This article explores how integration of data from clinical registries and electronic health records produces a quality impact within orthopedic practices. Data are differentiated from information, and several types of data that are collected and used in orthopedic outcome measurement are defined. Furthermore, the concept of comparative effectiveness and its impact on orthopedic clinical research are assessed. This article places emphasis on how the concept of big data produces health care challenges balanced with benefits that may be faced by patients and orthopedic surgeons. Finally, essential characteristics of an electronic health record that interlinks musculoskeletal care and big data initiatives are reviewed.
On selection of electronic health record (EHR) systems, orthopedic surgeons must consider the capability of an EHR to ensure data quality, interoperability, and assistance at point of musculoskeletal care.
On selection of electronic health record (EHR) systems, orthopedic surgeons must consider the capability of an EHR to ensure data quality, interoperability, and assistance at point of musculoskeletal care.
Another challenge faced by orthopedic surgeons is that big data is statistically lagged and may represent findings from time periods that are outdated. Several observational studies assess outcome analysis from select time frames that are restricted by the type of data set used. For example, a study Westermann and colleagues assessed the epidemiology of reverse shoulder arthroplasty in the United States using National Inpatient Sample. The study was inherently limited by coding practices in that before 2011 total shoulder arthroplasty and reverse shoulder arthroplasty were coded identically; however, after 2011 separate codes were formed for each procedure. Therefore, any study assessing shoulder arthroplasty dating before 2011 must be closely assessed by practicing surgeons. Seldom does big data information represent anything in real-time and therefore must be thoroughly investigated before application in clinical settings.
References
- 1. Mathias B., Lipori G., Moldawer L.L., et al: Integrating “big data” into surgical practice. Surgery 2015; 159: pp. 371-374
- 2. Fernandes L., O’Connor M., and Weaver V.: Big data, bigger outcomes: healthcare is embracing the big data movement, hoping to revolutionize HIM by distilling vast collection of data for specific analysis. J AHIMA 2012; 83: pp. 38-43
- 3. Feldman B, Martin EM, Skotnes T. Big data in healthcare hype and hope 2012. Available at: http://www.west-info.eu/files/big-data-in-healthcare.pdf. Accessed November 6, 2015.
- 4. Perry D.C., Parsons N., and Costa M.L.: ‘Big data’ reporting guidelines: how to answer big questions, yet avoid big problems. Bone Joint J 2014; 96-b: pp. 1575-1577
- 5. Wasser T., Haynes K., Barron J., et al: Using ‘big data’ to validate claims made in the pharmaceutical approval process. J Med Econ 2015; 18: pp. 1013-1019
- 6. Berger M.L., and Doban V.: Big data, advanced analytics and the future of comparative effectiveness research. J Comp Eff Res 2014; 3: pp. 167-176
- 7. Drowning in big data? Reducing information technology complexities and costs for healthcare organizations. Frost and Sullivan – website. Available at: http://www.emc.com/campaign/global/healthcare/reduce-health-it-costs-complexity.htm. Accessed November 6, 2015.
- 8. Association AM. An inventory of national clinical registries 2014. Available at: http://www.abms.org/media/1356/nqrn-registry-inventory.pdf. Accessed November 6, 2015.
- 9. Porter M.E., and Teisberg E.O.: Redefining competition in health care. Harv Bus Rev 2004; 82: pp. 64-76
- 10. Marjoua Y., Butler C.A., and Bozic K.J.: Public reporting of cost and quality information in orthopaedics. Clin Orthop Relat Res 2012; 470: pp. 1017-1026
- 11. Saleh K.J., Bozic K.J., Graham D.B., et al: Quality in orthopaedic surgery: an international perspective. AOA critical issues. J Bone Joint Surg Am 2013; 95: pp. e3
- 12. Dranove D., Kessler D., McClellan M., et al: Is more information better? The effects of ‘report cards’ on health care providers. J Polit Econ 2003; 111: pp. 555-588
- 13. Zins C.: Conceptual approaches for defining data, information, and knowledge. J Am Soc Inf Sci Technol 2007; 58: pp. 479-493
- 14. Pugely A.J., Martin C.T., Harwood J., et al: Database and registry research in orthopaedic surgery. Part I: claims-based data. J Bone Joint Surg Am 2015; 97: pp. 1278-1287
- 15. Pugely A.J., Martin C.T., Harwood J., et al: Database and registry research in orthopaedic surgery. Part 2: clinical registry data. J Bone Joint Surg Am 2015; 97: pp. 1799-1808
- 16. Ayers D.C., Zheng H., and Franklin P.D.: Integrating patient-reported outcomes into orthopaedic clinical practice: proof of concept from FORCE-TJR. Clin Orthop Relat Res 2013; 471: pp. 3419-3425
- 17. Franklin P.D., Harrold L., and Ayers D.C.: Incorporating patient-reported outcomes in total joint arthroplasty registries: challenges and opportunities. Clin Orthop Relat Res 2013; 471: pp. 3482-3488
- 18. Mushlin A.I., and Ghomrawi H.M.: Comparative effectiveness research: a cornerstone of healthcare reform? Trans Am Clin Climatol Assoc 2010; 121: pp. 141-154
- 19. Institute of Medicine : Initial national priorities for comparative effectiveness research. Washington, DC: The National Academic Press, 2009.
