks. The BCI can therefore be configured such that it maps the decoded brain signals onto useful feedback on the performed task for both patient and therapist. This feedback can take many forms, including those of a visual, auditory or haptic nature. The decoded signal can even be used for the control of external devices that executed the intended movement, providing proprioceptive feedback. Consequently, one can consider BCI as a form of rehabilitation technology, which extends therapeutic possibilities to all patients regardless of the severity of paresis. When normal motor function is lost, the BCI may promote the recruitment of brain areas involved in the particular task, inducing neural plasticity required for recovery of function.
2
Neural plasticity
Neural plasticity is the ability of our nervous system to reorganize its structure, function and connections in response to training. The type and extent of neural plasticity is task-specific, highly time-sensitive and strongly influenced by environmental factors as well as motivation and attention . By providing feedback on the intended movement and thereby restoring the “action–perception coupling”, BCI have already been shown to induce neural plasticity . However, we know that not all plasticity is necessarily beneficial. Examples of maladaptive plasticity post-stroke are abnormal and non-functional movements, like syncinesia, chronic shoulder pain or new onset epilepsy . The goal of neurorehabilitation is therefore to simultaneously improve behaviour by driving adaptive changes in dysfunctional neuronal system, whilst avoiding maladaptive plasticity through carefully designed exercises in combination with (neuro)feedback.
The processes of learning how to operate a BCI device depend on the existence of neural plasticity and are suspected to follow the similar principles as conventional learning processes (Daly et al., 2008). Various studies have shown that we are not only able to reorganize brain connections, but also to modulate our brain activity by training. Especially this modulating brain activity is used to control BCI. Wolpaw et al. demonstrated in 1991 that humans are able to control a cursor on a computer screen by modulating the sensorimotor rhythm amplitude, in the absence of actual movement or sensation . More recently, Ramos-Murguialday showed that patients post-stroke are able to learn how to control the sensorimotor rhythm desynchronization, especially when contingent feedback is provided .
2
Neural plasticity
Neural plasticity is the ability of our nervous system to reorganize its structure, function and connections in response to training. The type and extent of neural plasticity is task-specific, highly time-sensitive and strongly influenced by environmental factors as well as motivation and attention . By providing feedback on the intended movement and thereby restoring the “action–perception coupling”, BCI have already been shown to induce neural plasticity . However, we know that not all plasticity is necessarily beneficial. Examples of maladaptive plasticity post-stroke are abnormal and non-functional movements, like syncinesia, chronic shoulder pain or new onset epilepsy . The goal of neurorehabilitation is therefore to simultaneously improve behaviour by driving adaptive changes in dysfunctional neuronal system, whilst avoiding maladaptive plasticity through carefully designed exercises in combination with (neuro)feedback.
The processes of learning how to operate a BCI device depend on the existence of neural plasticity and are suspected to follow the similar principles as conventional learning processes (Daly et al., 2008). Various studies have shown that we are not only able to reorganize brain connections, but also to modulate our brain activity by training. Especially this modulating brain activity is used to control BCI. Wolpaw et al. demonstrated in 1991 that humans are able to control a cursor on a computer screen by modulating the sensorimotor rhythm amplitude, in the absence of actual movement or sensation . More recently, Ramos-Murguialday showed that patients post-stroke are able to learn how to control the sensorimotor rhythm desynchronization, especially when contingent feedback is provided .
3
Brain computer interfaces
Brain computer interfaces translate, as described above, the patterns of local brain activation into a desired action, when our motor system is lacking the capacity to perform the action. It forms a bridge between our desired or imagined movements and reality. The common aim of BCI systems post-brain injury is to restore the lost motor function by helping the patient learn to produce normal brain activity and/or to use the brain activity to operate training devices. By doing so, BCI integrates a bottom-up (inducing changes at the neural level by acting on the periphery of the body) with top-down (neurological intervention to alter peripheral behaviour) approaches.