- 20. O’Connor D.P., and Brinker M.R.: Challenges in outcome measurement: clinical research perspective. Clin Orthop Relat Res 2013; 471: pp. 3496-3503
- 21. Noble P.C., Fuller-Lafreniere S., Meftah M., et al: Challenges in outcome measurement: discrepancies between patient and provider definitions of success. Clin Orthop Relat Res 2013; 471: pp. 3437-3445
- 22. Franklin P.D., Allison J.J., and Ayers D.C.: Beyond joint implant registries: a patient-centered research consortium for comparative effectiveness in total joint replacement. JAMA 2012; 308: pp. 1217-1218
- 23. Weiskopf N.G., and Weng C.: Methods and dimensions of electronic health record data quality assessment: enabling reuse for clinical research. J Am Med Inform Assoc 2013; 20: pp. 144-151
- 24. Weng C., Appelbaum P., Hripcsak G., et al: Using EHRs to integrate research with patient care: promises and challenges. J Am Med Inform Assoc 2012; 19: pp. 684-687
- 25. Barrack R.L.: The results of TKA: what the registries don’t tell us. Orthopedics 2011; 34: pp. e485-e487
- 26. Little R.J., Cohen M.L., Dickersin K., et al: The design and conduct of clinical trials to limit missing data. Stat Med 2012; 31: pp. 3433-3443
- 27. Wood A.M., White I.R., and Thompson S.G.: Are missing outcome data adequately handled? A review of published randomized controlled trials in major medical journals. Clin Trials 2004; 1: pp. 368-376
- 28. Singhal R., and Rana R.: Intricacy of missing data in clinical trials: deterrence and management. Int J Appl Basic Med Res 2014; 4: pp. S2-S5
- 29. Louie D.L., Earp B.E., and Blazar P.E.: Finding orthopedic patients lost to follow-up for long-term outcomes research using the Internet: an update for 2012. Orthopedics 2012; 35: pp. 595-599
- 30. Hersh W.R.: Adding value to the electronic health record through secondary use of data for quality assurance, research, and surveillance. Am J Manag Care 2007; 13: pp. 277-278
- 31. Charlson M.E., Pompei P., Ales K.L., et al: A new method of classifying prognostic comorbidity in longitudinal studies: development and validation. J Chronic Dis 1987; 40: pp. 373-383
- 32. Vandenbroucke J.P., von Elm E., Altman D.G., et al: Strengthening the Reporting of Observational Studies in Epidemiology (STROBE): explanation and elaboration. Int J Surg 2014; 12: pp. 1500-1524
- 33. Hoppe D.J., Schemitsch E.H., Morshed S., et al: Hierarchy of evidence: where observational studies fit in and why we need them. J Bone Joint Surg Am 2009; 91: pp. 2-9
- 34. Westermann R.W., Pugely A.J., Martin C.T., et al: Reverse shoulder arthroplasty in the United States: a comparison of national volume, patient demographics, complications, and surgical indications. Iowa Orthop J 2015; 35: pp. 1-7
- 35. Soto M., Capurro D., and Catalan S.: Evaluating the data completeness in the electronic health record after the implementation of an outpatient electronic health record. Stud Health Technol Inform 2015; 216: pp. 885
- 36. Hogan W.R., and Wagner M.M.: Accuracy of data in computer-based patient records. J Am Med Inform Assoc 1997; 4: pp. 342-355
- 37. Chan K.S., Fowles J.B., and Weiner J.P.: Review: electronic health records and the reliability and validity of quality measures: a review of the literature. Med Care Res Rev 2010; 67: pp. 503-527
- 38. Jha A.K., Burke M.F., DesRoches C., et al: Progress toward meaningful use: hospitals’ adoption of electronic health records. Am J Manag Care 2011; 17: pp. Sp117-Sp124
- 39. Wilkonson J. Electronic medical records. 2015. Available at: http://www.aaos.org/AAOSNow/2015/May/managing/managing1/?ssopc=1. Accessed November 6, 2015.
- 40. Balas A., and Al Sanousi A.: Interoperable electronic patient records for health care improvement. Stud Health Technol Inform 2009; 150: pp. 19-23
- 41. Surgeons AAoO. Position statement on electronic health record. 2015. Available at: http://www.aaos.org/CustomTemplates/Content.aspx?id=5619&ssopc=1. Accessed November 6, 2015.
- 42. Rothman B., Leonard J.C., and Vigoda M.M.: Future of electronic health records: implications for decision support. Mount Sinai J Med 2012; 79: pp. 757-768
- 43. Zaouter C., Wehbe M., Cyr S., et al: Use of a decision support system improves the management of hemodynamic and respiratory events in orthopedic patients under propofol sedation and spinal analgesia: a randomized trial. J Clin Monit Comput 2014; 28: pp. 41-47
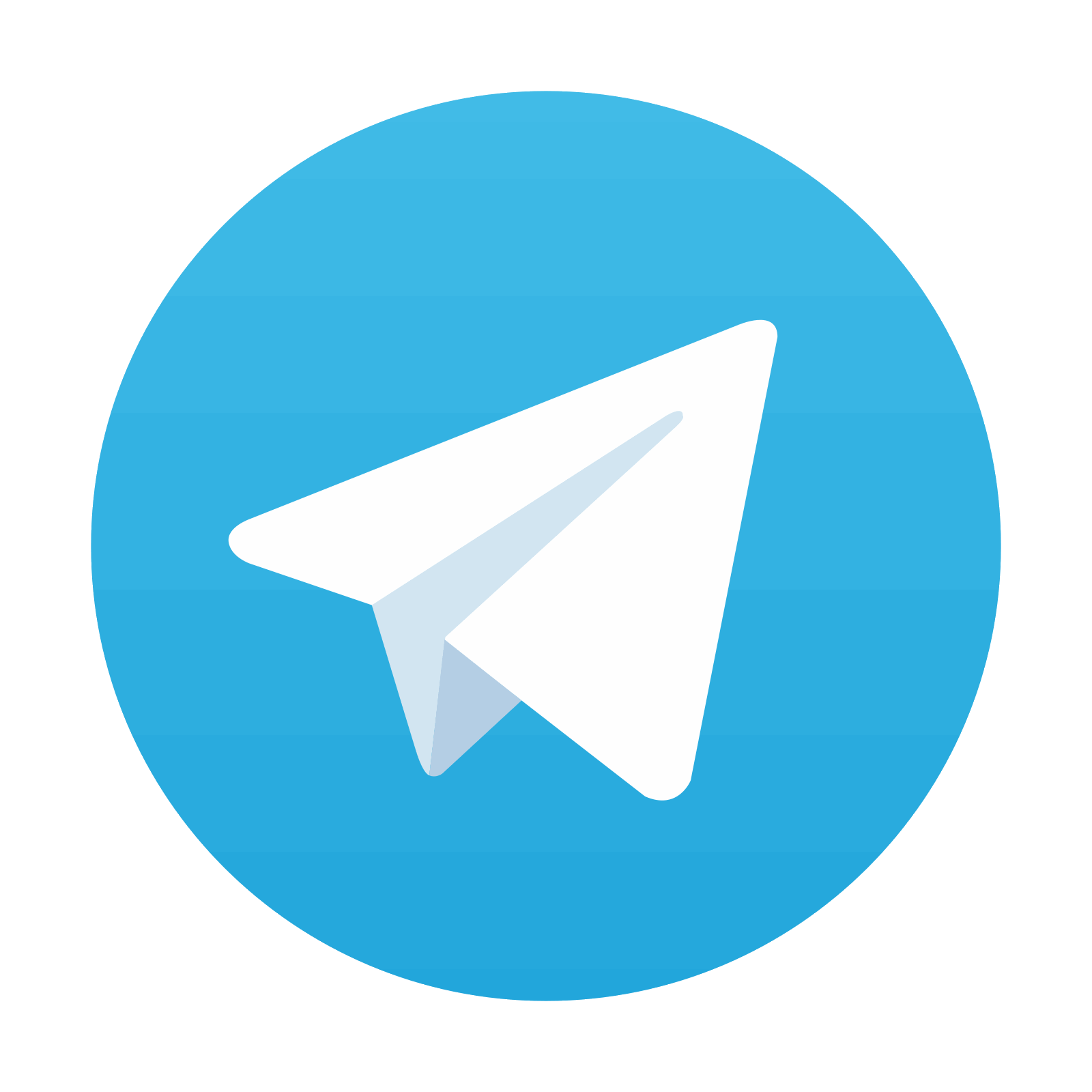
Stay updated, free articles. Join our Telegram channel

Full access? Get Clinical Tree
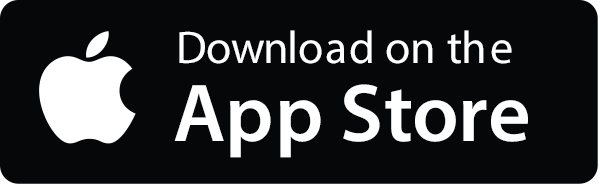