Currently, there are several non-invasive methods available for the recording of brain activity in a way that is useful for BCI applications. These methods include electroencepahlography (EEG) , magnetoenchephalography (MEG) , functional near-infrared spectroscopy (fNRIS) and functional magnetic resonance imaging (fMRI) . The selection of methods is mainly dependent on the trade-off between ease of use, resolution of states, and cost of the device. Based on their relative portable nature and low costs, EEG and fNIRS seem to be the best potential candidates for usage in post-brain injury rehabilitation.
Beyond the choice of measurement modality used for recording brain activity, there are numerous signal features, which have the capacity to convey information regarding underlying brain dynamics. Frequently used features in EEG, for example include the amplitudes of a particular evoked potential (e.g. P300), the composition of slow cortical potentials (SCP) or spectral features, such as the (de)synchronisation of the sensorimotor rhythm (SMR). The latter especially has been used in motor rehabilitation due to its robust association with motor area activation during real and imagined movement, a fact exploited in the exploration of the recovering stroke-damaged brain . When there is no motor activity – the sensorimotor brain areas are at rest or inhibited – the amplitude of the SMR is high (SMR synchronisation), reflecting an “idling” state. When motor information is processed, a decrease of SMR amplitude can be observed (SMR desynchronisation). These pattern changes in SMR amplitude can be used to trigger an external device in order to display real-time sensory feedback or to execute the intended action.
In the following sections, we will focus on several applications of BCI systems to restore motor function in three domains:
- •
real-time neurobiological feedback during motor imagery;
- •
representation of the performed action in virtual realities;
- •
activation of external devices inducing actual movement by means of an orthosis or by functional electrical stimulation (FES).
4
BCI and motor imagery
Motor imagery (MI) can be described as a dynamic state during which a subject mentally repeats a specific movement (sequence), without any overt motor output . It shares many of the same neural mechanisms with actual movement execution, with an emphasis on the prefrontal cortex, which is responsible for the creation and maintenance of an explicit representation used in thought and action . MI can be either kinaesthetic (you “feel” the movement in your mind) or visual (you “see” the movement in your mind) from an internal (first person) perspective or an external (third person) perspective, each of which are linked with different neuronal subsystems that can be activated simultaneously and seem inherently tied to each other . For motor recovery, an internal kinaesthetic imagery seems optimal as it activates the motor network of the brain . However, before the development of BCI systems, it was difficult for a therapist to monitor whether patients were indeed performing kinaesthetic MI. By coupling MI to BCI, MI can be visualized, providing feedback to both the patient and the therapist on the strategy used, thus, ameliorating both motor learning and therapy engagement alongside.
Prasad et al. in 2010 integrated an EEG-based BCI for MI within a rehabilitation protocol combining physical practice with MI. The MI consisted of imagining the performance of motor sequences and kinaesthetic sensations associated with it, while holding the upper limb still. In each training session participants (5 chronic patients post-stroke) first performed/tried to execute the movement physically, followed by the MI of the same movement. This was done for the non-impaired and impaired upper limb respectively. The neurofeedback of MI performance was provided by means of a simple visual representation called the “ball-basket” game, in which a ball falls with constant speed from the top of the screen. The ball has to be placed in a target basket appearing on either the left or the right bottom of the screen, using MI of the respective limb movement. The trajectory of the ball was defined as a result of the patient’s MI, confirming patient engagement online. After six weeks of training, with two sessions per week, all participants tended to improve their motor function of the impaired arm around the minimally clinical important difference on the Action Research Arm Test. Therefore, the authors concluded that BCI supported MI is a feasible intervention as part of a post-stroke protocol combining both physical practice and MI. However, it still needs to be verified whether the improvement was due to the neurofeedback, as studies with only MI have also shown functional improvements post-stroke .
Mihara et al. attempted to clarify this question in two steps. Using an fNIRS based BCI system, they first asked 21 healthy participants to kinaesthetically imagine finger flexion and extension alternated with rest periods. The associated brain activity was visualized by means of a vertical bar. Participants were told that the bar was higher when they achieved good kinaesthetic MI during the task period and were more relaxed during rest periods. One group received “relevant feedback” that was contingent with the MI task – reflecting the true kinaesthetic imagery performance. The other group received “sham-feedback”, meaning that the size of the bar was unrelated to the brain activity measured. Contingent and thus relevant neurofeedback induced significantly greater activation of the contralateral premotor cortex as well as higher self-assessment scores for kinaesthetic motor imagery. The premotor cortex is crucial for both motor control and the generation of MI . In contrast, sham-feedback was related to activations of the parietal association cortex. The parietal association cortex is rather linked to memory related visuospatial imagery . These results demonstrate the importance of task contingent feedback when targeting plasticity in the motor network. To verify whether feedback contingent training indeed leads to motor improvement post-stroke, Mihara et al. (2013) subsequently repeated the protocol in a training design with 20 patients post-subcortical stroke. Participants received 6 training sessions with BCI driven MI of distal upper limb control in addition to standard rehabilitation. Patients were randomly allocated to a contingent or a sham-feedback based group. As expected, the cortical activation of the premotor area in relation to MI was greater in the contingent feedback group. Also, it was associated with a greater functional gain on the hand/finger subscale of the Fugl–Meyer Assessment; even in participants with severe motor deficits. Together these results clearly indicate that only contingent feedback on kinaesthetic motor imagery leads to activations in the targeted motor regions. It facilitates adaptive neural plasticity to improve motor functioning post-stroke, emphasizing the promise of BCI-MI as a rehabilitation strategy post-stroke.
5
BCI and motor imagery in conjunction with virtual reality environments
In contrast to the simple visual representation described above, virtual reality (VR) representations allow for three-dimensional (3D) feedback of MI. The complexity of this 3D environment can thereby vary from very simple to highly complex. With 3D representation, a deeper immersion in the virtual environment is suspected, which on its turn increases the motivation and potentially the engagement of the patient-player. While playing, the therapeutic aspect becomes less evident and motor learning becomes more intrinsic with the attention focussed on exploring the environment rather than on training/imagining paretic movement . Leeb et al. proposed a BCI whereby the control signal is used to navigate within a virtual environment, by turning left (left hand MI) or right (right hand MI). In their pilot study, they demonstrated the importance of virtual feedback being as real as possible. For example, when the turning angles in the virtual environment were too large compared to physical capacities, subjects lost the feeling of performing real movements. Based on these results, the researchers here emphasized that feedback that behaves in an unknown or unpredicted way could disturb the focused attention and interfere with the sense of immersion provided, decreasing the motor learning potential .
Subsequently, knowing that people post-stroke are able to perceive motor characteristics in virtual environments , VR also opens doors to observational learning of performed movement. It is well established that the observation of movement activates, just like imagery does, the same neural networks as during overt movement execution . The movements perceived are thought to be mapped onto the observers’ motor repertory, inducing “motor resonance” . The motor resonance following observation may thus facilitate plasticity when the observed action is directly matching the internal simulated action. demonstrated by combining observation of daily actions with concomitant physical training of the observed actions, that stroke patients are also able to learn from action–observation and that it had a positive impact on rehabilitation of motor deficits post-stroke.
A first step towards applying action–observation in BCI has been made by Holper et al. , who observed brain activity during coupled imagery and observation: participants had to imagine that a movement presented in VR was their own movement. The VR presentation was from a first person perspective – as if the participant was watching their own movements. This double condition led to stronger brain activations in the sensorimotor network. The next step envisaged for that work is to control the movement shown in the virtual environment based on the brain activity measured with the BCI system, increasing the coupling between imagination, observation and back to imagination .
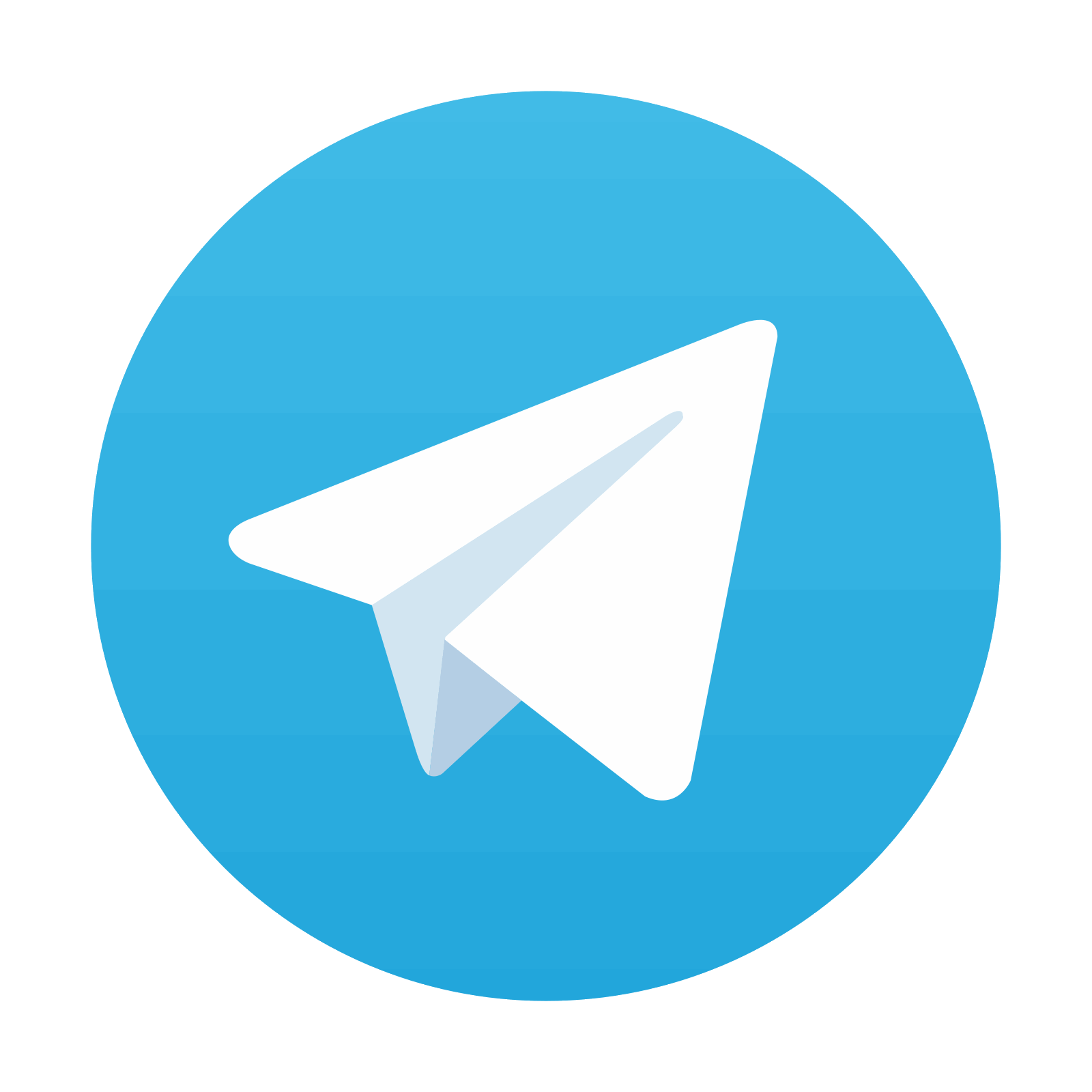
Stay updated, free articles. Join our Telegram channel

Full access? Get Clinical Tree
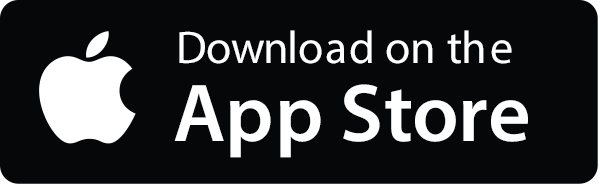
